- Search Menu
- Sign in through your institution
- Advance articles
- Author Guidelines
- Submission Site
- Open Access
- Why publish?
- About Journal of Semantics
- Editorial Board
- Advertising and Corporate Services
- Journals Career Network
- Self-Archiving Policy
- Dispatch Dates
- Terms and Conditions
- Journals on Oxford Academic
- Books on Oxford Academic


Managing Editor
Emmanuel Chemla
About the journal
Journal of Semantics covers all areas in the study of meaning, with a focus on formal and experimental methods. It welcomes submissions on semantics, pragmatics, the syntax/semantics interface, cross-linguistic semantics, experimental studies of meaning, and semantically informed philosophy of language.
Latest articles

Editor's choice
Read a selection of the editor's favourite articles from Journal of Semantic 's archives, for free.

Open Access articles
Explore the full archive of Open Access articles from the Journal of Semantics .

Short contributions
Introducing a new article type: short contributions will be Open Access, and should be compact yet make an influential contribution to the field.
Resources for Authors and Researchers

Interested in submitting your research?
Read the Instructions for Authors and learn more about the Applied Linguistics submission process and requirements.
Make an impact with your work
Have you published an article? What should you do now? Read our top tips on promoting your work to reach a wider audience and ensure your work makes an impact.
Top Tips for Publishing in Linguistics Journals
Watch our top tips for publishing in Linguistics Journals video, featuring helpful advice from our Linguistics Journals editors.

Email alerts
Register to receive table of contents email alerts as soon as new issues of Journal of Semantics are published online.

Recommend to your library
Fill out our simple online form to recommend Journal of Semantics to your library.
Recommend now
The Journal of Semantics is accepting high quality clinical and scientific papers relating to all aspects of semantics.
Related Titles
- Recommend to your Library
Affiliations
- Online ISSN 1477-4593
- Print ISSN 0167-5133
- Copyright © 2024 Oxford University Press
- About Oxford Academic
- Publish journals with us
- University press partners
- What we publish
- New features
- Open access
- Institutional account management
- Rights and permissions
- Get help with access
- Accessibility
- Advertising
- Media enquiries
- Oxford University Press
- Oxford Languages
- University of Oxford
Oxford University Press is a department of the University of Oxford. It furthers the University's objective of excellence in research, scholarship, and education by publishing worldwide
- Copyright © 2024 Oxford University Press
- Cookie settings
- Cookie policy
- Privacy policy
- Legal notice
This Feature Is Available To Subscribers Only
Sign In or Create an Account
This PDF is available to Subscribers Only
For full access to this pdf, sign in to an existing account, or purchase an annual subscription.
Semantics and Pragmatics
Semantics and Pragmatics, founded in 2007 and first published in 2008, is a Diamond Open Access journal published by the Linguistic Society of America.
Current Issue
Vol. 17 (2024)
Published: 2024-01-05
Main Articles
The semantics and probabilistic pragmatics of deadjectival intensifiers, free choice and presuppositional exhaustification, formalizing spatial-causal polysemy of agent prepositions, covert mixed quotation, indefinites in negated intensional contexts an argument for world-skolemized choice functions, metalinguistic gradability, intensional anaphora, cataphoric resolution of projective content the case of occasion verbs, modal indefinites and semantic variation lessons from chuj, squibs, remarks, and replies, limitations of a modal analysis of before and after, strategies for anderson conditionals their implications to the typology of o- and x-markings, social identity affects imprecision resolution across different tasks, previous six years (2023-2018).
Vol. 16 (2023)
Published: 2023-01-05
Context Dynamics
Probabilities and logic in implicature computation.
Two puzzles with embedded disjunction
Putting oughts together
Using the anna karenina principle to explain why cause favors negative-sentiment complements, imperatives in a dynamic pragmatics, a square of necessities.
X-marking weak and strong necessity modals
A semantic universal for modality
Pair-list answers to questions with plural definites.
Vol. 15 (2022)
Published: 2022-01-19
“More is up” for domain restriction in ASL
Referential transparency as the proper treatment for quantification, logic and conversation.
The case of free choice
Keep only strong
Exceptional wide scope of bare nominals, pragmatic reasoning and semantic convention.
A case study on gradable adjectives
Attentional Pragmatics
A new pragmatic approach to exhaustivity
Two paths to habituality
The semantics of habitual mode in Tlingit
Probabilistic modeling of rational communication with conditionals
Belief or consequences, presupposition projection as a scope phenomenon, alternatives and attention in language and reasoning.
A reply to Mascarenhas & Picat 2019
Varieties of Hurford disjunctions
The role of alternatives in the interpretation of scalars and numbers.
Insights from the inference task
Scorekeeping in a chess game
Vol. 14 (2021)
Published: 2021-03-02
Fine-grained semantics for attitude reports
Formal properties of now revisited, explaining gaps in the logical lexicon of natural languages.
A decision-theoretic perspective on the square of Aristotle
A variably exhaustive and scalar focus particle and pragmatic focus concord in Burmese
The landscape of speech reporting, the most , the fewest and the least.
On the relative readings of quantity superlatives
Anyone might but everyone won’t
Presuppositional exhaustification, actualistic interpretations in french, negative free choice, npis, intervention, and collectivity, does intonation automatically strengthen scalar implicatures, on believing and hoping whether.
Vol. 13 (2020)
Published: 2020-04-04
Understanding focus
Pitch, placement and coherence
Unveiling multiple wh - free relative clauses and their functional wh -words
Numerals and the theory of number, a preference semantics for imperatives, multi-modal meaning.
An empirically-founded process algebra approach
Conjectural questions
The case of German verb-final wohl questions
Reciprocity
Anaphora, scope, and quantification
Predicting the end
Epistemic change in Romance
Weak necessity without weak possibility
The composition of modal strength distinctions in Javanese
Expressions in focus
Compositional trace conversion, similative plurality and the nature of alternatives, intervention in deontic reasoning, numeral differential constructions in arabic, non-informative assertions.
The case of non-optional wh -in-situ
Eavesdropping
What is it good for?
The logic of Quantifier Raising
Evidential meaning and (not-)at-issueness, alternative questions.
Distinguishing between negated and complementary disjuncts
The semantics and pragmatics of nouns in concealed questions
Vol. 12 (2019)
Published: 2019-11-14
Donkeys under discussion
Modal interpretation of tense in subjunctive conditionals, different again, learnability and semantic universals, distributive ignorance inferences with wonder and believe, the proprial article and the semantics of names, the discourse commitments of illocutionary reportatives, coercion by modification.
The adaptive capacities of event-sensitive adnominal modifiers
Experimental studies on it -clefts and predicate interpretation
Attitudes in discourse.
Italian polar questions and the particle mica
Pluralities across categories and plural projection
Polarity reversals under sluicing, know whether and -ever free relative clauses, free choice and homogeneity, on the grammatical source of adjective ordering preferences, where is the destructive update problem, filtering free choice, anankastic conditionals are still a mystery, polarity particles revisited, sluicing on free choice, presupposing counterfactuality, vagueness, contextualism, and ellipsis.
Vol. 11 (2018)
Published: 2018-02-09
Symmetric predicates and the semantics of reciprocal alternations
Counterfactual de se, that’s not quite it.
An experimental investigation of (non‑)exhaustivity in clefts
Disentangling two distinct notions of NEG raising
Might do better: flexible relativism and the qud, a formal semantics for situated conversation, free choice and distribution over disjunction.
The case of free choice ability
Reconstructing the syntax of focus operators
The case of the missing ‘if’.
Accessibility relations in Stalnaker’s theory of conditionals
Complex sentential operators refute unrestricted Simplification of Disjunctive Antecedents
A Survey of Semantic Analysis Approaches
- Conference paper
- First Online: 24 March 2020
- Cite this conference paper
- Said A. Salloum ORCID: orcid.org/0000-0002-6073-3981 19 , 20 ,
- Rehan Khan 19 &
- Khaled Shaalan ORCID: orcid.org/0000-0003-0823-8390 19
Part of the book series: Advances in Intelligent Systems and Computing ((AISC,volume 1153))
Included in the following conference series:
- The International Conference on Artificial Intelligence and Computer Vision
4696 Accesses
42 Citations
Semantics is a branch of linguistics, which aims to investigate the meaning of language. Semantics deals with the meaning of sentences and words as fundamentals in the world. Semantic analysis within the framework of natural language processing evaluates and represents human language and analyzes texts written in the English language and other natural languages with the interpretation similar to those of human beings. This study aimed to critically review semantic analysis and revealed that explicit semantic analysis, latent semantic analysis, and sentiment analysis contribute to the leaning of natural languages and texts, enable computers to process natural languages, and reveal opinion attitudes in texts. The future prospect is in the domain of sentiment lexes. The overall results of the study were that semantics is paramount in processing natural languages and aid in machine learning. This study has covered various aspects including the Natural Language Processing (NLP), Latent Semantic Analysis (LSA), Explicit Semantic Analysis (ESA), and Sentiment Analysis (SA) in different sections of this study. However, LSA has been covered in detail with specific inputs from various sources. This study also highlights the future prospects of semantic analysis domain and finally the study is concluded with the result section where areas of improvement are highlighted and the recommendations are made for the future research. This study also highlights the weakness and the limitations of the study in the discussion (Sect. 4 ) and results (Sect. 5 ).
This is a preview of subscription content, log in via an institution to check access.
Access this chapter
Subscribe and save.
- Get 10 units per month
- Download Article/Chapter or eBook
- 1 Unit = 1 Article or 1 Chapter
- Cancel anytime
- Available as PDF
- Read on any device
- Instant download
- Own it forever
- Available as EPUB and PDF
- Compact, lightweight edition
- Dispatched in 3 to 5 business days
- Free shipping worldwide - see info
Tax calculation will be finalised at checkout
Purchases are for personal use only
Institutional subscriptions
Similar content being viewed by others
ESWC 15 Challenge on Concept-Level Sentiment Analysis
Challenge on Fine-Grained Sentiment Analysis Within ESWC2016
Semantic Sentiment Analysis Challenge at ESWC2018
Alshurideh, M.T., Salloum, S.A., Al Kurdi, B., Monem, A.A., Shaalan, K.: Understanding the quality determinants that influence the intention to use the mobile learning platforms: a practical study. Int. J. Interact. Mob. Technol. 13 (11), 157–183 (2019)
Article Google Scholar
Salloum, S.A.S., Shaalan, K.: Investigating students’ acceptance of e-learning system in higher educational environments in the UAE: applying the extended technology acceptance model (TAM). The British University in Dubai (2018)
Google Scholar
Salloum, S.A., Al-Emran, M., Shaalan, K., Tarhini, A.: Factors affecting the E-learning acceptance: a case study from UAE. Educ. Inf. Technol. 24 , 1–22 (2018)
Salloum, S.A., Alhamad, A.Q.M., Al-Emran, M., Monem, A.A., Shaalan, K.: Exploring students’ acceptance of E-learning through the development of a comprehensive technology acceptance model. IEEE Access 7 , 128445–128462 (2019)
Mhamdi, C., Al-Emran, M., Salloum, S.A.: Text mining and analytics: a case study from news channels posts on Facebook, vol. 740 (2018)
Salloum, S.A., AlHamad, A.Q., Al-Emran, M., Shaalan, K.: A survey of Arabic text mining, vol. 740 (2018)
Salloum, S.A., Al-Emran, M., Abdallah, S., Shaalan, K.: Analyzing the Arab Gulf newspapers using text mining techniques. In: International Conference on Advanced Intelligent Systems and Informatics, pp. 396–405 (2017)
Salloum, S.A., Al-Emran, M., Shaalan, K.: Mining social media text: extracting knowledge from Facebook. Int. J. Comput. Digit. Syst. 6 (2), 73–81 (2017)
Salloum, S.A., Al-Emran, M., Monem, A.A., Shaalan, K.: Using text mining techniques for extracting information from research articles. In: Studies in Computational Intelligence, vol. 740. Springer (2018)
Salloum, S.A., Mhamdi, C., Al-Emran, M., Shaalan, K.: Analysis and classification of Arabic newspapers’ Facebook pages using text mining techniques. Int. J. Inf. Technol. Lang. Stud. 1 (2), 8–17 (2017)
Salloum, S.A., Al-Emran, M., Shaalan, K.: Mining text in news channels: a case study from Facebook. Int. J. Inf. Technol. Lang. Stud. 1 (1), 1–9 (2017)
Salloum, S.A., Al-Emran, M., Monem, A.A., Shaalan, K.: A survey of text mining in social media: Facebook and Twitter perspectives. Adv. Sci. Technol. Eng. Syst. J 2 (1), 127–133 (2017)
Chien, J.-T., Wu, M.-S.: Adaptive Bayesian latent semantic analysis. IEEE Trans. Audio. Speech. Lang. Processing 16 (1), 198–207 (2007)
Chang, C.-H., Kayed, M., Girgis, M.R., Shaalan, K.F.: A survey of web information extraction systems. IEEE Trans. Knowl. Data Eng. 18 (10), 1411–1428 (2006)
Al-Emran, M., Mezhuyev, V., Kamaludin, A., Shaalan, K.: The impact of knowledge management processes on information systems: a systematic review. Int. J. Inf. Manage. 43 (July), 173–187 (2018)
Evangelopoulos, N., Zhang, X., Prybutok, V.R.: Latent semantic analysis: five methodological recommendations. Eur. J. Inf. Syst. 21 (1), 70–86 (2012)
Kou, G., Peng, Y.: An application of latent semantic analysis for text categorization. Int. J. Comput. Commun. Control 10 (3), 357–369 (2015)
Alhashmi, S.F.S., Salloum, S. A., Abdallah, S.: Critical success factors for implementing artificial intelligence (AI) projects in dubai government United Arab Emirates (UAE) health sector: applying the extended technology acceptance model (TAM). In: International Conference on Advanced Intelligent Systems and Informatics, pp. 393–405(2019)
Rajani, S., Hanumanthappa, M.: Techniques of semantic analysis for natural language processing – a detailed survey. Int. J. Adv. Res. Comput. Commun. Eng. 5 (2) (2016)
Farghaly, A., Shaalan, K.: Arabic natural language processing: challenges and solutions. ACM Trans. Asian Lang. Inf. Process. 8 (4), 14 (2009)
Liu, X., He, P., Chen, W., Gao, J.: Multi-task deep neural networks for natural language understanding. arXiv Prepr. arXiv1901.11504 (2019)
Cambria, E., White, B.: Jumping NLP curves: a review of natural language processing research. IEEE Comput. Intell. Mag. 9 (2), 48–57 (2014)
Mahesh, K., Nirenburg, S.: Semantic classification for practical natural language processing. In: Proceedings of Sixth ASIS SIG/CR Classification Research Workshop: An Interdisciplinary Meeting, pp. 116–139 (1995)
Chowdhury, G.G.: Natural language processing. Annu. Rev. Inf. Sci. Technol. 37 (1), 51–89 (2003)
Article MathSciNet Google Scholar
Calcagno, M.V., Barklund, P.J., Zhao, L., Azzam, S., Knoll, S.S., Chang, S.: Semantic analysis system for interpreting linguistic structures output by a natural language linguistic analysis system. Google Patents, 13 February 2007
Katayama, Y., Nakanishi, K., Yoshiura, H., Hirasawa, K.: System for processing natural language including identifying grammatical rule and semantic concept of an undefined word. Google Patents, 28 April 1992
Khan, A., Baharudin, B., Lee, L.H., Khan, K.: A review of machine learning algorithms for text-documents classification. J. Adv. Inf. Technol. 1 (1), 4–20 (2010)
Hutchison, P.D., Daigle, R.J., George, B.: Application of latent semantic analysis in AIS academic research. Int. J. Account. Inf. Syst. 31 , 83–96 (2018)
Hofmann, T.: Unsupervised learning by probabilistic latent semantic analysis. Mach. Learn. 42 (1–2), 177–196 (2001)
Article MATH Google Scholar
Gabrilovich, E., Markovitch, S.: Computing semantic relatedness using Wikipedia-based explicit semantic analysis. In: IJcAI, vol. 7, pp. 1606–1611 (2007)
Witten, I.H., Milne, D.N.: An effective, low-cost measure of semantic relatedness obtained from Wikipedia links (2008)
Akerkar, R.: Natural language processing. In: Artificial Intelligence for Business, pp. 53–62. Springer (2019)
Mäntylä, M.V., Graziotin, D., Kuutila, M.: The evolution of sentiment analysis—a review of research topics, venues, and top cited papers. Comput. Sci. Rev. 27 , 16–32 (2018)
Nasukawa, T., Yi, J.: Sentiment analysis: capturing favorability using natural language processing. In: Proceedings of the 2nd International Conference on Knowledge Capture, pp. 70–77 (2003)
Abraham, A., Hassanien, A.-E., Snášel, V.: Computational Social Network Analysis: Trends. Tools and Research Advances. Springer, Heidelberg (2009)
Ghali, N., Panda, M., Hassanien, A.E., Abraham, A., Snasel, V.: Social networks analysis: tools, measures and visualization. In: Computational Social Networks, pp. 3–23. Springer (2012)
Salloum, S.A., Mhamdi, C., Al Kurdi, B., Shaalan, K.: Factors affecting the adoption and meaningful use of social media: a structural equation modeling approach. Int. J. Inf. Technol. Lang. Stud. 2 (3), 96–109 (2018)
Al-Maroof, R.S., Salloum, S.A., AlHamadand, A.Q.M., Shaalan, K.: A unified model for the use and acceptance of stickers in social media messaging. In: International Conference on Advanced Intelligent Systems and Informatics, pp. 370–381 (2019)
Agarwal, A., Xie, B., Vovsha, I., Rambow, O., Passonneau, R.: Sentiment analysis of Twitter data. In: Proceedings of the Workshop on Language in Social Media (LSM 2011), pp. 30–38 (2011)
Baets, W., Oldenboom, E., Hosken, C.: The potential of semantic analysis for business (education). Available SSRN 3364133 (2019)
Salloum, S.A., Al-Emran, M., Shaalan, K.: A survey of lexical functional grammar in the Arabic context. Int. J. Com. Net. Tech. 4 (3), 141–147 (2016)
Godbole, N., Srinivasaiah, M., Skiena, S.: Large-scale Sentiment analysis for news and blogs. Icwsm 7 (21), 219–222 (2007)
Delmonte, R. Pallotta, V.: Opinion mining and sentiment analysis need text understanding. In: Advances in Distributed Agent-Based Retrieval Tools, pp. 81–95. Springer (2011)
Al-Batah, M.S., Mrayyen, S., Alzaqebah, M.: Investigation of naive bayes combined with multilayer perceptron for arabic sentiment analysis and opinion mining. JCS 14 (8), 1104–1114 (2018)
Chopra, F.K., Bhatia, R.: A critical review of sentiment analysis. Int. J. Comput. Appl. 149 (10), 37–40 (2016)
Pak, A., Paroubek, P.: Twitter as a corpus for sentiment analysis and opinion mining. In: LREc, vol. 10, no. 2010, pp. 1320–1326 (2010)
Rahate, R.S., Emmanuel, M.: Feature selection for sentiment analysis by using svm. Int. J. Comput. Appl. 84 (5), 24–32 (2013)
Hofmann, T.: Probabilistic latent semantic analysis. In: Proceedings of the Fifteenth Conference on Uncertainty in Artificial Intelligence, pp. 289–296 (1999)
Medagoda, N., Shanmuganathan, S., Whalley, J.: A comparative analysis of opinion mining and sentiment classification in non-English languages. In: 2013 International Conference on Advances in ICT for Emerging Regions (ICTer), pp. 144–148 (2013)
Mukherjee, S., Bhattacharyya, P.: Sentiment analysis in Twitter with lightweight discourse analysis. In: Proceedings of COLING 2012, pp. 1847–1864 (2012)
Helbig, H., Hartrumpf, S.: Word class functions for syntactic-semantic analysis. In: Proceedings of the 2nd International Conference on Recent Advances in Natural Language Processing (RANLP 1997), pp. 312–317 (1997)
Download references
Author information
Authors and affiliations.
Faculty of Engineering and IT, The British University in Dubai, Dubai, UAE
Said A. Salloum, Rehan Khan & Khaled Shaalan
Research Institute of Sciences and Engineering, University of Sharjah, Sharjah, UAE
Said A. Salloum
You can also search for this author in PubMed Google Scholar
Corresponding author
Correspondence to Said A. Salloum .
Editor information
Editors and affiliations.
Information Technology Department, Cairo University, Faculty of Computers and Information, Giza, Egypt
Aboul-Ella Hassanien
Faculty of Computers and Information, Benha University, Banha, Egypt
Ahmad Taher Azar
Faculty of Computers and Information, Suez Canal University, Ismailia, Egypt
Tarek Gaber
Departamento de Ciencias Computacionales, Universidad de Guadalajara, CUCEI, Guadajalara, Jalisco, Mexico
Diego Oliva
Faculty of Computer and Information Sciences, Ain Shams University, Cairo, Egypt
Fahmy M. Tolba
Rights and permissions
Reprints and permissions
Copyright information
© 2020 Springer Nature Switzerland AG
About this paper
Cite this paper.
