- AI Collection
- Oxford Thesis Collection
- CC0 version of this metadata

Multi-Modal Deep Learning for Computer Vision and Its Application
Although the exponential growth of visual data in various forms, such as images and videos, enables unprecedented opportunities for us to interpret the surrounding environment, natural language is still the main way to convey knowledge and information among us. Therefore, there is currently an increasing demand for building a framework to achieve interaction between pieces of information from different modalities. In this thesis, I investigate three directions to achieve an effective interac...
Email this record
Please enter the email address that the record information will be sent to.
Please add any additional information to be included within the email.
Cite this record
Chicago style, access document.
- Li_2022_Multi-modal_deep_learning.pdf ( Preview , Dissemination version, pdf, 50.3MB, Terms of use )
Why is the content I wish to access not available via ORA?
Content may be unavailable for the following four reasons.
- Version unsuitable We have not obtained a suitable full-text for a given research output. See the versions advice for more information.
- Recently completed Sometimes content is held in ORA but is unavailable for a fixed period of time to comply with the policies and wishes of rights holders.
- Permissions All content made available in ORA should comply with relevant rights, such as copyright. See the copyright guide for more information.
- Clearance Some thesis volumes scanned as part of the digitisation scheme funded by Dr Leonard Polonsky are currently unavailable due to sensitive material or uncleared third-party copyright content. We are attempting to contact authors whose theses are affected.
Alternative access to the full-text
Request a copy.
We require your email address in order to let you know the outcome of your request.
Provide a statement outlining the basis of your request for the information of the author.
Please note any files released to you as part of your request are subject to the terms and conditions of use for the Oxford University Research Archive unless explicitly stated otherwise by the author.
Contributors
Bibliographic details, item description, terms of use, views and downloads.
If you are the owner of this record, you can report an update to it here: Report update to this record
Report an update
We require your email address in order to let you know the outcome of your enquiry.
PhD Thesis: Geometry and Uncertainty in Deep Learning for Computer Vision
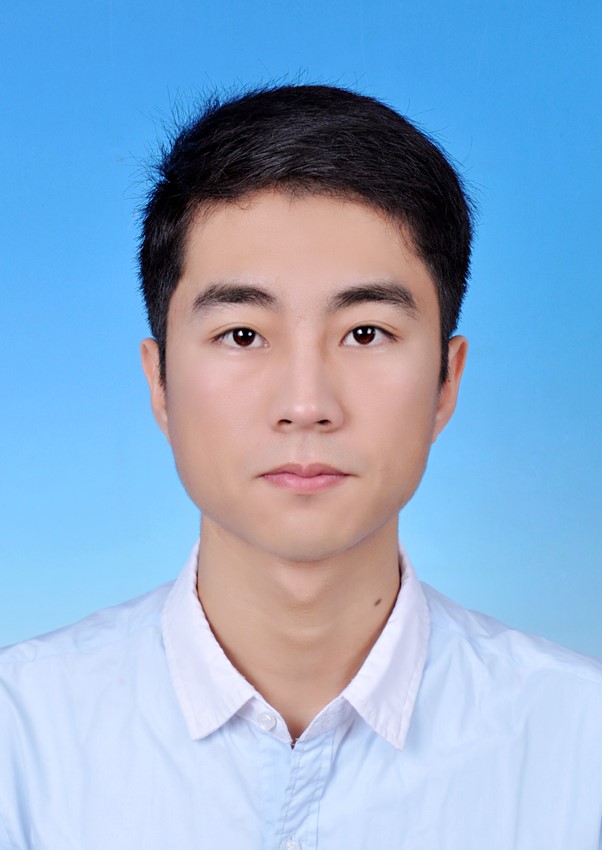
Chaoqiang Zhao
Monocular Depth Estimation, VO
- University of Bologna
- Google Scholar
Today I can share my final PhD thesis, which I submitted in November 2017. It was examined by Dr. Joan Lasenby and Prof. Andrew Zisserman in February 2018 and has just been approved for publication. This thesis presents the main narrative of my research at the University of Cambridge, under the supervision of Prof Roberto Cipolla. It contains 206 pages, 62 figures, 24 tables and 318 citations. You can download the complete .pdf here .
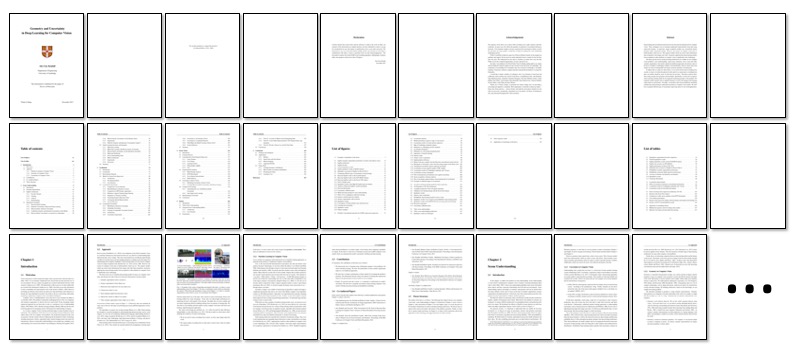
My thesis presents contributions to the field of computer vision, the science which enables machines to see. This blog post introduces the work and tells the story behind this research.
This thesis presents deep learning models for an array of computer vision problems: semantic segmentation , instance segmentation , depth prediction , localisation , stereo vision and video scene understanding .
The abstract
Deep learning and convolutional neural networks have become the dominant tool for computer vision. These techniques excel at learning complicated representations from data using supervised learning. In particular, image recognition models now out-perform human baselines under constrained settings. However, the science of computer vision aims to build machines which can see. This requires models which can extract richer information than recognition, from images and video. In general, applying these deep learning models from recognition to other problems in computer vision is significantly more challenging.
This thesis presents end-to-end deep learning architectures for a number of core computer vision problems; scene understanding, camera pose estimation, stereo vision and video semantic segmentation. Our models outperform traditional approaches and advance state-of-the-art on a number of challenging computer vision benchmarks. However, these end-to-end models are often not interpretable and require enormous quantities of training data.
To address this, we make two observations: (i) we do not need to learn everything from scratch, we know a lot about the physical world, and (ii) we cannot know everything from data, our models should be aware of what they do not know. This thesis explores these ideas using concepts from geometry and uncertainty. Specifically, we show how to improve end-to-end deep learning models by leveraging the underlying geometry of the problem. We explicitly model concepts such as epipolar geometry to learn with unsupervised learning, which improves performance. Secondly, we introduce ideas from probabilistic modelling and Bayesian deep learning to understand uncertainty in computer vision models. We show how to quantify different types of uncertainty, improving safety for real world applications.
I began my PhD in October 2014, joining the controls research group at Cambridge University Engineering Department. Looking back at my original research proposal, I said that I wanted to work on the ‘engineering questions to control autonomous vehicles… in uncertain and challenging environments.’ I spent three months or so reading literature, and quickly developed the opinion that the field of robotics was most limited by perception. If you could obtain a reliable state of the world, control was often simple. However, at this time, computer vision was very fragile in the wild. After many weeks of lobbying Prof. Roberto Cipolla (thanks!), I was able to join his research group in January 2015 and begin a PhD in computer vision.
When I began reading computer vision literature, deep learning had just become popular in image classification, following inspiring breakthroughs on the ImageNet dataset. But it was yet to become ubiquitous in the field and be used in richer computer vision tasks such as scene understanding. What excited me about deep learning was that it could learn representations from data that are too complicated to hand-design.
I initially focused on building end-to-end deep learning models for computer vision tasks which I thought were most interesting for robotics, such as scene understanding (SegNet) and localisation (PoseNet) . However, I quickly realised that, while it was a start, applying end-to-end deep learning wasn’t enough. In my thesis, I argue that we can do better than naive end-to-end convolutional networks. Especially with limited data and compute, we can form more powerful computer vision models by leveraging our knowledge of the world. Specifically, I focus on two ideas around geometry and uncertainty.
- Geometry is all about leveraging structure of the world. This is useful for improving architectures and learning with self-supervision.
- Uncertainty understands what our model doesn’t know. This is useful for robust learning, safety-critical systems and active learning.
Over the last three years, I have had the pleasure of working with some incredibly talented researchers, studying a number of core computer vision problems from localisation to segmentation to stereo vision.
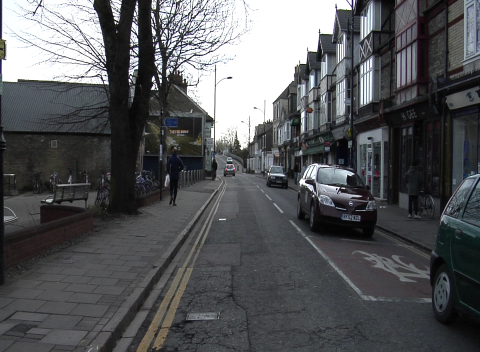
The science
This thesis consists of six chapters. Each of the main chapters introduces an end-to-end deep learning model and discusses how to apply the ideas of geometry and uncertainty.
Chatper 1 - Introduction. Motivates this work within the wider field of computer vision.
Chapter 2 - Scene Understanding. Introduces SegNet, modelling aleatoric and epistemic uncertainty and a method for learning multi-task scene understanding models for geometry and semantics.
Chapter 3 - Localisation. Describes PoseNet for efficient localisation, with improvements using geometric reprojection error and estimating relocalisation uncertainty.
Chapter 4 - Stereo Vision. Designs an end-to-end model for stereo vision, using geometry and shows how to leverage uncertainty and self-supervised learning to improve performance.
Chapter 5 - Video Scene Understanding. Illustrates a video scene understanding model for learning semantics, motion and geometry.
Chapter 6 - Conclusions. Describes limitations of this research and future challenges.
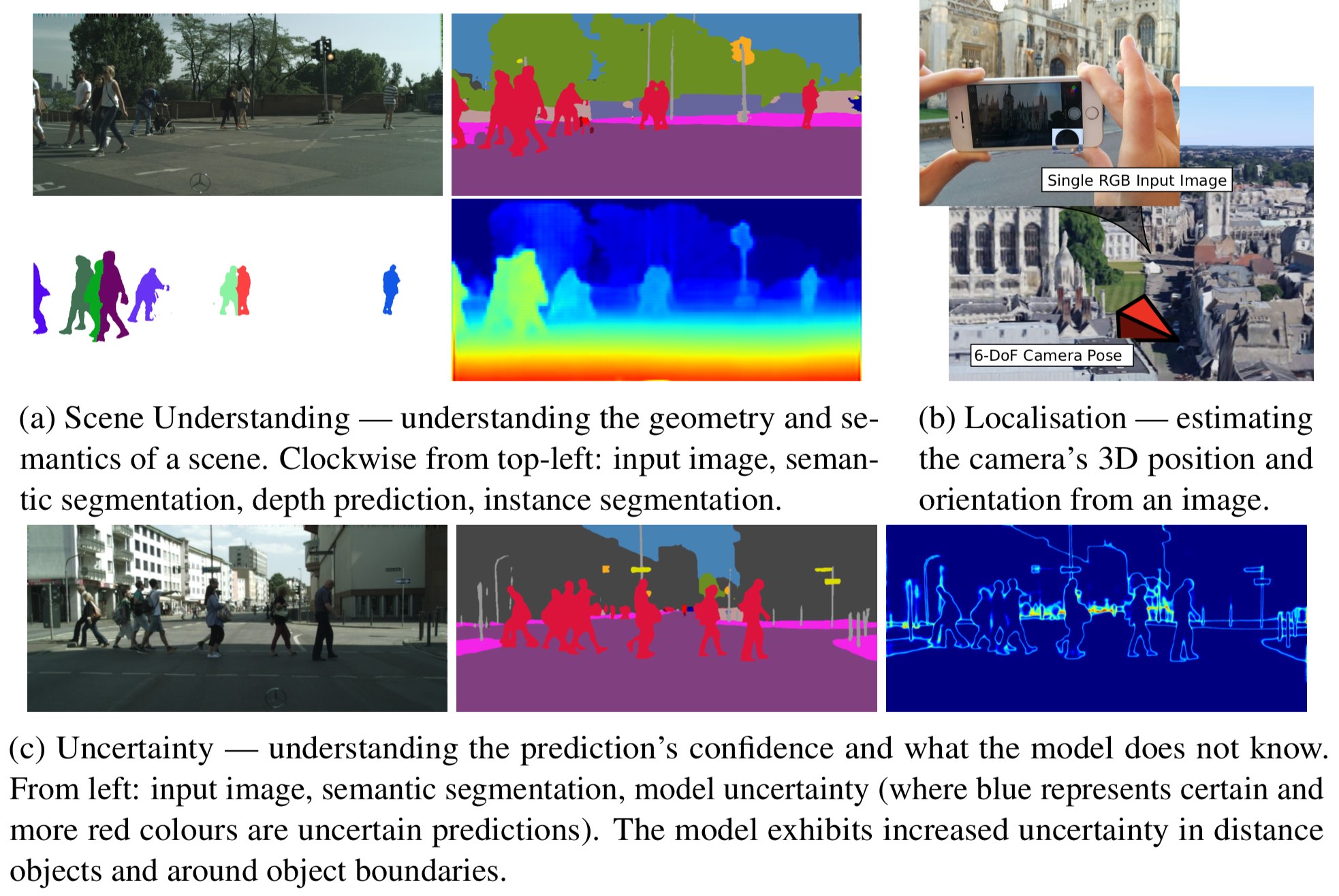
As for what’s next?
This thesis explains how to extract a robust state of the world – semantics, motion and geometry – from video. I’m now excited about applying these ideas to robotics and learning to reason from perception to action. I’m working with an amazing team on autonomous driving, bringing together the worlds of robotics and machine learning. We’re using ideas from computer vision and reinforcement learning to build the most data-efficient self-driving car. And, we’re hiring, come work with me! wayve.ai/careers
I’d like to give a huge thank you to everyone who motivated, distracted and inspired me while writing this thesis.
Here’s the bibtex if you’d like to cite this work.
And the source code for the latex document is here .
- Methods/Theory
- Source Code
- Publications
Ph.D. Theses
Information.
- Contact Info
- Internal Info
- Modeling motion using a simple (rigid) motion model strictly following the principles of perspective projection and segmenting the video into its different motion components by assigning each pixel to its most likely motion model in a Bayesian fashion. [ECCV16]
- Combining piecewise rigid motions to more complex, deformable and articulated objects, guided by learned semantic object segmentations. [CVPR18]
- Learning highly variable motion patterns using a neural network trained on synthetic (unlimited) training data. Training data is automatically generated strictly following the principles of perspective projection. In this way well-known geometric constraints are precisely characterized during training to learn the principles of motion segmentation rather than identifying well-known structures that are likely to move. [ECCV18 workshop]
by Li Yang Ku, May 2018. |
A list of completed theses and new thesis topics from the Computer Vision Group.
Are you about to start a BSc or MSc thesis? Please read our instructions for preparing and delivering your work.
Below we list possible thesis topics for Bachelor and Master students in the areas of Computer Vision, Machine Learning, Deep Learning and Pattern Recognition. The project descriptions leave plenty of room for your own ideas. If you would like to discuss a topic in detail, please contact the supervisor listed below and Prof. Paolo Favaro to schedule a meeting. Note that for MSc students in Computer Science it is required that the official advisor is a professor in CS.
AI deconvolution of light microscopy images
Level: master.
Background Light microscopy became an indispensable tool in life sciences research. Deconvolution is an important image processing step in improving the quality of microscopy images for removing out-of-focus light, higher resolution, and beter signal to noise ratio. Currently classical deconvolution methods, such as regularisation or blind deconvolution, are implemented in numerous commercial software packages and widely used in research. Recently AI deconvolution algorithms have been introduced and being currently actively developed, as they showed a high application potential.
Aim Adaptation of available AI algorithms for deconvolution of microscopy images. Validation of these methods against state-of-the -art commercially available deconvolution software.
Material and Methods Student will implement and further develop available AI deconvolution methods and acquire test microscopy images of different modalities. Performance of developed AI algorithms will be validated against available commercial deconvolution software.

- Al algorithm development and implementation: 50%.
- Data acquisition: 10%.
- Comparison of performance: 40 %.
Requirements
- Interest in imaging.
- Solid knowledge of AI.
- Good programming skills.
Supervisors Paolo Favaro, Guillaume Witz, Yury Belyaev.
Institutes Computer Vison Group, Digital Science Lab, Microscopy imaging Center.
Contact Yury Belyaev, Microscopy imaging Center, [email protected] , + 41 78 899 0110.
Instance segmentation of cryo-ET images
Level: bachelor/master.
In the 1600s, a pioneering Dutch scientist named Antonie van Leeuwenhoek embarked on a remarkable journey that would forever transform our understanding of the natural world. Armed with a simple yet ingenious invention, the light microscope, he delved into uncharted territory, peering through its lens to reveal the hidden wonders of microscopic structures. Fast forward to today, where cryo-electron tomography (cryo-ET) has emerged as a groundbreaking technique, allowing researchers to study proteins within their natural cellular environments. Proteins, functioning as vital nano-machines, play crucial roles in life and understanding their localization and interactions is key to both basic research and disease comprehension. However, cryo-ET images pose challenges due to inherent noise and a scarcity of annotated data for training deep learning models.

Credit: S. Albert et al./PNAS (CC BY 4.0)
To address these challenges, this project aims to develop a self-supervised pipeline utilizing diffusion models for instance segmentation in cryo-ET images. By leveraging the power of diffusion models, which iteratively diffuse information to capture underlying patterns, the pipeline aims to refine and accurately segment cryo-ET images. Self-supervised learning, which relies on unlabeled data, reduces the dependence on extensive manual annotations. Successful implementation of this pipeline could revolutionize the field of structural biology, facilitating the analysis of protein distribution and organization within cellular contexts. Moreover, it has the potential to alleviate the limitations posed by limited annotated data, enabling more efficient extraction of valuable information from cryo-ET images and advancing biomedical applications by enhancing our understanding of protein behavior.
Methods The segmentation pipeline for cryo-electron tomography (cryo-ET) images consists of two stages: training a diffusion model for image generation and training an instance segmentation U-Net using synthetic and real segmentation masks.
1. Diffusion Model Training: a. Data Collection: Collect and curate cryo-ET image datasets from the EMPIAR database (https://www.ebi.ac.uk/empiar/). b. Architecture Design: Select an appropriate architecture for the diffusion model. c. Model Evaluation: Cryo-ET experts will help assess image quality and fidelity through visual inspection and quantitative measures 2. Building the Segmentation dataset: a. Synthetic and real mask generation: Use the trained diffusion model to generate synthetic cryo-ET images. The diffusion process will be seeded from either a real or a synthetic segmentation mask. This will yield to pairs of cryo-ET images and segmentation masks. 3. Instance Segmentation U-Net Training: a. Architecture Design: Choose an appropriate instance segmentation U-Net architecture. b. Model Evaluation: Evaluate the trained U-Net using precision, recall, and F1 score metrics.
By combining the diffusion model for cryo-ET image generation and the instance segmentation U-Net, this pipeline provides an efficient and accurate approach to segment structures in cryo-ET images, facilitating further analysis and interpretation.
References 1. Kwon, Diana. "The secret lives of cells-as never seen before." Nature 598.7882 (2021): 558-560. 2. Moebel, Emmanuel, et al. "Deep learning improves macromolecule identification in 3D cellular cryo-electron tomograms." Nature methods 18.11 (2021): 1386-1394. 3. Rice, Gavin, et al. "TomoTwin: generalized 3D localization of macromolecules in cryo-electron tomograms with structural data mining." Nature Methods (2023): 1-10.
Contacts Prof. Thomas Lemmin Institute of Biochemistry and Molecular Medicine Bühlstrasse 28, 3012 Bern ( [email protected] )
Prof. Paolo Favaro Institute of Computer Science Neubrückstrasse 10 3012 Bern ( [email protected] )
Adding and removing multiple sclerosis lesions with to imaging with diffusion networks
Background multiple sclerosis lesions are the result of demyelination: they appear as dark spots on t1 weighted mri imaging and as bright spots on flair mri imaging. image analysis for ms patients requires both the accurate detection of new and enhancing lesions, and the assessment of atrophy via local thickness and/or volume changes in the cortex. detection of new and growing lesions is possible using deep learning, but made difficult by the relative lack of training data: meanwhile cortical morphometry can be affected by the presence of lesions, meaning that removing lesions prior to morphometry may be more robust. existing ‘lesion filling’ methods are rather crude, yielding unrealistic-appearing brains where the borders of the removed lesions are clearly visible., aim: denoising diffusion networks are the current gold standard in mri image generation [1]: we aim to leverage this technology to remove and add lesions to existing mri images. this will allow us to create realistic synthetic mri images for training and validating ms lesion segmentation algorithms, and for investigating the sensitivity of morphometry software to the presence of ms lesions at a variety of lesion load levels., materials and methods: a large, annotated, heterogeneous dataset of mri data from ms patients, as well as images of healthy controls without white matter lesions, will be available for developing the method. the student will work in a research group with a long track record in applying deep learning methods to neuroimaging data, as well as experience training denoising diffusion networks..
Nature of the Thesis:
Literature review: 10%
Replication of Blob Loss paper: 10%
Implementation of the sliding window metrics:10%
Training on MS lesion segmentation task: 30%
Extension to other datasets: 20%
Results analysis: 20%
Fig. Results of an existing lesion filling algorithm, showing inadequate performance
Requirements:
Interest/Experience with image processing
Python programming knowledge (Pytorch bonus)
Interest in neuroimaging
Supervisor(s):
PD. Dr. Richard McKinley
Institutes: Diagnostic and Interventional Neuroradiology
Center for Artificial Intelligence in Medicine (CAIM), University of Bern
References: [1] Brain Imaging Generation with Latent Diffusion Models , Pinaya et al, Accepted in the Deep Generative Models workshop @ MICCAI 2022 , https://arxiv.org/abs/2209.07162
Contact : PD Dr Richard McKinley, Support Centre for Advanced Neuroimaging ( [email protected] )
Improving metrics and loss functions for targets with imbalanced size: sliding window Dice coefficient and loss.