Salloum, S.A., Khan, R., Shaalan, K. (2020). A Survey of Semantic Analysis Approaches. In: Hassanien, AE., Azar, A., Gaber, T., Oliva, D., Tolba, F. (eds) Proceedings of the International Conference on Artificial Intelligence and Computer Vision (AICV2020). AICV 2020. Advances in Intelligent Systems and Computing, vol 1153. Springer, Cham. https://doi.org/10.1007/978-3-030-44289-7_6
Download citation
DOI : https://doi.org/10.1007/978-3-030-44289-7_6
Published : 24 March 2020
Publisher Name : Springer, Cham
Print ISBN : 978-3-030-44288-0
Online ISBN : 978-3-030-44289-7
eBook Packages : Intelligent Technologies and Robotics Intelligent Technologies and Robotics (R0)
Share this paper
Anyone you share the following link with will be able to read this content:
Sorry, a shareable link is not currently available for this article.
Provided by the Springer Nature SharedIt content-sharing initiative
- Publish with us
Policies and ethics
- Find a journal
- Track your research
Linguistics
- Finding Articles
- General Reference Materials
- Primary Materials and Data
- Applied Linguistics
- Computational Linguistics
- Corpus-based Linguistics
- Neurolinguistics
- Philosophy of Language
- Sociolinguistics and Linguistic Anthropology
- Handbooks & Atlases
About this page
There are specialized reference resources in all branches of linguistics. Most of these resources can be found either electronically or in the 9th floor reference area.
Specialized titles
NOTE: See also the online volumes of this title: Volume 1, Volume 2, Volume 3, Volume 4
NOTE: See also the online volumes of this title: Volume 1 , Volume 2 , Volume 3 , Volume 4 , Volume 5 , Volume 6 , Volume 7 , Volume 8 , Volume 9 , Volume 10 , Volume 11 , Volume 12 , Volume 13 , Volume 14 , Volume 15 , Volume 16 , Volume 17 , Volume 18
- << Previous: Pragmatics
- Next: Sociolinguistics and Linguistic Anthropology >>
- Last Updated: Aug 30, 2024 11:53 AM
- URL: https://guides.nyu.edu/linguistics
Semantics and Pragmatics
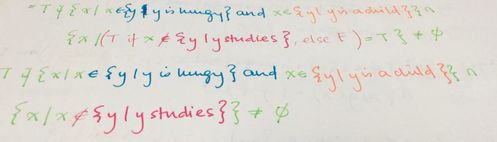
Semantics and pragmatics research at Stanford aims to develop theoretical models that appreciate and explain the complexity of meaning witnessed in language use.
The Stanford semantics and pragmatics community encompasses a broad range of interests including:
- Lexical semantics
- Formal semantics and pragmatics, and their interfaces with syntax
- Psycholinguistics
- Numerous sub-areas of psychology, philosophy, and computer science
We share the goal of grounding theories of meaning in diverse research methodologies, including:
- Formal linguistic analysis
- Psycholinguistic experimentation
- Computational modeling
- Corpus studies
- Fieldwork on less widely studied languages
We offer courses ranging from introductory courses at the undergraduate and graduate levels to seminars on cutting edge topics and workshops focused on developing key research skills.
Recent seminar topics have included:
- Temporal interpretation
- Experimental studies of quantification
- Definiteness
- Conditionals
Faculty and students participate in a wide range of research projects, many of them collaborative. Many of them also span subfields of linguistics or reach out to neighboring disciplines. There are usually informal reading or working groups that reflect the community's ever evolving research interests, as well as occasional larger gatherings, such as Construction of Meaning Workshops and an annual SemFest .
Ongoing Labs & Projects
- Computational Semantics in the Stanford NLP Group
- CSLI Computational Semantics Lab
- CSLI Language and Natural Reasoning Group
- CSLI Pragmatic Enrichment and Contextual Inference Lab
- CSLI Psychosemantics Lab
- The interActive Language Processing (ALPS) Lab
People in this subfield
Related news, look who’s talking: coppock and jasbi, look who’s talking: krejci, look who’s talking: stanford linguists at lsrl54, welcome to our incoming ph.d. students, qp fest is next friday, upcoming events.
A new look of the Semantic Web
Research areas.
Data Management
Data Mining and Modeling
Learn more about how we conduct our research
We maintain a portfolio of research projects, providing individuals and teams the freedom to emphasize specific types of work.
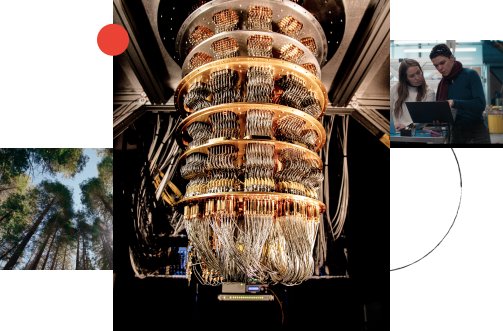

Topics in Semantics and Pragmatics
This course will provide a comprehensive overview of the empirical patterns, analytical challenges and broader theoretical issues surrounding a particular topic, such as information structure, presupposition, scalar implicature, binding, aspectual composition, nominal reference, and so forth.
- Open access
- Published: 29 June 2017
Text mining and semantics: a systematic mapping study
- Roberta Akemi Sinoara ORCID: orcid.org/0000-0001-8572-2747 1 ,
- João Antunes 1 &
- Solange Oliveira Rezende 1
Journal of the Brazilian Computer Society volume 23 , Article number: 9 ( 2017 ) Cite this article
16k Accesses
31 Citations
1 Altmetric
Metrics details
As text semantics has an important role in text meaning, the term semantics has been seen in a vast sort of text mining studies. However, there is a lack of studies that integrate the different research branches and summarize the developed works. This paper reports a systematic mapping about semantics-concerned text mining studies. This systematic mapping study followed a well-defined protocol. Its results were based on 1693 studies, selected among 3984 studies identified in five digital libraries. The produced mapping gives a general summary of the subject, points some areas that lacks the development of primary or secondary studies, and can be a guide for researchers working with semantics-concerned text mining. It demonstrates that, although several studies have been developed, the processing of semantic aspects in text mining remains an open research problem.
Introduction
Text mining techniques have become essential for supporting knowledge discovery as the volume and variety of digital text documents have increased, either in social networks and the Web or inside organizations. Text sources, as well as text mining applications, are varied. Although there is not a consensual definition established among the different research communities [ 1 ], text mining can be seen as a set of methods used to analyze unstructured data and discover patterns that were unknown beforehand [ 2 ].
A general text mining process can be seen as a five-step process, as illustrated in Fig. 1 . The process starts with the specification of its objectives in the problem identification step. The text mining analyst, preferably working along with a domain expert, must delimit the text mining application scope, including the text collection that will be mined and how the result will be used. The specifications stated in the problem identification step will guide the next steps of the text mining process, which can be executed in cycles of data preparation (pre-processing step), knowledge discovery (pattern extraction step), and knowledge evaluation (post-processing step).
A general text mining process
The pre-processing step is about preparing data for pattern extraction. In this step, raw text is transformed into some data representation format that can be used as input for the knowledge extraction algorithms. The activities performed in the pre-processing step are crucial for the success of the whole text mining process. The data representation must preserve the patterns hidden in the documents in a way that they can be discovered in the next step. In the pattern extraction step, the analyst applies a suitable algorithm to extract the hidden patterns. The algorithm is chosen based on the data available and the type of pattern that is expected. The extracted knowledge is evaluated in the post-processing step. If this knowledge meets the process objectives, it can be put available to the users, starting the final step of the process, the knowledge usage. Otherwise, another cycle must be performed, making changes in the data preparation activities and/or in pattern extraction parameters. If any changes in the stated objectives or selected text collection must be made, the text mining process should be restarted at the problem identification step.
Text data are not naturally in a format that is suitable for the pattern extraction, which brings additional challenges to an automatic knowledge discovery process. The meaning of natural language texts basically depends on lexical, syntactic, and semantic levels of linguistic knowledge. Each level is more complex and requires a more sophisticated processing than the previous level. This is a common trade-off when dealing with natural language processing: expressiveness versus processing cost. Thus, lexical and syntactic components have been more broadly explored in text mining than the semantic component [ 2 ]. Recently, text mining researchers have become more interested in text semantics, looking for improvements in the text mining results. The reason for this increasing interest can be assigned both to the progress of the computing capacity, which is constantly reducing the processing time, and to developments in the natural language processing field, which allow a deeper processing of raw texts.
In order to compare the expressiveness of each level of text interpretation (lexical, syntactic, and semantic), consider two simple sentences:
Company A acquired Company B.
Company B acquired Company A.
Sentences 1 and 2 have opposite meanings, but they have the same terms (“Company”, “A”, “B”, “acquired”). Thus, if we analyze these sentences only in the lexical level, it is not possible to differentiate them. However, considering the sentence syntax, we can see that they are opposite. They have the same verb, and the subject of one sentence is the object of the other sentence and vice versa. If we analyze a little deeper, now considering the sentence semantics, we find that in sentence 1, “Company A” has the semantic role of agent regarding the verb “acquire” and “Company B” has the semantic role of theme . The same can be said to a third sentence:
Company B was acquired by Company A.
Despite the fact that syntactically sentences 1 and 3 have opposite subjects and objects, they have the same semantic roles. Thus, at the semantic level, they have the same meaning. If we go deeper and consider semantic relations among words (as the synonymy, for example), we can find that sentence 4 also expresses the same event:
Company A purchased Company B.
Besides, going even deeper in the interpretation of the sentences, we can understand their meaning—they are related to some takeover—and we can, for example, infer that there will be some impacts on the business environment.
Traditionally, text mining techniques are based on both a bag-of-words representation and application of data mining techniques. In this approach, only the lexical component of the texts are considered. In order to get a more complete analysis of text collections and get better text mining results, several researchers directed their attention to text semantics.
Text semantics can be considered in the three main steps of text mining process: pre-processing, pattern extraction and post-processing. In the pre-processing step, data representation can be based on some sort of semantic aspect of the text collection. In the pattern extraction, semantic information can be used to guide the model generation or to refine it. In the post-processing step, the extracted patterns can be evaluated based on semantic aspects. Either way, text mining based on text semantics can go further than text mining based only on lexicon or syntax. A proper treatment of text semantics can lead to more appropriate results for certain applications [ 2 ]. For example, semantic information has an important impact on document content and can be crucial to differentiate documents which, despite the use of the same vocabulary, present different ideas about the same subject.
The term semantics has been seen in a vast sort of text mining studies. However, there is a lack of studies that integrate the different branches of research performed to incorporate text semantics in the text mining process. Secondary studies, such as surveys and reviews, can integrate and organize the studies that were already developed and guide future works.
Thus, this paper reports a systematic mapping study to overview the development of semantics-concerned studies and fill a literature review gap in this broad research field through a well-defined review process. Semantics can be related to a vast number of subjects, and most of them are studied in the natural language processing field. As examples of semantics-related subjects, we can mention representation of meaning, semantic parsing and interpretation, word sense disambiguation, and coreference resolution. Nevertheless, the focus of this paper is not on semantics but on semantics-concerned text mining studies. As the term semantics appears in text mining studies in different contexts, this systematic mapping aims to present a general overview and point some areas that lack the development of primary studies and those areas that secondary studies would be of great help. This paper aims to point some directions to the reader who is interested in semantics-concerned text mining researches.
As it covers a wide research field, this systematic mapping study started with a space of 3984 studies, identified in five digital libraries. Due to time and resource limitations, except for survey papers, the mapping was done primarily through information found in paper abstracts. Therefore, our intention is to present an overview of semantics-concerned text mining, presenting a map of studies that has been developed by the research community, and not to present deep details of the studies. The papers were analyzed in relation to their application domains, performed tasks, applied methods and resources, and level of user’s interaction. The contribution of this paper is threefold: (i) it presents an overview of semantics-concerned text mining studies from a text mining viewpoint, organizing the studies according to seven aspects (application domains, languages, external knowledge sources, tasks, methods and algorithms, representation models, and user’s interaction); (ii) it quantifies and confirms some previous feelings that we had about our study subject; and (iii) it provides a starting point for those, researchers or practitioners, who are initiating works on semantics-concerned text mining.
The remainder of this paper is organized as follows. The “ Method applied for systematic mapping ” section presents an overview of systematic mapping method, since this is the type of literature review selected to develop this study and it is not widespread in the text mining community. In this section, we also present the protocol applied to conduct the systematic mapping study, including the research questions that guided this study and how it was conducted. The results of the systematic mapping, as well as identified future trends, are presented in the “ Results and discussion ” section. The “ Conclusion ” section concludes this work.
Method applied for systematic mapping
The review reported in this paper is the result of a systematic mapping study, which is a particular type of systematic literature review [ 3 , 4 ]. Systematic literature review is a formal literature review adopted to identify, evaluate, and synthesize evidences of empirical results in order to answer a research question. It is extensively applied in medicine, as part of the evidence-based medicine [ 5 ]. This type of literature review is not as disseminated in the computer science field as it is in the medicine and health care fields 1 , although computer science researches can also take advantage of this type of review. We can find important reports on the use of systematic reviews specially in the software engineering community [ 3 , 4 , 6 , 7 ]. Other sparse initiatives can also be found in other computer science areas, as cloud-based environments [ 8 ], image pattern recognition [ 9 ], biometric authentication [ 10 ], recommender systems [ 11 ], and opinion mining [ 12 ].
A systematic review is performed in order to answer a research question and must follow a defined protocol. The protocol is developed when planning the systematic review, and it is mainly composed by the research questions, the strategies and criteria for searching for primary studies, study selection, and data extraction. The protocol is a documentation of the review process and must have all the information needed to perform the literature review in a systematic way. The analysis of selected studies, which is performed in the data extraction phase, will provide the answers to the research questions that motivated the literature review. Kitchenham and Charters [ 3 ] present a very useful guideline for planning and conducting systematic literature reviews. As systematic reviews follow a formal, well-defined, and documented protocol, they tend to be less biased and more reproducible than a regular literature review.
When the field of interest is broad and the objective is to have an overview of what is being developed in the research field, it is recommended to apply a particular type of systematic review named systematic mapping study [ 3 , 4 ]. Systematic mapping studies follow an well-defined protocol as in any systematic review. The main differences between a traditional systematic review and a systematic mapping are their breadth and depth. While a systematic review deeply analyzes a low number of primary studies, in a systematic mapping a wider number of studies are analyzed, but less detailed. Thus, the search terms of a systematic mapping are broader and the results are usually presented through graphs. Systematic mapping studies can be used to get a mapping of the publications about some subject or field and identify areas that require the development of more primary studies and areas in which a narrower systematic literature review would be of great help to the research community.
This paper reports a systematic mapping study conducted to get a general overview of how text semantics is being treated in text mining studies. It fills a literature review gap in this broad research field through a well-defined review process. As a systematic mapping, our study follows the principles of a systematic mapping/review. However, as our goal was to develop a general mapping of a broad field, our study differs from the procedure suggested by Kitchenham and Charters [ 3 ] in two ways. Firstly, Kitchenham and Charters [ 3 ] state that the systematic review should be performed by two or more researchers. Although our mapping study was planned by two researchers, the study selection and the information extraction phases were conducted by only one due to the resource constraints. In this process, the other researchers reviewed the execution of each systematic mapping phase and their results. Secondly, systematic reviews usually are done based on primary studies only, nevertheless we have also accepted secondary studies (reviews or surveys) as we want an overview of all publications related to the theme.
In the following subsections, we describe our systematic mapping protocol and how this study was conducted.
Systematic mapping planning
The first step of a systematic review or systematic mapping study is its planning. The researchers conducting the study must define its protocol, i.e., its research questions and the strategies for identification, selection of studies, and information extraction, as well as how the study results will be reported. The main parts of the protocol that guided the systematic mapping study reported in this paper are presented in the following.
Research question: the main research question that guided this study was “How is semantics considered in text mining studies?” The main question was detailed in seven secondary questions, all of them related to text mining studies that consider text semantics in some way:
What are the application domains that focus on text semantics?
What are the natural languages being considered when working with text semantics?
Which external sources are frequently used in text mining studies when text semantics is considered?
In what text mining tasks is the text semantics most considered?
What methods and algorithms are commonly used?
How can texts be represented?
Do users or domain experts take part in the text mining process?
Study identification: the study identification was performed through searches for studies conducted in five digital libraries: ACM Digital Library, IEEE Xplore, Science Direct, Web of Science, and Scopus. The following general search expression was applied in both Title and Keywords fields, when allowed by the digital library search engine: semantic* AND text* AND (mining OR representation OR clustering OR classification OR association rules) .
Study selection: every study returned in the search phase went to the selection phase. Studies were selected based on title, abstract, and paper information (as number of pages, for example). Through this analysis, duplicated studies (most of them were studies found in more than one database) were identified. Besides, studies which match at least one of the following exclusion criteria were rejected: (i) one page papers, posters, presentations, abstracts, and editorials; (ii) papers hosted in services with restricted access and not accessible; (iii) papers written in languages different from English or Portuguese; and (iv) studies that do not deal with text mining and text semantics.
Information extraction: the information extraction phase was performed with papers accepted in the selection phase (papers that were not identified as duplicated or rejected). The abstracts were read in order to extract the information presented in Fig. 2 .
Information extraction form
As any literature review, this study has some bias. The advantage of a systematic literature review is that the protocol clearly specifies its bias, since the review process is well-defined. There are bias related to (i) study identification, i.e., only papers matching the search expression and returned by the searched digital libraries were selected; (ii) selection criteria, i.e., papers that matches the exclusion criteria were rejected; and (iii) information extraction, i.e., the information were mainly extracted considering only title and abstracts. It is not feasible to conduct a literature review free of bias. However, it is possible to conduct it in a controlled and well-defined way through a systematic process.
Systematic mapping conduction
The conduction of this systematic mapping followed the protocol presented in the last subsection and is illustrated in Fig. 3 . The selection and the information extraction phases were performed with support of the Start tool [ 13 ].
Systematic mapping conduction phases. The numbers in the shaded areas indicate the quantity of studies involved
This paper reports the results obtained after the execution of two cycles of the systematic mapping phases. The first cycle was executed based on searches performed in January 2014. The second cycle was an update of the first cycle, with searches performed in February 2016 2 . A total of 3984 papers were found using the search expression in the five digital libraries. In the selection phase, 725 duplicated studies were identified and 1566 papers were rejected according to the exclusion criteria, mainly based on their title and abstract. Most of the rejected papers match the last exclusion criteria ( Studies that do not deal with text mining and text semantics ). Among them, we can find studies that deal with multimedia data (images, videos, or audio) and with construction, description, or annotation of corpus.
After the selection phase, 1693 studies were accepted for the information extraction phase. In this phase, information about each study was extracted mainly based on the abstracts, although some information was extracted from the full text. The results of the accepted paper mapping are presented in the next section.
Results and discussion
The mapping reported in this paper was conducted with the general goal of providing an overview of the researches developed by the text mining community and that are concerned about text semantics. This mapping is based on 1693 studies selected as described in the previous section. The distribution of these studies by publication year is presented in Fig. 4 . We can note that text semantics has been addressed more frequently in the last years, when a higher number of text mining studies showed some interest in text semantics. The peak was in 2011, with 223 identified studies. The lower number of studies in the year 2016 can be assigned to the fact that the last searches were conducted in February 2016.
Distribution of the 1693 accepted studies by publication year. Searches for studies identification were executed in January 2014 and February 2016
The results of the systematic mapping study is presented in the following subsections. We start our report presenting, in the “ Surveys ” section, a discussion about the eighteen secondary studies (surveys and reviews) that were identified in the systematic mapping. Then, each following section from “ Application domains ” to “ User’s interaction ” is related to a secondary research question that guided our study, i.e., application domains, languages, external knowledge sources, text mining tasks, methods and algorithms, representation model, and user’s interaction. In the “ Systematic mapping summary and future trends ” section, we present a consolidation of our results and point some gaps of both primary and secondary studies.
Some studies accepted in this systematic mapping are cited along the presentation of our mapping. We do not present the reference of every accepted paper in order to present a clear reporting of the results.
In this systematic mapping, we identified 18 survey papers associated to the theme text mining and semantics [ 14 – 31 ]. Each paper exploits some particularity of this broad theme. In the following, we present a short overview of these papers, which is based on the full text of the papers.
Grobelnik [ 14 ] presents, briefly but in a very clear form, an interesting discussion of text processing in his three-page paper. The author organizes the field in three main dimensions, which can be used to classify text processing approaches: representation, technique, and task. The task dimension is about the kind of problems, we solve through the text processing. Document search, clustering, classification, summarization, trend detection, and monitoring are examples of tasks. Considering how text representations are manipulated (technique dimension), we have the methods and algorithms that can be used, including machine learning algorithms, statistical analysis, part-of-speech tagging, semantic annotation, and semantic disambiguation. In the representation dimension, we can find different options for text representation, such as words, phrases, bag-of-words, part-of-speech, subject-predicate-object triples and semantically annotated triples.