Background The Dice coefficient is the most commonly used metric for segmentation quality in medical imaging, and a differentiable version of the coefficient is often used as a loss function, in particular for small target classes such as multiple sclerosis lesions. Dice coefficient has the benefit that it is applicable in instances where the target class is in the minority (for example, in case of segmenting small lesions). However, if lesion sizes are mixed, the loss and metric is biased towards performance on large lesions, leading smaller lesions to be missed and harming overall lesion detection. A recently proposed loss function (blob loss[1]) aims to combat this by treating each connected component of a lesion mask separately, and claims improvements over Dice loss on lesion detection scores in a variety of tasks.
Aim: The aim of this thesisis twofold. First, to benchmark blob loss against a simple, potentially superior loss for instance detection: sliding window Dice loss, in which the Dice loss is calculated over a sliding window across the area/volume of the medical image. Second, we will investigate whether a sliding window Dice coefficient is better corellated with lesion-wise detection metrics than Dice coefficient and may serve as an alternative metric capturing both global and instance-wise detection.
Materials and Methods: A large, annotated, heterogeneous dataset of MRI data from MS patients will be available for benchmarking the method, as well as our existing codebases for MS lesion segmentation. Extension of the method to other diseases and datasets (such as covered in the blob loss paper) will make the method more plausible for publication. The student will work alongside clinicians and engineers carrying out research in multiple sclerosis lesion segmentation, in particular in the context of our running project supported by the CAIM grant.

Fig. An annotated MS lesion case, showing the variety of lesion sizes
References: [1] blob loss: instance imbalance aware loss functions for semantic segmentation, Kofler et al, https://arxiv.org/abs/2205.08209
Idempotent and partial skull-stripping in multispectral MRI imaging
Background Skull stripping (or brain extraction) refers to the masking of non-brain tissue from structural MRI imaging. Since 3D MRI sequences allow reconstruction of facial features, many data providers supply data only after skull-stripping, making this a vital tool in data sharing. Furthermore, skull-stripping is an important pre-processing step in many neuroimaging pipelines, even in the deep-learning era: while many methods could now operate on data with skull present, they have been trained only on skull-stripped data and therefore produce spurious results on data with the skull present.
High-quality skull-stripping algorithms based on deep learning are now widely available: the most prominent example is HD-BET [1]. A major downside of HD-BET is its behaviour on datasets to which skull-stripping has already been applied: in this case the algorithm falsely identifies brain tissue as skull and masks it. A skull-stripping algorithm F not exhibiting this behaviour would be idempotent: F(F(x)) = F(x) for any image x. Furthermore, legacy datasets from before the availability of high-quality skull-stripping algorithms may still contain images which have been inadequately skull-stripped: currently the only solution to improve the skull-stripping on this data is to go back to the original datasource or to manually correct the skull-stripping, which is time-consuming and prone to error.
Aim: In this project, the student will develop an idempotent skull-stripping network which can also handle partially skull-stripped inputs. In the best case, the network will operate well on a large subset of the data we work with (e.g. structural MRI, diffusion-weighted MRI, Perfusion-weighted MRI, susceptibility-weighted MRI, at a variety of field strengths) to maximize the future applicability of the network across the teams in our group.
Materials and Methods: Multiple datasets, both publicly available and internal (encompassing thousands of 3D volumes) will be available. Silver standard reference data for standard sequences at 1.5T and 3T can be generated using existing tools such as HD-BET: for other sequences and field strengths semi-supervised learning or methods improving robustness to domain shift may be employed. Robustness to partial skull-stripping may be induced by a combination of learning theory and model-based approaches.

Dataset curation: 10%
Idempotent skull-stripping model building: 30%
Modelling of partial skull-stripping:10%
Extension of model to handle partial skull: 30%
Results analysis: 10%
Fig. An example of failed skull-stripping requiring manual correction
References: [1] Isensee, F, Schell, M, Pflueger, I, et al. Automated brain extraction of multisequence MRI using artificial neural networks. Hum Brain Mapp . 2019; 40: 4952– 4964. https://doi.org/10.1002/hbm.24750
Automated leaf detection and leaf area estimation (for Arabidopsis thaliana)
Correlating plant phenotypes such as leaf area or number of leaves to the genotype (i.e. changes in DNA) is a common goal for plant breeders and molecular biologists. Such data can not only help to understand fundamental processes in nature, but also can help to improve ecotypes, e.g., to perform better under climate change, or reduce fertiliser input. However, collecting data for many plants is very time consuming and automated data acquisition is necessary.
The project aims at building a machine learning model to automatically detect plants in top-view images (see examples below), segment their leaves (see Fig C) and to estimate the leaf area. This information will then be used to determine the leaf area of different Arabidopsis ecotypes. The project will be carried out in collaboration with researchers of the Institute of Plant Sciences at the University of Bern. It will also involve the design and creation of a dataset of plant top-views with the corresponding annotation (provided by experts at the Institute of Plant Sciences).

Contact: Prof. Dr. Paolo Favaro ( [email protected] )
Master Projects at the ARTORG Center
The Gerontechnology and Rehabilitation group at the ARTORG Center for Biomedical Engineering is offering multiple MSc thesis projects to students, which are interested in working with real patient data, artificial intelligence and machine learning algorithms. The goal of these projects is to transfer the findings to the clinic in order to solve today’s healthcare problems and thus to improve the quality of life of patients. Assessment of Digital Biomarkers at Home by Radar. [PDF] Comparison of Radar, Seismograph and Ballistocardiography and to Monitor Sleep at Home. [PDF] Sentimental Analysis in Speech. [PDF] Contact: Dr. Stephan Gerber ( [email protected] )
Internship in Computational Imaging at Prophesee
A 6 month intership at Prophesee, Grenoble is offered to a talented Master Student.
The topic of the internship is working on burst imaging following the work of Sam Hasinoff , and exploring ways to improve it using event-based vision.
A compensation to cover the expenses of living in Grenoble is offered. Only students that have legal rights to work in France can apply.
Anyone interested can send an email with the CV to Daniele Perrone ( [email protected] ).
Using machine learning applied to wearables to predict mental health
This Master’s project lies at the intersection of psychiatry and computer science and aims to use machine learning techniques to improve health. Using sensors to detect sleep and waking behavior has as of yet unexplored potential to reveal insights into health. In this study, we make use of a watch-like device, called an actigraph, which tracks motion to quantify sleep behavior and waking activity. Participants in the study consist of healthy and depressed adolescents and wear actigraphs for a year during which time we query their mental health status monthly using online questionnaires. For this masters thesis we aim to make use of machine learning methods to predict mental health based on the data from the actigraph. The ability to predict mental health crises based on sleep and wake behavior would provide an opportunity for intervention, significantly impacting the lives of patients and their families. This Masters thesis is a collaboration between Professor Paolo Favaro at the Institute of Computer Science ( [email protected] ) and Dr Leila Tarokh at the Universitäre Psychiatrische Dienste (UPD) ( [email protected] ). We are looking for a highly motivated individual interested in bridging disciplines.
Bachelor or Master Projects at the ARTORG Center
The Gerontechnology and Rehabilitation group at the ARTORG Center for Biomedical Engineering is offering multiple BSc- and MSc thesis projects to students, which are interested in working with real patient data, artificial intelligence and machine learning algorithms. The goal of these projects is to transfer the findings to the clinic in order to solve today’s healthcare problems and thus to improve the quality of life of patients. Machine Learning Based Gait-Parameter Extraction by Using Simple Rangefinder Technology. [PDF] Detection of Motion in Video Recordings [PDF] Home-Monitoring of Elderly by Radar [PDF] Gait feature detection in Parkinson's Disease [PDF] Development of an arthroscopic training device using virtual reality [PDF] Contact: Dr. Stephan Gerber ( [email protected] ), Michael Single ( [email protected]. ch )
Dynamic Transformer
Level: bachelor.
Visual Transformers have obtained state of the art classification accuracies [ViT, DeiT, T2T, BoTNet]. Mixture of experts could be used to increase the capacity of a neural network by learning instance dependent execution pathways in a network [MoE]. In this research project we aim to push the transformers to their limit and combine their dynamic attention with MoEs, compared to Switch Transformer [Switch], we will use a much more efficient formulation of mixing [CondConv, DynamicConv] and we will use this idea in the attention part of the transformer, not the fully connected layer.
- Input dependent attention kernel generation for better transformer layers.
Publication Opportunity: Dynamic Neural Networks Meets Computer Vision (a CVPR 2021 Workshop)
Extensions:
- The same idea could be extended to other ViT/Transformer based models [DETR, SETR, LSTR, TrackFormer, BERT]
Related Papers:
- Visual Transformers: Token-based Image Representation and Processing for Computer Vision [ViT]
- DeiT: Data-efficient Image Transformers [DeiT]
- Bottleneck Transformers for Visual Recognition [BoTNet]
- Tokens-to-Token ViT: Training Vision Transformers from Scratch on ImageNet [T2TViT]
- Outrageously Large Neural Networks: The Sparsely-Gated Mixture-of-Experts Layer [MoE]
- Switch Transformers: Scaling to Trillion Parameter Models with Simple and Efficient Sparsity [Switch]
- CondConv: Conditionally Parameterized Convolutions for Efficient Inference [CondConv]
- Dynamic Convolution: Attention over Convolution Kernels [DynamicConv]
- End-to-End Object Detection with Transformers [DETR]
- Rethinking Semantic Segmentation from a Sequence-to-Sequence Perspective with Transformers [SETR]
- End-to-end Lane Shape Prediction with Transformers [LSTR]
- TrackFormer: Multi-Object Tracking with Transformers [TrackFormer]
- BERT: Pre-training of Deep Bidirectional Transformers for Language Understanding [BERT]
Contact: Sepehr Sameni
Visual Transformers have obtained state of the art classification accuracies for 2d images[ViT, DeiT, T2T, BoTNet]. In this project, we aim to extend the same ideas to 3d data (videos), which requires a more efficient attention mechanism [Performer, Axial, Linformer]. In order to accelerate the training process, we could use [Multigrid] technique.
- Better video understanding by attention blocks.
Publication Opportunity: LOVEU (a CVPR workshop) , Holistic Video Understanding (a CVPR workshop) , ActivityNet (a CVPR workshop)
- Rethinking Attention with Performers [Performer]
- Axial Attention in Multidimensional Transformers [Axial]
- Linformer: Self-Attention with Linear Complexity [Linformer]
- A Multigrid Method for Efficiently Training Video Models [Multigrid]
GIRAFFE is a newly introduced GAN that can generate scenes via composition with minimal supervision [GIRAFFE]. Generative methods can implicitly learn interpretable representation as can be seen in GAN image interpretations [GANSpace, GanLatentDiscovery]. Decoding GIRAFFE could give us per-object interpretable representations that could be used for scene manipulation, data augmentation, scene understanding, semantic segmentation, pose estimation [iNeRF], and more.
In order to invert a GIRAFFE model, we will first train the generative model on Clevr and CompCars datasets, then we add a decoder to the pipeline and train this autoencoder. We can make the task easier by knowing the number of objects in the scene and/or knowing their positions.
Goals:
Scene Manipulation and Decomposition by Inverting the GIRAFFE
Publication Opportunity: DynaVis 2021 (a CVPR workshop on Dynamic Scene Reconstruction)
Related Papers:
- GIRAFFE: Representing Scenes as Compositional Generative Neural Feature Fields [GIRAFFE]
- Neural Scene Graphs for Dynamic Scenes
- pixelNeRF: Neural Radiance Fields from One or Few Images [pixelNeRF]
- NeRF: Representing Scenes as Neural Radiance Fields for View Synthesis [NeRF]
- Neural Volume Rendering: NeRF And Beyond
- GANSpace: Discovering Interpretable GAN Controls [GANSpace]
- Unsupervised Discovery of Interpretable Directions in the GAN Latent Space [GanLatentDiscovery]
- Inverting Neural Radiance Fields for Pose Estimation [iNeRF]
Quantized ViT
Visual Transformers have obtained state of the art classification accuracies [ViT, CLIP, DeiT], but the best ViT models are extremely compute heavy and running them even only for inference (not doing backpropagation) is expensive. Running transformers cheaply by quantization is not a new problem and it has been tackled before for BERT [BERT] in NLP [Q-BERT, Q8BERT, TernaryBERT, BinaryBERT]. In this project we will be trying to quantize pretrained ViT models.
Quantizing ViT models for faster inference and smaller models without losing accuracy
Publication Opportunity: Binary Networks for Computer Vision 2021 (a CVPR workshop)
Extensions:
- Having a fast pipeline for image inference with ViT will allow us to dig deep into the attention of ViT and analyze it, we might be able to prune some attention heads or replace them with static patterns (like local convolution or dilated patterns), We might be even able to replace the transformer with performer and increase the throughput even more [Performer].
- The same idea could be extended to other ViT based models [DETR, SETR, LSTR, TrackFormer, CPTR, BoTNet, T2TViT]
- Learning Transferable Visual Models From Natural Language Supervision [CLIP]
- Visual Transformers: Token-based Image Representation and Processing for Computer Vision [ViT]
- DeiT: Data-efficient Image Transformers [DeiT]
- BERT: Pre-training of Deep Bidirectional Transformers for Language Understanding [BERT]
- Q-BERT: Hessian Based Ultra Low Precision Quantization of BERT [Q-BERT]
- Q8BERT: Quantized 8Bit BERT [Q8BERT]
- TernaryBERT: Distillation-aware Ultra-low Bit BERT [TernaryBERT]
- BinaryBERT: Pushing the Limit of BERT Quantization [BinaryBERT]
- Rethinking Attention with Performers [Performer]
- End-to-End Object Detection with Transformers [DETR]
- Rethinking Semantic Segmentation from a Sequence-to-Sequence Perspective with Transformers [SETR]
- End-to-end Lane Shape Prediction with Transformers [LSTR]
- TrackFormer: Multi-Object Tracking with Transformers [TrackFormer]
- CPTR: Full Transformer Network for Image Captioning [CPTR]
- Bottleneck Transformers for Visual Recognition [BoTNet]
- Tokens-to-Token ViT: Training Vision Transformers from Scratch on ImageNet [T2TViT]
Multimodal Contrastive Learning
Recently contrastive learning has gained a lot of attention for self-supervised image representation learning [SimCLR, MoCo]. Contrastive learning could be extended to multimodal data, like videos (images and audio) [CMC, CoCLR]. Most contrastive methods require large batch sizes (or large memory pools) which makes them expensive for training. In this project we are going to use non batch size dependent contrastive methods [SwAV, BYOL, SimSiam] to train multimodal representation extractors.
Our main goal is to compare the proposed method with the CMC baseline, so we will be working with STL10, ImageNet, UCF101, HMDB51, and NYU Depth-V2 datasets.
Inspired by the recent works on smaller datasets [ConVIRT, CPD], to accelerate the training speed, we could start with two pretrained single-modal models and finetune them with the proposed method.
- Extending SwAV to multimodal datasets
- Grasping a better understanding of the BYOL
Publication Opportunity: MULA 2021 (a CVPR workshop on Multimodal Learning and Applications)
- Most knowledge distillation methods for contrastive learners also use large batch sizes (or memory pools) [CRD, SEED], the proposed method could be extended for knowledge distillation.
- One could easily extend this idea to multiview learning, for example one could have two different networks working on the same input and train them with contrastive learning, this may lead to better models [DeiT] by cross-model inductive biases communications.
- Self-supervised Co-training for Video Representation Learning [CoCLR]
- Learning Spatiotemporal Features via Video and Text Pair Discrimination [CPD]
- Audio-Visual Instance Discrimination with Cross-Modal Agreement [AVID-CMA]
- Self-Supervised Learning by Cross-Modal Audio-Video Clustering [XDC]
- Contrastive Multiview Coding [CPC]
- Contrastive Learning of Medical Visual Representations from Paired Images and Text [ConVIRT]
- A Simple Framework for Contrastive Learning of Visual Representations [SimCLR]
- Momentum Contrast for Unsupervised Visual Representation Learning [MoCo]
- Bootstrap your own latent: A new approach to self-supervised Learning [BYOL]
- Exploring Simple Siamese Representation Learning [SimSiam]
- Unsupervised Learning of Visual Features by Contrasting Cluster Assignments [SwAV]
- Contrastive Representation Distillation [CRD]
- SEED: Self-supervised Distillation For Visual Representation [SEED]
Robustness of Neural Networks
Neural Networks have been found to achieve surprising performance in several tasks such as classification, detection and segmentation. However, they are also very sensitive to small (controlled) changes to the input. It has been shown that some changes to an image that are not visible to the naked eye may lead the network to output an incorrect label. This thesis will focus on studying recent progress in this area and aim to build a procedure for a trained network to self-assess its reliability in classification or one of the popular computer vision tasks.
Contact: Paolo Favaro
Masters projects at sitem center
The Personalised Medicine Research Group at the sitem Center for Translational Medicine and Biomedical Entrepreneurship is offering multiple MSc thesis projects to the biomed eng MSc students that may also be of interest to the computer science students. Automated quantification of cartilage quality for hip treatment decision support. PDF Automated quantification of massive rotator cuff tears from MRI. PDF Deep learning-based segmentation and fat fraction analysis of the shoulder muscles using quantitative MRI. PDF Unsupervised Domain Adaption for Cross-Modality Hip Joint Segmentation. PDF Contact: Dr. Kate Gerber
Internships/Master thesis @ Chronocam
3-6 months internships on event-based computer vision. Chronocam is a rapidly growing startup developing event-based technology, with more than 15 PhDs working on problems like tracking, detection, classification, SLAM, etc. Event-based computer vision has the potential to solve many long-standing problems in traditional computer vision, and this is a super exciting time as this potential is becoming more and more tangible in many real-world applications. For next year we are looking for motivated Master and PhD students with good software engineering skills (C++ and/or python), and preferable good computer vision and deep learning background. PhD internships will be more research focused and possibly lead to a publication. For each intern we offer a compensation to cover the expenses of living in Paris. List of some of the topics we want to explore:
- Photo-realistic image synthesis and super-resolution from event-based data (PhD)
- Self-supervised representation learning (PhD)
- End-to-end Feature Learning for Event-based Data
- Bio-inspired Filtering using Spiking Networks
- On-the fly Compression of Event-based Streams for Low-Power IoT Cameras
- Tracking of Multiple Objects with a Dual-Frequency Tracker
- Event-based Autofocus
- Stabilizing an Event-based Stream using an IMU
- Crowd Monitoring for Low-power IoT Cameras
- Road Extraction from an Event-based Camera Mounted in a Car for Autonomous Driving
- Sign detection from an Event-based Camera Mounted in a Car for Autonomous Driving
- High-frequency Eye Tracking
Email with attached CV to Daniele Perrone at [email protected] .
Contact: Daniele Perrone
Object Detection in 3D Point Clouds
Today we have many 3D scanning techniques that allow us to capture the shape and appearance of objects. It is easier than ever to scan real 3D objects and transform them into a digital model for further processing, such as modeling, rendering or animation. However, the output of a 3D scanner is often a raw point cloud with little to no annotations. The unstructured nature of the point cloud representation makes it difficult for processing, e.g. surface reconstruction. One application is the detection and segmentation of an object of interest. In this project, the student is challenged to design a system that takes a point cloud (a 3D scan) as input and outputs the names of objects contained in the scan. This output can then be used to eliminate outliers or points that belong to the background. The approach involves collecting a large dataset of 3D scans and training a neural network on it.
Contact: Adrian Wälchli
Shape Reconstruction from a Single RGB Image or Depth Map
A photograph accurately captures the world in a moment of time and from a specific perspective. Since it is a projection of the 3D space to a 2D image plane, the depth information is lost. Is it possible to restore it, given only a single photograph? In general, the answer is no. This problem is ill-posed, meaning that many different plausible depth maps exist, and there is no way of telling which one is the correct one. However, if we cover one of our eyes, we are still able to recognize objects and estimate how far away they are. This motivates the exploration of an approach where prior knowledge can be leveraged to reduce the ill-posedness of the problem. Such a prior could be learned by a deep neural network, trained with many images and depth maps.
CNN Based Deblurring on Mobile
Deblurring finds many applications in our everyday life. It is particularly useful when taking pictures on handheld devices (e.g. smartphones) where camera shake can degrade important details. Therefore, it is desired to have a good deblurring algorithm implemented directly in the device. In this project, the student will implement and optimize a state-of-the-art deblurring method based on a deep neural network for deployment on mobile phones (Android). The goal is to reduce the number of network weights in order to reduce the memory footprint while preserving the quality of the deblurred images. The result will be a camera app that automatically deblurs the pictures, giving the user a choice of keeping the original or the deblurred image.
Depth from Blur
If an object in front of the camera or the camera itself moves while the aperture is open, the region of motion becomes blurred because the incoming light is accumulated in different positions across the sensor. If there is camera motion, there is also parallax. Thus, a motion blurred image contains depth information. In this project, the student will tackle the problem of recovering a depth-map from a motion-blurred image. This includes the collection of a large dataset of blurred- and sharp images or videos using a pair or triplet of GoPro action cameras. Two cameras will be used in stereo to estimate the depth map, and the third captures the blurred frames. This data is then used to train a convolutional neural network that will predict the depth map from the blurry image.
Unsupervised Clustering Based on Pretext Tasks
The idea of this project is that we have two types of neural networks that work together: There is one network A that assigns images to k clusters and k (simple) networks of type B perform a self-supervised task on those clusters. The goal of all the networks is to make the k networks of type B perform well on the task. The assumption is that clustering in semantically similar groups will help the networks of type B to perform well. This could be done on the MNIST dataset with B being linear classifiers and the task being rotation prediction.
Adversarial Data-Augmentation
The student designs a data augmentation network that transforms training images in such a way that image realism is preserved (e.g. with a constrained spatial transformer network) and the transformed images are more difficult to classify (trained via adversarial loss against an image classifier). The model will be evaluated for different data settings (especially in the low data regime), for example on the MNIST and CIFAR datasets.