Grobelnik [ 14 ] also presents the levels of text representations, that differ from each other by the complexity of processing and expressiveness. The most simple level is the lexical level, which includes the common bag-of-words and n-grams representations. The next level is the syntactic level, that includes representations based on word co-location or part-of-speech tags. The most complete representation level is the semantic level and includes the representations based on word relationships, as the ontologies. Several different research fields deal with text, such as text mining, computational linguistics, machine learning, information retrieval, semantic web and crowdsourcing. Grobelnik [ 14 ] states the importance of an integration of these research areas in order to reach a complete solution to the problem of text understanding.
Stavrianou et al. [ 15 ] present a survey of semantic issues of text mining, which are originated from natural language particularities. This is a good survey focused on a linguistic point of view, rather than focusing only on statistics. The authors discuss a series of questions concerning natural language issues that should be considered when applying the text mining process. Most of the questions are related to text pre-processing and the authors present the impacts of performing or not some pre-processing activities, such as stopwords removal, stemming, word sense disambiguation, and tagging. The authors also discuss some existing text representation approaches in terms of features, representation model, and application task. The set of different approaches to measure the similarity between documents is also presented, categorizing the similarity measures by type (statistical or semantic) and by unit (words, phrases, vectors, or hierarchies).
Stavrianou et al. [ 15 ] also present the relation between ontologies and text mining. Ontologies can be used as background knowledge in a text mining process, and the text mining techniques can be used to generate and update ontologies. The authors conclude the survey stating that text mining is an open research area and that the objectives of the text mining process must be clarified before starting the data analysis, since the approaches must be chosen according to the requirements of the task being performed.
Methods that deal with latent semantics are reviewed in the study of Daud et al. [ 16 ]. The authors present a chronological analysis from 1999 to 2009 of directed probabilistic topic models, such as probabilistic latent semantic analysis, latent Dirichlet allocation, and their extensions. The models are classified according to their main functionality. They describe their advantages, disadvantages, and applications.
Wimalasuriya and Dou [ 17 ], Bharathi and Venkatesan [ 18 ], and Reshadat and Feizi-Derakhshi [ 19 ] consider the use of external knowledge sources (e.g., ontology or thesaurus) in the text mining process, each one dealing with a specific task. Wimalasuriya and Dou [ 17 ] present a detailed literature review of ontology-based information extraction. The authors define the recent information extraction subfield, named ontology-based information extraction (OBIE), identifying key characteristics of the OBIE systems that differentiate them from general information extraction systems. Besides, they identify a common architecture of the OBIE systems and classify existing systems along with different dimensions, as information extraction method applied, whether it constructs and updates the ontology, components of the ontology, and type of documents the system deals with. Bharathi and Venkatesan [ 18 ] present a brief description of several studies that use external knowledge sources as background knowledge for document clustering. Reshadat and Feizi-Derakhshi [ 19 ] present several semantic similarity measures based on external knowledge sources (specially WordNet and MeSH) and a review of comparison results from previous studies.
Schiessl and Bräscher [ 20 ] and Cimiano et al. [ 21 ] review the automatic construction of ontologies. Schiessl and Bräscher [ 20 ], the only identified review written in Portuguese, formally define the term ontology and discuss the automatic building of ontologies from texts. The authors state that automatic ontology building from texts is the way to the timely production of ontologies for current applications and that many questions are still open in this field. Also, in the theme of automatic building of ontologies from texts, Cimiano et al. [ 21 ] argue that automatically learned ontologies might not meet the demands of many possible applications, although they can already benefit several text mining tasks. The authors divide the ontology learning problem into seven tasks and discuss their developments. They state that ontology population task seems to be easier than learning ontology schema tasks.
Jovanovic et al. [ 22 ] discuss the task of semantic tagging in their paper directed at IT practitioners. Semantic tagging can be seen as an expansion of named entity recognition task, in which the entities are identified, disambiguated, and linked to a real-world entity, normally using a ontology or knowledge base. The authors compare 12 semantic tagging tools and present some characteristics that should be considered when choosing such type of tools.
Specifically for the task of irony detection, Wallace [ 23 ] presents both philosophical formalisms and machine learning approaches. The author argues that a model of the speaker is necessary to improve current machine learning methods and enable their application in a general problem, independently of domain. He discusses the gaps of current methods and proposes a pragmatic context model for irony detection.
The application of text mining methods in information extraction of biomedical literature is reviewed by Winnenburg et al. [ 24 ]. The paper describes the state-of-the-art text mining approaches for supporting manual text annotation, such as ontology learning, named entity and concept identification. They also describe and compare biomedical search engines, in the context of information retrieval, literature retrieval, result processing, knowledge retrieval, semantic processing, and integration of external tools. The authors argue that search engines must also be able to find results that are indirectly related to the user’s keywords, considering the semantics and relationships between possible search results. They point that a good source for synonyms is WordNet.
Leser and Hakenberg [ 25 ] presents a survey of biomedical named entity recognition. The authors present the difficulties of both identifying entities (like genes, proteins, and diseases) and evaluating named entity recognition systems. They describe some annotated corpora and named entity recognition tools and state that the lack of corpora is an important bottleneck in the field.
Dagan et al. [ 26 ] introduce a special issue of the Journal of Natural Language Engineering on textual entailment recognition, which is a natural language task that aims to identify if a piece of text can be inferred from another. The authors present an overview of relevant aspects in textual entailment, discussing four PASCAL Recognising Textual Entailment (RTE) Challenges. They declared that the systems submitted to those challenges use cross-pair similarity measures, machine learning, and logical inference. The authors also describe tools, resources, and approaches commonly used in textual entailment tasks and conclude with the perspective that in the future, the constructed entailment “engines” will be used as a basic module by the text-understanding applications.
Irfan et al. [ 27 ] present a survey on the application of text mining methods in social network data. They present an overview of pre-processing, classification and clustering techniques to discover patterns from social networking sites. They point out that the application of text mining techniques can reveal patterns related to people’s interaction behaviors. The authors present two basic pre-processing activities: feature extraction and feature selection. The authors also review classification and clustering approaches. They present different machine learning algorithms and discuss the importance of ontology usage to introduce explicit concepts, descriptions, and the semantic relationships among concepts. Irfan et al. [ 27 ] identify the main challenges related to the manipulation of social network texts (such as large data, data with impurities, dynamic data, emotions interpretations, privacy, and data confidence) and to text mining infrastructure (such as usage of cloud computing and improvement of the usability of text mining methods).
In the context of semantic web, Sheth et al. [ 28 ] define three types of semantics: implicit semantics, formal semantics, and powerful (or soft) semantics. Implicit semantics are those implicitly present in data patterns and is not explicitly represented in any machine processable syntax. Machine learning methods exploit this type of semantics. Formal semantics are those represented in some well-formed syntactic form and are machine-processable. The powerful semantics are the sort of semantics that allow uncertainty (that is, the representation of degree of membership and degree of certainty) and, therefore, allowing abductive or inductive reasoning. The authors also correlates the types of semantics with some core capabilities required by a practical semantic web application. The authors conclude their review asserting the importance of focusing research efforts in representation mechanisms for powerful semantics in order to move towards the development of semantic applications.
The formal semantics defined by Sheth et al. [ 28 ] is commonly represented by description logics, a formalism for knowledge representation. The application of description logics in natural language processing is the theme of the brief review presented by Cheng et al. [ 29 ].
The broad field of computational linguistics is presented by Martinez and Martinez [ 30 ]. Considering areas of computational linguistics that can be interesting to statisticians, the authors describe three main aspects of computational linguistics: formal language, information retrieval, and machine learning. The authors present common models for knowledge representation, addressing their statistical characteristics and providing an overview of information retrieval and machine learning methods related to computational linguistics. They describe some of the major statistical contributions to the areas of machine learning and computational linguistics, from the point of view of classification and clustering algorithms. Martinez and Martinez [ 30 ] emphasize that machine translation, part-of-speech tagging, word sense disambiguation, and text summarization are some of the identified applications that statisticians can contribute.
Bos [ 31 ] presents an extensive survey of computational semantics, a research area focused on computationally understanding human language in written or spoken form. He discusses how to represent semantics in order to capture the meaning of human language, how to construct these representations from natural language expressions, and how to draw inferences from the semantic representations. The author also discusses the generation of background knowledge, which can support reasoning tasks. Bos [ 31 ] indicates machine learning, knowledge resources, and scaling inference as topics that can have a big impact on computational semantics in the future.
As presented in this section, the reviewed secondary studies exploit some specific issues of semantics-concerned text mining researches. In contrast to them, this paper reviews a broader range of text mining studies that deal with semantic aspects. To the best of our knowledge, this is the first report of a mapping of this field. We present the results of our systematic mapping study in the following sections, organized in seven dimensions of the text mining studies derived from our secondary research questions: application domains, languages, external knowledge usage, tasks, methods and algorithms, representation model, and user’s interaction.
Application domains
Research question:
Figure 5 presents the domains where text semantics is most present in text mining applications. Health care and life sciences is the domain that stands out when talking about text semantics in text mining applications. This fact is not unexpected, since life sciences have a long time concern about standardization of vocabularies and taxonomies. The building of taxonomies and ontologies is such a common practice in health care and life sciences that World Wide Web Consortium (W3C) has an interest group specific for developing, evaluating, and supporting semantic web technologies for this field [ 32 ]. Among the most common problems treated through the use of text mining in the health care and life science is the information retrieval from publications of the field. The search engine PubMed [ 33 ] and the MEDLINE database are the main text sources among these studies. There are also studies related to the extraction of events, genes, proteins and their associations [ 34 – 36 ], detection of adverse drug reaction [ 37 ], and the extraction of cause-effect and disease-treatment relations [ 38 – 40 ].
Application domains identified in the literature mapping accepted studies
The second most frequent identified application domain is the mining of web texts, comprising web pages, blogs, reviews, web forums, social medias, and email filtering [ 41 – 46 ]. The high interest in getting some knowledge from web texts can be justified by the large amount and diversity of text available and by the difficulty found in manual analysis. Nowadays, any person can create content in the web, either to share his/her opinion about some product or service or to report something that is taking place in his/her neighborhood. Companies, organizations, and researchers are aware of this fact, so they are increasingly interested in using this information in their favor. Some competitive advantages that business can gain from the analysis of social media texts are presented in [ 47 – 49 ]. The authors developed case studies demonstrating how text mining can be applied in social media intelligence. From our systematic mapping data, we found that Twitter is the most popular source of web texts and its posts are commonly used for sentiment analysis or event extraction.
Besides the top 2 application domains, other domains that show up in our mapping refers to the mining of specific types of texts. We found research studies in mining news, scientific papers corpora, patents, and texts with economic and financial content.
Whether using machine learning or statistical techniques, the text mining approaches are usually language independent. However, specially in the natural language processing field, annotated corpora is often required to train models in order to resolve a certain task for each specific language (semantic role labeling problem is an example). Besides, linguistic resources as semantic networks or lexical databases, which are language-specific, can be used to enrich textual data. Most of the resources available are English resources. Thus, the low number of annotated data or linguistic resources can be a bottleneck when working with another language. There are important initiatives to the development of researches for other languages, as an example, we have the ACM Transactions on Asian and Low-Resource Language Information Processing [ 50 ], an ACM journal specific for that subject.
In this study, we identified the languages that were mentioned in paper abstracts. The collected data are summarized in Fig. 6 . We must note that English can be seen as a standard language in scientific publications; thus, papers whose results were tested only in English datasets may not mention the language, as examples, we can cite [ 51 – 56 ]. Besides, we can find some studies that do not use any linguistic resource and thus are language independent, as in [ 57 – 61 ]. These facts can justify that English was mentioned in only 45.0% of the considered studies.
Languages identified in the literature mapping accepted studies
Chinese is the second most mentioned language (26.4% of the studies reference the Chinese language). Wu et al. [ 62 ] point two differences between English and Chinese: in Chinese, there are no white spaces between words in a sentence and there are a higher number of frequent words (the number of frequent words in Chinese is more than twice the number of English frequent words). These characteristics motivate the development of methods and experimental evaluations specifically for Chinese.
This mapping shows that there is a lack of studies considering languages other than English or Chinese. The low number of studies considering other languages suggests that there is a need for construction or expansion of language-specific resources (as discussed in “ External knowledge sources ” section). These resources can be used for enrichment of texts and for the development of language specific methods, based on natural language processing.
External knowledge sources
Text mining initiatives can get some advantage by using external sources of knowledge. Thesauruses, taxonomies, ontologies, and semantic networks are knowledge sources that are commonly used by the text mining community. Semantic networks is a network whose nodes are concepts that are linked by semantic relations. The most popular example is the WordNet [ 63 ], an electronic lexical database developed at the Princeton University. Depending on its usage, WordNet can also be seen as a thesaurus or a dictionary [ 64 ].
There is not a complete definition for the terms thesaurus, taxonomy, and ontology that is unanimously accepted by all research areas. Weller [ 65 ] presents an interesting discussion about the term ontology , including its origin and proposed definitions. She concluded the discussion stating that: “Ontologies should unambiguously represent shared background knowledge that helps people within a community of interest to understand each other. And they should make computer-readable indexing of information possible on the Web” [ 65 ]. The same can be said about thesauruses and taxonomies. In a general way, thesauruses, taxonomies, and ontologies are normally specialized in a specific domain and they usually differs from each other by their degree of expressiveness and complexity in their relational constructions [ 66 ]. Ontology would be the most expressive type of knowledge representation, having the most complex relations and formalized construction.
When looking at the external knowledge sources used in semantics-concerned text mining studies (Fig. 7 ), WordNet is the most used source. This lexical resource is cited by 29.9% of the studies that uses information beyond the text data. WordNet can be used to create or expand the current set of features for subsequent text classification or clustering. The use of features based on WordNet has been applied with and without good results [ 55 , 67 – 69 ]. Besides, WordNet can support the computation of semantic similarity [ 70 , 71 ] and the evaluation of the discovered knowledge [ 72 ].
External sources identified in the literature mapping accepted studies
The second most used source is Wikipedia [ 73 ], which covers a wide range of subjects and has the advantage of presenting the same concept in different languages. Wikipedia concepts, as well as their links and categories, are also useful for enriching text representation [ 74 – 77 ] or classifying documents [ 78 – 80 ]. Medelyan et al. [ 81 ] present the value of Wikipedia and discuss how the community of researchers are making use of it in natural language processing tasks (in special word sense disambiguation), information retrieval, information extraction, and ontology building.
The use of Wikipedia is followed by the use of the Chinese-English knowledge database HowNet [ 82 ]. Finding HowNet as one of the most used external knowledge source it is not surprising, since Chinese is one of the most cited languages in the studies selected in this mapping (see the “ Languages ” section). As well as WordNet, HowNet is usually used for feature expansion [ 83 – 85 ] and computing semantic similarity [ 86 – 88 ].
Web pages are also used as external sources [ 89 – 91 ]. Normally, web search results are used to measure similarity between terms. We also found some studies that use SentiWordNet [ 92 ], which is a lexical resource for sentiment analysis and opinion mining [ 93 , 94 ]. Among other external sources, we can find knowledge sources related to Medicine, like the UMLS Metathesaurus [ 95 – 98 ], MeSH thesaurus [ 99 – 102 ], and the Gene Ontology [ 103 – 105 ].
Text mining tasks
The distribution of text mining tasks identified in this literature mapping is presented in Fig. 8 . Classification and clustering are the most frequent tasks. Classification corresponds to the task of finding a model from examples with known classes (labeled instances) in order to predict the classes of new examples. On the other hand, clustering is the task of grouping examples (whose classes are unknown) based on their similarities. Classification was identified in 27.4% and clustering in 17.0% of the studies. As these are basic text mining tasks, they are often the basis of other more specific text mining tasks, such as sentiment analysis and automatic ontology building. Therefore, it was expected that classification and clustering would be the most frequently applied tasks.
Text mining tasks identified in the literature mapping accepted studies
Besides classification and clustering, we can note that semantic concern are present in tasks as information extraction [ 106 – 108 ], information retrieval [ 109 – 111 ], sentiment analysis [ 112 – 115 ], and automatic ontology building [ 116 , 117 ], as well as the pre-processing step itself [ 118 , 119 ].
Methods and algorithms
A word cloud 3 of methods and algorithms identified in this literature mapping is presented in Fig. 9 , in which the font size reflects the frequency of the methods and algorithms among the accepted papers. We can note that the most common approach deals with latent semantics through Latent Semantic Indexing (LSI) [ 2 , 120 ], a method that can be used for data dimension reduction and that is also known as latent semantic analysis. The Latent Semantic Index low-dimensional space is also called semantic space. In this semantic space, alternative forms expressing the same concept are projected to a common representation. It reduces the noise caused by synonymy and polysemy; thus, it latently deals with text semantics. Another technique in this direction that is commonly used for topic modeling is latent Dirichlet allocation (LDA) [ 121 ]. The topic model obtained by LDA has been used for representing text collections as in [ 58 , 122 , 123 ].
Word cloud of methods and algorithms identified in the literature mapping studies. To enable a better reading of the word cloud, the frequency of the methods and algorithms higher than one was rounded up to the nearest ten (for example, a method applied in 75 studies is represented in the word cloud in a word size corresponding to the frequency 80)
Beyond latent semantics, the use of concepts or topics found in the documents is also a common approach. The concept-based semantic exploitation is normally based on external knowledge sources (as discussed in the “ External knowledge sources ” section) [ 74 , 124 – 128 ]. As an example, explicit semantic analysis [ 129 ] rely on Wikipedia to represent the documents by a concept vector. In a similar way, Spanakis et al. [ 125 ] improved hierarchical clustering quality by using a text representation based on concepts and other Wikipedia features, such as links and categories.
The issue of text ambiguity has also been the focus of studies. Word sense disambiguation can contribute to a better document representation. It is normally based on external knowledge sources and can also be based on machine learning methods [ 36 , 130 – 133 ].
Other approaches include analysis of verbs in order to identify relations on textual data [ 134 – 138 ]. However, the proposed solutions are normally developed for a specific domain or are language dependent.
In Fig. 9 , we can observe the predominance of traditional machine learning algorithms, such as Support Vector Machines (SVM), Naive Bayes, K-means, and k-Nearest Neighbors (KNN), in addition to artificial neural networks and genetic algorithms. The application of natural language processing methods (NLP) is also frequent. Among these methods, we can find named entity recognition (NER) and semantic role labeling. It shows that there is a concern about developing richer text representations to be input for traditional machine learning algorithms, as we can see in the studies of [ 55 , 139 – 142 ].
Text representation models
The most popular text representation model is the vector space model. In this model, each document is represented by a vector whose dimensions correspond to features found in the corpus. When features are single words, the text representation is called bag-of-words. Despite the good results achieved with a bag-of-words, this representation, based on independent words, cannot express word relationships, text syntax, or semantics. Therefore, it is not a proper representation for all possible text mining applications.
The use of richer text representations is the focus of several studies [ 62 , 79 , 97 , 143 – 148 ]. Most of the studies concentrate on proposing more elaborated features to represent documents in the vector space model, including the use of topic model techniques, such as LSI and LDA, to obtain latent semantic features. Deep learning [ 149 ] is currently applied to represent independent terms through their associated concepts, in an attempt to narrow the relationships between the terms [ 150 , 151 ]. The use of distributed word representations (word embeddings) can be seen in several works of this area in tasks such as classification [ 88 , 152 , 153 ], summarization [ 154 ], and information retrieval [ 155 ].
Besides the vector space model, there are text representations based on networks (or graphs), which can make use of some text semantic features. Network-based representations, such as bipartite networks and co-occurrence networks, can represent relationships between terms or between documents, which is not possible through the vector space model [ 147 , 156 – 158 ].
In addition to the text representation model, text semantics can also be incorporated to text mining process through the use of external knowledge sources, like semantic networks and ontologies, as discussed in the “ External knowledge sources ” section.
User’s interaction
Text mining is a process to automatically discover knowledge from unstructured data. Nevertheless, it is also an interactive process, and there are some points where a user, normally a domain expert, can contribute to the process by providing his/her previous knowledge and interests. As an example, in the pre-processing step, the user can provide additional information to define a stoplist and support feature selection. In the pattern extraction step, user’s participation can be required when applying a semi-supervised approach. In the post-processing step, the user can evaluate the results according to the expected knowledge usage.
Despite the fact that the user would have an important role in a real application of text mining methods, there is not much investment on user’s interaction in text mining research studies. A probable reason is the difficulty inherent to an evaluation based on the user’s needs. In empirical research, researchers use to execute several experiments in order to evaluate proposed methods and algorithms, which would require the involvement of several users, therefore making the evaluation not feasible in practical ways.