Unsupervised Learning of Lip-reading from Videos
People with sensory impairment (hearing, speech, vision) depend heavily on assistive technologies to communicate and navigate in everyday life. The mass production of media content today makes it impossible to manually translate everything into a common language for assistive technologies, e.g. captions or sign language. In this project, the student employs a neural network to learn a representation for lip-movement in videos in an unsupervised fashion, possibly with an encoder-decoder structure where the decoder reconstructs the audio signal. This requires collecting a large dataset of videos (e.g. from YouTube) of speakers or conversations where lip movement is visible. The outcome will be a neural network that learns an audio-visual representation of lip movement in videos, which can then be leveraged to generate captions for hearing impaired persons.
Learning to Generate Topographic Maps from Satellite Images
Satellite images have many applications, e.g. in meteorology, geography, education, cartography and warfare. They are an accurate and detailed depiction of the surface of the earth from above. Although it is relatively simple to collect many satellite images in an automated way, challenges arise when processing them for use in navigation and cartography. The idea of this project is to automatically convert an arbitrary satellite image, of e.g. a city, to a map of simple 2D shapes (streets, houses, forests) and label them with colors (semantic segmentation). The student will collect a dataset of satellite image and topological maps and train a deep neural network that learns to map from one domain to the other. The data could be obtained from a Google Maps database or similar.
Optimization of OmniMotion, a tracking algorithm
Martí farré farrús · june 2024.
This thesis presents Quasi-OmniFastTrack, an improved version of the OmniMotion algorithm for long-term pixel tracking in videos. The key contribution is reducing the computational expense and training time of OmniMotion while maintaining comparable tracking performance. The main bottleneck in OmniMotion was identified to be the NeRF network used for 3D scene representation. Quasi-OmniFastTrack replaces this with a pre-trained depth estimation model, significantly reducing training time, based on the work introduced in OmniFastTrack, hence the name. The invertible neural network for mapping between local and canonical coordinates is retained, but optimized depths are used to lift 2D pixels to 3D. Experiments show that Quasi-OmniFastTrack reduces training time by over 50% compared to OmniMotion while achieving similar qualitative tracking results on sequences with occlusions. Performance degrades somewhat on fast-moving scenes. The ablation studies demonstrate the importance of optimizing the initial depth estimates during training. While not matching OmniMotion's robustness in all scenarios, Quasi-OmniFastTrack offers a compelling speed-accuracy tradeoff, enabling long-term tracking on more videos in practical timeframes. Future work on incorporating other modifications introduced in OmniFastTrack, like long-term semantic features, could further improve tracking consistency.
New Variables of Brain Morphometry: the Potential and Limitations of CNN Regression
Timo blattner · sept. 2022.
The calculation of variables of brain morphology is computationally very expensive and time-consuming. A previous work showed the feasibility of ex- tracting the variables directly from T1-weighted brain MRI images using a con- volutional neural network. We used significantly more data and extended their model to a new set of neuromorphological variables, which could become inter- esting biomarkers in the future for the diagnosis of brain diseases. The model shows for nearly all subjects a less than 5% mean relative absolute error. This high relative accuracy can be attributed to the low morphological variance be- tween subjects and the ability of the model to predict the cortical atrophy age trend. The model however fails to capture all the variance in the data and shows large regional differences. We attribute these limitations in part to the moderate to poor reliability of the ground truth generated by FreeSurfer. We further investigated the effects of training data size and model complexity on this regression task and found that the size of the dataset had a significant impact on performance, while deeper models did not perform better. Lack of interpretability and dependence on a silver ground truth are the main drawbacks of this direct regression approach.
Home Monitoring by Radar
Lars ziegler · sept. 2022.
Detection and tracking of humans via UWB radars is a promising and continuously evolving field with great potential for medical technology. This contactless method of acquiring data of a patients movement patterns is ideal for in home application. As irregularities in a patients movement patterns are an indicator for various health problems including neurodegenerative diseases, the insight this data could provide may enable earlier detection of such problems. In this thesis a signal processing pipeline is presented with which a persons movement is modeled. During an experiment 142 measurements were recorded by two separate radar systems and one lidar system which each consisted of multiple sensors. The models that were calculated on these measurements by the signal processing pipeline were used to predict the times when a person stood up or sat down. The predictions showed an accuracy of 72.2%.
Revisiting non-learning based 3D reconstruction from multiple images
Aaron sägesser · oct. 2021.
Arthroscopy consists of challenging tasks and requires skills that even today, young surgeons still train directly throughout the surgery. Existing simulators are expensive and rarely available. Through the growing potential of virtual reality(VR) (head-mounted) devices for simulation and their applicability in the medical context, these devices have become a promising alternative that would be orders of magnitude cheaper and could be made widely available. To build a VR-based training device for arthroscopy is the overall aim of our project, as this would be of great benefit and might even be applicable in other minimally invasive surgery (MIS). This thesis marks a first step of the project with its focus to explore and compare well-known algorithms in a multi-view stereo (MVS) based 3D reconstruction with respect to imagery acquired by an arthroscopic camera. Simultaneously with this reconstruction, we aim to gain essential measures to compare the VR environment to the real world, as validation of the realism of future VR tasks. We evaluate 3 different feature extraction algorithms with 3 different matching techniques and 2 different algorithms for the estimation of the fundamental (F) matrix. The evaluation of these 18 different setups is made with a reconstruction pipeline embedded in a jupyter notebook implemented in python based on common computer vision libraries and compared with imagery generated with a mobile phone as well as with the reconstruction results of state-of-the-art (SOTA) structure-from-motion (SfM) software COLMAP and Multi-View Environment (MVE). Our comparative analysis manifests the challenges of heavy distortion, the fish-eye shape and weak image quality of arthroscopic imagery, as all results are substantially worse using this data. However, there are huge differences regarding the different setups. Scale Invariant Feature Transform (SIFT) and Oriented FAST Rotated BRIEF (ORB) in combination with k-Nearest Neighbour (kNN) matching and Least Median of Squares (LMedS) present the most promising results. Overall, the 3D reconstruction pipeline is a useful tool to foster the process of gaining measurements from the arthroscopic exploration device and to complement the comparative research in this context.
Examination of Unsupervised Representation Learning by Predicting Image Rotations
Eric lagger · sept. 2020.
In recent years deep convolutional neural networks achieved a lot of progress. To train such a network a lot of data is required and in supervised learning algorithms it is necessary that the data is labeled. To label data there is a lot of human work needed and this takes a lot of time and money to be done. To avoid the inconveniences that come with this we would like to find systems that don’t need labeled data and therefore are unsupervised learning algorithms. This is the importance of unsupervised algorithms, even though their outcome is not yet on the same qualitative level as supervised algorithms. In this thesis we will discuss an approach of such a system and compare the results to other papers. A deep convolutional neural network is trained to learn the rotations that have been applied to a picture. So we take a large amount of images and apply some simple rotations and the task of the network is to discover in which direction the image has been rotated. The data doesn’t need to be labeled to any category or anything else. As long as all the pictures are upside down we hope to find some high dimensional patterns for the network to learn.
StitchNet: Image Stitching using Autoencoders and Deep Convolutional Neural Networks
Maurice rupp · sept. 2019.
This thesis explores the prospect of artificial neural networks for image processing tasks. More specifically, it aims to achieve the goal of stitching multiple overlapping images to form a bigger, panoramic picture. Until now, this task is solely approached with ”classical”, hardcoded algorithms while deep learning is at most used for specific subtasks. This thesis introduces a novel end-to-end neural network approach to image stitching called StitchNet, which uses a pre-trained autoencoder and deep convolutional networks. Additionally to presenting several new datasets for the task of supervised image stitching with each 120’000 training and 5’000 validation samples, this thesis also conducts various experiments with different kinds of existing networks designed for image superresolution and image segmentation adapted to the task of image stitching. StitchNet outperforms most of the adapted networks in both quantitative as well as qualitative results.
Facial Expression Recognition in the Wild
Luca rolshoven · sept. 2019.
The idea of inferring the emotional state of a subject by looking at their face is nothing new. Neither is the idea of automating this process using computers. Researchers used to computationally extract handcrafted features from face images that had proven themselves to be effective and then used machine learning techniques to classify the facial expressions using these features. Recently, there has been a trend towards using deeplearning and especially Convolutional Neural Networks (CNNs) for the classification of these facial expressions. Researchers were able to achieve good results on images that were taken in laboratories under the same or at least similar conditions. However, these models do not perform very well on more arbitrary face images with different head poses and illumination. This thesis aims to show the challenges of Facial Expression Recognition (FER) in this wild setting. It presents the currently used datasets and the present state-of-the-art results on one of the biggest facial expression datasets currently available. The contributions of this thesis are twofold. Firstly, I analyze three famous neural network architectures and their effectiveness on the classification of facial expressions. Secondly, I present two modifications of one of these networks that lead to the proposed STN-COV model. While this model does not outperform all of the current state-of-the-art models, it does beat several ones of them.
A Study of 3D Reconstruction of Varying Objects with Deformable Parts Models
Raoul grossenbacher · july 2019.
This work covers a new approach to 3D reconstruction. In traditional 3D reconstruction one uses multiple images of the same object to calculate a 3D model by taking information gained from the differences between the images, like camera position, illumination of the images, rotation of the object and so on, to compute a point cloud representing the object. The characteristic trait shared by all these approaches is that one can almost change everything about the image, but it is not possible to change the object itself, because one needs to find correspondences between the images. To be able to use different instances of the same object, we used a 3D DPM model that can find different parts of an object in an image, thereby detecting the correspondences between the different pictures, which we then can use to calculate the 3D model. To take this theory to practise, we gave a 3D DPM model, which was trained to detect cars, pictures of different car brands, where no pair of images showed the same vehicle and used the detected correspondences and the Factorization Method to compute the 3D point cloud. This technique leads to a completely new approach in 3D reconstruction, because changing the object itself was never done before.
Motion deblurring in the wild replication and improvements
Alvaro juan lahiguera · jan. 2019, coma outcome prediction with convolutional neural networks, stefan jonas · oct. 2018, automatic correction of self-introduced errors in source code, sven kellenberger · aug. 2018, neural face transfer: training a deep neural network to face-swap, till nikolaus schnabel · july 2018.
This thesis explores the field of artificial neural networks with realistic looking visual outputs. It aims at morphing face pictures of a specific identity to look like another individual by only modifying key features, such as eye color, while leaving identity-independent features unchanged. Prior works have covered the topic of symmetric translation between two specific domains but failed to optimize it on faces where only parts of the image may be changed. This work applies a face masking operation to the output at training time, which forces the image generator to preserve colors while altering the face, fitting it naturally inside the unmorphed surroundings. Various experiments are conducted including an ablation study on the final setting, decreasing the baseline identity switching performance from 81.7% to 75.8 % whilst improving the average χ2 color distance from 0.551 to 0.434. The provided code-based software gives users easy access to apply this neural face swap to images and videos of arbitrary crop and brings Computer Vision one step closer to replacing Computer Graphics in this specific area.
A Study of the Importance of Parts in the Deformable Parts Model
Sammer puran · june 2017, self-similarity as a meta feature, lucas husi · april 2017, a study of 3d deformable parts models for detection and pose-estimation, simon jenni · march 2015, accelerated federated learning on client silos with label noise: rho selection in classification and segmentation, irakli kelbakiani · may 2024.
Federated Learning has recently gained more research interest. This increased attention is caused by factors including the growth of decentralized data, privacy concerns, and new privacy regulations. In Federated Learning, remote servers keep training a model on local datasets independently, and subsequently, local models are aggregated into a global model, which achieves better overall performance. Sending local model weights instead of the entire dataset is a significant advantage of Federated Learning over centralized classical machine learning algorithms. Federated learning involves uploading and downloading model parameters multiple times, so there are multiple communication rounds between the global server and remote client servers, which imposes challenges. The high number of necessary communication rounds not only increases high-cost communication overheads but is also a critical limitation for servers with low network bandwidth, which leads to latency and a higher probability of training failures caused by communication breakdowns. To mitigate these challenges, we aim to provide a fast-convergent Federated Learning training methodology that decreases the number of necessary communication rounds. We found a paper about Reducible Holdout Loss Selection (RHO-Loss) batch selection methodology, which ”selects low-noise, task-relevant, non-redundant points for training” [1]; we hypothesize, if client silos employ RHO-Loss methodology and successfully avoid training their local models on noisy and non-relevant samples, clients may offer stable and consistent updates to the global server, which could lead to faster convergence of the global model. Our contribution focuses on investigating the RHO-Loss method in a simulated federated setting for the Clothing1M dataset. We also examine its applicability to medical datasets and check its effectiveness in a simulated federated environment. Our experimental results show a promising outcome, specifically a reduction in communication rounds for the Clothing1M dataset. However, as the success of the RHO-Loss selection method depends on the availability of sufficient training data for the target RHO model and for the Irreducible RHO model, we emphasize that our contribution applies to those Federated Learning scenarios where client silos hold enough training data to successfully train and benefit from their RHO model on their local dataset.
Amodal Leaf Segmentation
Nicolas maier · nov. 2023.
Plant phenotyping is the process of measuring and analyzing various traits of plants. It provides essential information on how genetic and environmental factors affect plant growth and development. Manual phenotyping is highly time-consuming; therefore, many computer vision and machine learning based methods have been proposed in the past years to perform this task automatically based on images of the plants. However, the publicly available datasets (in particular, of Arabidopsis thaliana) are limited in size and diversity, making them unsuitable to generalize to new unseen environments. In this work, we propose a complete pipeline able to automatically extract traits of interest from an image of Arabidopsis thaliana. Our method uses a minimal amount of existing annotated data from a source domain to generate a large synthetic dataset adapted to a different target domain (e.g., different backgrounds, lighting conditions, and plant layouts). In addition, unlike the source dataset, the synthetic one provides ground-truth annotations for the occluded parts of the leaves, which are relevant when measuring some characteristics of the plant, e.g., its total area. This synthetic dataset is then used to train a model to perform amodal instance segmentation of the leaves to obtain the total area, leaf count, and color of each plant. To validate our approach, we create a small dataset composed of manually annotated real images of Arabidopsis thaliana, which is used to assess the performance of the models.
Assessment of movement and pose in a hospital bed by ambient and wearable sensor technology in healthy subjects
Tony licata · sept. 2022.
The use of automated systems describing the human motion has become possible in various domains. Most of the proposed systems are designed to work with people moving around in a standing position. Because such system could be interesting in a medical environment, we propose in this work a pipeline that can effectively predict human motion from people lying on beds. The proposed pipeline is tested with a data set composed of 41 participants executing 7 predefined tasks in a bed. The motion of the participants is measured with video cameras, accelerometers and pressure mat. Various experiments are carried with the information retrieved from the data set. Two approaches combining the data from the different measure technologies are explored. The performance of the different carried experiments is measured, and the proposed pipeline is composed with components providing the best results. Later on, we show that the proposed pipeline only needs to use the video cameras, which make the proposed environment easier to implement in real life situations.
Machine Learning Based Prediction of Mental Health Using Wearable-measured Time Series
Seyedeh sharareh mirzargar · sept. 2022.
Depression is the second major cause for years spent in disability and has a growing prevalence in adolescents. The recent Covid-19 pandemic has intensified the situation and limited in-person patient monitoring due to distancing measures. Recent advances in wearable devices have made it possible to record the rest/activity cycle remotely with high precision and in real-world contexts. We aim to use machine learning methods to predict an individual's mental health based on wearable-measured sleep and physical activity. Predicting an impending mental health crisis of an adolescent allows for prompt intervention, detection of depression onset or its recursion, and remote monitoring. To achieve this goal, we train three primary forecasting models; linear regression, random forest, and light gradient boosted machine (LightGBM); and two deep learning models; block recurrent neural network (block RNN) and temporal convolutional network (TCN); on Actigraph measurements to forecast mental health in terms of depression, anxiety, sleepiness, stress, sleep quality, and behavioral problems. Our models achieve a high forecasting performance, the random forest being the winner to reach an accuracy of 98% for forecasting the trait anxiety. We perform extensive experiments to evaluate the models' performance in accuracy, generalization, and feature utilization, using a naive forecaster as the baseline. Our analysis shows minimal mental health changes over two months, making the prediction task easily achievable. Due to these minimal changes in mental health, the models tend to primarily use the historical values of mental health evaluation instead of Actigraph features. At the time of this master thesis, the data acquisition step is still in progress. In future work, we plan to train the models on the complete dataset using a longer forecasting horizon to increase the level of mental health changes and perform transfer learning to compensate for the small dataset size. This interdisciplinary project demonstrates the opportunities and challenges in machine learning based prediction of mental health, paving the way toward using the same techniques to forecast other mental disorders such as internalizing disorder, Parkinson's disease, Alzheimer's disease, etc. and improving the quality of life for individuals who have some mental disorder.
CNN Spike Detector: Detection of Spikes in Intracranial EEG using Convolutional Neural Networks
Stefan jonas · oct. 2021.
The detection of interictal epileptiform discharges in the visual analysis of electroencephalography (EEG) is an important but very difficult, tedious, and time-consuming task. There have been decades of research on computer-assisted detection algorithms, most recently focused on using Convolutional Neural Networks (CNNs). In this thesis, we present the CNN Spike Detector, a convolutional neural network to detect spikes in intracranial EEG. Our dataset of 70 intracranial EEG recordings from 26 subjects with epilepsy introduces new challenges in this research field. We report cross-validation results with a mean AUC of 0.926 (+- 0.04), an area under the precision-recall curve (AUPRC) of 0.652 (+- 0.10) and 12.3 (+- 7.47) false positive epochs per minute for a sensitivity of 80%. A visual examination of false positive segments is performed to understand the model behavior leading to a relatively high false detection rate. We notice issues with the evaluation measures and highlight a major limitation of the common approach of detecting spikes using short segments, namely that the network is not capable to consider the greater context of the segment with regards to its origination. For this reason, we present the Context Model, an extension in which the CNN Spike Detector is supplied with additional information about the channel. Results show promising but limited performance improvements. This thesis provides important findings about the spike detection task for intracranial EEG and lays out promising future research directions to develop a network capable of assisting experts in real-world clinical applications.
PolitBERT - Deepfake Detection of American Politicians using Natural Language Processing
Maurice rupp · april 2021.
This thesis explores the application of modern Natural Language Processing techniques to the detection of artificially generated videos of popular American politicians. Instead of focusing on detecting anomalies and artifacts in images and sounds, this thesis focuses on detecting irregularities and inconsistencies in the words themselves, opening up a new possibility to detect fake content. A novel, domain-adapted, pre-trained version of the language model BERT combined with several mechanisms to overcome severe dataset imbalances yielded the best quantitative as well as qualitative results. Additionally to the creation of the biggest publicly available dataset of English-speaking politicians consisting of 1.5 M sentences from over 1000 persons, this thesis conducts various experiments with different kinds of text classification and sequence processing algorithms applied to the political domain. Furthermore, multiple ablations to manage severe data imbalance are presented and evaluated.
A Study on the Inversion of Generative Adversarial Networks
Ramona beck · march 2021.
The desire to use generative adversarial networks (GANs) for real-world tasks such as object segmentation or image manipulation is increasing as synthesis quality improves, which has given rise to an emerging research area called GAN inversion that focuses on exploring methods for embedding real images into the latent space of a GAN. In this work, we investigate different GAN inversion approaches using an existing generative model architecture that takes a completely unsupervised approach to object segmentation and is based on StyleGAN2. In particular, we propose and analyze algorithms for embedding real images into the different latent spaces Z, W, and W+ of StyleGAN following an optimization-based inversion approach, while also investigating a novel approach that allows fine-tuning of the generator during the inversion process. Furthermore, we investigate a hybrid and a learning-based inversion approach, where in the former we train an encoder with embeddings optimized by our best optimization-based inversion approach, and in the latter we define an autoencoder, consisting of an encoder and the generator of our generative model as a decoder, and train it to map an image into the latent space. We demonstrate the effectiveness of our methods as well as their limitations through a quantitative comparison with existing inversion methods and by conducting extensive qualitative and quantitative experiments with synthetic data as well as real images from a complex image dataset. We show that we achieve qualitatively satisfying embeddings in the W and W+ spaces with our optimization-based algorithms, that fine-tuning the generator during the inversion process leads to qualitatively better embeddings in all latent spaces studied, and that the learning-based approach also benefits from a variable generator as well as a pre-training with our hybrid approach. Furthermore, we evaluate our approaches on the object segmentation task and show that both our optimization-based and our hybrid and learning-based methods are able to generate meaningful embeddings that achieve reasonable object segmentations. Overall, our proposed methods illustrate the potential that lies in the GAN inversion and its application to real-world tasks, especially in the relaxed version of the GAN inversion where the weights of the generator are allowed to vary.
Multi-scale Momentum Contrast for Self-supervised Image Classification
Zhao xueqi · dec. 2020.
With the maturity of supervised learning technology, people gradually shift the research focus to the field of self-supervised learning. ”Momentum Contrast” (MoCo) proposes a new self-supervised learning method and raises the correct rate of self-supervised learning to a new level. Inspired by another article ”Representation Learning by Learning to Count”, if a picture is divided into four parts and passed through a neural network, it is possible to further improve the accuracy of MoCo. Different from the original MoCo, this MoCo variant (Multi-scale MoCo) does not directly pass the image through the encoder after the augmented images. Multi-scale MoCo crops and resizes the augmented images, and the obtained four parts are respectively passed through the encoder and then summed (upsampled version do not do resize to input but resize the contrastive samples). This method of images crop is not only used for queue q but also used for comparison queue k, otherwise the weights of queue k might be damaged during the moment update. This will further discussed in the experiments chapter between downsampled Multi-scale version and downsampled both Multi-scale version. Human beings also have the same principle of object recognition: when human beings see something they are familiar with, even if the object is not fully displayed, people can still guess the object itself with a high probability. Because of this, Multi-scale MoCo applies this concept to the pretext part of MoCo, hoping to obtain better feature extraction. In this thesis, there are three versions of Multi-scale MoCo, downsampled input samples version, downsampled input samples and contrast samples version and upsampled input samples version. The differences between these versions will be described in more detail later. The neural network architecture comparison includes ResNet50 , and the tested data set is STL-10. The weights obtained in pretext will be transferred to self-supervised learning, and in the process of self-supervised learning, the weights of other layers except the final linear layer are frozen without changing (these weights come from pretext).