Less than 1% of the studies that were accepted in the first mapping cycle presented information about requiring some sort of user’s interaction in their abstract. To better analyze this question, in the mapping update performed in 2016, the full text of the studies were also considered. Figure 10 presents types of user’s participation identified in the literature mapping studies. The most common user’s interactions are the revision or refinement of text mining results [ 159 – 161 ] and the development of a standard reference, also called as gold standard or ground truth, which is used to evaluate text mining results [ 162 – 165 ]. Besides that, users are also requested to manually annotate or provide a few labeled data [ 166 , 167 ] or generate of hand-crafted rules [ 168 , 169 ].
Types of user participation identified in the literature mapping accepted studies
Systematic mapping summary and future trends
How is semantics considered in text mining studies?
Semantics is an important component in natural language texts. Consequently, in order to improve text mining results, many text mining researches claim that their solutions treat or consider text semantics in some way. However, text mining is a wide research field and there is a lack of secondary studies that summarize and integrate the different approaches. How is semantics considered in text mining studies? Looking for the answer to this question, we conducted this systematic mapping based on 1693 studies, accepted among the 3984 studies identified in five digital libraries. In the previous subsections, we presented the mapping regarding to each secondary research question. In this subsection, we present a consolidation of our results and point some future trends of semantics-concerned text mining.
As previously stated, the objective of this systematic mapping is to provide a general overview of semantics-concerned text mining studies. The papers considered in this systematic mapping study, as well as the mapping results, are limited by the applied search expression and the research questions. It is not feasible to cover all published papers in this broad field. Therefore, the reader can miss in this systematic mapping report some previously known studies. It is not our objective to present a detailed survey of every specific topic, method, or text mining task. This systematic mapping is a starting point, and surveys with a narrower focus should be conducted for reviewing the literature of specific subjects, according to one’s interests.
The quantitative analysis of the scientific production by each text mining dimension (presented from the “ Application domains ” section to the “ User’s interaction ” section) confirmed some previous feelings that we had about our study subject and highlighted other interesting characteristics of the field. Text semantics is closely related to ontologies and other similar types of knowledge representation. We also know that health care and life sciences is traditionally concerned about standardization of their concepts and concepts relationships. Thus, as we already expected, health care and life sciences was the most cited application domain among the literature accepted studies. This application domain is followed by the Web domain, what can be explained by the constant growth, in both quantity and coverage, of Web content.
It was surprising to find the high presence of the Chinese language among the studies. Chinese language is the second most cited language, and the HowNet, a Chinese-English knowledge database, is the third most applied external source in semantics-concerned text mining studies. Looking at the languages addressed in the studies, we found that there is a lack of studies specific to languages other than English or Chinese. We also found an expressive use of WordNet as an external knowledge source, followed by Wikipedia, HowNet, Web pages, SentiWordNet, and other knowledge sources related to Medicine.
Text classification and text clustering, as basic text mining tasks, are frequently applied in semantics-concerned text mining researches. Among other more specific tasks, sentiment analysis is a recent research field that is almost as applied as information retrieval and information extraction, which are more consolidated research areas. SentiWordNet, a lexical resource for sentiment analysis and opinion mining, is already among the most used external knowledge sources.
The treatment of latent semantics, through the application of LSI, stands out when looking at methods and algorithms. Besides that, traditional text mining methods and algorithms, like SVM, KNN, and K-means, are frequently applied and researches tend to enhance the text representation by applying NLP methods or using external knowledge sources. Thus, text semantics can be incorporated to the text mining process mainly through two approaches: the construction of richer terms in the vector space representation model or the use of networks or graphs to represent semantic relations between terms or documents.
In real application of the text mining process, the participation of domain experts can be crucial to its success. However, the participation of users (domain experts) is seldom explored in scientific papers. The difficulty inherent to the evaluation of a method based on user’s interaction is a probable reason for the lack of studies considering this approach.
The mapping indicates that there is space for secondary studies in areas that has a high number of primary studies, such as studies of feature enrichment for a better text representation in the vector space model; use of classification methods; use of clustering methods; and the use of latent semantics in text mining. A detailed literature review, as the review of Wimalasuriya and Dou [ 17 ] (described in “ Surveys ” section), would be worthy for organization and summarization of these specific research subjects.
Considering the development of primary studies, we identified three main future trends: user’s interaction, non-English text processing, and graph-based representation. We expect an increase in the number of studies that have some level of user’s interaction to bring his/her needs and interests to the process. This is particularly valuable for the clustering task, because a considered good clustering solution can vary from user to user [ 170 ]. We also expect a raise of resources (linguistic resources and annotated corpora) for non-English languages. These resources are very important to the development of semantics-concerned text mining techniques. Higher availability of non-English resources will allow a higher number of studies dealing with these languages. Another future trend is the development and use of graph-based text representation. Nowadays, there are already important researches in this direction, and we expect that it will increase as graph-based representations are more expressive than traditional representations in the vector space model.
As an alternative summary of this systematic mapping, additional visualizations of both the selected studies and systematic mapping results can be found online at http://sites.labic.icmc.usp.br/pinda_sm . For this purpose, the prototype of the Pinda tool was adapted for hierarchical visualization of the textual data, using K-means algorithm to group the results. The tool allows the analysis of data (title + abstract of selected studies or information extracted from them) through multiple visualization techniques (Thumbnail, Snippets, Directories, Scatterplot, Treemap, and Sunburst), coordinating the user’s interactions for a better understanding of existing relationships. Figure 11 illustrates the Scatterplot visualization of studies accepted in this systematic mapping. Some of the possible visualizations of the systematic mapping results are presented in Fig. 12 .
Scatterplot visualization of accepted studies of the systematic mapping
Directories and Treemap visualizations of the systematic mapping results
Text semantics are frequently addressed in text mining studies, since it has an important influence in text meaning. However, there is a lack of secondary studies that consolidate these researches. This paper reported a systematic mapping study conducted to overview semantics-concerned text mining literature. The scope of this mapping is wide (3984 papers matched the search expression). Thus, due to limitations of time and resources, the mapping was mainly performed based on abstracts of papers. Nevertheless, we believe that our limitations do not have a crucial impact on the results, since our study has a broad coverage.
The main contributions of this work are (i) it presents a quantitative analysis of the research field; (ii) its conduction followed a well-defined literature review protocol; (iii) it discusses the area regarding seven important text mining dimensions: application domain, language, external knowledge source, text mining task, method and algorithm, representation model, and user’s interaction; and (iv) the produced mapping can give a general summary of the subject and can be of great help for researchers working with semantics and text mining. Thus, this work filled a gap in the literature as, to the best of our knowledge, this is the first general literature review of this wide subject.
Although several researches have been developed in the text mining field, the processing of text semantics remains an open research problem. The field lacks secondary studies in areas that has a high number of primary studies, such as feature enrichment for a better text representation in the vector space model. Another highlight is about a language-related issue. We found considerable differences in numbers of studies among different languages, since 71.4% of the identified studies deal with English and Chinese. Thus, there is a lack of studies dealing with texts written in other languages. When considering semantics-concerned text mining, we believe that this lack can be filled with the development of good knowledge bases and natural language processing methods specific for these languages. Besides, the analysis of the impact of languages in semantic-concerned text mining is also an interesting open research question. A comparison among semantic aspects of different languages and their impact on the results of text mining techniques would also be interesting.
1 A simple search for “systematic review” on the Scopus database in June 2016 returned, by subject area, 130,546 Health Sciences documents (125,254 of them for Medicine) and only 5,539 Physical Sciences (1328 of them for Computer Science). The coverage of Scopus publications are balanced between Health Sciences (32% of total Scopus publication) and Physical Sciences (29% of total Scopus publication).
2 It was not possible to perform the second cycle of searches in ACM Digital Library because of a change in the interface of this search engine. However, it must be notice that only eight studies that was found only in this database was accepted in the first cycle. All other studies was also retrieved by other search engines (specially Scopus, which retrieved more than 89% of accepted studies.)
3 Word cloud created with support of Wordle [ 171 ].
Miner G, Elder J, Hill T, Nisbet R, Delen D, Fast A (2012) Practical text mining and statistical analysis for non-structured text data applications. 1st edn. Academic Press, Boston.
Google Scholar
Aggarwal CC, Zhai C (eds)2012. Mining text data. Springer, Durham.
Kitchenham B, Charters S (2007) Guidelines for performing systematic literature reviews in software engineering. EBSE Technical Report EBSE-2007-01. Keele University and Durham University Joint Report, Durham, UK.
Petersen K, Feldt R, Mujtaba S, Mattsson M (2008) Systematic mapping studies in software engineering In: EASE 2008: Proceedings of the 12th International Conference on Evaluation and Assessment in Software Engineering. EASE’08, 68–77. British Computer Society, Swinton, UK.
Brereton P, Kitchenham BA, Budgen D, Turner M, Khalil M (2007) Lessons from applying the systematic literature review process within the software engineering domain. J Syst Softw80(4): 571–583.
Article Google Scholar
Kitchenham B, Pretorius R, Budgen D, Brereton OP, Turner M, Niazi M, et al (2010) Systematic literature reviews in software engineering—a tertiary study. Inf Softw Technol52(8): 792–805.
Felizardo KR, Nakagawa EY, MacDonell SG, Maldonado JC (2014) A visual analysis approach to update systematic reviews In: EASE’14: Proceedings of the 18th International Conference on Evaluation and Assessment in Software Engineering, 4:1–4:10. ACM, New York.
Moghaddam FA, Lago P, Grosso P (2015) Energy-efficient networking solutions in cloud-based environments: a systematic literature review. ACM Comput Surv47(4): 64:1–64:32.
Pedro RWD, Nunes FLS, Machado-Lima A (2013) Using grammars for pattern recognition in images: a systematic review. ACM Comput Surv46(2): 26:1–26:34.
Pisani PH, Lorena AC (2013) A systematic review on keystroke dynamics. J Braz Comput Soc19(4): 573–587.
Park DH, Kim HK, Choi IY, Kim JK (2012) A literature review and classification of recommender systems research. Expert Syst Appl39(11): 10059–10072.
Khan K, Baharudin BB, Khan A, et al (2009) Mining opinion from text documents: a survey In: DEST’09: Proceedings of the 3rd IEEE International Conference on Digital Ecosystems and Technologies, 217–222. IEEE.
Laboratory of Research on Software Engineering (LaPES) - StArt Tool. http://lapes.dc.ufscar.br/tools/start_tool . Accessed 8 June 2016.
Grobelnik M (2011) Many faces of text processing In: WIMS’11: Proceedings of the International Conference on Web Intelligence, Mining and Semantics, 5. ACM.
Stavrianou A, Andritsos P, Nicoloyannis N (2007) Overview and semantic issues of text mining. SIGMOD Rec36(3): 23–34.
Daud A, Li J, Zhou L, Muhammad F (2010) Knowledge discovery through directed probabilistic topic models: a survey. Front Comput Sci China4(2): 280–301.
Wimalasuriya DC, Dou D (2010) Ontology-based information extraction: an introduction and a survey of current approaches. J Inf Sci36(3): 306–323.
Bharathi G, Venkatesan D (2012) Study of ontology or thesaurus based document clustering and information retrieval. J Eng Appl Sci7(4): 342–347.
Reshadat V, Feizi-Derakhshi MR (2012) Studying of semantic similarity methods in ontology. Res J Appl Sci Eng Technol4(12): 1815–1821.
Schiessl M, Bräscher M (2012) Do texto às ontologias: uma perspectiva para a ciência da informação. Ciência da Informação40(2): 301–311.
Cimiano P, Völker J, Studer R (2006) Ontologies on demand?—a description of the state-of-the-art, applications, challenges and trends for ontology learning from text. Inf Wiss Prax57(6-7): 315–320.
Jovanovic J, Bagheri E, Cuzzola J, Gasevic D, Jeremic Z, Bashash R (2014) Automated semantic tagging of textual content. IT Prof16(6): 38–46.
Wallace BC (2015) Computational irony: a survey and new perspectives. Artif Intell Rev43(4): 467–483.
Winnenburg R, Wächter T, Plake C, Doms A, Schroeder M (2008) Facts from text: can text mining help to scale-up high-quality manual curation of gene products with ontologies?Brief Bioinform9(6): 466–478.
Leser U, Hakenberg J (2005) What makes a gene name? Named entity recognition in the biomedical literature. Brief Bioinform6(4): 357–369.
Dagan I, Dolan B, Magnini B, Roth D (2009) Recognizing textual entailment: rational, evaluation and approaches. Nat Lang Eng15(04): i–xvii.
Irfan R, King CK, Grages D, Ewen S, Khan SU, Madani SA, et al. (2015) A survey on text mining in social networks. Knowl Eng Rev30(02): 157–170.
Sheth A, Ramakrishnan C, Thomas C (2005) Semantics for the semantic web: the implicit, the formal and the powerful. Int J Semant Web Inf Syst1(1): 1–18.
Cheng XY, Cheng C, Zhu Q (2011) The applications of description logics in natural language processing. Adv Mater Res204: 381–386.
Martinez A, Martinez W (2015) At the interface of computational linguistics and statistics. Wiley Interdiscip Rev Comput Stat7(4): 258–274.
Article MathSciNet Google Scholar
Bos J (2011) A survey of computational semantics: representation, inference and knowledge in wide-coverage text understanding. Lang Linguist Compass5(6): 336–366.
W, 3C - Semantic Web Health Care and Life Sciences Interest Group. https://www.w3.org/blog/hcls/ . Accessed 8 June 2016.
National Center for Biotechnology Information - PubMed. http://www.ncbi.nlm.nih.gov/pubmed/ . Accessed 8 June 2016.
Miwa M, Thompson P, McNaught J, Kell DB, Ananiadou S (2012) Extracting semantically enriched events from biomedical literature. BMC Bioinforma13(1): 1–24.
Ravikumar KE, Liu H, Cohn JD, Wall ME, Verspoor K (2011) Pattern learning through distant supervision for extraction of protein-residue associations in the biomedical literature, vol. 2. pp 59–65. IEEE, Honolulu. http://ieeexplore.ieee.org/document/6147049/ .
Xia N, Lin H, Yang Z, Li Y (2011) Combining multiple disambiguation methods for gene mention normalization. Expert Syst Appl38(7): 7994–7999.
Sarker A, Gonzalez G (2015) Portable automatic text classification for adverse drug reaction detection via multi-corpus training. J Biomed Inform53: 196–207.
Wu JL, Yu LC, Chang PC (2012) Detecting causality from online psychiatric texts using inter-sentential language patterns. BMC Med Inform Dec Making12(1): 1–10.
Abacha AB, Zweigenbaum P (2011) A hybrid approach for the extraction of semantic relations from MEDLINE abstracts. Lect Notes Comput Sci (Incl Subseries Lect Notes Artif Intell Lect Notes Bioinforma)6609 LNCS(PART 2): 139–150.
Yu LC, Wu CH, Jang FL (2007) Psychiatric consultation record retrieval using scenario-based representation and multilevel mixture model. IEEE IEEE Trans Inf Technol Biomed11(4): 415–427.
Musto C, Semeraro G, Lops P, Gemmis MD (2015) CrowdPulse: a framework for real-time semantic analysis of social streams. Inf Syst54: 127–146.
García-Moya L, Kudama S, Aramburu MJ, Berlanga R (2013) Storing and analysing voice of the market data in the corporate data warehouse. Inf Syst Front15(3): 331–349.
Eugenio BD, Green N, Subba R (2013) Detecting life events in feeds from Twitter In: ICSC 2013: Proceedings of the IEEE Seventh International Conference on Semantic Computing, 274–277. IEEE, Irvine, http://ieeexplore.ieee.org/document/6693529/ .
Chapter Google Scholar
Torunoglu D, Telseren G, Sagturk O, Ganiz MC (2013) Wikipedia based semantic smoothing for twitter sentiment classification In: INISTA 2013: Proceedings of the IEEE International Symposium on Innovations in Intelligent Systems and Applications, 1–5. IEEE, Albena.
Cao Q, Duan W, Gan Q (2011) Exploring determinants of voting for the “helpfulness” of online user reviews: a text mining approach. Decis Support Syst50(2): 511–521.
Levi A, Mokryn O, Diot C, Taft N (2012) Finding a needle in a haystack of reviews: cold start context-based hotel recommender system In: RecSys’12: Proceedings of the sixth ACM Conference on Recommender Systems, 115–122. ACM, New York.
He W, Shen J, Tian X, Li Y, Akula V, Yan G, et al (2015) Gaining competitive intelligence from social media data: evidence from two largest retail chains in the world. Ind Manag Data Syst115(9): 1622–1636.
He W, Tian X, Chen Y, Chong D (2016) Actionable social media competitive analytics for understanding customer experiences. J Comput Inf Syst56(2): 145–155.
Tian X, He W, Tao R, Akula V (2016) Mining online hotel reviews: a case study from hotels in China In: AMCIS 2016: Proceedings of the 22nd Americas Conference on Information Systems, 1–8.
ACM - Asian and Low-Resource Language Information Processing (TALLIP). http://tallip.acm.org/ . Accessed 8 June 2016.
Chen CL, Liu CL, Chang YC, Tsai HP (2011) Mining opinion holders and opinion patterns in US financial statements In: TAAI 2011: Proceedings of the International Conference on Technologies and Applications of Artificial Intelligence, 62–68. IEEE, Chung-Li,
Chen J, Liu J, Yu W, Wu P (2009) Combining lexical stability and improved lexical chain for unsupervised word sense disambiguation In: KAM’09: Proceedings of the Second International Symposium on Knowledge Acquisition and Modeling, 430–433. IEEE, Wuhan. http://ieeexplore.ieee.org/document/5362135/ .
Rusu D, Fortuna B, Grobelnik M, Mladenic D (2009) Semantic graphs derived from triplets with application in document summarization. Informatica (Slovenia)33(3): 357–362.
Krachina O, Raskin V, Triezenberg K (2007) Reconciling privacy policies and regulations: ontological semantics perspective In: Human Interface and the Management of Information. Interacting in Information Environments, 730–739. Springer, Berlin,
Mansuy T, Hilderman RJ (2006) A characterization of WordNet features in Boolean models for text classification In: AusDM 2006: Proceedings of the fifth Australasian Conference on Data Mining and Analystics, 103–109. Australian Computer Society, Inc, Darlinghurst,
Ciaramita M, Gangemi A, Ratsch E, Šaric J, Rojas I (2005) Unsupervised learning of semantic relations between concepts of a molecular biology ontology In: IJCAI’05: Proceedings of the 19th International Joint Conference on Artificial Intelligence, 659–664. Morgan Kaufmann Publishers Inc., San Francisco, CA.
Kim K, Chung BS, Choi Y, Lee S, Jung JY, Park J (2014) Language independent semantic kernels for short-text classification. Expert Syst Appl41(2): 735–743.
Gujraniya D, Murty MN (2012) Efficient classification using phrases generated by topic models In: ICPR 2012: Proceedings of the 21st International Conference on Pattern Recognition, 2331–2334. IEEE, Tsukuba,
Du C, Zhuang F, He Q, Shi Z (2012) Multi-task semi-supervised semantic feature learning for classification In: ICDM 2012: Proceedings of the IEEE 12th International Conference on Data Mining, 191–200. IEEE, Brussels, http://ieeexplore.ieee.org/document/6413903/ .
Wu Q, Zhang C, Deng X, Jiang C (2011) LDA-based model for topic evolution mining on text In: ICCSE 2011: Proceedings of the 6th International Conference on Computer Science & Education, 946–949. IEEE, Singapore,
Lu X, Zheng B, Velivelli A, Zhai C (2006) Enhancing text categorization with semantic-enriched representation and training data augmentation. J Am Med Inform Assoc13(5): 526–535.
Wu J, Dang Y, Pan D, Xuan Z, Liu Q (2010) Textual knowledge representation through the semantic-based graph structure in clustering applications In: HICSS 2010: Proceedings of the 43rd Hawaii International Conference on System Sciences, 1–8. IEEE, Washington,
Princeton University - WordNet. http://wordnet.princeton.edu/ . Accessed 8 June 2016.
Fellbaum C (1998) WordNet: an electronic lexical database. MIT Press, Cambridge.
MATH Google Scholar
Weller K (2010) Knowledge representation in the social semantic web. Walter de Gruyter.
Weller K, et al (2007) Folksonomies and ontologies: two new players in indexing and knowledge representation In: Proceedings of the Online Information Conference, 108–115.
Wei TA, Lu YC, Chang HB, Zhou QA, Bao XD (2015) A semantic approach for text clustering using WordNet and lexical chains. Expert Syst Appl42(4): 2264–2275.
Li J, Zhao Y, Liu B (2009) Fully automatic text categorization by exploiting wordnet In: Information Retrieval Technology, 1–12. Springer, Berlin,
Mansuy TN, Hilderman RJ (2006) Evaluating WordNet features in text classification models In: FLAIRS Conference 2006: Proceedings of the Nineteenth International Florida Artificial Intelligence Research Society Conference, 568–573. AAAI PRESS, Florida,
Shin Y, Ahn Y, Kim H, Lee SG (2015) Exploiting synonymy to measure semantic similarity of sentences In: IMCOM ’15: Proceedings of the 9th International Conference on Ubiquitous Information Management and Communication, 40:1–40:4. ACM, New York,
Batet M, Valls A, Gibert K (2010) Performance of ontology-based semantic similarities in clustering In: Artificial Intelligence and Soft Computing, 281–288. Springer, Berlin,
Basu S, Mooney RJ, Pasupuleti KV, Ghosh J (2001) Evaluating the novelty of text-mined rules using lexical knowledge In: KDD’01: Proceedings of the seventh ACM SIGKDD international conference on Knowledge discovery and data mining, 233–238. ACM, San Francisco,
Wikipedia. https://www.wikipedia.org/ . Accessed 8 June 2016.