Self-Supervised Learning Using Siamese Networks and Binary Classifier
Dušan mihajlov · march 2020.
In this thesis, we present several approaches for training a convolutional neural network using only unlabeled data. Our autonomously supervised learning algorithms are based on connections between image patch i. e. zoomed image and its original. Using the siamese architecture neural network we aim to recognize, if the image patch, which is input to the first neural network part, comes from the same image presented to the second neural network part. By applying transformations to both images, and different zoom sizes at different positions, we force the network to extract high level features using its convolutional layers. At the top of our siamese architecture, we have a simple binary classifier that measures the difference between feature maps that we extract and makes a decision. Thus, the only way that the classifier will solve the task correctly is when our convolutional layers are extracting useful representations. Those representations we can than use to solve many different tasks that are related to the data used for unsupervised training. As the main benchmark for all of our models, we used STL10 dataset, where we train a linear classifier on the top of our convolutional layers with a small amount of manually labeled images, which is a widely used benchmark for unsupervised learning tasks. We also combine our idea with recent work on the same topic, and the network called RotNet, which makes use of image rotations and therefore forces the network to learn rotation dependent features from the dataset. As a result of this combination we create a new procedure that outperforms original RotNet.
Learning Object Representations by Mixing Scenes
Lukas zbinden · may 2019.
In the digital age of ever increasing data amassment and accessibility, the demand for scalable machine learning models effective at refining the new oil is unprecedented. Unsupervised representation learning methods present a promising approach to exploit this invaluable yet unlabeled digital resource at scale. However, a majority of these approaches focuses on synthetic or simplified datasets of images. What if a method could learn directly from natural Internet-scale image data? In this thesis, we propose a novel approach for unsupervised learning of object representations by mixing natural image scenes. Without any human help, our method mixes visually similar images to synthesize new realistic scenes using adversarial training. In this process the model learns to represent and understand the objects prevalent in natural image data and makes them available for downstream applications. For example, it enables the transfer of objects from one scene to another. Through qualitative experiments on complex image data we show the effectiveness of our method along with its limitations. Moreover, we benchmark our approach quantitatively against state-of-the-art works on the STL-10 dataset. Our proposed method demonstrates the potential that lies in learning representations directly from natural image data and reinforces it as a promising avenue for future research.
Representation Learning using Semantic Distances
Markus roth · may 2019, zero-shot learning using generative adversarial networks, hamed hemati · dec. 2018, dimensionality reduction via cnns - learning the distance between images, ioannis glampedakis · sept. 2018, learning to play othello using deep reinforcement learning and self play, thomas simon steinmann · sept. 2018, aba-j interactive multi-modality tissue sectionto-volume alignment: a brain atlasing toolkit for imagej, felix meyenhofer · march 2018, learning visual odometry with recurrent neural networks, adrian wälchli · feb. 2018.
In computer vision, Visual Odometry is the problem of recovering the camera motion from a video. It is related to Structure from Motion, the problem of reconstructing the 3D geometry from a collection of images. Decades of research in these areas have brought successful algorithms that are used in applications like autonomous navigation, motion capture, augmented reality and others. Despite the success of these prior works in real-world environments, their robustness is highly dependent on manual calibration and the magnitude of noise present in the images in form of, e.g., non-Lambertian surfaces, dynamic motion and other forms of ambiguity. This thesis explores an alternative approach to the Visual Odometry problem via Deep Learning, that is, a specific form of machine learning with artificial neural networks. It describes and focuses on the implementation of a recent work that proposes the use of Recurrent Neural Networks to learn dependencies over time due to the sequential nature of the input. Together with a convolutional neural network that extracts motion features from the input stream, the recurrent part accumulates knowledge from the past to make camera pose estimations at each point in time. An analysis on the performance of this system is carried out on real and synthetic data. The evaluation covers several ways of training the network as well as the impact and limitations of the recurrent connection for Visual Odometry.

Crime location and timing prediction
Bernard swart · jan. 2018, from cartoons to real images: an approach to unsupervised visual representation learning, simon jenni · feb. 2017, automatic and large-scale assessment of fluid in retinal oct volume, nina mujkanovic · dec. 2016, segmentation in 3d using eye-tracking technology, michele wyss · july 2016, accurate scale thresholding via logarithmic total variation prior, remo diethelm · aug. 2014, unsupervised object segmentation with generative models, adam jakub bielski · april 2024.
Advances in computer vision have transformed how we interact with technology, driven by significant breakthroughs in scalable deep learning and the availability of large datasets. These technologies now play a crucial role in various applications, from improving user experience through applications like organizing digital photo libraries, to advancing medical diagnostics and treatments. Despite these valuable applications, the creation of annotated datasets remains a significant bottleneck. It is not only costly and labor-intensive but also prone to inaccuracies and human biases. Moreover, it often requires specialized knowledge or careful handling of sensitive information. Among the tasks in computer vision, image segmentation particularly highlights these challenges, with its need for precise pixel-level annotations. This context underscores the need for unsupervised approaches in computer vision, which can leverage the large volumes of unlabeled images produced every day. This thesis introduces several novel methods for learning fully unsupervised object segmentation models using only collections of images. Unlike much prior work, our approaches are effective on complex real-world images and do not rely on any form of annotations, including pre-trained supervised networks, bounding boxes, or class labels. We identify and leverage intrinsic properties of objects – most notably, the cohesive movement of object parts – as powerful signals for driving unsupervised object segmentation. Utilizing innovative generative adversarial models, we employ this principle to either generate segmented objects or directly segment them in a manner that allows for realistic movement within scenes. Our work demonstrates how such generated data can train a segmentation model that effectively generalizes to realworld images. Furthermore, we introduce a method that, in conjunction with recent advances in self-supervised learning, achieves state-of-the-art results in unsupervised object segmentation. Our methods rely on the effectiveness of Generative Adversarial Networks, which are known to be challenging to train and exhibit mode collapse. We propose a new, more principled GAN loss, whose gradients encourage the generator model to explore missing modes in its distribution, addressing these limitations and enhancing the robustness of generative models.
Novel Techniques for Robust and Generalizable Machine Learning
Abdelhak lemkhenter · sept. 2023.
Neural networks have transcended their status of powerful proof-of-concept machine learning into the realm of a highly disruptive technology that has revolutionized many quantitative fields such as drug discovery, autonomous vehicles, and machine translation. Today, it is nearly impossible to go a single day without interacting with a neural network-powered application. From search engines to on-device photo-processing, neural networks have become the go-to solution thanks to recent advances in computational hardware and an unprecedented scale of training data. Larger and less curated datasets, typically obtained through web crawling, have greatly propelled the capabilities of neural networks forward. However, this increase in scale amplifies certain challenges associated with training such models. Beyond toy or carefully curated datasets, data in the wild is plagued with biases, imbalances, and various noisy components. Given the larger size of modern neural networks, such models run the risk of learning spurious correlations that fail to generalize beyond their training data. This thesis addresses the problem of training more robust and generalizable machine learning models across a wide range of learning paradigms for medical time series and computer vision tasks. The former is a typical example of a low signal-to-noise ratio data modality with a high degree of variability between subjects and datasets. There, we tailor the training scheme to focus on robust patterns that generalize to new subjects and ignore the noisier and subject-specific patterns. To achieve this, we first introduce a physiologically inspired unsupervised training task and then extend it by explicitly optimizing for cross-dataset generalization using meta-learning. In the context of image classification, we address the challenge of training semi-supervised models under class imbalance by designing a novel label refinement strategy with higher local sensitivity to minority class samples while preserving the global data distribution. Lastly, we introduce a new Generative Adversarial Networks training loss. Such generative models could be applied to improve the training of subsequent models in the low data regime by augmenting the dataset using generated samples. Unfortunately, GAN training relies on a delicate balance between its components, making it prone mode collapse. Our contribution consists of defining a more principled GAN loss whose gradients incentivize the generator model to seek out missing modes in its distribution. All in all, this thesis tackles the challenge of training more robust machine learning models that can generalize beyond their training data. This necessitates the development of methods specifically tailored to handle the diverse biases and spurious correlations inherent in the data. It is important to note that achieving greater generalizability in models goes beyond simply increasing the volume of data; it requires meticulous consideration of training objectives and model architecture. By tackling these challenges, this research contributes to advancing the field of machine learning and underscores the significance of thoughtful design in obtaining more resilient and versatile models.
Automated Sleep Scoring, Deep Learning and Physician Supervision
Luigi fiorillo · oct. 2022.
Sleep plays a crucial role in human well-being. Polysomnography is used in sleep medicine as a diagnostic tool, so as to objectively analyze the quality of sleep. Sleep scoring is the procedure of extracting sleep cycle information from the wholenight electrophysiological signals. The scoring is done worldwide by the sleep physicians according to the official American Academy of Sleep Medicine (AASM) scoring manual. In the last decades, a wide variety of deep learning based algorithms have been proposed to automatise the sleep scoring task. In this thesis we study the reasons why these algorithms fail to be introduced in the daily clinical routine, with the perspective of bridging the existing gap between the automatic sleep scoring models and the sleep physicians. In this light, the primary step is the design of a simplified sleep scoring architecture, also providing an estimate of the model uncertainty. Beside achieving results on par with most up-to-date scoring systems, we demonstrate the efficiency of ensemble learning based algorithms, together with label smoothing techniques, in both enhancing the performance and calibrating the simplified scoring model. We introduced an uncertainty estimate procedure, so as to identify the most challenging sleep stage predictions, and to quantify the disagreement between the predictions given by the model and the annotation given by the physicians. In this thesis we also propose a novel method to integrate the inter-scorer variability into the training procedure of a sleep scoring model. We clearly show that a deep learning model is able to encode this variability, so as to better adapt to the consensus of a group of scorers-physicians. We finally address the generalization ability of a deep learning based sleep scoring system, further studying its resilience to the sleep complexity and to the AASM scoring rules. We can state that there is no need to train the algorithm strictly following the AASM guidelines. Most importantly, using data from multiple data centers results in a better performing model compared with training on a single data cohort. The variability among different scorers and data centers needs to be taken into account, more than the variability among sleep disorders.
Learning Representations for Controllable Image Restoration
Givi meishvili · march 2022.
Deep Convolutional Neural Networks have sparked a renaissance in all the sub-fields of computer vision. Tremendous progress has been made in the area of image restoration. The research community has pushed the boundaries of image deblurring, super-resolution, and denoising. However, given a distorted image, most existing methods typically produce a single restored output. The tasks mentioned above are inherently ill-posed, leading to an infinite number of plausible solutions. This thesis focuses on designing image restoration techniques capable of producing multiple restored results and granting users more control over the restoration process. Towards this goal, we demonstrate how one could leverage the power of unsupervised representation learning. Image restoration is vital when applied to distorted images of human faces due to their social significance. Generative Adversarial Networks enable an unprecedented level of generated facial details combined with smooth latent space. We leverage the power of GANs towards the goal of learning controllable neural face representations. We demonstrate how to learn an inverse mapping from image space to these latent representations, tuning these representations towards a specific task, and finally manipulating latent codes in these spaces. For example, we show how GANs and their inverse mappings enable the restoration and editing of faces in the context of extreme face super-resolution and the generation of novel view sharp videos from a single motion-blurred image of a face. This thesis also addresses more general blind super-resolution, denoising, and scratch removal problems, where blur kernels and noise levels are unknown. We resort to contrastive representation learning and first learn the latent space of degradations. We demonstrate that the learned representation allows inference of ground-truth degradation parameters and can guide the restoration process. Moreover, it enables control over the amount of deblurring and denoising in the restoration via manipulation of latent degradation features.
Learning Generalizable Visual Patterns Without Human Supervision
Simon jenni · oct. 2021.
Owing to the existence of large labeled datasets, Deep Convolutional Neural Networks have ushered in a renaissance in computer vision. However, almost all of the visual data we generate daily - several human lives worth of it - remains unlabeled and thus out of reach of today’s dominant supervised learning paradigm. This thesis focuses on techniques that steer deep models towards learning generalizable visual patterns without human supervision. Our primary tool in this endeavor is the design of Self-Supervised Learning tasks, i.e., pretext-tasks for which labels do not involve human labor. Besides enabling the learning from large amounts of unlabeled data, we demonstrate how self-supervision can capture relevant patterns that supervised learning largely misses. For example, we design learning tasks that learn deep representations capturing shape from images, motion from video, and 3D pose features from multi-view data. Notably, these tasks’ design follows a common principle: The recognition of data transformations. The strong performance of the learned representations on downstream vision tasks such as classification, segmentation, action recognition, or pose estimation validate this pretext-task design. This thesis also explores the use of Generative Adversarial Networks (GANs) for unsupervised representation learning. Besides leveraging generative adversarial learning to define image transformation for self-supervised learning tasks, we also address training instabilities of GANs through the use of noise. While unsupervised techniques can significantly reduce the burden of supervision, in the end, we still rely on some annotated examples to fine-tune learned representations towards a target task. To improve the learning from scarce or noisy labels, we describe a supervised learning algorithm with improved generalization in these challenging settings.
Learning Interpretable Representations of Images
Attila szabó · june 2019.
Computers represent images with pixels and each pixel contains three numbers for red, green and blue colour values. These numbers are meaningless for humans and they are mostly useless when used directly with classical machine learning techniques like linear classifiers. Interpretable representations are the attributes that humans understand: the colour of the hair, viewpoint of a car or the 3D shape of the object in the scene. Many computer vision tasks can be viewed as learning interpretable representations, for example a supervised classification algorithm directly learns to represent images with their class labels. In this work we aim to learn interpretable representations (or features) indirectly with lower levels of supervision. This approach has the advantage of cost savings on dataset annotations and the flexibility of using the features for multiple follow-up tasks. We made contributions in three main areas: weakly supervised learning, unsupervised learning and 3D reconstruction. In the weakly supervised case we use image pairs as supervision. Each pair shares a common attribute and differs in a varying attribute. We propose a training method that learns to separate the attributes into separate feature vectors. These features then are used for attribute transfer and classification. We also show theoretical results on the ambiguities of the learning task and the ways to avoid degenerate solutions. We show a method for unsupervised representation learning, that separates semantically meaningful concepts. We explain and show ablation studies how the components of our proposed method work: a mixing autoencoder, a generative adversarial net and a classifier. We propose a method for learning single image 3D reconstruction. It is done using only the images, no human annotation, stereo, synthetic renderings or ground truth depth map is needed. We train a generative model that learns the 3D shape distribution and an encoder to reconstruct the 3D shape. For that we exploit the notion of image realism. It means that the 3D reconstruction of the object has to look realistic when it is rendered from different random angles. We prove the efficacy of our method from first principles.
Learning Controllable Representations for Image Synthesis
Qiyang hu · june 2019.
In this thesis, our focus is learning a controllable representation and applying the learned controllable feature representation on images synthesis, video generation, and even 3D reconstruction. We propose different methods to disentangle the feature representation in neural network and analyze the challenges in disentanglement such as reference ambiguity and shortcut problem when using the weak label. We use the disentangled feature representation to transfer attributes between images such as exchanging hairstyle between two face images. Furthermore, we study the problem of how another type of feature, sketch, works in a neural network. The sketch can provide shape and contour of an object such as the silhouette of the side-view face. We leverage the silhouette constraint to improve the 3D face reconstruction from 2D images. The sketch can also provide the moving directions of one object, thus we investigate how one can manipulate the object to follow the trajectory provided by a user sketch. We propose a method to automatically generate video clips from a single image input using the sketch as motion and trajectory guidance to animate the object in that image. We demonstrate the efficiency of our approaches on several synthetic and real datasets.
Beyond Supervised Representation Learning
Mehdi noroozi · jan. 2019.
The complexity of any information processing task is highly dependent on the space where data is represented. Unfortunately, pixel space is not appropriate for the computer vision tasks such as object classification. The traditional computer vision approaches involve a multi-stage pipeline where at first images are transformed to a feature space through a handcrafted function and then consequenced by the solution in the feature space. The challenge with this approach is the complexity of designing handcrafted functions that extract robust features. The deep learning based approaches address this issue by end-to-end training of a neural network for some tasks that lets the network to discover the appropriate representation for the training tasks automatically. It turns out that image classification task on large scale annotated datasets yields a representation transferable to other computer vision tasks. However, supervised representation learning is limited to annotations. In this thesis we study self-supervised representation learning where the goal is to alleviate these limitations by substituting the classification task with pseudo tasks where the labels come for free. We discuss self-supervised learning by solving jigsaw puzzles that uses context as supervisory signal. The rational behind this task is that the network requires to extract features about object parts and their spatial configurations to solve the jigsaw puzzles. We also discuss a method for representation learning that uses an artificial supervisory signal based on counting visual primitives. This supervisory signal is obtained from an equivariance relation. We use two image transformations in the context of counting: scaling and tiling. The first transformation exploits the fact that the number of visual primitives should be invariant to scale. The second transformation allows us to equate the total number of visual primitives in each tile to that in the whole image. The most effective transfer strategy is fine-tuning, which restricts one to use the same model or parts thereof for both pretext and target tasks. We discuss a novel framework for self-supervised learning that overcomes limitations in designing and comparing different tasks, models, and data domains. In particular, our framework decouples the structure of the self-supervised model from the final task-specific finetuned model. Finally, we study the problem of multi-task representation learning. A naive approach to enhance the representation learned by a task is to train the task jointly with other tasks that capture orthogonal attributes. Having a diverse set of auxiliary tasks, imposes challenges on multi-task training from scratch. We propose a framework that allows us to combine arbitrarily different feature spaces into a single deep neural network. We reduce the auxiliary tasks to classification tasks and the multi-task learning to multi-label classification task consequently. Nevertheless, combining multiple representation space without being aware of the target task might be suboptimal. As our second contribution, we show empirically that this is indeed the case and propose to combine multiple tasks after the fine-tuning on the target task.
Motion Deblurring from a Single Image
Meiguang jin · dec. 2018.
With the information explosion, a tremendous amount photos is captured and shared via social media everyday. Technically, a photo requires a finite exposure to accumulate light from the scene. Thus, objects moving during the exposure generate motion blur in a photo. Motion blur is an image degradation that makes visual content less interpretable and is therefore often seen as a nuisance. Although motion blur can be reduced by setting a short exposure time, an insufficient amount of light has to be compensated through increasing the sensor’s sensitivity, which will inevitably bring large amount of sensor noise. Thus this motivates the necessity of removing motion blur computationally. Motion deblurring is an important problem in computer vision and it is challenging due to its ill-posed nature, which means the solution is not well defined. Mathematically, a blurry image caused by uniform motion is formed by the convolution operation between a blur kernel and a latent sharp image. Potentially there are infinite pairs of blur kernel and latent sharp image that can result in the same blurry image. Hence, some prior knowledge or regularization is required to address this problem. Even if the blur kernel is known, restoring the latent sharp image is still difficult as the high frequency information has been removed. Although we can model the uniform motion deblurring problem mathematically, it can only address the camera in-plane translational motion. Practically, motion is more complicated and can be non-uniform. Non-uniform motion blur can come from many sources, camera out-of-plane rotation, scene depth change, object motion and so on. Thus, it is more challenging to remove non-uniform motion blur. In this thesis, our focus is motion blur removal. We aim to address four challenging motion deblurring problems. We start from the noise blind image deblurring scenario where blur kernel is known but the noise level is unknown. We introduce an efficient and robust solution based on a Bayesian framework using a smooth generalization of the 0−1 loss to address this problem. Then we study the blind uniform motion deblurring scenario where both the blur kernel and the latent sharp image are unknown. We explore the relative scale ambiguity between the latent sharp image and blur kernel to address this issue. Moreover, we study the face deblurring problem and introduce a novel deep learning network architecture to solve it. We also address the general motion deblurring problem and particularly we aim at recovering a sequence of 7 frames each depicting some instantaneous motion of the objects in the scene.
Towards a Novel Paradigm in Blind Deconvolution: From Natural to Cartooned Image Statistics
Daniele perrone · july 2015.
In this thesis we study the blind deconvolution problem. Blind deconvolution consists in the estimation of a sharp image and a blur kernel from an observed blurry image. Because the blur model admits several solutions it is necessary to devise an image prior that favors the true blur kernel and sharp image. Recently it has been shown that a class of blind deconvolution formulations and image priors has the no-blur solution as global minimum. Despite this shortcoming, algorithms based on these formulations and priors can successfully solve blind deconvolution. In this thesis we show that a suitable initialization can exploit the non-convexity of the problem and yield the desired solution. Based on these conclusions, we propose a novel “vanilla” algorithm stripped of any enhancement typically used in the literature. Our algorithm, despite its simplicity, is able to compete with the top performers on several datasets. We have also investigated a remarkable behavior of a 1998 algorithm, whose formulation has the no-blur solution as global minimum: even when initialized at the no-blur solution, it converges to the correct solution. We show that this behavior is caused by an apparently insignificant implementation strategy that makes the algorithm no longer minimize the original cost functional. We also demonstrate that this strategy improves the results of our “vanilla” algorithm. Finally, we present a study of image priors for blind deconvolution. We provide experimental evidence supporting the recent belief that a good image prior is one that leads to a good blur estimate rather than being a good natural image statistical model. By focusing the attention on the blur estimation alone, we show that good blur estimates can be obtained even when using images quite different from the true sharp image. This allows using image priors, such as those leading to “cartooned” images, that avoid the no-blur solution. By using an image prior that produces “cartooned” images we achieve state-of-the-art results on different publicly available datasets. We therefore suggests a shift of paradigm in blind deconvolution: from modeling natural image statistics to modeling cartooned image statistics.
New Perspectives on Uncalibrated Photometric Stereo
Thoma papadhimitri · june 2014.
This thesis investigates the problem of 3D reconstruction of a scene from 2D images. In particular, we focus on photometric stereo which is a technique that computes the 3D geometry from at least three images taken from the same viewpoint and under different illumination conditions. When the illumination is unknown (uncalibrated photometric stereo) the problem is ambiguous: different combinations of geometry and illumination can generate the same images. First, we solve the ambiguity by exploiting the Lambertian reflectance maxima. These are points defined on curved surfaces where the normals are parallel to the light direction. Then, we propose a solution that can be computed in closed-form and thus very efficiently. Our algorithm is also very robust and yields always the same estimate regardless of the initial ambiguity. We validate our method on real world experiments and achieve state-of-art results. In this thesis we also solve for the first time the uncalibrated photometric stereo problem under the perspective projection model. We show that unlike in the orthographic case, one can uniquely reconstruct the normals of the object and the lights given only the input images and the camera calibration (focal length and image center). We also propose a very efficient algorithm which we validate on synthetic and real world experiments and show that the proposed technique is a generalization of the orthographic case. Finally, we investigate the uncalibrated photometric stereo problem in the case where the lights are distributed near the scene. In this case we propose an alternating minimization technique which converges quickly and overcomes the limitations of prior work that assumes distant illumination. We show experimentally that adopting a near-light model for real world scenes yields very accurate reconstructions.
The power of computer vision: A critical analysis
Research output : Thesis › PhD Thesis - Research UT, graduation UT
Original language | English |
---|---|
Qualification | Doctor of Philosophy |
Awarding Institution | |
Supervisors/Advisors | , Supervisor , Co-Supervisor |
Award date | 27 Sept 2023 |
Place of Publication | Enschede |
Publisher | |
Print ISBNs | 978-90-365-5793-1 |
Electronic ISBNs | 978-90-365-5794-8 |
DOIs | |
Publication status | Published - 2023 |
Access to Document
- 10.3990/1.9789036557948
- Simon Stevin proefschrift Rosalie Waelen Proof, 4.48 MB
Fingerprint
- Critical Approach Computer Science 100%
- Individual Life Computer Science 33%
- Dynamic Power Computer Science 33%
T1 - The power of computer vision
T2 - A critical analysis
AU - Waelen, Rosalie
N2 - Computer vision is a subfield of artificial intelligence (AI), focused on automizing the analysis of images and videos. This dissertation offers a critical analysis of computer vision, discussing the potential ethical and societal implications of the technology. The critical analysis is based on a new approach to AI ethics, which is inspired by the tradition of critical theory. The goal of the critical analysis of computer vision is to uncover the multitude of ways in which computer vision can impact human autonomy. Some topics discussed in light of this goal are: the history of cameras and their constitutive effects, the ways in which facial recognition tools can misrecognize people in a normative sense, and the exploitative nature of datafication practices. The critical approach to AI ethics that is presented in this dissertation, is critical in the sense that it shares critical theory’s emancipatory aim and preoccupation with power dynamics. The critical approach consists of a framework that allows AI ethicists to identify AI’s ethical and societal implications in terms of power, and to evaluate these issues in light of their impact on human autonomy. However, the approach also encourages more direct and in-depth applications of the critical theory literature to address AI’s impact on individual lives and society. The critical analysis of computer vision functions as a case study for testing out this new, critical approach to AI ethics.
AB - Computer vision is a subfield of artificial intelligence (AI), focused on automizing the analysis of images and videos. This dissertation offers a critical analysis of computer vision, discussing the potential ethical and societal implications of the technology. The critical analysis is based on a new approach to AI ethics, which is inspired by the tradition of critical theory. The goal of the critical analysis of computer vision is to uncover the multitude of ways in which computer vision can impact human autonomy. Some topics discussed in light of this goal are: the history of cameras and their constitutive effects, the ways in which facial recognition tools can misrecognize people in a normative sense, and the exploitative nature of datafication practices. The critical approach to AI ethics that is presented in this dissertation, is critical in the sense that it shares critical theory’s emancipatory aim and preoccupation with power dynamics. The critical approach consists of a framework that allows AI ethicists to identify AI’s ethical and societal implications in terms of power, and to evaluate these issues in light of their impact on human autonomy. However, the approach also encourages more direct and in-depth applications of the critical theory literature to address AI’s impact on individual lives and society. The critical analysis of computer vision functions as a case study for testing out this new, critical approach to AI ethics.
U2 - 10.3990/1.9789036557948
DO - 10.3990/1.9789036557948
M3 - PhD Thesis - Research UT, graduation UT
SN - 978-90-365-5793-1
T3 - Simon Stevin Series in Ethics of Technology
PB - University of Twente
CY - Enschede
arXiv's Accessibility Forum starts next month!
Help | Advanced Search
Computer Science > Artificial Intelligence
Title: phd thesis: exploring the role of (self-)attention in cognitive and computer vision architecture.
Abstract: We investigate the role of attention and memory in complex reasoning tasks. We analyze Transformer-based self-attention as a model and extend it with memory. By studying a synthetic visual reasoning test, we refine the taxonomy of reasoning tasks. Incorporating self-attention with ResNet50, we enhance feature maps using feature-based and spatial attention, achieving efficient solving of challenging visual reasoning tasks. Our findings contribute to understanding the attentional needs of SVRT tasks. Additionally, we propose GAMR, a cognitive architecture combining attention and memory, inspired by active vision theory. GAMR outperforms other architectures in sample efficiency, robustness, and compositionality, and shows zero-shot generalization on new reasoning tasks.
Comments: | PhD Thesis, 152 pages, 32 figures, 6 tables |
Subjects: | Artificial Intelligence (cs.AI); Computer Vision and Pattern Recognition (cs.CV); Machine Learning (cs.LG); Symbolic Computation (cs.SC) |
Cite as: | [cs.AI] |
(or [cs.AI] for this version) | |
Focus to learn more arXiv-issued DOI via DataCite |
Submission history
Access paper:.
- Other Formats

References & Citations
- Google Scholar
- Semantic Scholar
BibTeX formatted citation

Bibliographic and Citation Tools
Code, data and media associated with this article, recommenders and search tools.
- Institution
arXivLabs: experimental projects with community collaborators
arXivLabs is a framework that allows collaborators to develop and share new arXiv features directly on our website.
Both individuals and organizations that work with arXivLabs have embraced and accepted our values of openness, community, excellence, and user data privacy. arXiv is committed to these values and only works with partners that adhere to them.
Have an idea for a project that will add value for arXiv's community? Learn more about arXivLabs .
- Press Enter to activate screen reader mode.
Computer Vision Lab
Doctoral theses.
- AI - General
- Computer Vision - General
- Academic Paper(s)
- Facial Recognition
- Image enhancing
- Object Detection/Tracking
- Phone Technology
- How Stuff Works
- Controversies
- Book Reviews
Be an Optimist Prime in the world of Computer Vision and AI
How to find a good thesis topic in computer vision.
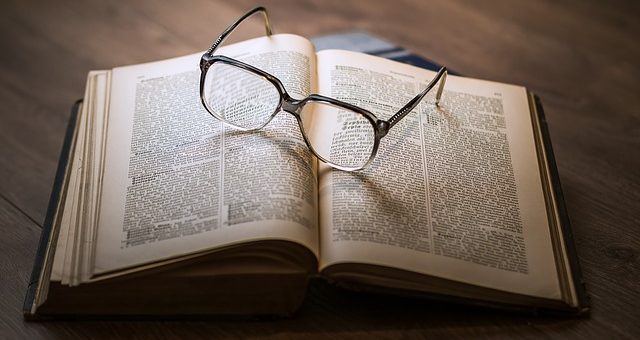
“What are some good thesis topics in Computer Vision?”
This is a common question that people ask in forums – and it’s an important question to ask for two reasons:
- There’s nothing worse than starting over in research because the path you decided to take turned out to be a dead end.
- There’s also nothing worse than being stuck with a generally good topic but one that doesn’t interest you at all. A “good” thesis topic has to be one that interests you and will keep you involved and stimulated for as long as possible.
For these reasons, it’s best to do as much research as you can to avoid the above pitfalls or your days of research will slowly become torturous for you – and that would be a shame because computer vision can truly be a lot of fun 🙂
So, down to business.
The purpose of this post is to propose ways to find that one perfect topic that will keep you engaged for months (or years) to come – and something you’ll be proud to talk about amongst friends and family.
I’ll start the discussion off by saying that your search strategy for topics depends entirely on whether you’re preparing for a Master’s thesis or a PhD. The former can be more general, the latter is (nearly always) very fine-grained specific. Let’s start with undergraduate topics first.
Undergraduate Studies
I’ll propose here three steps you can take to assist in your search: looking at the applications of computer vision, examining the OpenCV library, and talking to potential supervisors.
Applications of Computer Vision
Computer Vision has so many uses in the world. Why not look through a comprehensive list of them and see if anything on that list draws you in? Here’s one such list I collected from the British Machine Vision Association :
- agriculture
- augmented reality
- autonomous vehicles (big one nowadays!)
- character recognition
- industrial quality inspection
- face recognition
- gesture analysis
- image restoration
- medical image analysis
- pollution monitoring
- process control
- remote sensing
- robotics (e.g. navigation)
- security and surveillance
Go through this list and work out if something stands out for you. Perhaps your family is involved in agriculture? Look up how computer vision is helping in this field! The Economist wrote a fascinating article entitled “ The Future of Agriculture ” in which they discuss, among other things, the use of drones to monitor crops, create contour maps of fields, etc. Perhaps Computer Vision can assist with some of these tasks? Look into this!
OpenCV is the best library out there for image and video processing (I’ll be writing a lot more about it on this blog). Other libraries do exist that do certain specific things a little better, e.g. Tracking.js , which performs things like tracking inside the browser, but generally speaking, there’s nothing better than OpenCV.
On the topic of searching for thesis topics, I recall once reading a suggestion of going through the functions that OpenCV has to offer and seeing if anything sticks out at you there. A brilliant idea. Work down the list of the OpenCV documentation . Perhaps face recognition interests you? There are so many interesting projects where this can be utilised!
Talk to potential supervisors
You can’t go past this suggestion. Every academic has ideas constantly buzzing around his head. Academics are immersed in their field of research and are always to talking to people in the industry to look for interesting projects that they could get funding for. Go and talk to the academics at your university that are involved in Computer Vision. I’m sure they’ll have at least one project proposal ready to go for you.
You should also run any ideas of yours past them that may have emerged from the two previous steps. Or at least mention things that stood out for you (e.g. agriculture). They may be able to come up with something themselves.
PhD Studies
Well, if you’ve reached this far in your studies then chances are you have a fairly good idea of how this all works now. I won’t patronise you too much, then. But I will mention three points that I wish someone had told me prior to starting my PhD adventure:
- You should be building your research topic around a supervisor . They’ve been in the field for a long time and know where the niches and dead ends are. Use their experience! If there’s a supervisor who is constantly publishing in object tracking, then doing research with them in this area makes sense.
- If your supervisor has a ready-made topic for you, CONSIDER TAKING IT . I can’t stress this enough. Usually the first year of your PhD involves you searching (often blindly) around various fields in Computer Vision and then just going deeper and deeper into one specific area to find a niche. If your supervisor has a topic on hand for you, this means that you are already one year ahead of the crowd. And that means one year saved of frustration because searching around in a vast realm of publications can be daunting – believe me, I’ve been there.
- Avoid going into trending topics. For example, object recognition using Convolutional Neural Networks is a topic that currently everyone is going crazy about in the world of Computer Vision. This means that in your studies, you will be competing for publications with big players (e.g. Google) who have money, manpower, and computer power at their disposal. You don’t want to enter into this war unless you are confident that your supervisor knows what they’re doing and/or your university has the capabilities to play in this big league also.
Spending time looking for a thesis topic is time worth spending. It could save you from future pitfalls. With respect to undergraduate thesis topics looking at Computer Vision applications is one place to start. The OpenCV library is another. And talking to potential supervisors at your university is also a good idea.
With respect to PhD thesis topics, it’s important to take into consideration what the fields of expertise of your potential supervisors are and then searching for topics in these areas. If these supervisors have ready-made topics for you, it is worth considering them to save you a lot of time and stress in the first year or so of your studies. Finally, it’s usually good to avoid trending topics because of the people you will be competing against for publications.
But the bottom line is, devote time to finding a topic that truly interests you . It’ll be the difference between wanting to get out of bed to do more and more research in your field or dreading each time you have to walk into your Computer Science building in the morning.
To be informed when new content like this is posted, subscribe to the mailing list:
Related posts
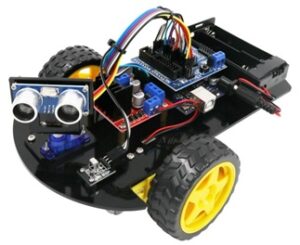
Arduino – Rotor Sweeping for Obstacle Detection Code
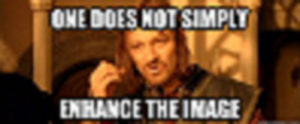
Security Film or Image Enhancing is Possible
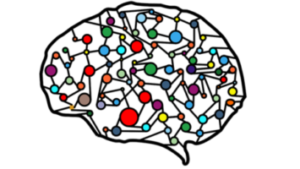
How Deep Learning Works – The Very Basics
One reply to “how to find a good thesis topic in computer vision”.
- Pingback: My Top 5 Posts So Far |
Leave a Reply Cancel reply
Your email address will not be published. Required fields are marked *
Save my name, email, and website in this browser for the next time I comment.
- Bibliography
- More Referencing guides Blog Automated transliteration Relevant bibliographies by topics
- Automated transliteration
- Relevant bibliographies by topics
- Referencing guides
Visual vibration analysis
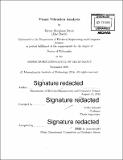
Other Contributors
Terms of use, description, date issued, collections.
Show Statistical Information
|
Welcome to the on-line version of the UNC dissertation proposal collection. The purpose of this collection is to provide examples of proposals for those of you who are thinking of writing a proposal of your own. I hope that this on-line collection proves to be more difficult to misplace than the physical collection that periodically disappears. If you are preparing to write a proposal you should make a point of reading the excellent document The Path to the Ph.D., written by James Coggins. It includes advice about selecting a topic, preparing a proposal, taking your oral exam and finishing your dissertation. It also includes accounts by many people about the process that each of them went through to find a thesis topic. Adding to the Collection This collection of proposals becomes more useful with each new proposal that is added. If you have an accepted proposal, please help by including it in this collection. You may notice that the bulk of the proposals currently in this collection are in the area of computer graphics. This is an artifact of me knowing more computer graphics folks to pester for their proposals. Add your non-graphics proposal to the collection and help remedy this imbalance! There are only two requirements for a UNC proposal to be added to this collection. The first requirement is that your proposal must be completely approved by your committee. If we adhere to this, then each proposal in the collection serves as an example of a document that five faculty members have signed off on. The second requirement is that you supply, as best you can, exactly the document that your committee approved. While reading over my own proposal I winced at a few of the things that I had written. I resisted the temptation to change the document, however, because this collection should truely reflect what an accepted thesis proposal looks like. Note that there is no requirement that the author has finished his/her Ph.D. Several of the proposals in the collection were written by people who, as of this writing, are still working on their dissertation. This is fine! I encourage people to submit their proposals in any form they wish. Perhaps the most useful forms at the present are Postscript and HTML, but this may not always be so. Greg Coombe has generously provided LaTeX thesis style files , which, he says, conform to the 2004-2005 stlye requirements.
Many thanks to everyone who contributed to this collection!
Greg Coombe, "Incremental Construction of Surface Light Fields" in PDF . Karl Hillesland, "Image-Based Modelling Using Nonlinear Function Fitting on a Stream Architecture" in PDF . Martin Isenburg, "Compressing, Streaming, and Processing of Large Polygon Meshes" in PDF . Ajith Mascarenhas, "A Topological Framework for Visualizing Time-varying Volumetric Datasets" in PDF . Josh Steinhurst, "Practical Photon Mapping in Hardware" in PDF . Ronald Azuma, "Predictive Tracking for Head-Mounted Displays," in Postscript Mike Bajura, "Virtual Reality Meets Computer Vision," in Postscript David Ellsworth, "Polygon Rendering for Interactive Scientific Visualization on Multicomputers," in Postscript Richard Holloway, "A Systems-Engineering Study of the Registration Errors in a Virtual-Environment System for Cranio-Facial Surgery Planning," in Postscript Victoria Interrante, "Uses of Shading Techniques, Artistic Devices and Interaction to Improve the Visual Understanding of Multiple Interpenetrating Volume Data Sets," in Postscript Mark Mine, "Modeling From Within: A Proposal for the Investigation of Modeling Within the Immersive Environment" in Postscript Steve Molnar, "High-Speed Rendering using Scan-Line Image Composition," in Postscript Carl Mueller, " High-Performance Rendering via the Sort-First Architecture ," in Postscript Ulrich Neumann, "Direct Volume Rendering on Multicomputers," in Postscript Marc Olano, "Programmability in an Interactive Graphics Pipeline," in Postscript Krish Ponamgi, "Collision Detection for Interactive Environments and Simulations," in Postscript Russell Taylor, "Nanomanipulator Proposal," in Postscript Greg Turk, " Generating Textures on Arbitrary Surfaces ," in HTML and Postscript Terry Yoo, " Statistical Control of Nonlinear Diffusion ," in Postscript
| |
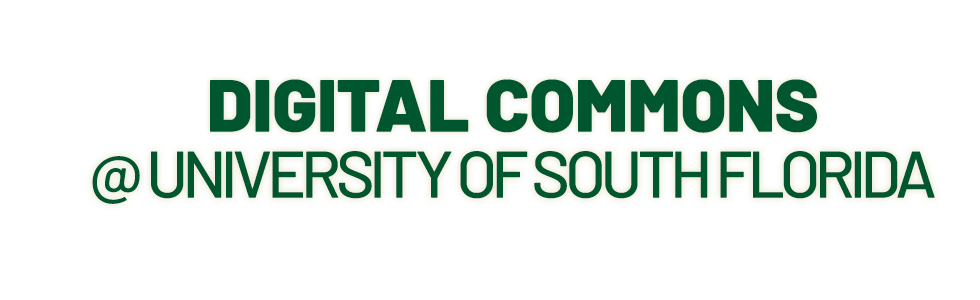
- USF Research
- USF Libraries
Digital Commons @ USF > College of Engineering > Computer Science and Engineering > Theses and Dissertations
Computer Science and Engineering Theses and Dissertations
Theses/dissertations from 2024 2024.
Automatic Image-Based Nutritional Calculator App , Kejvi Cupa
Individual Behavioral Modeling Across Games of Strategy , Logan Fields
Semi-automated Cell Annotation Framework Using Deep Learning , Abhiram Kandiyana
Predicting Gender of Author Using Large Language Models (LLMs) , Satya Uday Sanku
Context-aware Affective Behavior Modeling and Analytics , Md Taufeeq Uddin
Exploring the Use of Enhanced SWAD Towards Building Learned Models that Generalize Better to Unseen Sources , Brandon M. Weinhofer
Theses/Dissertations from 2023 2023
Refining the Machine Learning Pipeline for US-based Public Transit Systems , Jennifer Adorno
Insect Classification and Explainability from Image Data via Deep Learning Techniques , Tanvir Hossain Bhuiyan
Brain-Inspired Spatio-Temporal Learning with Application to Robotics , Thiago André Ferreira Medeiros
Evaluating Methods for Improving DNN Robustness Against Adversarial Attacks , Laureano Griffin
Analyzing Multi-Robot Leader-Follower Formations in Obstacle-Laden Environments , Zachary J. Hinnen
Secure Lightweight Cryptographic Hardware Constructions for Deeply Embedded Systems , Jasmin Kaur
A Psychometric Analysis of Natural Language Inference Using Transformer Language Models , Antonio Laverghetta Jr.
Graph Analysis on Social Networks , Shen Lu
Deep Learning-based Automatic Stereology for High- and Low-magnification Images , Hunter Morera
Deciphering Trends and Tactics: Data-driven Techniques for Forecasting Information Spread and Detecting Coordinated Campaigns in Social Media , Kin Wai Ng Lugo
Automated Approaches to Enable Innovative Civic Applications from Citizen Generated Imagery , Hye Seon Yi
Theses/Dissertations from 2022 2022
Towards High Performing and Reliable Deep Convolutional Neural Network Models for Typically Limited Medical Imaging Datasets , Kaoutar Ben Ahmed
Task Progress Assessment and Monitoring Using Self-Supervised Learning , Sainath Reddy Bobbala
Towards More Task-Generalized and Explainable AI Through Psychometrics , Alec Braynen
A Multiple Input Multiple Output Framework for the Automatic Optical Fractionator-based Cell Counting in Z-Stacks Using Deep Learning , Palak Dave
On the Reliability of Wearable Sensors for Assessing Movement Disorder-Related Gait Quality and Imbalance: A Case Study of Multiple Sclerosis , Steven Díaz Hernández
Securing Critical Cyber Infrastructures and Functionalities via Machine Learning Empowered Strategies , Tao Hou
Social Media Time Series Forecasting and User-Level Activity Prediction with Gradient Boosting, Deep Learning, and Data Augmentation , Fred Mubang
A Study of Deep Learning Silhouette Extractors for Gait Recognition , Sneha Oladhri
Analyzing Decision-making in Robot Soccer for Attacking Behaviors , Justin Rodney
Generative Spatio-Temporal and Multimodal Analysis of Neonatal Pain , Md Sirajus Salekin
Secure Hardware Constructions for Fault Detection of Lattice-based Post-quantum Cryptosystems , Ausmita Sarker
Adaptive Multi-scale Place Cell Representations and Replay for Spatial Navigation and Learning in Autonomous Robots , Pablo Scleidorovich
Predicting the Number of Objects in a Robotic Grasp , Utkarsh Tamrakar
Humanoid Robot Motion Control for Ramps and Stairs , Tommy Truong
Preventing Variadic Function Attacks Through Argument Width Counting , Brennan Ward
Theses/Dissertations from 2021 2021
Knowledge Extraction and Inference Based on Visual Understanding of Cooking Contents , Ahmad Babaeian Babaeian Jelodar
Efficient Post-Quantum and Compact Cryptographic Constructions for the Internet of Things , Rouzbeh Behnia
Efficient Hardware Constructions for Error Detection of Post-Quantum Cryptographic Schemes , Alvaro Cintas Canto
Using Hyper-Dimensional Spanning Trees to Improve Structure Preservation During Dimensionality Reduction , Curtis Thomas Davis
Design, Deployment, and Validation of Computer Vision Techniques for Societal Scale Applications , Arup Kanti Dey
AffectiveTDA: Using Topological Data Analysis to Improve Analysis and Explainability in Affective Computing , Hamza Elhamdadi
Automatic Detection of Vehicles in Satellite Images for Economic Monitoring , Cole Hill
Analysis of Contextual Emotions Using Multimodal Data , Saurabh Hinduja
Data-driven Studies on Social Networks: Privacy and Simulation , Yasanka Sameera Horawalavithana
Automated Identification of Stages in Gonotrophic Cycle of Mosquitoes Using Computer Vision Techniques , Sherzod Kariev
Exploring the Use of Neural Transformers for Psycholinguistics , Antonio Laverghetta Jr.