Kim HJA, Hong KJA, Chang JYb (2015) Semantically enriching text representation model for document clustering In: Proceedings of the ACM Symposium on Applied Computing,922–925. ACM, New York, http://dl.acm.org.ez67.periodicos.capes.gov.br/citation.cfm?id=2696055 .
Yun J, Jing L, Yu J, Huang H (2011) Unsupervised feature weighting based on local feature relatedness In: Advances in Knowledge Discovery and Data Mining, 38–49. Springer, Berlin,
Gabrilovich E, Markovitch S (2009) Wikipedia-based semantic interpretation for natural language processing. J Artif Intell Res34: 443–498.
Hu X, Zhang X, Lu C, Park EK, Zhou X (2009) Exploiting Wikipedia as external knowledge for document clustering In: KDD’09: Proceedings of the 15th ACM SIGKDD international conference on Knowledge discovery and data mining, 389–396. ACM, New York,
Mizzaro S, Pavan M, Scagnetto I, Valenti M (2014) Short text categorization exploiting contextual enrichment and external knowledge In: Proceedings of the First International Workshop on Social Media Retrieval and Analysis, 57–62. ACM, New York,
Janik M, Kochut KJ (2008) Wikipedia in action: ontological knowledge in text categorization In: ICSC 2008: Proceedings of the International Conference on Semantic Computing, 268–275. IEEE, Santa Monica,
Chang MW, Ratinov LA, Roth D, Srikumar V (2008) Importance of semantic representation: dataless classification In: AAAI-08: Proceedings of the Twenty-Third AAAI Conference on Artificial Intelligence, 830–835.
Medelyan O, Milne D, Legg C, Witten IH (2009) Mining meaning from Wikipedia. Int J Human-Computer Stud67(9): 716–754.
HowNet Knowledge Database. http://www.keenage.com/ . Accessed 8 June 2016.
Jin CX, Zhou HY, Bai QC (2012) Short text clustering algorithm with feature keyword expansion. Adv Mater Res532: 1716–1720.
Liu Z, Yu W, Chen W, Wang S, Wu F (2010) Short text feature selection for micro-blog mining In: CiSE 2010: Proceedings of the International Conference on Computational Intelligence and Software Engineering, 1–4. IEEE, Wuhan,
Hu P, He T, Ji D, Wang M (2004) A study of Chinese text summarization using adaptive clustering of paragraphs In: CIT’04: Proceedings of the Fourth International Conference on Computer and Information Technology, 1159–1164. IEEE, Wuhan,
Zhu ZY, Dong SJ, Yu CL, He J (2011) A text hybrid clustering algorithm based on HowNet semantics. Key Eng Mater474: 2071–2078.
Zheng D, Liu H, Zhao T (2011) Search results clustering based on a linear weighting method of similarity In: IALP 2011: Proceedings of the International Conference on Asian Language Processing, 123–126. IEEE, Penang,
Wang R (2010) Cognitive-based emotion classifier of Chinese vocabulary design In: ISISE 2010: Proceedings of the International Symposium on Information Science and Engineering, 582–585. IEEE.
Thorleuchter D, Van den Poel D (2014) Semantic compared cross impact analysis. Expert Syst Appl41(7): 3477–3483.
Roussinov D, Turetken O (2009) Exploring models for semantic category verification. Inf Syst34(8): 753–765.
Zelikovitz S, Kogan M (2006) Using Web searches on important words to create background sets for LSI classification In: FLAIRS Conference 2006: Proceedings of the Nineteenth International Florida Artificial Intelligence Research Society Conference, 298–603.
SentiWordNet. http://sentiwordnet.isti.cnr.it/ . Accessed 8 June 2016.
Al Nasseri A, Tucker A, de Cesare S (2015) Quantifying StockTwits semantic terms’ trading behavior in financial markets: an effective application of decision tree algorithms. Expert Syst Appl42(23): 9192–9210.
Kumar V, Minz S (2013) Mood classifiaction of lyrics using SentiWordNet In: ICCCI 2013: Proceedings of the International Conference on Computer Communication and Informatics, 1–5. IEEE, Coimbatore,
Unified Medical Language System (UMLS) Metathesaurus. https://www.nlm.nih.gov/research/umls/knowledge_sources/metathesaurus/ . Accessed 8 June 2016.
Garla VN, Brandt C (2012) Ontology-guided feature engineering for clinical text classification. J Biomed Inform45(5): 992–998.
Plaza L, Díaz A, Gervás P (2011) A semantic graph-based approach to biomedical summarisation. Artif Intell Med53(1): 1–14.
Aljaber B, Martinez D, Stokes N, Bailey J (2011) Improving MeSH classification of biomedical articles using citation contexts. J Biomed Inform44(5): 881–896.
Medical Subject Headings (MeSH). https://www.nlm.nih.gov/mesh/ . Accessed 8 June 2016.
Logeswari S, Premalatha K (2013) Biomedical document clustering using ontology based concept weight In: ICCCI 2013: Proceedings of the International Conference on Computer Communication and Informatics, 1–4. IEEE, Coimbatore,
Nguyen SH, Jaśkiewicz G, Świeboda W, Nguyen HS (2012) Enhancing search result clustering with semantic indexing In: SoICT’12: Proceedings of the Third Symposium on Information and Communication Technology, 71–80. ACM, New York,
Ginter F, Pyysalo S, Boberg J, Järvinen J, Salakoski T (2004) Ontology-based feature transformations: a data-driven approach In: Advances in Natural Language Processing, 279–290. Springer, Berlin,
Kanavos A, Makris C, Theodoridis E (2012) On topic categorization of PubMed query results In: Artificial Intelligence Applications and Innovations, 556–565. Springer.
Zheng HT, Borchert C, Kim HG (2008) Exploiting gene ontology to conceptualize biomedical document collections In: The Semantic Web, 375–389. Springer, Berlin,
Jin B, Muller B, Zhai C, Lu X (2008) Multi-label literature classification based on the Gene Ontology graph. BMC Bioinforma9(1): 525.
Mannai M, Ben Abdessalem Karaa W (2013) Bayesian information extraction network for Medline abstract. In: 2013 World Congress on Computer and Information Technology (WCCIT), 1–3. IEEE, Sousse,
Jiana B, Tingyu L, Tianfang Y (2012) Event information extraction approach based on complex Chinese texts In: IALP 2012: Proceedings of the International Conference on Asian Language Processing, 61–64.
Hengliang W, Weiwei Z (2012) A web information extraction method based on ontology. Adv Inf Sci Serv Sci4(8): 199–206.
Aghassi H, Sheykhlar Z (2012) Extending information retrieval by adjusting text feature vectors. Commun Comput Inform Sci295 CCIS: 133–142.
Bharathi G, Venkatesan D (2012) Improving information retrieval using document clusters and semantic synonym extraction. J Theor Appl Inf Technol36(2): 167–173.
Egozi O, Markovitch S, Gabrilovich E (2011) Concept-based information retrieval using explicit semantic analysis. ACM Trans Inf Syst29(2): 8:1–8:34.
Nassirtoussi AK, Aghabozorgi S, Wah TY, Ngo DCL (2015) Text mining of news-headlines for FOREX market prediction: a multi-layer dimension reduction algorithm with semantics and sentiment. Expert Syst Appl42(1): 306–324.
Batool R, Khattak AM, Maqbool J, Lee S (2013) Precise tweet classification and sentiment analysis In: 2013 IEEE/ACIS 12th International Conference on Computer and Information Science (ICIS), 461–466. IEEE, Niigata,
Veselovská K (2012) Sentence-level sentiment analysis in Czech In: WIMS’12:Proceedings of the 2Nd International Conference on Web Intelligence, Mining and Semantics, 65:1–65:4. ACM, New York,
Petersen MK, Hansen LK (2012) On an emotional node: modeling sentiment in graphs of action verbs In: 2012 International Conference on Audio, Language and Image Processing, 308–313. IEEE, Shanghai,
Domínguez García R, Schmidt S, Rensing C, Steinmetz R (2012) Automatic taxonomy extraction in different languages using wikipedia and minimal language-specific information. Lect Notes Comp Sci (Incl Subseries Lect Notes Artif Intell Lect Notes Bioinforma)7181 LNCS(PART 1): 42–53.
Punuru J, Chen J (2012) Learning non-taxonomical semantic relations from domain texts. J Intell Inf Syst38(1): 191–207.
Stenetorp P, Soyer H, Pyysalo S, Ananiadou S, Chikayama T (2012) Size (and domain) matters: evaluating semantic word space representations for biomedical text In: SMBM 2012: Proceedings of the 5th International Symposium on Semantic Mining in Biomedicine,42–49.
Froud H, Lachkar A, Ouatik SA (2012) Stemming versus light stemming for measuring the simitilarity between Arabic words with latent semantic analysis model In: 2012 Colloquium in Information Science and Technology, 69–73. IEEE, Fez,
Kuhn A, Ducasse S, Gírba T (2007) Semantic clustering: identifying topics in source code. Inf Softw Technol49: 230–243.
Blei DM, Ng AY, Jordan MI (2003) Latent Dirichlet allocation. J Mach Learn Res3(Jan): 993–1022.
Zrigui M, Ayadi R, Mars M, Maraoui M (2012) Arabic text classification framework based on latent dirichlet allocation. J Comput Inf Technol20(2): 125–140.
Liu Z, Li M, Liu Y, Ponraj M (2011) Performance evaluation of latent Dirichlet allocation in text mining In: FSKD 2011: Proceedings of the Eighth International Conference on Fuzzy Systems and Knowledge Discovery, 2695–2698. IEEE, Shanghai.
Xiang W, Yan J, Ruhua C, Hua F (2013) Improving text categorization with semantic knowledge in Wikipedia. IEICE Trans Inf Syst96(12): 2786–2794.
Spanakis G, Siolas G, Stafylopatis A (2012) Exploiting Wikipedia knowledge for conceptual hierarchical clustering of documents. Comput J55(3): 299–312.
Article MATH Google Scholar
Andreasen T, Bulskov H, Jensen PA, Lassen T (2011) Extracting conceptual feature structures from text In: ISMIS 2011: Proceedings 19th International Symposium on Methodologies for Intelligent Systems, 396–406. Springer, Berlin,
Goossen F, IJntema W, Frasincar F, Hogenboom F, Kaymak U (2011) News personalization using the CF-IDF semantic recommender In: WIMS’11: Proceedings of the International Conference on Web Intelligence, Mining and Semantics, 10. ACM, New York,
Huang A, Milne D, Frank E, Witten IH (2008) Clustering documents with active learning using Wikipedia In: ICDM’08: Eighth IEEE International Conference on Data Mining, 839–844. IEEE, Pisa,
Gabrilovich E, Markovitch S (2007) Computing semantic relatedness using Wikipedia-based explicit semantic analysis In: IJCAI-07: Proceedings of the 20th International Joint Conference on Artifical Intelligence, 1606–1611. Morgan Kaufmann Publishers Inc, San Francisco, http://dl.acm.org.ez67.periodicos.capes.gov.br/citation.cfm?id=1625535 .
Navigli R, Faralli S, Soroa A, de Lacalle O, Agirre E (2011) Two birds with one stone: learning semantic models for text Categorization and word sense disambiguation In: CIKM’11: Proceedings of the 20th ACM International Conference on Information and Knowledge Management, 2317–2320. ACM, Glasgow,
Mostafa MS, Haggag MH, Gomaa WH (2008) Document clustering using word sense disambiguation In: SEDE 2008: Proceedings of 17th International Conference on Software Engineering and Data Engineering, 19–24.
Andreopoulos B, Alexopoulou D, Schroeder M (2008) Word sense disambiguation in biomedical ontologies with term co-occurrence analysis and document clustering. Int J Data Min Bioinforma2(3): 193–215.
Koeling R, McCarthy D, Carroll J (2007) Text categorization for improved priors of word meaning In: Computational Linguistics and Intelligent Text Processing, 241–252. Springer, Berlin,
Sharma A, Swaminathan R, Yang H (2010) A verb-centric approach for relationship extraction in biomedical text In: ICSC 2010: Proceedings of the IEEE Fourth International Conference on Semantic Computing, 377–385. IEEE, Pittsburgh,
Wang W, Zhao D, Zou L, Wang D, Zheng W (2010) Extracting 5W1H event semantic elements from Chinese online news In: WAIM 2010: Proceedings of the Workshops of the 11th International Conference on Web-Age Information Management, 644–655. Springer, Berlin,
Rebholz-Schuhmann D, Jimeno-Yepes A, Arregui M, Kirsch H (2010) Measuring prediction capacity of individual verbs for the identification of protein interactions. J Biomed Inform43(2): 200–207.
Van Der Horn P, Bakker B, Geleijnse G, Korst J, Kurkin S (2008) Classifying verbs in biomedical text using subject-verb-object relationships In: SMBM 2008: Proceedings of the 3rd International Symposium on Semantic Mining in Biomedicine, 137–140.
Kontos J, Malagardi I, Alexandris C, Bouligaraki M (2000) Greek verb semantic processing for stock market text mining In: NLP’00: Proceedings of the Second International Conference on Natural Language Processing, 395–405. Springer-Verlag, London.
Stankov I, Todorov D, Setchi R (2013) Enhanced cross-domain document clustering with a semantically enhanced text stemmer (SETS). Int J Knowl-Based Intell Eng Syst17(2): 113–126.
Huang CH, Yin J, Hou F (2011) A text similarity measurement combining word semantic information with TF-IDF method. Jisuanji Xuebao(Chin J Comput)34(5): 856–864.
Doan S, Kawazoe A, Conway M, Collier N (2009) Towards role-based filtering of disease outbreak reports. J Biomed Inform42(5): 773–780.
Meng X, Chen Q, Wang X (2008) Semantic feature reduction in chinese document clustering In: SMC 2008: Proceedings of the IEEE International Conference on Systems, Man and Cybernetics, 3721–3726. IEEE, Singapore,
Freitas A, O’Riain S, Curry E, da Silva JCP, Carvalho DS (2013) Representing texts as contextualized entity-centric linked data graphs In: DEXA 2013: Proceedings of the 24th International Workshop on Database and Expert Systems Applications, 133–137. IEEE, Los Alamitos,
Fathy I, Fadl D, Aref M (2012) Rich semantic representation based approach for text generation In: INFOS 2012: Proceedings of the 8th International Conference on Informatics and Systems, NLP–20. IEEE, Cairo,
Wu J, Xuan Z, Pan D (2011) Enhancing text representation for classification tasks with semantic graph structures. Int J Innov Comput Inf Control (ICIC)7(5): 2689–2698.
Alencar ROD, Davis Jr CA, Gonçalves MA (2010) Geographical classification of documents using evidence from Wikipedia In: GIR ’10: Proceedings of the 6th Workshop on Geographic Information Retrieval, 12. ACM, New York,
Smirnov I, Tikhomirov I (2009) Heterogeneous semantic networks for text representation in intelligent search engine EXACTUS In: SENSE’09: Proceedings of the Workshop on Conceptual Structures for Extracting Natural Language Semantics, 1–9.
Chau R, Tsoi AC, Hagenbuchner M, Lee V (2009) A conceptlink graph for text structure mining In: ACSC’09: Proceedings of the Thirty-Second Australasian Conference on Computer Science - Volume 91, 141–150. Australian Computer Society, Inc., Darlinghurst,
Schmidhuber J (2015) Deep learning in neural networks: an overview. Neural Netw61: 85–117.
Lebret R, Collobert R (2015) Rehabilitation of count-based models for word vector representations. Lect Notes Comput Sci (Incl Subseries Lect Notes Artif Intell Lect Notes Bioinforma)9041: 417–429.
Li R, Shindo H (2015) Distributed document representation for document classification. Lect Notes Comput Sci (Incl Subseries Lect Notes Artif Intell Lect Notes Bioinforma)9077: 212–225.
Sohrab MG, Miwa M, Sasaki Y (2015) Centroid-means-embedding: an approach to infusing word embeddings into features for text classification. Lect Notes Comput Sci (Incl Subseries Lect Notes Artif Intell Lect Notes Bioinforma)9077: 289–300.
Wang P, Xu B, Xu J, Tian G, Liu CL, Hao H (2016) Semantic expansion using word embedding clustering and convolutional neural network for improving short text classification. Neurocomputing174: 806–814.
Zhang C, Zhang L, Wang CJ, Xie JY (2014) Text summarization based on sentence selection with semantic representation In: Proceedings of the International Conference on Tools with Artificial Intelligence,Vol. 2014-December. IEEE, Limassol. 584–590.
Vulić I, Moens MF (2015) Monolingual and cross-lingual information retrieval models based on (Bilingual) word embeddings In: SIGIR’15: Proceedings of the 38th International ACM SIGIR Conference on Research and Development in Information Retrieval, 363–372. ACM, New York,
Kamal A, Abulaish M, Anwar T (2012) Mining feature-opinion pairs and their reliability scores from web opinion sources In: WIMS’12: Proceedings of the 2nd International Conference on Web Intelligence, Mining and Semantics, 15. ACM, New York,
Kong L, Yan R, He Y, Zhang Y, Zhang Z, Fu L (2011) DVD: a model for event diversified versions discovery In: Web Technologies and Applications, 168–180. Springer, Berlin,
Jing L, Yun J, Yu J, Huang J (2011) High-order co-clustering text data on semantics-based representation model In: Advances in Knowledge Discovery and Data Mining, 171–182. Springer, Berlin,
Krajewski R, Rybinski H, Kozlowski M (2016) A novel method for dictionary translation. J Intell Inf Syst47(3): 491–514.
Luo Z, Miotto R, Weng C (2013) A human–computer collaborative approach to identifying common data elements in clinical trial eligibility criteria. J Biomed Inform46(1): 33–39.
Kayed A (2005) Building e-laws ontology: new approach In: Proceedings of the On the Move to Meaningful Internet Systems 2005: OTM 2005 Workshops, 826–835. Springer, Berlin,
Sevenster M, van Ommering R, Qian Y (2012) Automatically correlating clinical findings and body locations in radiology reports using MedLEE. J Digit Imaging25(2): 240–249.
Volkova S, Caragea D, Hsu WH, Drouhard J, Fowles L (2010) Boosting biomedical entity extraction by using syntactic patterns for semantic relation discovery In: WI-IAT 2010: Proceedings of the IEEE/WIC/ACM International Conference on Web Intelligence and Intelligent Agent Technology, 272–278. IEEE, Toronto.
Waltinger U, Mehler A (2009) Social semantics and its evaluation by means of semantic relatedness and open topic models In: WI-IAT’09: Proceedings of the 2009 IEEE/WIC/ACM International Joint Conference on Web Intelligence and Intelligent Agent Technology-Volume 01, 42–49. IEEE Computer Society, Milan,
Kass A, Cowell-Shah C (2006) Using lightweight NLP and semantic modeling to realize the internet’s potential as a corporate radar In: AAAI Fall Symposium. AAAI PRESS.
Blake C (2010) Beyond genes, proteins, and abstracts: identifying scientific claims from full-text biomedical articles. J Biomed Inform43(2): 173–189.
Hu J, Fang L, Cao Y, Zeng HJ, Li H, Yang Q, et al (2008) Enhancing text clustering by leveraging Wikipedia semantics In: SIGIR’08: Proceedings of the 31st annual international ACM SIGIR conference on Research and development in information retrieval, 179–186. ACM, New York,
Lu CY, Lin SH, Liu JC, Cruz-Lara S, Hong JS (2010) Automatic event-level textual emotion sensing using mutual action histogram between entities. Expert Syst Appl37(2): 1643–1653.
Ahmed ST, Nair R, Patel C, Davulcu H (2009) BioEve: bio-molecular event extraction from text using semantic classification and dependency parsing In: BioNLP’09: Proceedings of the Workshop on Current Trends in Biomedical Natural Language Processing: Shared Task, 99–102. Association for Computational Linguistics.
Jain AK (2010) Data clustering: 50 years beyond k-means. Pattern Recogn Lett31(8): 651–666.
Wordle. http://www.wordle.net/ . Accessed 15 June 2016.
Download references
Acknowledgements
The authors would like to thank the financial support of grant #132666/2016-2, National Council for Scientific and Technological Development (CNPq); grants #2013/14757-6, #2014/08996-0, and #2016/07620-2, São Paulo Research Foundation (FAPESP); and Coordination for the Improvement of Higher Education Personnel (CAPES).