Secure VLSI Hardware Design Against Intellectual Property (IP) Theft and Cryptographic Vulnerabilities , Matthew Dean Lewandowski
Turkic Interlingua: A Case Study of Machine Translation in Low-resource Languages , Jamshidbek Mirzakhalov
Automated Wound Segmentation and Dimension Measurement Using RGB-D Image , Chih-Yun Pai
Constructing Frameworks for Task-Optimized Visualizations , Ghulam Jilani Abdul Rahim Quadri
Trilateration-Based Localization in Known Environments with Object Detection , Valeria M. Salas Pacheco
Recognizing Patterns from Vital Signs Using Spectrograms , Sidharth Srivatsav Sribhashyam
Recognizing Emotion in the Wild Using Multimodal Data , Shivam Srivastava
A Modular Framework for Multi-Rotor Unmanned Aerial Vehicles for Military Operations , Dante Tezza
Human-centered Cybersecurity Research — Anthropological Findings from Two Longitudinal Studies , Anwesh Tuladhar
Learning State-Dependent Sensor Measurement Models To Improve Robot Localization Accuracy , Troi André Williams
Human-centric Cybersecurity Research: From Trapping the Bad Guys to Helping the Good Ones , Armin Ziaie Tabari
Theses/Dissertations from 2020 2020
Classifying Emotions with EEG and Peripheral Physiological Data Using 1D Convolutional Long Short-Term Memory Neural Network , Rupal Agarwal
Keyless Anti-Jamming Communication via Randomized DSSS , Ahmad Alagil
Active Deep Learning Method to Automate Unbiased Stereology Cell Counting , Saeed Alahmari
Composition of Atomic-Obligation Security Policies , Yan Cao Albright
Action Recognition Using the Motion Taxonomy , Maxat Alibayev
Sentiment Analysis in Peer Review , Zachariah J. Beasley
Spatial Heterogeneity Utilization in CT Images for Lung Nodule Classication , Dmitrii Cherezov
Feature Selection Via Random Subsets Of Uncorrelated Features , Long Kim Dang
Unifying Security Policy Enforcement: Theory and Practice , Shamaria Engram
PsiDB: A Framework for Batched Query Processing and Optimization , Mehrad Eslami
Composition of Atomic-Obligation Security Policies , Danielle Ferguson
Algorithms To Profile Driver Behavior From Zero-permission Embedded Sensors , Bharti Goel
The Efficiency and Accuracy of YOLO for Neonate Face Detection in the Clinical Setting , Jacqueline Hausmann
Beyond the Hype: Challenges of Neural Networks as Applied to Social Networks , Anthony Hernandez
Privacy-Preserving and Functional Information Systems , Thang Hoang
Managing Off-Grid Power Use for Solar Fueled Residences with Smart Appliances, Prices-to-Devices and IoT , Donnelle L. January
Novel Bit-Sliced In-Memory Computing Based VLSI Architecture for Fast Sobel Edge Detection in IoT Edge Devices , Rajeev Joshi
Edge Computing for Deep Learning-Based Distributed Real-time Object Detection on IoT Constrained Platforms at Low Frame Rate , Lakshmikavya Kalyanam
Establishing Topological Data Analysis: A Comparison of Visualization Techniques , Tanmay J. Kotha
Machine Learning for the Internet of Things: Applications, Implementation, and Security , Vishalini Laguduva Ramnath
System Support of Concurrent Database Query Processing on a GPU , Hao Li
Deep Learning Predictive Modeling with Data Challenges (Small, Big, or Imbalanced) , Renhao Liu
Countermeasures Against Various Network Attacks Using Machine Learning Methods , Yi Li
Towards Safe Power Oversubscription and Energy Efficiency of Data Centers , Sulav Malla
Design of Support Measures for Counting Frequent Patterns in Graphs , Jinghan Meng
Automating the Classification of Mosquito Specimens Using Image Processing Techniques , Mona Minakshi
Models of Secure Software Enforcement and Development , Hernan M. Palombo
Functional Object-Oriented Network: A Knowledge Representation for Service Robotics , David Andrés Paulius Ramos
Lung Nodule Malignancy Prediction from Computed Tomography Images Using Deep Learning , Rahul Paul
Algorithms and Framework for Computing 2-body Statistics on Graphics Processing Units , Napath Pitaksirianan
Efficient Viewshed Computation Algorithms On GPUs and CPUs , Faisal F. Qarah
Relational Joins on GPUs for In-Memory Database Query Processing , Ran Rui
Micro-architectural Countermeasures for Control Flow and Misspeculation Based Software Attacks , Love Kumar Sah
Efficient Forward-Secure and Compact Signatures for the Internet of Things (IoT) , Efe Ulas Akay Seyitoglu
Detecting Symptoms of Chronic Obstructive Pulmonary Disease and Congestive Heart Failure via Cough and Wheezing Sounds Using Smart-Phones and Machine Learning , Anthony Windmon
Toward Culturally Relevant Emotion Detection Using Physiological Signals , Khadija Zanna
Theses/Dissertations from 2019 2019
Beyond Labels and Captions: Contextualizing Grounded Semantics for Explainable Visual Interpretation , Sathyanarayanan Narasimhan Aakur
Empirical Analysis of a Cybersecurity Scoring System , Jaleel Ahmed
Phenomena of Social Dynamics in Online Games , Essa Alhazmi
A Machine Learning Approach to Predicting Community Engagement on Social Media During Disasters , Adel Alshehri
Interactive Fitness Domains in Competitive Coevolutionary Algorithm , ATM Golam Bari
Measuring Influence Across Social Media Platforms: Empirical Analysis Using Symbolic Transfer Entropy , Abhishek Bhattacharjee
A Communication-Centric Framework for Post-Silicon System-on-chip Integration Debug , Yuting Cao
Authentication and SQL-Injection Prevention Techniques in Web Applications , Cagri Cetin
Multimodal Emotion Recognition Using 3D Facial Landmarks, Action Units, and Physiological Data , Diego Fabiano
Robotic Motion Generation by Using Spatial-Temporal Patterns from Human Demonstrations , Yongqiang Huang
Advanced Search
- Email Notifications and RSS
- All Collections
- USF Faculty Publications
- Open Access Journals
- Conferences and Events
- Theses and Dissertations
- Textbooks Collection
Useful Links
- Rights Information
- SelectedWorks
- Submit Research
Home | About | Help | My Account | Accessibility Statement | Language and Diversity Statements
Privacy Copyright
Home > FACULTIES > Computer Science > CSD-ETD
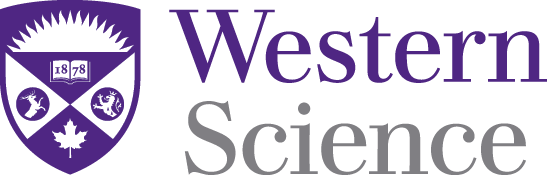
Computer Science Theses and Dissertations
This collection contains theses and dissertations from the Department of Computer Science, collected from the Scholarship@Western Electronic Thesis and Dissertation Repository
Theses/Dissertations from 2024 2024
A Target-Based and A Targetless Extrinsic Calibration Methods for Thermal Camera and 3D LiDAR , Farhad Dalirani
Using Driver Gaze and On-Road Driving Data for Predicting Driver Maneuvers in Advanced Driving Assistance Systems , Farzan Heidari
Protein-Protein Interaction Prediction , SeyedMohsen Hosseini
UTILIZING MACHINE LEARNING TECHNIQUES FOR DISPERSION MEASURE ESTIMATION IN FAST RADIO BURSTS STUDIES , Hosein Rajabi
Investigating Tree- and Graph-based Neural Networks for Natural Language Processing Applications , Sudipta Singha Roy
Framework for Bug Inducing Commit Prediction Using Quality Metrics , Alireza Tavakkoli Barzoki
Knowledge-grounded Natural Language Understanding of Biomedical and Clinical Literature , Xindi Wang
Theses/Dissertations from 2023 2023
Classification of DDoS Attack with Machine Learning Architectures and Exploratory Analysis , Amreen Anbar
Multi-view Contrastive Learning for Unsupervised Domain Adaptation in Brain-Computer Interfaces , Sepehr Asgarian
Improved Protein Sequence Alignments Using Deep Learning , Seyed Sepehr Ashrafzadeh
INVESTIGATING IMPROVEMENTS TO MESH INDEXING , Anurag Bhattacharjee
Algorithms and Software for Oligonucleotide Design , Qin Dong
Framework for Assessing Information System Security Posture Risks , Syed Waqas Hamdani
De novo sequencing of multiple tandem mass spectra of peptide containing SILAC labeling , Fang Han
Local Model Agnostic XAI Methodologies Applied to Breast Cancer Malignancy Predictions , Heather Hartley
A Quantitative Analysis Between Software Quality Posture and Bug-fixing Commit , Rongji He
A Novel Method for Assessment of Batch Effect on single cell RNA sequencing data , Behnam Jabbarizadeh
Dynamically Finding Optimal Kernel Launch Parameters for CUDA Programs , Taabish Jeshani
Citation Polarity Identification From Scientific Articles Using Deep Learning Methods , Souvik Kundu
Denoising-Based Domain Adaptation Network for EEG Source Imaging , Runze Li
Decoy-Target Database Strategy and False Discovery Rate Analysis for Glycan Identification , Xiaoou Li
DpNovo: A DEEP LEARNING MODEL COMBINED WITH DYNAMIC PROGRAMMING FOR DE NOVO PEPTIDE SEQUENCING , Yizhou Li
Developing A Smart Home Surveillance System Using Autonomous Drones , Chongju Mai
Look-Ahead Selective Plasticity for Continual Learning , Rouzbeh Meshkinnejad
The Two Visual Processing Streams Through The Lens Of Deep Neural Networks , Aidasadat Mirebrahimi Tafreshi
Source-free Domain Adaptation for Sleep Stage Classification , Yasmin Niknam
Data Heterogeneity and Its Implications for Fairness , Ghazaleh Noroozi
Enhancing Urban Life: A Policy-Based Autonomic Smart City Management System for Efficient, Sustainable, and Self-Adaptive Urban Environments , Elham Okhovat
Evaluating the Likelihood of Bug Inducing Commits Using Metrics Trend Analysis , Parul Parul
On Computing Optimal Repairs for Conditional Independence , Alireza Pirhadi
Open-Set Source-Free Domain Adaptation in Fundus Images Analysis , Masoud Pourreza
Migration in Edge Computing , Arshin Rezazadeh
A Modified Hopfield Network for the K-Median Problem , Cody Rossiter
Predicting Network Failures with AI Techniques , Chandrika Saha
Toward Building an Intelligent and Secure Network: An Internet Traffic Forecasting Perspective , Sajal Saha
An Exploration of Visual Analytic Techniques for XAI: Applications in Clinical Decision Support , Mozhgan Salimiparsa
Attention-based Multi-Source-Free Domain Adaptation for EEG Emotion Recognition , Amir Hesam Salimnia
Global Cyber Attack Forecast using AI Techniques , Nusrat Kabir Samia
IMPLEMENTATION OF A PRE-ASSESSMENT MODULE TO IMPROVE THE INITIAL PLAYER EXPERIENCE USING PREVIOUS GAMING INFORMATION , Rafael David Segistan Canizales
A Computational Framework For Identifying Relevant Cell Types And Specific Regulatory Mechanisms In Schizophrenia Using Data Integration Methods , Kayvan Shabani
Weakly-Supervised Anomaly Detection in Surveillance Videos Based on Two-Stream I3D Convolution Network , Sareh Soltani Nejad
Smartphone Loss Prevention System Using BLE and GPS Technology , Noshin Tasnim
A Hybrid Continual Machine Learning Model for Efficient Hierarchical Classification of Domain-Specific Text in The Presence of Class Overlap (Case Study: IT Support Tickets) , Yasmen M. Wahba
Reducing Negative Transfer of Random Data in Source-Free Unsupervised Domain Adaptation , Anthony Wong
Deep Neural Methods for True/Pseudo- Invasion Classification in Colorectal Polyp Whole-Slide Images , Zhiyuan Yang
Developing a Relay-based Autonomous Drone Delivery System , Muhammad Zakar
Learning Mortality Risk for COVID-19 Using Machine Learning and Statistical Methods , Shaoshi Zhang
Machine Learning Techniques for Improved Functional Brain Parcellation , Da Zhi
Theses/Dissertations from 2022 2022
The Design and Implementation of a High-Performance Polynomial System Solver , Alexander Brandt
Defining Service Level Agreements in Serverless Computing , Mohamed Elsakhawy
Algorithms for Regular Chains of Dimension One , Juan P. Gonzalez Trochez
Towards a Novel and Intelligent e-commerce Framework for Smart-Shopping Applications , Susmitha Hanumanthu
Multi-Device Data Analysis for Fault Localization in Electrical Distribution Grids , Jacob D L Hunte
Towards Parking Lot Occupancy Assessment Using Aerial Imagery and Computer Vision , John Jewell
Potential of Vision Transformers for Advanced Driver-Assistance Systems: An Evaluative Approach , Andrew Katoch
Psychological Understanding of Textual journals using Natural Language Processing approaches , Amirmohammad Kazemeinizadeh
Driver Behavior Analysis Based on Real On-Road Driving Data in the Design of Advanced Driving Assistance Systems , Nima Khairdoost
Solving Challenges in Deep Unsupervised Methods for Anomaly Detection , Vahid Reza Khazaie
Developing an Efficient Real-Time Terrestrial Infrastructure Inspection System Using Autonomous Drones and Deep Learning , Marlin Manka
Predictive Modelling For Topic Handling Of Natural Language Dialogue With Virtual Agents , Lareina Milambiling
Improving Deep Entity Resolution by Constraints , Soudeh Nilforoushan
Respiratory Pattern Analysis for COVID-19 Digital Screening Using AI Techniques , Annita Tahsin Priyoti
Extracting Microservice Dependencies Using Log Analysis , Andres O. Rodriguez Ishida
False Discovery Rate Analysis for Glycopeptide Identification , Shun Saito
Towards a Generalization of Fulton's Intersection Multiplicity Algorithm , Ryan Sandford
An Investigation Into Time Gazed At Traffic Objects By Drivers , Kolby R. Sarson
Exploring Artificial Intelligence (AI) Techniques for Forecasting Network Traffic: Network QoS and Security Perspectives , Ibrahim Mohammed Sayem
A Unified Representation and Deep Learning Architecture for Persuasive Essays in English , Muhammad Tawsif Sazid
Towards the development of a cost-effective Image-Sensing-Smart-Parking Systems (ISenSmaP) , Aakriti Sharma
Advances in the Automatic Detection of Optimization Opportunities in Computer Programs , Delaram Talaashrafi
Reputation-Based Trust Assessment of Transacting Service Components , Konstantinos Tsiounis
Fully Autonomous UAV Exploration in Confined and Connectionless Environments , Kirk P. Vander Ploeg
Three Contributions to the Theory and Practice of Optimizing Compilers , Linxiao Wang
Developing Intelligent Routing Algorithm over SDN: Reusable Reinforcement Learning Approach , Wumian Wang
Predicting and Modifying Memorability of Images , Mohammad Younesi
Theses/Dissertations from 2021 2021
Generating Effective Sentence Representations: Deep Learning and Reinforcement Learning Approaches , Mahtab Ahmed
A Physical Layer Framework for a Smart City Using Accumulative Bayesian Machine Learning , Razan E. AlFar
Load Balancing and Resource Allocation in Smart Cities using Reinforcement Learning , Aseel AlOrbani
Contrastive Learning of Auditory Representations , Haider Al-Tahan
Cache-Friendly, Modular and Parallel Schemes For Computing Subresultant Chains , Mohammadali Asadi
Protein Interaction Sites Prediction using Deep Learning , Sourajit Basak
Predicting Stock Market Sector Sentiment Through News Article Based Textual Analysis , William A. Beldman
Improving Reader Motivation with Machine Learning , Tanner A. Bohn
A Black-box Approach for Containerized Microservice Monitoring in Fog Computing , Shi Chang
Visualization and Interpretation of Protein Interactions , Dipanjan Chatterjee
A Framework for Characterising Performance in Multi-Class Classification Problems with Applications in Cancer Single Cell RNA Sequencing , Erik R. Christensen
Exploratory Search with Archetype-based Language Models , Brent D. Davis
Evolutionary Design of Search and Triage Interfaces for Large Document Sets , Jonathan A. Demelo
Building Effective Network Security Frameworks using Deep Transfer Learning Techniques , Harsh Dhillon
A Deep Topical N-gram Model and Topic Discovery on COVID-19 News and Research Manuscripts , Yuan Du
Automatic extraction of requirements-related information from regulatory documents cited in the project contract , Sara Fotouhi
Developing a Resource and Energy Efficient Real-time Delivery Scheduling Framework for a Network of Autonomous Drones , Gopi Gugan
A Visual Analytics System for Rapid Sensemaking of Scientific Documents , Amirreza Haghverdiloo Barzegar
Calibration Between Eye Tracker and Stereoscopic Vision System Employing a Linear Closed-Form Perspective-n-Point (PNP) Algorithm , Mohammad Karami
Fuzzy and Probabilistic Rule-Based Approaches to Identify Fault Prone Files , Piyush Kumar Korlepara
Parallel Arbitrary-precision Integer Arithmetic , Davood Mohajerani
A Technique for Evaluating the Health Status of a Software Module Using Process Metrics , . Ria
Visual Analytics for Performing Complex Tasks with Electronic Health Records , Neda Rostamzadeh
Predictive Model of Driver's Eye Fixation for Maneuver Prediction in the Design of Advanced Driving Assistance Systems , Mohsen Shirpour
A Generative-Discriminative Approach to Human Brain Mapping , Deepanshu Wadhwa
- Accessible Formats
Advanced Search
- Notify me via email or RSS
- Expert Gallery
- Online Journals
- eBook Collections
- Reports and Working Papers
- Conferences and Symposiums
- Electronic Theses and Dissertations
- Digitized Special Collections
- All Collections
- Disciplines
Author Corner
- Submit Thesis/Dissertation
Home | About | FAQ | My Account | Accessibility Statement | Privacy | Copyright
©1878 - 2016 Western University
Computer vision tasks for intelligent aerospace perception: An overview
- Published: 21 August 2024
Cite this article
- HuiLin Chen 1 ,
- QiYu Sun 1 ,
- FangFei Li 2 &
- Yang Tang 1
Explore all metrics
Computer vision tasks are crucial for aerospace missions as they help spacecraft to understand and interpret the space environment, such as estimating position and orientation, reconstructing 3D models, and recognizing objects, which have been extensively studied to successfully carry out the missions. However, traditional methods like Kalman filtering, structure from motion, and multi-view stereo are not robust enough to handle harsh conditions, leading to unreliable results. In recent years, deep learning (DL)-based perception technologies have shown great potential and outperformed traditional methods, especially in terms of their robustness to changing environments. To further advance DL-based aerospace perception, various frameworks, datasets, and strategies have been proposed, indicating significant potential for future applications. In this survey, we aim to explore the promising techniques used in perception tasks and emphasize the importance of DL-based aerospace perception. We begin by providing an overview of aerospace perception, including classical space programs developed in recent years, commonly used sensors, and traditional perception methods. Subsequently, we delve into three fundamental perception tasks in aerospace missions: pose estimation, 3D reconstruction, and recognition, as they are basic and crucial for subsequent decision-making and control. Finally, we discuss the limitations and possibilities in current research and provide an outlook on future developments, including the challenges of working with limited datasets, the need for improved algorithms, and the potential benefits of multi-source information fusion.
This is a preview of subscription content, log in via an institution to check access.
Access this article
Subscribe and save.
- Get 10 units per month
- Download Article/Chapter or eBook
- 1 Unit = 1 Article or 1 Chapter
- Cancel anytime
Price includes VAT (Russian Federation)
Instant access to the full article PDF.
Rent this article via DeepDyve
Institutional subscriptions
Similar content being viewed by others
Computer Vision for 3D Perception
Vision and Learning for Deliberative Monocular Cluttered Flight
Monocular 6-DoF Pose Estimation for Non-cooperative Spacecrafts Using Riemannian Regression Network
Explore related subjects.
- Artificial Intelligence
Zhao P Y, Liu J G, Wu C C. Survey on research and development of on-orbit active debris removal methods. Sci China Tech Sci, 2020, 63: 2188–2210
Article Google Scholar
Yang J, Hou X, Liu Y, et al. A two-level scheme for multiobjective multidebris active removal mission planning in low Earth orbits. Sci China Inf Sci, 2022, 65: 152201
Article MathSciNet Google Scholar
Lillie C F. On-orbit assembly and servicing of future space observatories. In: Proceedings of the Space Telescopes and Instrumentation I: Optical, Infrared, and Millimeter. Orlando: SPIE, 2006. 62652D
Chapter Google Scholar
Ding X L, Wang Y C, Wang Y B, et al. A review of structures, verification, and calibration technologies of space robotic systems for on-orbit servicing. Sci China Tech Sci, 2021, 64: 462–480
Zhai G, Qiu Y, Liang B, et al. On-orbit capture with flexible tether-net system. Acta Astronaut, 2009, 65: 613–623
Feng F, Tang L N, Xu J F, et al. A review of the end-effector of large space manipulator with capabilities of misalignment tolerance and soft capture. Sci China Tech Sci, 2016, 59: 1621–1638
Guariniello C, Delaurentis D A. Maintenance and recycling in space: Functional dependency analysis of on-orbit servicing satellites team for modular spacecraft. In: Proceedings of the AIAA SPACE 2013 Conference and Exposition. San Diego, 2013. 5327
Google Scholar
Cui N G, Wang P, Guo J F, et al. Review on the development of space on-orbit service technology. Acta Astronaut, 2007, 28: 805–811
Pan B, Meng Y. Relative attitude stability analysis of double satellite formation for gravity field exploration in space debris environment. Sci Rep, 2023, 13: 15989
Zhang X F, Chen W, Zhu X C, et al. Space advanced technology demonstration satellite. Sci China Tech Sci, 2024, 67: 240–258
Eilertsen B, Bellido E, Kugelberg J, et al. On-orbit servicing of a geostationary satellite fleet-OLEV as a novel concept for future telecommunication services. In: Proceedings of the 60th IAF Congress. Daejeon, 2009
Kaiser C, Sjöberg F, Delcura J M, et al. SMART-OLEVłAn orbital life extension vehicle for servicing commercial spacecrafts in GEO. Acta Astronaut, 2008, 63: 400–410
Reintsema D, Thaeter J, Rathke A, et al. DEOS-the German robotics approach to secure and de-orbit malfunctioned satellites from low earth orbits. In: Proceedings of the i-SAIRAS. Japan Aerospace Exploration Agency (JAXA), 2010. 244–251
Wolf T. Deutsche Orbitale Servicing Mission. Technical Report, Space-Administration of the German Aerospace Center, 2011
Zhao C Q, Sun Q Y, Zhang C Z, et al. Monocular depth estimation based on deep learning: An overview. Sci China Tech Sci, 2020, 63: 1612–1627
Tang Y, Zhao C, Wang J, et al. Perception and navigation in autonomous systems in the era of learning: A survey. IEEE Trans Neural Netw Learn Syst, 2023, 34: 9604–9624
Xia R H, Zhao C Q, Zheng M, et al. CMDA: Cross-modality domain adaptation for nighttime semantic segmentation. In: Proceedings of the IEEE/CVF International Conference on Computer Vision (ICCV). Piscataway: IEEE, 2023. 21572–21581
Zhao C Q, Poggi M, Tosi F, et al. GasMono: Geometry-aided self-supervised monocular depth estimation for indoor scenes. In: Proceedings of the IEEE/CVF International Conference on Computer Vision (ICCV). Piscataway: IEEE, 2023. 16209–16220
Liu F C. Application of artificial intelligence in spacecraft. Flight Control Detection, 2018, 1: 16–25
Zhang Z, Liu C K, Wang M M, et al. Development and prospects of space intelligent operation (in Chinese). Sci Sin Tech, 2024, 54: 289–303
Lu K, Liu H, Zeng L, et al. Applications and prospects of artificial intelligence in covert satellite communication: A review. Sci China Inf Sci, 2023, 66: 121301
Hao Y M, Fu S F, Fan X P, et al. Vision perception technology for space manipulator on-orbit service operations. Unmanned Syst Tech, 2018, 1: 54–65
Cai H L, Gao Y M, Bing Q J. The research status and key technology analysis of foreign non-cooperative target in space capture system. J Equip Command Acad, 2010, 20: 71–77
Caruso B, Mahendrakar T, Nguyen V M, et al. 3D reconstruction of non-cooperative resident space objects using instant NGP-accelerated nerf and d-nerf. arXiv: 2301.09060
Zhang H P, Liu Z Y, Jiang Z G, et al. BUAA-SID 1.0 space object image dataset. Spacecr Recovery Remote Sens, 2010, 31: 65–71
Papadopoulos E, Aghili F, Ma O, et al. Robotic manipulation and capture in space: A survey. Front Robot AI, 2021, 8: 686723
Card M F, HeardJr W L, Akin D L. Construction and control of large space structures. No. NASA-TM-87689, NASA, 1986. 1–20
Poirier C, Bataille M, Carazo A R, et al. NASA/GSFC. OSAM-1: On-orbit servicing, assembly, and manufacturing-1. 2021, https://www.nasa.gov/mission/on-orbit-servicing-assembly-and-manufacturing-1/
Rajan K, Saffiotti A. Towards a science of integrated AI and robotics. Artif Intell, 2017, 247: 1–9
Bohg J, Ciocarlie M, Civera J, et al. Big data on robotics. Big Data, 2016, 4: 195–196
Kendoul F. Survey of advances in guidance, navigation, and control of unmanned rotorcraft systems. J Field Robot, 2012, 29: 315–378
Li C H, Zou H G, Shi D W, et al. Dual-quaternion-based satellite pose estimation and control with event-triggered data transmission. Sci China Tech Sci, 2023, 66: 1214–1224
Liu M, Liu Q, Zhang L, et al. Adaptive dynamic programming-based fault-tolerant attitude control for flexible spacecraft with limited wireless resources. Sci China Inf Sci, 2023, 66: 202201
Oche P A, Ewa G A, Ibekwe N. Applications and challenges of artificial intelligence in space missions. IEEE Access, 2021, 12: 44481–44509
Zhou R, Liu Y, Qi N, et al. Overview of visual pose estimation methods for space missions. Opt Precis Eng, 2022, 30: 2538–2553
Davis T, Baker M T, Belchak T, et al. XSS-10 micro-satellite flight demonstration program. In: Proceedings of the 17th Annual AIAA/USU Conference on Small Satellites. Logan, 2003
Debus T, Dougherty S. Overview and Performance of the front-end robotics enabling near-term demonstration (FREND) robotic arm. In: Proceedings of the AIAA Infotech@Aerospace Conference and AIAA Unmanned Unlimited Conference. Seattle, 2009. 1870
Barnhart D, Sullivan B, Hunter R, et al. Phoenix program status 2013. In: Proceedings of the AIAA SPACE 2013 Conference and Exposition. San Diego, 2013. 5341
Stéphane E, Jürgen T, Lange M, et al. Definition of an automated vehicle with autonomous fail-safe reaction behavior to capture and deorbit envisat. In: Proceedings of the 7th European Conference on Space Debris. Darmstadt, 2017. 101
Biesbroek R, Innocenti L, Wolahan A, et al. e.Deorbit-ESA’s active debris removal mission. In: Proceedings of the 7th European Conference on Space Debris. Darmstadt, 2017. 18–21
Sedelnikov A V, Salmin V V. Modeling the disturbing effect on the aist small spacecraft based on the measurements data. Sci Rep, 2022, 12: 1300
Wang D Y, Hu Q Y, Hu H D, et al. Review of autonomous relative navigation for non-cooperative spacecraft. Control Theor Appl, 2018, 35: 1392–1404
Opromolla R, Fasano G, Rufino G, et al. A review of cooperative and uncooperative spacecraft pose determination techniques for close-proximity operations. Prog Aerosp Sci, 2017, 93: 53–72
Ruel S, Luu T, Berube A. Space shuttle testing of the TriDAR 3D rendezvous and docking sensor. J Field Robot, 2012, 29: 535–553
Liu L, Zhao G, Bo Y. Point cloud based relative pose estimation of a satellite in close range. Sensors, 2016, 16: 824
Preusker F, Scholten F, Matz K D, et al. Topography of vesta from dawn FC stereo images. In: Proceedings of the European Planetary Science Congress 7. San Francisco, 2012
Shtark T, Gurfil P. Tracking a non-cooperative target using real-time stereovision-based control: An experimental study. Sensors, 2017, 17: 735
Segal S, Carmi A, Gurfil P. Vision-based relative state estimation of non-cooperative spacecraft under modeling uncertainty. In: Proceedings of the 2011 Aerospace Conference. Big Sky, 2011. 1–8
Feng Q, Liu Y, Zhu Z H, et al. Vision-based relative state estimation for a non-cooperative target. In: Proceedings of the 2018 AIAA Guidance, Navigation, and Control Conference. Kissimmee, 2018. 2101
Fourie D, Tweddle B E, Ulrich S, et al. Flight results of vision-based navigation for autonomous spacecraft inspection of unknown objects. J Spacecr Rockets, 2014, 51: 2016–2026
Augenstein S. Monocular pose and shape estimation of moving targets for autonomous rendezvous and docking. Dissertation for the Master’s Degree. California: Stanford University, 2011
Augenstein S, Rock S M. Improved frame-to-frame pose tracking during vision-only SLAM/SFM with a tumbling target. In: Proceedings of the 2011 IEEE International Conference on Robotics and Automation. Shanghai: IEEE, 2011. 3131–3138
Deng R, Wang D, E W, et al. Motion estimation of non-cooperative space objects based on monocular sequence images. Appl Sci, 2022, 12: 12625
Hao G T, Du X. Advances in optical measurement of position and pose for space non-cooperative target. Laser Optoelectron Prog, 2013, 50: 240–248
MathSciNet Google Scholar
Liang B, He Y, Zou Y, et al. Application of time-of-flight camera for relative measurement of non-cooperative target in close range. J Astronaut, 2016, 37: 1080
Zhang S J, Cao X B, Zhang F, et al. Monocular vision-based iterative pose estimation algorithm from corresponding feature points. Sci China Inf Sci, 2010, 53: 1682–1696
Hu H D, Du H, Wang D Y, et al. Feature-extraction and motion-measurement method for noncooperative space targets. Sci Sin-Phys Mech Astron, 2022, 52: 214513
Zeng T, Li C X, Liu Q H, et al. Tracking with nonlinear measurement model by coordinate rotation transformation. Sci China Tech Sci, 2014, 57: 2396–2406
Liang C X, Xue W C, Fang H T, et al. On distributed Kalman filter based state estimation algorithm over a bearings-only sensor network. Sci China Tech Sci, 2023, 66: 3174–3185
Mo Y, Jiang Z H, Li H, et al. A novel space target-tracking method based on generalized Gaussian distribution for on-orbit maintenance robot in Tiangong-2 space laboratory. Sci China Tech Sci, 2019, 62: 1045–1054
Ning X, Chen P, Huang Y, et al. Angular velocity estimation using characteristics of star trails obtained by star sensor for spacecraft. Sci China Inf Sci, 2021, 64: 112209
Ruel S, English C, Anctil M, et al. 3DLASSO: Real-time pose estimation from 3D data for autonomous satellite servicing. In: Proceedings of the ISAIRAS 2005 Conference. Munich, 2005
Blais F, Picard M, Godin G. Accurate 3D acquisition of freely moving objects. In: Proceedings of the 2nd International Symposium on 3D Data Processing, Visualization and Transmission. Thessaloniki: IEEE, 2004. 422–429
Ma Y. Research on proximity capture technology of failure spacecraft based on slam using LiDAR. Nanjing: Nanjing University of Aeronautics and Astronautics, 2018. 32–40
Cao X B, Zhang S J. An iterative method for vision-based relative pose parameters of RVD spacecraft. J Harbin Inst Tech, 2005, 37: 1123–1126
Opromolla R, Fasano G, Rufino G, et al. Uncooperative pose estimation with a LiDAR-based system. Acta Astronaut, 2015, 110: 287–297
Wang K, Liu H, Guo B, et al. A 6D-ICP approach for 3D reconstruction and motion estimate of unknown and non-cooperative target. In: Proceedings of the Chinese Control and Decision Conference. Yinchuan, 2016
Oumer N W, Kriegel S, Ali H, et al. Appearance learning for 3D pose detection of a satellite at close-range. ISPRS J Photogramm Remote Sens, 2017, 125: 1–15
Dor M, Tsiotrasp P. ORB-SLAM applied to spacecraft non-cooperative rendezvous. In: Proceedings of the 2018 Space Flight Mechanics Meeting. Kissimmee, 2018. 1963
Sharma S, D’Amico S. Reduced-dynamics pose estimation for non-cooperative spacecraft rendezvous using monocular vision. In: Proceedings of the 38th AAS Guidance and Control Conference. Breckenridge, 2017
Mu J Z, Wen K R, Liu Z M. Real-time pose estimation for slow rotation non-cooperative targets. Navig Pos Timing, 2020, 7: 114–120
Ge D, Wang D, Zou Y, et al. Motion and inertial parameter estimation of non-cooperative target on orbit using stereo vision. Adv Space Res, 2020, 66: 1475–1484
Peng J, Xu W, Yan L, et al. A pose measurement method of a space noncooperative target based on maximum outer contour recognition. IEEE Trans Aerosp Electron Syst, 2020, 56: 512–526
Liu K, Wang L, Liu H, et al. A relative pose estimation method of non-cooperative space targets. J Phys-Conf Ser, 2022, 2228: 012029
He Y. Modeling and pose measuring of non-cooperative target based on point cloud in close range (in Chinese). Dissertation for the Masters Degree. Harbin: Harbin Institute of Technology, 2017. 5–12
Li Y F, Wang S C, Yang D F, et al. Aerial relative measurement based on monocular reconstruction of non-cooperation target. Chin Space Sci Tech, 2016, 36: 48–56
Dziura M, Wiese T, Harder J. 3D reconstruction in orbital proximity operations. In: Proceedings of the IEEE Aerospace Conference. Big Sky: IEEE, 2017. 1–10
Zhang H, Wei Q, Jiang Z. 3D Reconstruction of space objects from multi-views by a visible sensor. Sensors, 2017, 17: 1689
Stacey N, D’Amico S. Autonomous swarming for simultaneous navigation and asteroid characterization. In: Proceedings of the AIAA/AAS Astrodynamics Specialist Conference. Snowbird, 2018
Dor M, Tsiotras P. ORB-SLAM applied to spacecraft non-cooperative rendezvous. In: Proceedings of the AAS/AIAA Space Flight Mechanics Meeting. Kissimmee, 2018. 1963
Wong X I, Majji M, Singla P. Photometric stereopsis for 3D reconstruction of space objects. Handbook of Dynamic Data Driven Applications Systems. Springer, 2018. 253–291
Chen Z S, Zhang C, Su D, et al. 3D reconstruction of spatial non cooperative target based on improved traditional algorithm. In: Proceedings of the 2021 4th International Conference on Algorithms, Computing and Artificial Intelligence (ACAI). Sanya, 2021. 1–6
Hu C, Wei M, Huang J, et al. A 3-D shape reconstruction strategy for small solar system bodies with single flyby spaceborne radar. Earth Space Sci, 2023, 10: e2022EA002515
Zeng F, Yi J, Wang L, et al. Point cloud 3D reconstruction of non-cooperative object based on multi-satellite collaborations. In: Proceedings of the 2023 3rd Asia-Pacific Conference on Communications Technology and Computer Science (ACCTCS). Shenyang, 2023. 461–467
Dennison K, D’Amico S. Vision-based 3D reconstruction for navigation and characterization of unknown, space-borne targets. Austin, 2023
Moons T. 3D Reconstruction from multiple images Part 1: Principles. FNT Comput Graph Vision, 2010, 4: 287–404
Augenstein S. Monocular pose and shape estimation of moving targets for autonomous rendezvous and docking. Dissertation for Doctoral Degree. Stanford: Stanford University, 2011
Takeishi N, Tanimoto A, Yairi T, et al. Evaluation of interest-region detectors and descriptors for automatic landmark tracking on asteroids. Trans Jpn Soc Aero S Sci, 2015, 58: 45–53
Lowe D G. Distinctive image features from scale-invariant keypoints. Int J Comput Vision, 2004, 60: 91–110
Rublee E, Rabaud V, Konolige K, et al. ORB: An efficient alternative to SIFT or SURF. In: Proceedings of the 2011 International Conference on Computer Vision (ICCV). Barcelona: IEEE, 2011. 2564–2571
Zhou Y, Kuang H Z, Mu J Z. Improved monocular ORB-SLAM for semi-dense 3D reconstruction. Comp Eng Appl, 2021, 57: 180–184
Newcombe R A, Izadi S, Hilliges O, et al. Kinectfusion: Real-time dense surface mapping and tracking. In: Proceedings of the 2011 10th IEEE International Symposium on Mixed and Augmented Reality. Basel: IEEE, 2011. 127–136
Whelan T, Kaess M, Fallon M, et al. Kintinuous: Spatially extended KinectFusion. Robot Auton Syst, 2012, 34: 598–626
Whelan T, Leutenegger S, Salas-Moreno R F, et al. ElasticFusion: Dense SLAM without a pose graph. Robot Sci Syst, 2015, 11: 3
Newcombe R A, Fox D, Seitz S M. Dynamicfusion: Reconstruction and tracking of non-rigid scenes in real-time. In: Proceedings of the IEEE Conference on Computer Vision and Pattern Recognition (CVPR). Piscataway: IEEE, 2015. 343–352
Kisantal M, Sharma S, Park T H, et al. Satellite pose estimation challenge: Dataset, competition design, and results. IEEE Trans Aerosp Electron Syst, 2020, 56: 4083–4098
Sharma S. Pose Estimation of uncooperative spacecraft using monocular vision and deep learning. Dissertation for Doctoral Degree. Stanford: Stanford University, 2019
Beierle C R. High fidelity validation of vision-based sensors and algorithms for spaceborne navigation. Dissertation for Doctoral Degree. Stanford: Stanford University, 2019
Park T H, Martens M, Lecuyer G, et al. SPEED+: Next-generation dataset for spacecraft pose estimation across domain gap. In: Proceedings of the 2022 IEEE Aerospace Conference (AERO). Big Sky: IEEE, 2022. 1–15
Proença P F, Gao Y. Deep learning for spacecraft pose estimation from photorealistic rendering. In: Proceedings of the 2020 IEEE International Conference on Robotics and Automation (ICRA). Paris: IEEE, 2020. 6007–6013
Sharma S, D’Amico S. Pose estimation for non-cooperative rendezvous using neural networks. In: Proceedings of the AAS/AIAA Astrodynamics Specialist Conference. Portland, 2019
Park T H, Sharma S, D’Amico S. Towards robust learning-based pose estimation of noncooperative spacecraft. In: Proceedings of the AAS/AIAA Astrodynamics Specialist Conference. Portland, 2019
Chen B, Cao J, Parra A, et al. Satellite pose estimation with deep landmark regression and nonlinear pose refinement. In: Proceedings of the IEEE/CVF International Conference on Computer Vision Workshops (ICCVW). Piscataway: IEEE, 2019
Qiao S, Zhang H, Meng G, et al. Deep-learning-based satellite relative pose estimation using monocular optical images and 3D structural information. Aerospace, 2022, 9: 768
Gao H, Li Z, Wang N, et al. SU-Net: Pose estimation network for non-cooperative spacecraft on-orbit. Sci Rep, 2023, 13: 11780
Kelsey J M, Byrne J, Cosgrove M, et al. Vision-based relative pose estimation for autonomous rendezvous and docking. In: Proceedings of the IEEE Aerospace Conference. Big Sky: IEEE, 2006. 20
Xu W, Liang B, Li C, et al. Autonomous rendezvous and robotic capturing of non-cooperative target in space. Robotica, 2010, 28: 705–718
Zbontar J, LeCun Y. Stereo matching by training a convolutional neural network to compare image patches. J Mach Learn Res, 2016, 17: 1–32
Luo W, Schwing A G, Urtasun R. Efficient deep learning for stereo matching. In: Proceedings of the IEEE Conference on Computer Vision and Pattern Recognition (CVPR). Piscataway: IEEE, 2016. 5695–5703
Seki A, Pollefeys M. SGM-Nets: Semi-global matching with neural networks. In: Proceedings of the IEEE Conference on Computer Vision and Pattern Recognition (CVPR). Honolulu: IEEE, 2017. 231-
Knobelreiter P, Reinbacher C, Shekhovtsov A, et al. End-to-end training of hybrid CNN-CRF models for stereo. In: Proceedings of the IEEE Conference on Computer Vision and Pattern Recognition (CVPR). Piscataway: IEEE, 2017. 2339–2348
Ji M, Gall J, Zheng H, et al. Surfacenet: An end-to-end 3D neural network for multiview stereopsis. In: Proceedings of the IEEE International Conference on Computer Vision (ICCV). Piscataway: IEEE, 2017. 2307–2315
Kar A, Hane C, Malik J. Learning a multi-view stereo machine. In: Proceedings of the 31st International Conference on Neural Information Processing Systems. Long Beach, 2017
Yao Y, Luo Z, Li S, et al. Mvsnet: Depth inference for unstructured multi-view stereo. In: Proceedings of the European Conference on Computer Vision (ECCV). Berlin: Springer, 2018. 767–783
Chen R, Han S, Xu J, et al. Point-based multi-view stereo network. In: Proceedings of the IEEE/CVF International Conference on Computer Vision (ICCV). Piscataway: IEEE, 2019. 1538–1547
Xu Q, Tao W. Pvsnet: Pixelwise visibility-aware multi-view stereo network. ArXiv: 2007.07714
Xie H, Yao H, Zhang S, et al. Pix2Vox++: Multi-scale context-aware 3D object reconstruction from single and multiple images. Int J Comput Vis, 2020, 128: 2919–2935
Niemeyer M, Mescheder L, Oechsle M, et al. Differentiable volumetric rendering: Learning implicit 3D representations without 3D supervision. In: Proceedings of the IEEE/CVF Conference on Computer Vision and Pattern Recognition (CVPR). Piscataway: IEEE, 2020. 3504–3515
Sitzmann V, Zollhöfer M, Wetzstein G. Scene representation networks: Continuous 3D-structure-aware neural scene representations. arXiv: 1906.01618
Mildenhall B, Srinivasan P P, Tancik M, et al. NeRF. Commun ACM, 2021, 65: 99–106
Müller T, Evans A, Schied C, et al. Instant neural graphics primitives with a multiresolution hash encoding. ACM Trans Graphics, 2022, 41: 1–15
Chen Z, Funkhouser T, Hedman P, et al. Mobilenerf: Exploiting the polygon rasterization pipeline for efficient neural field rendering on mobile architectures. In: Proceedings of the IEEE/CVF Conference on Computer Vision and Pattern Recognition (CVPR). Piscataway: IEEE, 2023. 16569–16578
Cao J, Wang H, Chemerys P, et al. Real-time neural light field on mobile devices. In: Proceedings of the IEEE/CVF Conference on Computer Vision and Pattern Recognition (CVPR). Piscataway: IEEE, 2023. 8328–8337