Authors’ contributions
RAS and SOR planned this systematic mapping study. RAS conducted its first cycle (searches performed in January 2014). JA and RAS conducted its second cycle (searches performed in February 2016). RAS and SOR analyzed the results and drafted the manuscript after the first cycle and updated it after the second cycle. JA was involved in updating the manuscript with the second cycle results. All authors revised and approved the final manuscript.
Competing interests
The authors declare that they have no competing interests.
Publisher’s Note
Springer Nature remains neutral with regard to jurisdictional claims in published maps and institutional affiliations.
Author information
Authors and affiliations.
Laboratório de Inteligência Computacional (LABIC), Instituto de Ciências Matemáticas e de Computação (ICMC), Universidade de São Paulo (USP), São Carlos, P.O. Box 668, 13561-970, SP, Brazil
Roberta Akemi Sinoara, João Antunes & Solange Oliveira Rezende
You can also search for this author in PubMed Google Scholar
Corresponding author
Correspondence to Roberta Akemi Sinoara .
Rights and permissions
Open Access This article is distributed under the terms of the Creative Commons Attribution 4.0 International License ( http://creativecommons.org/licenses/by/4.0/ ), which permits unrestricted use, distribution, and reproduction in any medium, provided you give appropriate credit to the original author(s) and the source, provide a link to the Creative Commons license, and indicate if changes were made.
Reprints and permissions
About this article
Cite this article.
Sinoara, R., Antunes, J. & Rezende, S. Text mining and semantics: a systematic mapping study. J Braz Comput Soc 23 , 9 (2017). https://doi.org/10.1186/s13173-017-0058-7
Download citation
Received : 24 March 2017
Accepted : 01 June 2017
Published : 29 June 2017
DOI : https://doi.org/10.1186/s13173-017-0058-7
Share this article
Anyone you share the following link with will be able to read this content:
Sorry, a shareable link is not currently available for this article.
Provided by the Springer Nature SharedIt content-sharing initiative
- Systematic review
- Text mining
- Text semantics
- Tools and Resources
- Customer Services
- Applied Linguistics
- Biology of Language
- Cognitive Science
- Computational Linguistics
- Historical Linguistics
- History of Linguistics
- Language Families/Areas/Contact
- Linguistic Theories
- Neurolinguistics
- Phonetics/Phonology
- Psycholinguistics
- Sign Languages
- Sociolinguistics
- Share This Facebook LinkedIn Twitter

Article contents
Lexical semantics.
- Dirk Geeraerts Dirk Geeraerts University of Leuven
- https://doi.org/10.1093/acrefore/9780199384655.013.29
- Published online: 25 January 2017
Lexical semantics is the study of word meaning. Descriptively speaking, the main topics studied within lexical semantics involve either the internal semantic structure of words, or the semantic relations that occur within the vocabulary. Within the first set, major phenomena include polysemy (in contrast with vagueness), metonymy, metaphor, and prototypicality. Within the second set, dominant topics include lexical fields, lexical relations, conceptual metaphor and metonymy, and frames. Theoretically speaking, the main theoretical approaches that have succeeded each other in the history of lexical semantics are prestructuralist historical semantics, structuralist semantics, and cognitive semantics. These theoretical frameworks differ as to whether they take a system-oriented rather than a usage-oriented approach to word-meaning research but, at the same time, in the historical development of the discipline, they have each contributed significantly to the descriptive and conceptual apparatus of lexical semantics.
- structuralism
- cognitive semantics
- lexical field theory
- componential analysis
- semasiology
- onomasiology
Lexical semantics is the study of word meaning. The following first presents an overview of the main phenomena studied in lexical semantics and then charts the different theoretical traditions that have contributed to the development of the field. The focus lies on the lexicological study of word meaning as a phenomenon in its own right, rather than on the interaction with neighboring disciplines. This implies that morphological semantics, that is the study of the meaning of morphemes and the way in which they combine into words, is not covered, as it is usually considered a separate field from lexical semantics proper. Similarly, the interface between lexical semantics and syntax will not be discussed extensively, as it is considered to be of primary interest for syntactic theorizing. There is no room to discuss the relationship between lexical semantics and lexicography as an applied discipline. For an entry-level text on lexical semantics, see Murphy ( 2010 ); for a more extensive and detailed overview of the main historical and contemporary trends of research in lexical semantics, see Geeraerts ( 2010 ).
1 The Descriptive Scope of Lexical Semantics
The main phenomena studied by lexical semantics are organized along two dimensions. First, it makes a difference whether we look at semantic phenomena within individual words or whether we look at meaningful structures within the vocabulary as a whole. Terminologically, this difference of perspective can be expressed by referring to a ‘semasiological’ and an ‘onomasiological’ perspective. (Semasiology looks at the relationship between words and meaning with the word as starting point: it is basically interested in the polysemy of words. Onomasiology takes the converse perspective: given a concept to be expressed or a thing to be categorized, what options does a language offer, and how are the choices made?) Second, a distinction needs to be made between an approach that focuses on elements and relations only and one that takes into account the differences of structural weight between those elements and relations. Even though the terms are not perfect, we can use the terms ‘qualitative approach’ and ‘quantitative approach’ to refer to this second distinction. If we cross-classify the two distinctions, we get four groups of topics. ‘Qualitative’ semasiology deals with word senses and the semantic links among those senses, like metaphor and metonymy at the level of individual words. ‘Qualitative’ onomasiology deals with the semantic relations among lexical items, like lexical fields and lexical relations. ‘Quantitative’ semasiology deals with prototype effects: differences of salience and structural weight within an item or a meaning. ‘Quantitative’ onomasiology deals with salience effects in the lexicon at large, like basic-level phenomena.
Table 1 The Descriptive Scope of Lexical Semantics
semasiology | onomasiology | |
---|---|---|
‘qualitative’ perspectives: elements and relations | ||
‘quantitative’ perspectives: differences of salience | Prototype effects and radial sets | Basic levels and onomasiological salience |
The four groups of topics are summarized in Table 1 . As will be seen later, this schematic representation is also useful to identify the contribution of the various theoretical approaches that have successively dominated the evolution of lexical semantics.
1.1 Polysemy and vagueness
Establishing which meanings a word has is arguably the basic step in lexical semantic research. Polysemy is the common term for the situation in which a lexical item has more than one meaning, such as when late can mean ‘after the usual, expected, or agreed time’ ( I am late again ), ‘advanced in day or night’ ( a late dinner ), or ‘no longer alive’ ( my late aunt Polly ). Terminologically speaking, polysemy needs to be contrasted with homonymy and, more importantly, vagueness. When two (or more) words have the same shape, such as bank (‘slope, elevation in sea or river bed’) and bank (‘financial institution’), they are homonyms; whereas polysemy refers to multiplicity of meaning within a single word, the multiplicity is distributed over various words in the case of homonymy. As such, making a distinction between polysemy and homonymy comes down to determining whether we are dealing with one and the same word or with two different ones. The distinction between vagueness and polysemy involves the question of whether a particular piece of semantic information is part of the underlying semantic structure of the item or is the result of a contextual (and hence pragmatic) specification. For instance, neighbor is not polysemous between the readings ‘male dweller next door’ and ‘female dweller next door,’ in the sense that the utterance my neighbor is a civil servant will not be recognized as requiring disambiguation in the way that she is smart might ( Do you mean ‘bright’ or ‘stylish’? ). The semantic information that is associated with the item neighbor in the lexicon does not, in other words, contain a specification regarding sex; neighbor is vague (or general, or unspecified) as to the dimension of gender.
To decide between polysemy and vagueness, a number of tests can be invoked. The three main ones are the following. First, from a truth-theoretical point of view, a lexical item is polysemous if it can simultaneously be clearly true and clearly false of the same referent. Considering the readings ‘harbor’ and ‘fortified sweet wine from Portugal’ of port , the polysemy of that item is established by sentences such as Sandeman is a port (in a bottle) , but not a port (with ships). This criterion basically captures a semantic intuition: are two interpretations of a given expression intuitively sufficiently dissimilar so that one may be said to apply and the other not?
Second, linguistic tests involve syntactic rather than semantic intuitions. Specifically, they are based on acceptability judgments about sentences that contain two related occurrences of the item under consideration (one of which may be implicit). If the grammatical relationship between both occurrences requires their semantic identity, the resulting sentence may be an indication for the polysemy of the item. For instance, the so-called identity test involves ‘identity-of-sense anaphora.’ Thus, at midnight the ship passed the port, and so did the bartender is awkward if the two lexical meanings of port are at stake. Disregarding puns, it can only mean that the ship and the bartender alike passed the harbor, or conversely that both moved a particular kind of wine from one place to another. A mixed reading, in which the first occurrence of port refers to the harbor and the second to wine, is normally excluded. By contrast, the fact that the notions ‘vintage sweet wine from Portugal’ and ‘blended sweet wine from Portugal’ can be combined in Vintage Noval is a port, and so is blended Sandeman indicates that port is vague rather than polysemous with regard to the distinction between blended and vintage wines.
Third, the definitional criterion specifies that an item has more than one lexical meaning if there is no minimally specific definition covering the extension of the item as a whole, and that it has no more lexical meanings than there are maximally general definitions necessary to describe its extension. Definitions of lexical items should be maximally general in the sense that they should cover as large a subset of the extension of an item as possible. Thus, separate definitions for ‘blended sweet fortified wine from Portugal’ and ‘vintage sweet fortified wine from Portugal’ could not be considered definitions of lexical meanings, because they can be brought together under the definition ‘sweet fortified wine from Portugal.’ On the other hand, definitions should be minimally specific in the sense that they should be sufficient to distinguish the item from other nonsynonymous items. A maximally general definition covering both port ‘harbor’ and port ‘kind of wine’ under the definition ‘thing, entity’ is excluded because it does not capture the specificity of port as distinct from other words.
The distinction between polysemy and vagueness is not unproblematic, methodologically speaking. An examination of different basic criteria for distinguishing between polysemy and vagueness reveals, first, that those criteria may be in mutual conflict (in the sense that they need not lead to the same conclusion in the same circumstances) and, second, that each of them taken separately need not lead to a stable distinction between polysemy and vagueness (in the sense that what is a distinct meaning according to one of the tests in one context may be reduced to a case of vagueness according to the same test in another context). Without going into detail (for a full treatment, see Geeraerts, 1993 ), let us illustrate the first type of problem. In the case of autohyponymous words, for instance, the definitional approach does not reveal an ambiguity, whereas the truth-theoretical criterion does. Dog is autohyponymous between the readings ‘Canis familiaris,’ contrasting with cat or wolf , and ‘male Canis familiaris,’ contrasting with bitch . A definition of dog as ‘male Canis familiaris,’ however, does not conform to the definitional criterion of maximal coverage, because it defines a proper subset of the ‘Canis familiaris’ reading. On the other hand, the sentence Lady is a dog, but not a dog , which exemplifies the logical criterion, cannot be ruled out as ungrammatical.
1.2 Semantic Relations
Once senses are identified (and assuming they can be identified with a reasonable degree of confidence), the type of relationship that exists between them needs to be established. The most common classification of semantic relations emerges from the tradition of historical semantics, that is, the vocabulary used to describe synchronic relations between word meanings is essentially the same as the vocabulary used to describe diachronic changes of meaning. In the simplest case, if sense a is synchronically related to sense b by metonymy, then a process of metonymy has acted diachronically to extend sense a to sense b : diachronic mechanisms of semasiological change reappear synchronically as semantic relations among word meanings.
The four basic types are specialization, generalization, metaphor, and metonymy (described here, from a diachronic perspective, as mechanisms rather than synchronic relations). In the case of semantic specialization , the new meaning is a restriction of the old meaning: the new meaning is a subcase of the old. In the case of semantic generalization , the reverse holds: the old meaning is a subcase of the new. Classical examples of specialization are corn (originally a cover-term for all kinds of grain, now specialized to ‘wheat’ in England, to ‘oats’ in Scotland, and to ‘maize’ in the United States), starve (moving from ‘to die’ to ‘to die of hunger’), and queen (originally ‘wife, woman,’ now restricted to ‘king’s wife, or female sovereign’). Examples of generalization are moon (primarily the earth’s satellite, but extended to any planet’s satellite), and French arriver (which originally meant ‘to reach the river’s shore, to embank,’ but which now signifies ‘to reach a destination’ in general). There is a lot of terminological variation in connection with specialization and generalization. ‘Restriction’ and ‘narrowing’ of meaning equal ‘specialization,’ while ‘extension,’ ‘schematization,’ and ‘broadening’ of meaning equal ‘generalization.’ Also, the meanings involved can be said to entertain relations of taxonomical subordination or superordination: in a taxonomy (a tree-like hierarchical classification) of concepts, the specialized meaning is subordinate with regard to the original one, whereas the generalized meaning is superordinate with regard to the original.
Like specialization and generalization, it is convenient and customary to introduce metaphor and metonymy together, even though the relationship is not as close as with the former pair. (More on metaphor and metonymy follows in section 1.6, “Conceptual Metaphor and Metonymy.” ) Metaphor is then said to be based on a relationship of similarity between the old and the new reading, and metonymy on a relationship of contiguity. Current computer terminology yields examples of both types. The desktop of your computer screen, for instance, is not the same as the desktop of your office desk—except that in both cases, it is the space (a literal space in one case, a virtual one in the other) where you position a number of items that you regularly use or that urgently need attention. The computer desktop, in other words, is not literally a desktop in the original sense, but it has a functional similarity with the original: the computer reading is a metaphorical extension of the original office furniture reading. Functional similarities also underlie metaphorical expressions like bookmark , clipboard , file , folder , cut , and paste . Mouse , on the other hand, is also metaphorically motivated, but here, the metaphorical similarity involves shape rather than function. But now consider a statement to the effect that your desktop will keep you busy for the next two weeks, or that you ask aloud where your mouse has gone when you are trying to locate the pointer on the screen. In such cases, desktop and mouse are used metonymically. In the former case, it’s not the virtual space as such that is relevant, but the items that are stored there. In the latter case, it’s not the mouse as such (the thing that you hold in your hand) that you refer to, but the pointer on the screen that is operated by the mouse. The desktop and the stored items, or the mouse and the pointer, have a relationship of real-world connectedness that is usually captured by the notion of ‘contiguity.’ When, for instance, one drinks a whole bottle, it is not the bottle but merely its contents that are consumed: bottle can be used to refer to a certain type of container, and the (spatially contiguous) contents of that container. When lexical semanticians state that metonymical changes are based on contiguity, contiguity should not be understood in a narrow sense as referring to spatial proximity only, but more broadly as a general term for various associations in the spatial, temporal, or causal domain.
1.3 Lexical Fields and Componential Analysis
A lexical field is a set of semantically related lexical items whose meanings are mutually interdependent. The single most influential study in the history of lexical field theory is Trier’s ( 1931 ) monograph, in which he presents a theoretical formulation of the field approach and investigates how the terminology for mental properties evolves from Old High German up to the beginning of the 13th century. Theoretically, Trier emphasizes that only a mutual demarcation of the words under consideration can provide a decisive answer regarding their exact value. Words should not be considered in isolation, but in their relationship to semantically related words: demarcation is always a demarcation relative to other words.
While different conceptions of the notion ‘lexical field’ were suggested after Trier’s initial formulation, the most important development is the emergence of componential analysis as a technique for formalizing the semantic relationships between the items in a field: once a lexical field has been demarcated, the internal relations within the field will have to be described in more detail. It is not sufficient to say that the items in the field are in mutual opposition—these oppositions will have to be identified and defined. Componential analysis is a method for describing such oppositions that takes its inspiration from structuralist phonology: just like phonemes are described structurally by their position on a set of contrastive dimensions, words may be characterized on the basis of the dimensions that structure a lexical field. Componential analysis provides a descriptive model for semantic content, based on the assumption that meanings can be described on the basis of a restricted set of conceptual building blocks—the semantic ‘components’ or ‘features.’
A brief illustration of the principles of componential analysis is given by Pottier ( 1964 ), who provides an example of a componential semantic analysis in his description of a field consisting of, among others, the terms siège , pouf , tabouret , chaise , fauteuil , and canapé (a subfield of the field of furniture terms in French). The word which acts as a superordinate to the field under consideration is siège , ‘seating equipment with legs.’ If we use the dimensions s1 ‘for seating,’ s2 ‘for one person,’ s3 ‘with legs,’ s4 ‘with back,’ s5 ‘with armrests,’ s6 ‘of rigid material,’ then chaise ‘chair’ can be componentially defined as [+ s1, + s2, + s3, + s4, − s5, + s6], and canapé ‘sofa’ as [+ s1, − s2, + s3, + s4, + s5, + s6], and so on.
While componential forms of description are common in formal types of semantic description (see the historical overview in section 2, “The Theoretical Evolution of Lexical Semantics,” specifically section 2.3, “Neostructuralist Semantics” ), the most important theoretical development after the introduction of componential analysis is probably Wierzbicka’s ( 1996 ) attempt to identify a restricted set of some 60 universally valid, innate components. The Natural Semantic Metalanguage aims at defining cross-linguistically transparent definitions by means of those allegedly universal building-blocks.
1.4 Lexical Relations
Like componential analysis, relational semantics, as introduced by Lyons ( 1963 ), develops the idea of describing the structural relations among related words. It, however, restricts the theoretical vocabulary to be used in such a description. In a componential analysis, the features are essentially of a ‘real world’ kind: as in Pottier’s example, they name properties of the things referred to, rather than properties of the meanings as such. But if linguistics is interested in the structure of the language rather than the structure of the world, it may want to use a descriptive apparatus that is more purely linguistic. Relational semantics looks for such an apparatus in the form of sense relations like synonymy (identity of meaning) and antonymy (oppositeness of meaning): the fact that aunt and uncle refer to the same genealogical generation is a fact about the world, but the fact that black and white are opposites is a fact about words and language. Instead of deriving statements about the synonymy or antonymy of a word (and in general, statements about the meaning relations it entertains) from a separate and independent description of the word’s meaning, the meaning of the word could be defined as the total set of meaning relations in which it participates. A traditional approach to synonymy would for instance describe the meaning of both quickly and speedily as ‘in a fast way, not taking up much time,’ and then conclude to the synonymy of both terms on the basis of their definitional identity. Lyons by contrast deliberately eschews such content descriptions, and equates the meaning of a word like quickly with the synonymy relation it has with speedily , plus any other relations of that kind.
In the actual practice of relational semantics, ‘relations of that kind’ specifically include—next to synonymy and antonymy—relations of hyponymy (or subordination) and hyperonymy (or superordination), which are both based on taxonomical inclusion. The major research line in relational semantics involves the refinement and extension of this initial set of relations. The most prominent contribution to this endeavor after Lyons is found in Cruse ( 1986 ). Murphy ( 2003 ) is a thoroughly documented critical overview of the relational research tradition.
1.5 Distributional Relations
Given a Saussurean distinction between paradigmatic and syntagmatic relations, lexical fields as originally conceived are based on paradigmatic relations of similarity. One extension of the field approach, then, consists of taking a syntagmatic point of view. Words may in fact have specific combinatorial features which it would be natural to include in a field analysis. A verb like to comb , for instance, selects direct objects that refer to hair, or hair-like things, or objects covered with hair. Describing that selectional preference should be part of the semantic description of to comb . For a considerable period, these syntagmatic affinities received less attention than the paradigmatic relations, but in the 1950s and 1960s, the idea surfaced under different names. Firth ( 1957 ) for instance introduced the (now widely used) term collocation .
The distributional approach can be more radical than the mere incorporation of lexical combinatorics into the description of words: if the environments in which a word occurs could be used to establish its meaning, lexical semantics could receive a firm methodological basis. The general approach of a distributionalist method is summarized by Firth’s dictum: ‘You shall know a word by the company it keeps,’ that is, words that occur in the same contexts tend to have similar meanings. In the final decades of the 20th century, major advances in the distributional approach to semantics were achieved by applying a distributional way of meaning analysis to large text corpora. Sinclair, a pioneer of the approach, developed his ideas (see Sinclair, 1991 ) through his work on the Collins Cobuild English Language Dictionary , for which a 20-million-word corpus of contemporary English was compiled. In Sinclair’s original conception, a collocational analysis is basically a heuristic device to support the lexicographer’s manual work. A further step in the development of the distributional approach was taken through the application of statistics as a method for establishing the relevance of a collocation and, more broadly, for analyzing the distributional co-occurrence patterns of words (see Glynn & Robinson, 2014 , for a state-of-the-art overview of quantitative corpus semantics).
1.6 Conceptual Metaphor and Metonymy
Metaphorical relations of the kind mentioned in section 1.2 ( “Semantic Relations” ) do not only exist between the readings of a given word: several words may exhibit similar metaphorical patterns. Conceptual metaphor theory, the approach introduced by Lakoff and Johnson ( 1980 ), includes two basic ideas: first, the view that metaphor is a cognitive phenomenon, rather than a purely lexical one; second, the view that metaphor should be analyzed as a mapping between two domains. To illustrate the first point, metaphor comes in patterns that transcend the individual lexical item. A typical example (Lakoff & Johnson, 1980 , pp. 44–45) is the following.
love is a journey Look how far we’ve come. We are at a crossroads. We’ll just have to go our separate ways. We cannot turn back now. We are stuck. This relationship is a dead-end street. I don’t think this relationship is going anywhere. It’s been a long, bumpy road. We have gotten off the track.
The second pillar of conceptual metaphor theory is the analysis of the mappings inherent in metaphorical patterns. Metaphors conceptualize a target domain in terms of the source domain, and such a mapping takes the form of an alignment between aspects of the source and target. For love is a journey , for instance, the following correspondences hold (compare Lakoff & Johnson, 1999 , p. 64).
|
|
---|---|
The travelers | The lovers |
The means of transport | The relationship itself |
The journey | The development of the relationship |
The obstacles encountered | The difficulties experienced |
Decisions about which way to go | Choices about what to do |
The destination of the journey | The goals of the relationship |
Metonymies too can be systematic in the sense that they form patterns that apply to more than just an individual lexical item. Thus, the bottle example mentioned in section 1.2 ( “Semantic Relations” ) exhibits the name of a container (source) being used for its contents (target), a pattern that can be abbreviated as container for contents . Making use of this abbreviated notation, other common types of metonymy are the following: a spatial location for what is located there ( the whole theater was in tears ); a period of time for what happens in that period, for the people who live then, or for what is produced during that period ( the 19th century had a nationalist approach to politics ); a material for the product made from it ( a cork ); the origin for what originates from it ( astrakhan , champagne , emmental ); an activity or event for its consequences (when the blow you have received hurts, it is not the activity of your adversary that is painful, but the physical effects that it has on your body); an attribute for the entity that possesses the attribute ’ ( majesty does not only refer to ‘royal dignity or status,’ but also to the sovereign himself); and of course part for whole ( a hired hand ). The relations can often work in the other direction as well. To fill up the car , for instance, illustrates a type whole for part : it’s obviously only a part of the car that gets filled. For the current state of affairs in metonymy research from a cognitive semantic point of view, see Benczes, Barcelona, and Ruiz de Mendoza Ibáñez ( 2011 ).
Yet another approach to semantic structure in the lexicon focuses on the way our knowledge of the world is organized in larger ‘chunks of knowledge’ and how these interact with language. The most articulate model in this respect is Fillmore’s frame theory (Fillmore & Atkins, 1992 ; and see Ruppenhofer, Ellsworth, Petruck, Johnson, & Scheffczyk, 2006 , for the large-scale application of frame theory in the FrameNet project). Frame theory is specifically interested in the way in which language may be used to perspectivize an underlying conceptualization of the world: it’s not just that we see the world in terms of conceptual models, but those models may be verbalized in different ways. Each different way of bringing a conceptual model to expression so to speak adds another layer of meaning: the models themselves are meaningful ways of thinking about the world, but the way we express the models while talking adds perspective. This overall starting point of Fillmorean frame theory leads to a description on two levels. On the one hand, a description of the referential situation or event consists of an identification of the relevant elements and entities, and the conceptual role they play in the situation or event. On the other hand, the more purely linguistic part of the analysis indicates how certain expressions and grammatical patterns highlight aspects of that situation or event.
An illustration comes from the standard example of frame theory, the commercial transaction frame. The commercial transaction frame involves words like buy and sell . The commercial transaction frame can be characterized informally by a scenario in which one person gets control or possession of something from a second person, as a result of a mutual agreement through which the first person gives the second person a sum of money. Background knowledge involved in this scenario includes an understanding of ownership relations, a money economy, and commercial contracts. The categories that are needed for describing the lexical meanings of the verbs linked to the commercial transaction scene include Buyer, Seller, Goods, and Money as basic categories. Verbs like buy and sell then each encode a certain perspective on the commercial transaction scene by highlighting specific elements of the scene. In the case of buy , for instance, the buyer appears in the participant role of the agent, for instance as the subject of the (active) sentence. In active sentences, the goods then appear as the direct object; the seller and the money appear in prepositional phrases: Paloma bought a book from Teresa for €30 . In the case of sell , on the other hand, it is the seller that appears in the participant role of the agent: Teresa sold a book to Paloma for €30 .
1.8 Prototype Effects and Radial Sets
The prototype-based conception of categorization originated in the mid-1970s with Rosch’s psycholinguistic research into the internal structure of categories (see, among others, Rosch, 1975 ). Rosch concluded that the tendency to define categories in a rigid way clashes with the actual psychological situation. Linguistic categories do not have sharply delimited borderlines. Instead of clear demarcations between equally important conceptual areas, one finds marginal areas between categories that are unambiguously defined only in their focal points. This observation was taken over and elaborated in linguistic lexical semantics (see Hanks, 2013 ; Taylor, 2003 ). Specifically, it was applied not just to the internal structure of a single word meaning, but also to the structure of polysemous words, that is, to the relationship between the various meanings of a word. Four characteristics, then, are frequently mentioned in the linguistic literature as typical of prototypicality.
Prototypical categories cannot be defined by means of a single set of criterial (necessary and sufficient) attributes.
Prototypical categories exhibit a family-resemblance structure, i.e., one like the similarities that exist between relatives (some have the same typical hair color, some have the same typically shaped nose, some have the same typical eyes, but none have all and only the typical family traits); the different uses of a word have several features in common with one or more other uses, but no features are common to all uses. More generally, their semantic structure takes the form of a set of clustered and overlapping meanings (which may be related by similarity or by other associative links, such as metonymy). Because this clustered set is often built up round a central meaning, the term ‘radial set’ is often used for this kind of polysemic structure.
Prototypical categories exhibit degrees of category membership; not every member is equally representative for a category.
Prototypical categories are blurred at the edges.
By way of example, consider fruit as referring to a type of food. If you ask people to list kinds of fruit, some types come to mind more easily than others. For American and European subjects (there is clear cultural variation on this point), oranges, apples, and bananas are the most typical fruits, while pineapples, watermelons, and pomegranates receive low typicality ratings. This illustrates the third characteristic mentioned above. But now, consider coconuts and olives. Is a coconut or an olive a fruit in the ordinary everyday sense of that word? For many people, the answer is not immediately obvious, which illustrates the fourth characteristic: if we zoom in on the least typical exemplars of a category, membership in the category may become fuzzy. A category like fruit should be considered not only with regard to the exemplars that belong to it, but also with regard to the features that these category members share and that together define the category. Types of fruit do not, however, share a single set of definitional features that sufficiently distinguishes fruit from, say, vegetables and other natural foodstuffs. All are edible seed-bearing parts of plants, but most other features that we think of as typical for fruit are not general: while most are sweet, some are not, like lemons; while most are juicy, some are not, like bananas; while most grow on trees and tree-like plants, some grow on bushes, like strawberries; and so on. This absence of a neat definition illustrates the first characteristic. Instead of such a single definition, what seems to hold together the category are overlapping clusters of representative features. Whereas the most typical kinds of fruit are the sweet and juicy ones that grow on trees, other kinds may lack one or even more of these features. This then illustrates the second characteristic mentioned above.
The four characteristics are systematically related along two dimensions. On the one hand, the third and the fourth characteristics take into account the referential, extensional structure of a category. In particular, they consider the members of a category; they observe, respectively, that not all referents of a category are equal in representativeness for that category and that the denotational boundaries of a category are not always determinate. On the other hand, these two aspects (centrality and nonrigidity) recur on the intensional level, where the definitional rather than the referential structure of a category is envisaged. For one thing, nonrigidity shows up in the fact that there is no single necessary and sufficient definition for a prototypical concept. For another, family resemblances imply overlapping of the subsets of a category; consequently, meanings exhibiting a greater degree of overlapping will have more structural weight than meanings that cover only peripheral members of the category. As such, the clustering of meanings that is typical of family resemblances implies that not every meaning is structurally equally important (and a similar observation can be made with regard to the components into which those meanings may be analyzed).
The four characteristics are not coextensive; that is, they do not necessarily occur together. In that sense, some words may exhibit more prototypicality effects than others. In the practice of linguistics, the second feature in particular has attracted the attention, and the radial set model (which graphically represents the way in which less central meanings branch out from the prototypical, core reading) is a popular representational format in lexical semantics; see Tyler and Evans ( 2001 ) for an example.
1.9 Basic Levels and Onomasiological Salience
Possibly the major innovation of the prototype model of categorization is to give salience a place in the description of semasiological structure: next to the qualitative relations among the elements in a semasiological structure (like metaphor and metonymy), a quantifiable center-periphery relationship is introduced as part of the architecture. But the concept of salience can also be applied to the onomasiological domain.
The initial step in the introduction of onomasiological salience is the basic-level hypothesis . The hypothesis is based on the ethnolinguistic observation that folk classifications of biological domains usually conform to a general organizational principle, in the sense that they consist of five or six taxonomical levels (Berlin, 1978 ). The basic-level hypothesis embodies a notion of onomasiological salience, because it is a hypothesis about alternative categorizations of referents: if a particular referent (a particular piece of clothing) can be alternatively categorized as a garment, a skirt, or a wrap-around skirt, the choice will be preferentially made for the basic-level category ‘skirt.’ But differences of onomasiological preference also occur among categories on the same level in a taxonomical hierarchy. If a particular referent can be alternatively categorized as a wrap-around skirt or a miniskirt, there could just as well be a preferential choice: when you encounter something that is both a wrap-around skirt and a miniskirt, the most natural way of naming that referent in a neutral context would probably be ‘miniskirt.’ If, then, we have to reckon with intra-level differences of salience next to inter-level differences, the concept of onomasiological salience has to be generalized in such a way that it relates to individual categories at any level of the hierarchy.
This notion of generalized onomasiological salience was first introduced in Geeraerts, Grondelaers, and Bakema ( 1994 ). Using corpus materials, this study established that the choice for one lexical item rather than the other as the name for a given referent is determined by the semasiological salience of the referent (i.e., the degree of prototypicality of the referent with regard to the semasiological structure of the category), by the overall onomasiological salience of the category represented by the expression, and by contextual features of a classical sociolinguistic and geographical nature, involving the competition between different language varieties. By zooming in on the last type of factor, a further refinement of the notion of onomasiological salience is introduced, in the form the distinction between conceptual and formal onomasiological variation. Whereas conceptual onomasiological variation involves the choice of different conceptual categories for a referent (like the examples presented so far), formal onomasiological variation merely involves the use of different synonymous names for the same conceptual category. The names jeans and trousers for denim leisure-wear trousers constitute an instance of conceptual variation, for they represent categories at different taxonomical levels. Jeans and denims , however, represent no more than different (but synonymous) names for the same denotational category.
2. The Theoretical Evolution of Lexical Semantics
Four broadly defined theoretical traditions may be distinguished in the history of word-meaning research.
2.1 Prestucturalist Historical Semantics
The prestructuralist period (ranging from the middle of the 19th century up to the 1930s) was the heyday of historical semantics, in the sense that the study of meaning change reigned supreme within semantics. The main theoretical achievement of prestructuralist historical semantics consists of various classifications of types of semantic change, coupled with considerable attention to psychological processes as the explanatory background of changes: the general mechanisms of change included in the classifications were generally considered to be based on the associative patterns of thought of the human mind. Important figures (among many others) are Hermann Paul, Michel Bréal, and Gustaf Stern (see Ullmann, 1962 , for an introductory overview). With the shift toward a structuralist approach that occurred round 1930 , lexical semantics switched from a preference for diachronic studies to a preference for synchronic studies. However, the poststructuralist cognitive approach provides a new impetus for historical lexical semantics.
2.2 Structuralist Semantics
Inspired by the Saussurean conception of language, structural semantics originated as a reaction against prestructural historical semantics. The origins of structural semantics are customarily attributed to Trier ( 1931 ), but while Trier’s monograph may indeed be the first major descriptive work in structural semantics, the first theoretical and methodological definition of the new approach is to be found in Weisgerber ( 1927 ), a polemical article that criticized historical linguistics on three points. First and foremost, because the vocabulary of a language is not simply an unstructured set of separate items, and because the meaning of a linguistic sign is determined by its position in the linguistic structures in which it takes part, the proper subject matter of semantics is not the atomistic changes of word meanings that historical semantics had concentrated on, but the semantic structure of the language that demarcates the meanings of individual words with regard to each other. Second, because that structure is a linguistic rather than a psychological phenomenon, linguistic meanings should not be studied from a psychological perspective, but from a purely linguistic one. And third, because semantic change has to be redefined as change in semantic structures, synchronic semantics methodologically precedes diachronic semantics: the synchronic structures have to be studied before their changes can be considered. The realization of this attempt to develop a synchronic, nonpsychological, structural theory of semantics depends on the way in which the notion of semantic structure is conceived. In actual practice, there are mainly three distinct definitions of semantic structure that have been employed by structuralist semanticians. More particularly, three distinct kinds of structural relations among lexical items have been singled out as the proper methodological basis of lexical semantics. First, there is the relationship of semantic similarity that lies at the basis of semantic field analysis and componential analysis: see section 1.3, “Lexical Fields and Componential Analysis.” Second, there are unanalyzed lexical relations such as synonymy, antonymy, and hyponymy: see section 1.4, “Lexical Relations.” Third, syntagmatic lexical relations lie at the basis of a distributional approach to semantics: see section 1.5, “Distributional Relations.”
2.3 Neostructuralist Semantics
While componential analysis was developed in the second half of the 1950s and the beginning of the 1960s by European as well as American structural linguists, its major impact came from its incorporation into generative grammar: the publication of Katz and Fodor ( 1963 ) marked a theoretical migration of lexical semantics from a structuralist to a generativist framework. As a model for lexical semantics, Katzian semantics combined an essentially structuralist approach with two novel characteristics: the explicit inclusion of lexical description in a generative grammar and, accordingly (given that the grammar is a formal one), an interest in the formalization of lexical descriptions. Although Katzian semantics as such has long been abandoned, both features continue to play a role in this ‘neostructuralist’ tradition (the label is not an established one, but it will do for lack of a more conventional one). On the one hand, the integration of the lexicon into the grammar informs the continuing debate about the interface of lexicon and syntax; see Wechsler ( 2015 ) for an overview. On the other hand, a number of models for the formalization of word meaning have been developed, the most prominent of which is Pustejovsky’s ‘generative lexicon’ approach ( 1995 ).
2.4 Cognitive Semantics
Compared to prestructuralist semantics, structuralism constitutes a move toward a more purely ‘linguistic’ type of lexical semantics, focusing on the linguistic system rather than the psychological background or the contextual flexibility of meaning. With the poststructuralist emergence of cognitive semantics, the pendulum swings back to a position in which the distinction between semantics and pragmatics is not a major issue, in which language is seen in the context of cognition at large, and in which language use is as much a focus of enquiry as the language system. Cognitive lexical semantics emerged in the 1980s as part of cognitive linguistics, a loosely structured theoretical movement that opposed the autonomy of grammar and the marginal position of semantics in the generativist theory of language. Important contributions to lexical semantics include prototype theory (see section 1.8, “Prototype Effects and Radial Sets” ), conceptual metaphor theory (see section 1.6, “Conceptual Metaphor and Metonymy” ), frame semantics (see section 1.8), and the emergence of usage-based onomasiology (see section 1.9, “Basic Levels and Onomasiological Salience” ).
From a theoretical perspective, the various traditions are to some extent at odds with each other (as may be expected). Specifically, structuralist (and to a large extent neostructuralist) theories tend to look at word meaning primarily as a property of the language, that is the linguistic system as an entity in its own right. Prestructuralist historical semantics and cognitive semantics, on the other hand, tend to emphasize the way in which word meanings are embedded in or interact with phenomena that lie outside language in a narrow sense, like general cognitive principles, or the cultural, social, historical experience of the language user. They then also take a more ‘pragmatic’ perspective: if the emphasis moves away from the linguistic system as a more or less stable, more or less autonomous repository of possibilities, there will be more attention to language use as the actualization of those possibilities.
Descriptively speaking, however, each of the major theoretical frameworks has contributed to the expansion of lexical semantics, that is they have drawn attention to specific phenomena and they have proposed terms, classifications, and representational formats for analyzing those phenomena. Focusing on the major topics, these contributions successively include the links between the various senses of words in prestructuralist historical semantics, the semantic relationships within the vocabulary in the structuralist era, and the importance of semasiological and onomasiological salience effects in cognitive semantics. Regardless of the theoretical oppositions, these phenomena all belong to the descriptive scope of current lexical semantics: the emergence of new points of attention has not made the older topics irrelevant.
Table 2 The Contribution of the Successive Theoretical Traditions
semasiology | onomasiology | |
---|---|---|
‘qualitative’ perspectives: elements and relations | Prestructuralist historical semantics | |
‘quantitative’ perspectives: differences of salience | Cognitive semantics | Cognitive semantics |
A summary of the contribution of the major theoretical approaches is given in Table 2 . If one keeps in mind the chronology of the various theories, it will be clear that regardless of the theoretical differences, lexical semantics has witnessed an outspoken descriptive expansion, from a semasiological starting point to various forms of onomasiological structure, and from a focus on elements and structures alone to the relevance of salience effects on the semasiological and onomasiological architecture of meaning.
Further Reading
- Goddard, C. (1998). Semantic analysis: A practical introduction . Oxford: Oxford University Press.
- Riemer, N. (2015). Word meanings. In J. R. Taylor (Ed.), The Oxford handbook of the word (pp. 315–319). Oxford: Oxford University Press.
- Benczes, R. , Barcelona, A. , & Ruiz de Mendoza Ibáñez, F. (Eds.). (2011). Defining metonymy in cognitive linguistics: Towards a consensus view . Amsterdam: John Benjamins.
- Berlin, B. (1978). Ethnobiological classification. In E. Rosch & B. B. Lloyd (Eds.), Cognition and categorization (pp. 9–26). Hillsdale, NJ: Lawrence Erlbaum.
- Cruse, D. A. (1986). Lexical semantics . Cambridge, U.K.: Cambridge University Press.
- Fillmore, C. J. , & Atkins, B. T. S. (1992). Toward a frame-based lexicon: The semantics of ‘risk’ and its neighbors. In A. Lehrer & E. F. Kittay (Eds.), Frames, fields and contrasts: New essays in semantic and lexical organization (pp. 75–102). Hillsdale, NJ: Lawrence Erlbaum.
- Firth, J. R. (1957). Papers in linguistics, 1934–51 . Oxford: Oxford University Press.
- Geeraerts, D. (1993). Vagueness’s puzzles, polysemy’s vagaries. Cognitive Linguistics , 4 , 223–272.
- Geeraerts, D. (2010). Theories of lexical semantics. Oxford: Oxford University Press.
- Geeraerts, D. , Grondelaers, S. , & Bakema, P. (1994). The structure of lexical variation: Meaning, naming, and context . Berlin: Mouton de Gruyter.
- Glynn, D. , & Robinson, J. A. (Eds.). (2014). Corpus methods for semantics: Quantitative studies in polysemy and synonymy . Amsterdam: John Benjamins.
- Hanks, P. W. (2013). Lexical analysis: Norms and exploitations . Cambridge, MA: MIT Press.
- Katz, J. J. , & Fodor, J. A. (1963). The structure of a semantic theory. Language , 39 , 170–210.
- Lakoff, G. , & Johnson, M. (1980). Metaphors we live by . Chicago: University of Chicago Press.
- Lakoff, G. , & Johnson, M. (1999). Philosophy in the flesh: The embodied mind and its challenges to western thought . Chicago: University of Chicago Press.
- Lyons, J. (1963). Structural semantics . Oxford: Blackwell.
- Murphy, M. L. (2003). Semantic relations and the lexicon: Antonymy, synonymy, and other paradigms . Cambridge, U.K.: Cambridge University Press.
- Murphy, M. L. (2010). Lexical meaning . Cambridge, U.K.: Cambridge University Press.
- Pottier, B. (1964). Vers une sémantique moderne. Travaux de linguistique et de littérature , 2 , 107–137.
- Pustejovsky, J. (1995). The generative lexicon. Cambridge, MA: MIT Press.
- Rosch, E. (1975). Cognitive representations of semantic categories. Journal of Experimental Psychology , 104 , 192–233.
- Ruppenhofer, J. , Ellsworth, M. , Petruck, M. R. L. , Johnson, C. R. , & Scheffczyk, J. (2006). FrameNet II: Extended theory and practice . Berkeley, CA: FrameNet.
- Sinclair, J. M. (1991). Corpus, concordance, collocation . Oxford: Oxford University Press.
- Taylor, J. R. (2003). Linguistic categorization . 3d ed. Oxford: Oxford University Press.
- Trier, J. (1931). Der deutsche Wortschatz im Sinnbezirk des Verstandes: Die Geschichte eines sprachlichen Feldes I. Von den Anfängen bis zum Beginn des 13. Jhdts. Heidelberg: Winter.
- Tyler, A. , & Evans, V. (2001). Reconsidering prepositional polysemy networks: the case of ‘over.’ Language , 77 , 724–765.
- Ullmann, S. (1962). Semantics: An introduction to the science of meaning . Oxford: Blackwell.
- Wechsler, S. (2015). Word meaning and syntax: Approaches to the interface . Oxford: Oxford University Press.