Pumarola A, Corona E, Pons-Moll G, et al. D-NeRF: Neural radiance fields for dynamic scenes. In: Proceedings of the IEEE/CVF Conference on Computer Vision and Pattern Recognition (CVPR). Piscataway: IEEE, 2021. 10318–10327
Song L, Chen A, Li Z, et al. NeRFPlayer: A streamable dynamic scene representation with decomposed neural radiance fields. IEEE Trans Vis Comput Graph, 2023, 29: 2732–2742
Mildenhall B, Hedman P, Martin-Brualla R, et al. Nerf in the dark: High dynamic range view synthesis from noisy raw images. In: Proceedings of the IEEE/CVF Conference on Computer Vision and Pattern Recognition (CVPR). Piscataway: IEEE, 2022. 16190–16199
Huang X, Zhang Q, Feng Y, et al. HDR-Nerf: High dynamic range neural radiance fields. In: Proceedings of the IEEE/CVF Conference on Computer Vision and Pattern Recognition (CVPR). Piscataway: IEEE, 2022. 18398–18408
Mergy A, Lecuyer G, Derksen D, et al. Vision-based neural scene representations for spacecraft. In: Proceedings of the IEEE/CVF Conference on Computer Vision and Pattern Recognition Workshops (CVPRW). Piscataway: IEEE, 2021. 2002–2011
Schwarz K, Liao Y, Niemeyer M, et al. GRAF: Generative radiance fields for 3D-aware image synthesis. In: Advances in Neural Information Processing Systems. Red Hook: Curran Associates, Inc., 2020. 20154–20166
Dung H A, Chen B, Chin T J. A spacecraft dataset for detection, segmentation and parts recognition. In: Proceedings of the 2021 IEEE/CVF Conference on Computer Vision and Pattern Recognition Workshops (CVPRW). Nashville: IEEE, 2021. 2012–2019
Musallam M A, Gaudilliere V, Ghorbel E, et al. Spacecraft recognition leveraging knowledge of space environment: Simulator, dataset, competition design and analysis. In: Proceedings of the 2021 IEEE International Conference on Image Processing Challenges (ICIPC). IEEE, 2021. 11–15
Musallam M A, Ismaeil K A, Oyedotun O, et al. SPARK: SPAcecraft recognition leveraging knowledge of space environment. arXiv: 2104.05978
Zeng H, Xia Y. Space target recognition based on deep learning. In: Proceedings of the 2017 20th international conference on information fusion (fusion). Xi’an: IEEE, 2017. 1–5
Wu T, Yang X, Song B, et al. T-SCNN: A two-stage convolutional neural network for space target recognition. In: Proceedings of the IGARSS 2019–2019 IEEE International Geoscience and Remote Sensing Symposium. Yokohama: IEEE, 2019. 1334–1337
Chen Y, Gao J, Zhang K. R-CNN-based satellite components detection in optical images. Int J Aerospace Eng, 2020, 2020: 1–10
AlDahoul N, Karim H A, De Castro A, et al. Localization and classification of space objects using EfficientDet detector for space situational awareness. Sci Rep, 2022, 12: 21896
Gong Y, Luo J, Shao H, et al. A transfer learning object detection model for defects detection in X-ray images of spacecraft composite structures. Compos Struct, 2022, 284: 115136
Xiang G, Chen W, Peng Y, et al. Deep transfer learning based on convolutional neural networks for intelligent fault diagnosis of spacecraft. In: Proceedings of the 2020 Chinese Automation Congress (CAC). Shanghai: IEEE, 2020. 5522–5526
AlDahoul N, Karim H A, Momo M A. RGB-D based multi-modal deep learning for spacecraft and debris recognition. Sci Rep, 2022, 12: 3924
Yang X, Nan X, Song B. D2N4: A discriminative deep nearest neighbor neural network for few-shot space target recognition. IEEE Trans Geosci Remote Sens, 2020, 58: 3667–3676
Liu B, Dong Q, Hu Z. Semantic-diversity transfer network for generalized zero-shot learning via inner disagreement based OOD detector. Knowl-Based Syst, 2021, 229: 107337
Lotti A, Modenini D, Tortora P, et al. Deep learning for real time satellite pose estimation on low power edge TPU. arXiv: 2204.03296
Cosmas K, Kenichi A. Utilization of FPGA for onboard inference of landmark localization in CNN-based spacecraft pose estimation. Aerospace, 2020, 7: 159
Wang S, Wang S, Jiao B, et al. CA-SpaceNet: Counterfactual analysis for 6D pose estimation in space. arXiv: 2207.07869
Zhou Z, Zhang Z, Wang Y. Distributed coordinated attitude tracking control of a multi-spacecraft system with dynamic leader under communication delays. Sci Rep, 2022, 12: 15048
Fazlyab A R, Fani Saberi F, Kabganian M. Fault-tolerant attitude control of the satellite in the presence of simultaneous actuator and sensor faults. Sci Rep, 2023, 13: 20802
Yang M F, Liu B, Gong J, et al. Architecture design for reliable and reconfigurable FPGA-based GNC computer for deep space exploration. Sci China Tech Sci, 2016, 59: 289–300
Xia K, Zou Y. Performance-guaranteed adaptive fault-tolerant tracking control of six-DOF spacecraft. Sci China Inf Sci, 2023, 66: 119202
Moghaddam B M, Chhabra R. On the guidance, navigation and control of in-orbit space robotic missions: A survey and prospective vision. Acta Astronaut, 2021, 184: 70–100
Hao Z, Shyam R B A, Rathinam A, et al. Intelligent spacecraft visual GNC architecture with the state-of-the-art AI components for on-orbit manipulation. Front Robot AI, 2021, 8: 639327
Aghili F, Parsa K. Motion and parameter estimation of space objects using laser-vision data. J Guid Control Dyn, 2009, 32: 538–550
Segal S, Carmi A, Gurfil P. Vision-based relative state estimation of non-cooperative spacecraft under modeling uncertainty. In: Proceedings of the 2011 Aerospace Conference. Big Sky: IEEE, 2011. 1–8
Pesce V, Lavagna M, Bevilacqua R. Stereovision-based pose and inertia estimation of unknown and uncooperative space objects. Adv Space Res, 2017, 59: 236–251
Shafaei A, Little J J, Schmidt M. Play and learn: Using video games to train computer vision models. arXiv: 1608.01745
Richter S R, Vineet V, Roth S, et al. Playing for data: Ground truth from computer games. In: Proceedings of the European Conference on Computer Vision (ECCV). Amsterdam: Springer, 2016. 102–118
Abu Alhaija H, Mustikovela S K, Mescheder L, et al. Augmented reality meets computer vision: Efficient data generation for urban driving scenes. Int J Comput Vis, 2018, 126: 961–972
Dewi C, Chen R C, Liu Y T, et al. Synthetic Data generation using DCGAN for improved traffic sign recognition. Neural Comput Applic, 2022, 34: 21465–21480
Wang Y, Yao Q, Kwok J T, et al. Generalizing from a few examples. ACM Comput Surv, 2021, 53: 1–34
Dosovitskiy A, Beyer L, Kolesnikov A, et al. An image is worth 16 × 16 words: Transformers for image recognition at scale. In: Proceedings of the International Conference on Learning Representations (ICLR). 2021
Zhao C, Zhang Y, Poggi M, et al. Monovit: Self-supervised monocular depth estimation with a vision transformer. In: Proceedings of the 2022 International Conference on 3D Vision (3DV). Prague: IEEE, 2022. 668–678
Likhosherstov V, Arnab A, Choromanski K, et al. Polyvit: Co-training vision transformers on images, videos and audio. arXiv: 2111.12993
Shao J, Chen S, Li Y, et al. Intern: A new learning paradigm towards general vision. arXiv: 2111.08687
Wu T, He S, Liu J, et al. A brief overview of ChatGPT: The history, status quo and potential future development. IEEE CAA J Autom Sin, 2023, 10: 1122–1136
Lin C H, Gao J, Tang L, et al. Magic3D: High-resolution text-to-3D content creation. In: Proceedings of the IEEE/CVF Conference on Computer Vision and Pattern Recognition (CVPR). Piscataway: IEEE, 2023. 300–309
Poole B, Jain A, Barron J T, et al. Dreamfusion: Text-to-3D using 2D diffusion. arXiv: 2209.14988
Yang T, Ying Y. AUC maximization in the era of big data and AI: A survey. ACM Comput Surv, 2023, 55: 1–37
Goel R, Sirikonda D, Saini S, et al. Interactive segmentation of radiance fields. In: Proceedings of the IEEE/CVF Conference on Computer Vision and Pattern Recognition (CVPR). Piscataway: IEEE, 2023. 4201–4211
Yuan Y J, Sun Y T, Lai Y K, et al. Nerf-editing: Geometry editing of neural radiance fields. In: Proceedings of the IEEE/CVF Conference on Computer Vision and Pattern Recognition (CVPR). Piscataway: IEEE, 2022. 18332–18343
von Rueden L, Mayer S, Beckh K, et al. Informed machine learning—A taxonomy and survey of integrating prior knowledge into learning systems. IEEE Trans Knowl Data Eng, 2021, 35: 614–633
Roscher R, Bohn B, Duarte M F, et al. Explainable machine learning for scientific insights and discoveries. IEEE Access, 2020, 8: 42200–42216
Raissi M, Perdikaris P, Karniadakis G E. Physics informed deep learning (Part I): Data-driven solutions of nonlinear partial differential equations. ArXiv: 1711.10561
Schiassi E, D’Ambrosio A, Scorsoglio A, et al. Class of optimal space guidance problems solved via indirect methods and physics-informed neural networks. In: Proceedings of the 31st AAS/AIAA Space Flight Mechanics Meeting. 2021
Xu X, Zhang L, Yang J, et al. A review of multi-sensor fusion slam systems based on 3D LiDAR. Remote Sens, 2022, 14: 2835
Aguileta A A, Brena R F, Mayora O, et al. Multi-sensor fusion for activity recognitionłA survey. Sensors, 2019, 19: 3808
Wang Z, Wu Y, Niu Q. Multi-sensor fusion in automated driving: A survey. IEEE Access, 2019, 8: 2847–2868
Liang M, Yang B, Chen Y, et al. Multi-task multi-sensor fusion for 3D object detection. In: Proceedings of the IEEE/CVF conference on computer vision and pattern recognition (CVPR). Piscataway: IEEE, 2019. 7345–7353
Li Z, Tang Y, Fan Y, et al. Formation control of multi-agent systems with constrained mismatched compasses. IEEE Trans Netw Sci Eng, 2022, 9: 2224–2236
Wang J, Hong Y, Wang J, et al. Cooperative and competitive multiagent systems: From optimization to games. IEEE CAA J Autom Sin, 2022, 9: 763–783
Hong Y, Jin Y, Tang Y. Rethinking individual global max in cooperative multi-agent reinforcement learning. In: Proceedings of the 36th International Conference on Neural Information Processing Systems. New Orleans, 2022. 32438–32449
Santi G, Corso A J, Garoli D, et al. Swarm of lightsail nanosatellites for Solar System exploration. Sci Rep, 2023, 13: 19583
Di Mauro G, Lawn M, Bevilacqua R. Survey on guidance navigation and control requirements for spacecraft formation-flying missions. J Guid Control Dyn, 2018, 41: 581–602
Jin X, Ho D W C, Tang Y. Synchronization of multiple rigid body systems: A survey. Chaos-An Interdiscipl J Nonlinear Sci, 2023, 33: 092102
Tapley B D, Bettadpur S, Watkins M, et al. The gravity recovery and climate experiment: Mission overview and early results. Geophys Res Lett, 2004, 31: 2004GL019920
Krieger G, Moreira A, Fiedler H, et al. TanDEM-X: A satellite formation for high-resolution SAR interferometry. IEEE Trans Geosci Remote Sens, 2007, 45: 3317–3341
Sanchez H, McIntosh D, Cannon H, et al. Starling1: Swarm technology demonstration. In: Proceedings of the 32nd Annual Small Satellite Conference, AIAA/USU. Logan, 2018
Stacey N, Dennison K, D’Amico S. Autonomous asteroid characterization through nanosatellite swarming. In: Proceedings of the 2022 IEEE Aerospace Conference (AERO). Big Sky: IEEE, 2022. 1–21
Stacey N, D’Amico S. Autonomous swarming for simultaneous navigation and asteroid characterization. In: Proceedings of AAS/AIAA Astrodynamics Specialist Conference. 2018. 1: 76
Giuffrida G, Nannipieri P, Diana L, et al. Satellite instrument control unit with artificial intelligence engine on a single chip: ICU4SAT. In: Proceedings of the European Workshop on On-Board Data Processing (OBDP). 2021. 14–17
Leon V, Minaidis P, Lentaris G, et al. Accelerating AI and computer vision for satellite pose estimation on the intel myriad X embedded SoC. Microprocess MicroSyst, 2023, 103: 104947
Lagunas E, Ortiz F, Eappen G, et al. Performance evaluation of neuro-morphic hardware for onboard satellite communication applications. arXiv: 2401.06911
Download references
Author information
Authors and affiliations.
School of Information Science and Engineering, East China University of Science and Technology, Shanghai, 200237, China
HuiLin Chen, QiYu Sun & Yang Tang
School of Mathematics, East China University of Science and Technology, Shanghai, 200237, China
You can also search for this author in PubMed Google Scholar
Corresponding authors
Correspondence to FangFei Li or Yang Tang .
Additional information
This work was supported by the National Natural Science Foundation of China (Grant Nos. 62233005 and 62293502), the Programme of Introducing Talents of Discipline to Universities (the 111 Project) (Grant No. B17017), the Fundamental Research Funds for the Central Universities (Grant No. 222202417006), and Shanghai AI Lab.
Rights and permissions
Reprints and permissions
About this article
Chen, H., Sun, Q., Li, F. et al. Computer vision tasks for intelligent aerospace perception: An overview. Sci. China Technol. Sci. (2024). https://doi.org/10.1007/s11431-024-2714-4
Download citation
Received : 08 March 2024
Accepted : 12 June 2024
Published : 21 August 2024
DOI : https://doi.org/10.1007/s11431-024-2714-4
Share this article
Anyone you share the following link with will be able to read this content:
Sorry, a shareable link is not currently available for this article.
Provided by the Springer Nature SharedIt content-sharing initiative
- deep learning
- aerospace missions
- pose estimation
- 3D reconstruction
- recognition
- Find a journal
- Publish with us
- Track your research

IMAGES
COMMENTS
In this thesis, I investigate three directions to achieve an effective interac... Expand abstract. ... Multi-Modal Deep Learning for Computer Vision and Its Application [PhD thesis]. University of Oxford. Copy APA Style MLA Style. Li, B. Multi-Modal Deep Learning for Computer Vision and Its Application. University of Oxford, 2023. Copy MLA ...
This thesis consists of six chapters. Each of the main chapters introduces an end-to-end deep learning model and discusses how to apply the ideas of geometry and uncertainty. Chatper 1 - Introduction. Motivates this work within the wider field of computer vision. Chapter 2 - Scene Understanding.
The doctoral dissertation represents the culmination of the entire graduate school experience. It is a snapshot of all that a student has accomplished and learned about their dissertation topics. ... In computer vision classification problems, it is often possible to generate an informative feature vector representation of an image, for example ...
AND COMPUTER VISION A DISSERTATION SUBMITTED TO THE DEPARTMENT OF COMPUTER SCIENCE AND THE COMMITTEE ON GRADUATE STUDIES OF STANFORD UNIVERSITY ... Those values are what led me through my PhD and let me have fun in the process. And speaking of love and support, thank you Eaming for
Please read our instructions for preparing and delivering your work. PhD Theses Master Theses Bachelor Theses Thesis Topics. Below we list possible thesis topics for Bachelor and Master students in the areas of Computer Vision, Machine Learning, Deep Learning and Pattern Recognition. The project descriptions leave plenty of room for your own ideas.
Computer vision is a subfield of artificial intelligence (AI), focused on automizing the analysis of images and videos. This dissertation offers a critical analysis of computer vision, discussing the potential ethical and societal implications of the technology. The critical analysis is based on a new approach to AI ethics, which is inspired by ...
In this thesis, we develop theoretical, algorithmic and experimental contributions for Machine Learning with limited labels, and more specifically for the tasks of Image Classification and Object Detection in Computer Vision. In a first contribution, we are interested in bridging the gap between theory and practice for popular Meta-Learning algorithms used in Few-Shot Classification. We make ...
Geometric and Structural-based Symbol Spotting. Application to Focused Retrieval in Graphic Document Collections. Theory and Algorithms on the Median Graph. Application to Graph-based. List of PhD theses on Computer Vision. Search by topic, find your next reading and download the full pdf or watch the thesis defence.
Deep Representation Learning in Computer Vision and Its Applications Fangyu Wu state-of-the-art performance on several benchmark datasets. For the few-shot classi cation, there are two main contributions in this thesis: rstly, we attempt to tackle the few-shot classi cation problem based on a novel representation learning
We investigate the role of attention and memory in complex reasoning tasks. We analyze Transformer-based self-attention as a model and extend it with memory. By studying a synthetic visual reasoning test, we refine the taxonomy of reasoning tasks. Incorporating self-attention with ResNet50, we enhance feature maps using feature-based and spatial attention, achieving efficient solving of ...
D-HEST Health Sciences and Technology. D-INFK Computer Science. D-ITET Information Technology and Electrical Engineering. D-MATH Mathematics. D-MATL Department of Materials. D-MAVT Mechanical and Process Engineering. D-MTEC Management, Technology and Economics. D-PHYS Physics. D-USYS Environmental Systems Science.
The aim of this PhD project is to advance the state-of-the-art in computer vision through the development and application of self-supervised learning (SSL) techniques. Read more. Supervisor: Prof B Li. 1 October 2024 PhD Research Project Self-Funded PhD Students Only.
This thesis considers the use of synthetic data to allow the use of DNN to solve problems in computer vision. First, we consider using synthetic data for problems where collection of real data is not feasible. We focus on the problem of magnifying small motion in videos. Using synthetic data allows us to train DNN models that magnify motion ...
Deep learning has become part of many state-of-the-art systems in multiple disciplines (specially in computer vision and speech processing). In this thesis Convolutional Neural Networks are used to solve the problem of recognizing people in images, both for verification and identification. Two different architectures, AlexNet and VGG19, both ...
computer is making a decision, image captioning could be used to ask a human for help or feedback. 1.2 Contributions of this Thesis This thesis presents work toward data-driven approaches to image captioning. In this work, vison and language features are learned jointly in a statistical model that is trained on images and human-
This Thesis describe a system for detecting and tracking peoples , from image and depth sen-sors data, to cope with the challenges of MAS perception.Our focus is on developing robust computer vision algorithms that provide robustness and efficiency for people detection and tracking from the MAS in real-time applications as mentioned earlier.
This thesis embarks on an exploratory journey into robustness in deep learning, with a keen focus on the intertwining facets of generalization, explainability, and edge cases within the realm of computer vision. In deep learning, robustness epitomizes a model's resilience and flexibility, grounded on its capacity to generalize across diverse ...
With respect to undergraduate thesis topics looking at Computer Vision applications is one place to start. The OpenCV library is another. And talking to potential supervisors at your university is also a good idea. With respect to PhD thesis topics, it's important to take into consideration what the fields of expertise of your potential ...
Video (online) Consult the top 50 dissertations / theses for your research on the topic 'Computer Science. Computer vision.'. Next to every source in the list of references, there is an 'Add to bibliography' button. Press on it, and we will generate automatically the bibliographic reference to the chosen work in the citation style you need: APA ...
Thesis: Ph. D., Massachusetts Institute of Technology, Department of Electrical Engineering and Computer Science, 2016. ... Our work impacts a variety of fields, ranging from computer vision, to long-distance structural health monitoring and nondestructive testing, surveillance, and even visual effects for film. By imaging the vibrations of ...
CSSA Sample PhD proposals. Purpose. Welcome to the on-line version of the UNC dissertation proposal collection. The purpose of this collection is to provide examples of proposals for those of you who are thinking of writing a proposal of your own. I hope that this on-line collection proves to be more difficult to misplace than the physical ...
Design, Deployment, and Validation of Computer Vision Techniques for Societal Scale Applications, Arup Kanti Dey. PDF. AffectiveTDA: Using Topological Data Analysis to Improve Analysis and Explainability in Affective Computing, Hamza Elhamdadi. PDF. Automatic Detection of Vehicles in Satellite Images for Economic Monitoring, Cole Hill. PDF
Theses/Dissertations from 2022. PDF. The Design and Implementation of a High-Performance Polynomial System Solver, Alexander Brandt. PDF. Defining Service Level Agreements in Serverless Computing, Mohamed Elsakhawy. PDF. Algorithms for Regular Chains of Dimension One, Juan P. Gonzalez Trochez. PDF.
Computer vision tasks are crucial for aerospace missions as they help spacecraft to understand and interpret the space environment, such as estimating position and orientation, reconstructing 3D models, and recognizing objects, which have been extensively studied to successfully carry out the missions. However, traditional methods like Kalman filtering, structure from motion, and multi-view ...