- Weisgerber, L. (1927). Die Bedeutungslehre: Ein Irrweg der Sprachwissenschaft? Germanisch-Romanische Monatsschrift , 15 , 161–183.
- Wierzbicka, A. (1996). Semantics: Primes and universals . Oxford: Oxford University Press.
Related Articles
- Middle English
- Chinese Semantics
- Phonological Templates in Development
- Argument Realization in Syntax
- Lexical Semantic Framework for Morphology
- Cognitively Oriented Theories of Meaning
- Acquisition of Pragmatics
- Type Theory for Natural Language Semantics
- Conversational Implicature
- Nominal Reference
- The Onomasiological Approach
- Nominalization: General Overview and Theoretical Issues
- Artificial Languages
Printed from Oxford Research Encyclopedias, Linguistics. Under the terms of the licence agreement, an individual user may print out a single article for personal use (for details see Privacy Policy and Legal Notice).
date: 02 September 2024
- Cookie Policy
- Privacy Policy
- Legal Notice
- [81.177.182.154]
- 81.177.182.154
Character limit 500 /500
This week: the arXiv Accessibility Forum
Help | Advanced Search
Computer Science > Computer Vision and Pattern Recognition
Title: semantic image segmentation: two decades of research.
Abstract: Semantic image segmentation (SiS) plays a fundamental role in a broad variety of computer vision applications, providing key information for the global understanding of an image. This survey is an effort to summarize two decades of research in the field of SiS, where we propose a literature review of solutions starting from early historical methods followed by an overview of more recent deep learning methods including the latest trend of using transformers. We complement the review by discussing particular cases of the weak supervision and side machine learning techniques that can be used to improve the semantic segmentation such as curriculum, incremental or self-supervised learning. State-of-the-art SiS models rely on a large amount of annotated samples, which are more expensive to obtain than labels for tasks such as image classification. Since unlabeled data is instead significantly cheaper to obtain, it is not surprising that Unsupervised Domain Adaptation (UDA) reached a broad success within the semantic segmentation community. Therefore, a second core contribution of this book is to summarize five years of a rapidly growing field, Domain Adaptation for Semantic Image Segmentation (DASiS) which embraces the importance of semantic segmentation itself and a critical need of adapting segmentation models to new environments. In addition to providing a comprehensive survey on DASiS techniques, we unveil also newer trends such as multi-domain learning, domain generalization, domain incremental learning, test-time adaptation and source-free domain adaptation. Finally, we conclude this survey by describing datasets and benchmarks most widely used in SiS and DASiS and briefly discuss related tasks such as instance and panoptic image segmentation, as well as applications such as medical image segmentation.
Comments: | Pre-print of the book: G. Csurka, R. Volpi and B. Chidlovski: Semantic Image Segmentation: Two Decades of Research, FTCGV (14): No. 1-2, . The authors retained the copyright and are allowed to post it on arXiv. Research only use, commercial use or systematic downloading (by robots or other automatic processes) is prohibited |
Subjects: | Computer Vision and Pattern Recognition (cs.CV) |
Cite as: | [cs.CV] |
(or [cs.CV] for this version) | |
Focus to learn more arXiv-issued DOI via DataCite |
Submission history
Access paper:.
- Other Formats
References & Citations
- Google Scholar
- Semantic Scholar
BibTeX formatted citation

Bibliographic and Citation Tools
Code, data and media associated with this article, recommenders and search tools.
- Institution
arXivLabs: experimental projects with community collaborators
arXivLabs is a framework that allows collaborators to develop and share new arXiv features directly on our website.
Both individuals and organizations that work with arXivLabs have embraced and accepted our values of openness, community, excellence, and user data privacy. arXiv is committed to these values and only works with partners that adhere to them.
Have an idea for a project that will add value for arXiv's community? Learn more about arXivLabs .
Our Product
Experience a Smarter Way to Search and Discover Research
Scholars like you make impactful, groundbreaking research work every single day around the world. We are here to support you by providing a better way to search and discover scientific knowledge.

Find Relevant Research
Search over 214 million papers from all fields of science, with filters such as journals and conferences, authors, publication types, and date range.
Scan Papers Faster with TLDRs

Identifying the right papers for you can be time-consuming. To save some time, look for TLDRs (Too Long; Didn't Read) on search results pages.
TLDRs are super-short summaries of the main objective and results of a paper, generated using expert background knowledge and NLP techniques, available for nearly 60 million papers in computer science, biology, and medicine.
Check Highly Influential Citations

Too many citations to wade through? Start with the Highly Influential Citations where the cited publication has a significant impact on the citing publication, determined by a machine-learning model that analyzes factors including the number of citations to a publication and the surrounding context for each.
Cite Any Paper
Any paper you find relevant for your research, select "Cite" on a paper page or in the search results.
A pop-up will offer you the option of multiple citation formats including BibTex, MLA, APA, or Chicago.
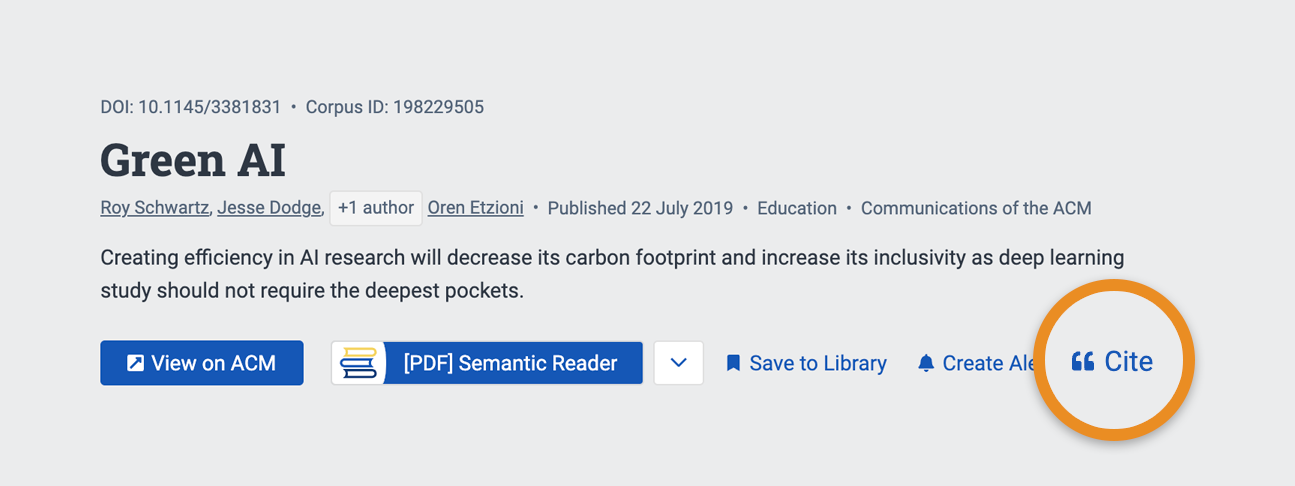
Manage Your Papers in One Place
Store and organize all the papers that interest you in your online library and access them anywhere, anytime when you sign in to Semantic Scholar.
With your library, you can organize papers into customized folders and bulk export citations. You can also create public folders and easily share them with collaborators, who can copy the folder to their own library in one click.
After creating a folder, you can also create AI-powered Research Feeds that automatically generate paper recommendations for you. Simply toggle the Research Feed to ‘On’ for each folder and you will start receiving regular recommendations from the next day.
Pro Tip: Place similar papers into one folder to generate better and more relevant recommendations.
Research Feeds
Personalized ai-powered paper recommendations.
After you add papers to your library, your Research Feeds will quickly learn what papers interest you and recommend the latest research to help you stay up-to-date.
You can turn on Research Feeds recommendations for any folder in your library and have them sent right to your email. To learn more about how Research Feeds work, visit our FAQ .
Tips to Get Better Recommendations
Ratings are essential to teach Semantic Scholar’s AI what is or isn't relevant to you. The more ratings you submit, the more relevant your recommended papers will be.
Add 5 relevant papers to a library folder. Semantic Scholar will show you papers similar to these.
Mark 3 non-relevant papers as "not relevant" in the corresponding Research Feed, and Semantic Scholar will show you fewer papers similar to these.
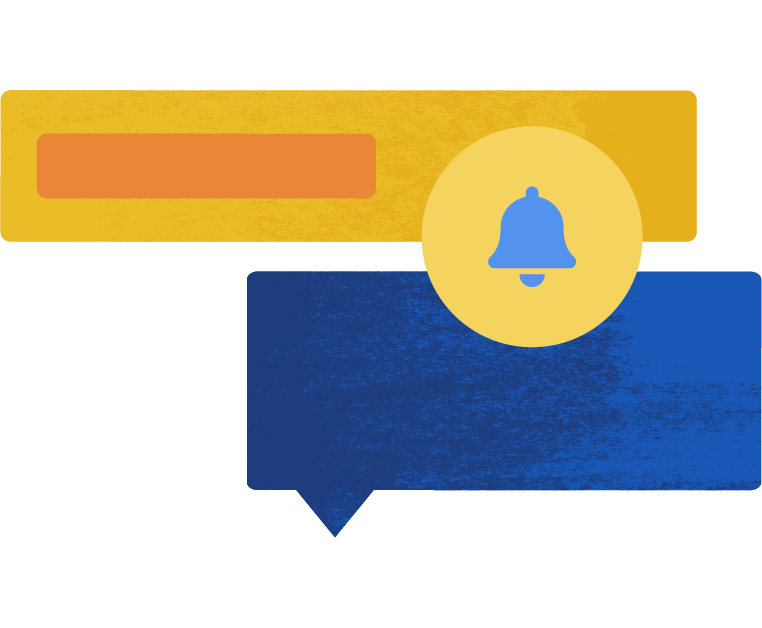
Get Notified for New Papers or Citations
No need to refresh the page every day. You can set up and customize automated email alerts to stay up-to-date with new citations and new papers.
Paper Alerts
Get notified when there are new citations for a specific paper. To create a paper alert, select “Create Alert” on any paper page.
Author Alerts
Get notified when there are new citations and papers for a specific author. To create an author alert, select “Follow Author” on any author page. Or, claim your author page to get notified about new papers citing your works.
Research Feed Alerts
Get new paper recommendations in your email when you turn on Research Feeds alerts for any of your library folders.
Research Dashboard
Check all your research updates.
When signed in to your account, your Research Dashboard serves as your Semantic Scholar homepage where you can view the latest recommended papers from your Research Feeds and new papers from your alerts.
If you have claimed your author page , you can also view the most recent citations for your papers and quick links to your author page and editing options.
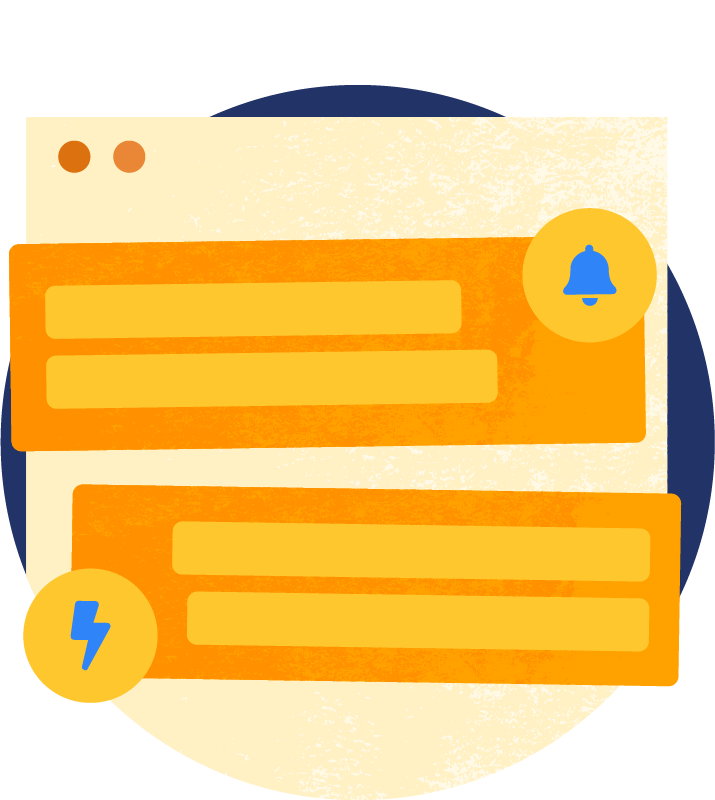
Explore Topics with AI
Topic pages aim to help you explore topics of interest by collecting AI-generated definitions, papers often cited for the topic, recent papers, and related topics.
In the Topics section on each paper page, you can find topics relevant to the paper and navigate to the topic pages. You can also directly search for a topic in the search bar. Currently, only topics in the Computer Science field are available.
Topics Examples
- SPECTER: Document-level Representation Learning using Citation-informed Transformers
- TriviaQA: A Large Scale Distantly Supervised Challenge Dataset for Reading Comprehension
- Longformer: The Long-Document Transformer
Ask This Paper
Understand a paper with ai.
Get AI-generated answers to your questions along with supporting statements from the paper.
In the Ask This Paper section on each paper page, select suggested questions or enter your own questions about the paper. You can instantly get an answer along with one or more supporting statements from the paper. Currently, Ask This Paper has been tested only on English-language papers and is available on limited papers.
Ask This Paper Examples
- BERT: Pre-training of Deep Bidirectional Transformers for Language Understanding
- CiteSee: Augmenting Citations in Scientific Papers with Persistent and Personalized Historical Context
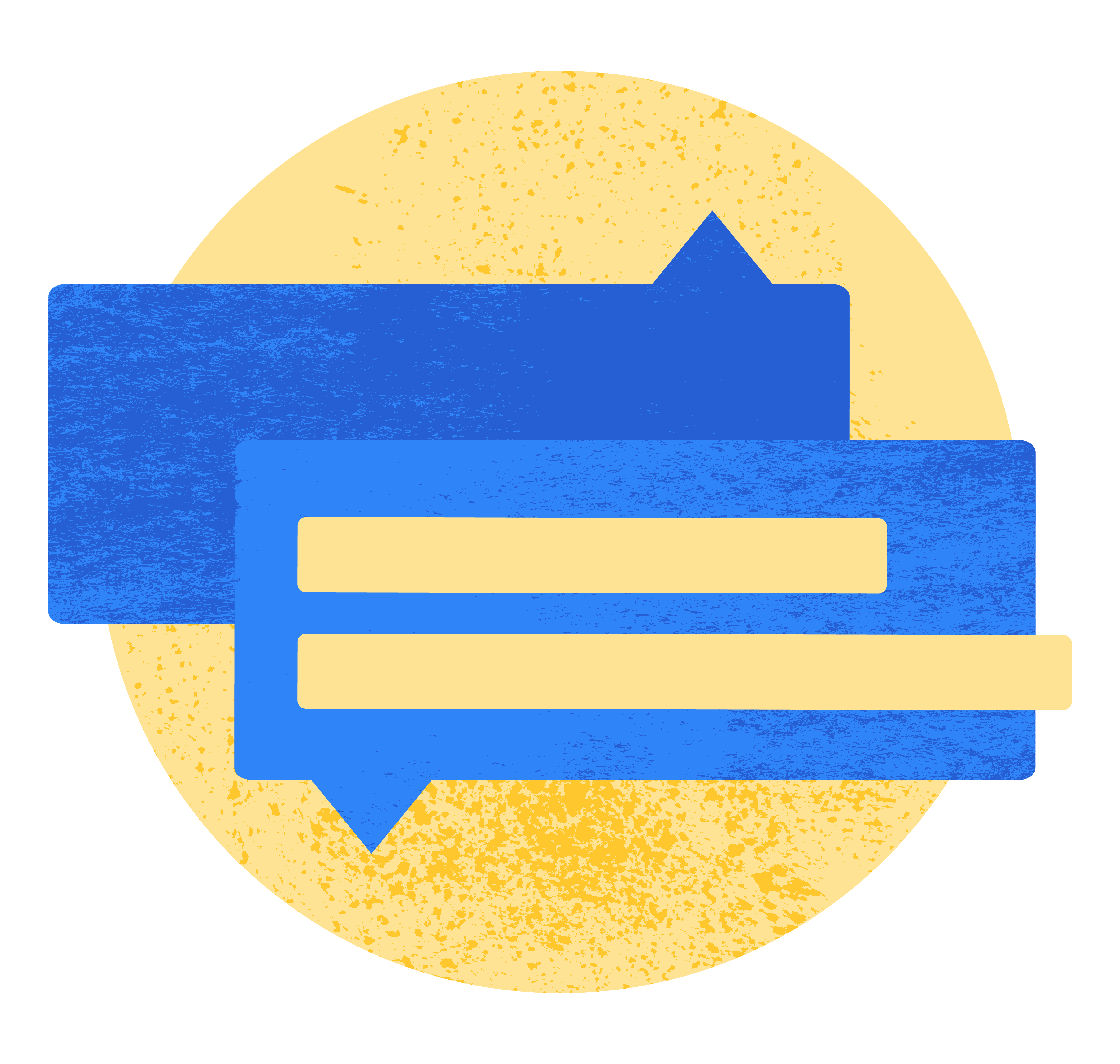
Try Other Exciting Research Tools
Semantic reader.
An augmented reading application that makes the reading experience more accessible and richly contextual by providing citation information directly within the context of a paper.
Semantic Scholar Academic Graph API
A reliable on-demand source of data for the global research community about authors, papers, citations, venues, and more that allows you to link directly to the corresponding page on Semantic Scholar.
Semantic Scholar Open Research Corpus (S2ORC)
A general-purpose corpus with 8.1M open access papers spanning many academic disciplines, which includes rich metadata, paper abstracts, resolved bibliographic references, as well as structured full text.
Hear what our users say...
On my lab website, I link to my Semantic Scholar author page (as opposed to Google or Pubmed) because the TLDR and influential citations encourage understanding and impact, rather than just blindly counting up articles. As it becomes more reliable, I fully expect these author pages to serve as a richer "contributor dashboard" that is more informative than a CV or other current attribution mechanisms.
Matthew Akamatsu Semantic Scholar user
Jordi Chanovas Semantic Scholar user
Research feeds helped me maintain up-to-date with the latest findings related with my research. Email alerts kept me aware of new articles in my research feed. Finally, TLDR uses AI to give a summarised version of publications which makes the decision of whether a publication is relevant or not to the field easier than ever.
Through the influential citations, I can know the follow-up progress of this work and follow up the latest progress of this research. Research feeds is very useful for me to understand the relevant literature in the field and broaden my horizons. TLDR allows me to quickly understand the content of this paper and saves my time and energy.
Mountain Semantic Scholar user
Mark Friedman Semantic Scholar user
Semantic Scholar appears to be very focused on the needs of people like me that want to be able to keep up with current research on specific topics (as defined by our explicit and implicit selections).
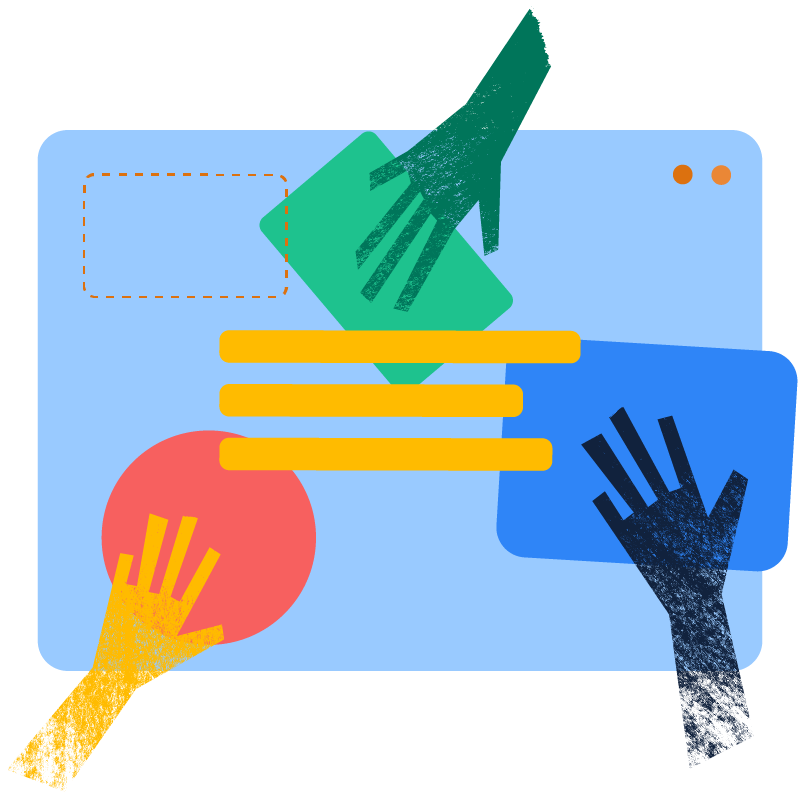
Interested in upcoming new features?
Join our Beta Program to provide feedback and help scholars around the globe.
Experience a smarter way to search and discover scholarly research.
Latest news & updates.
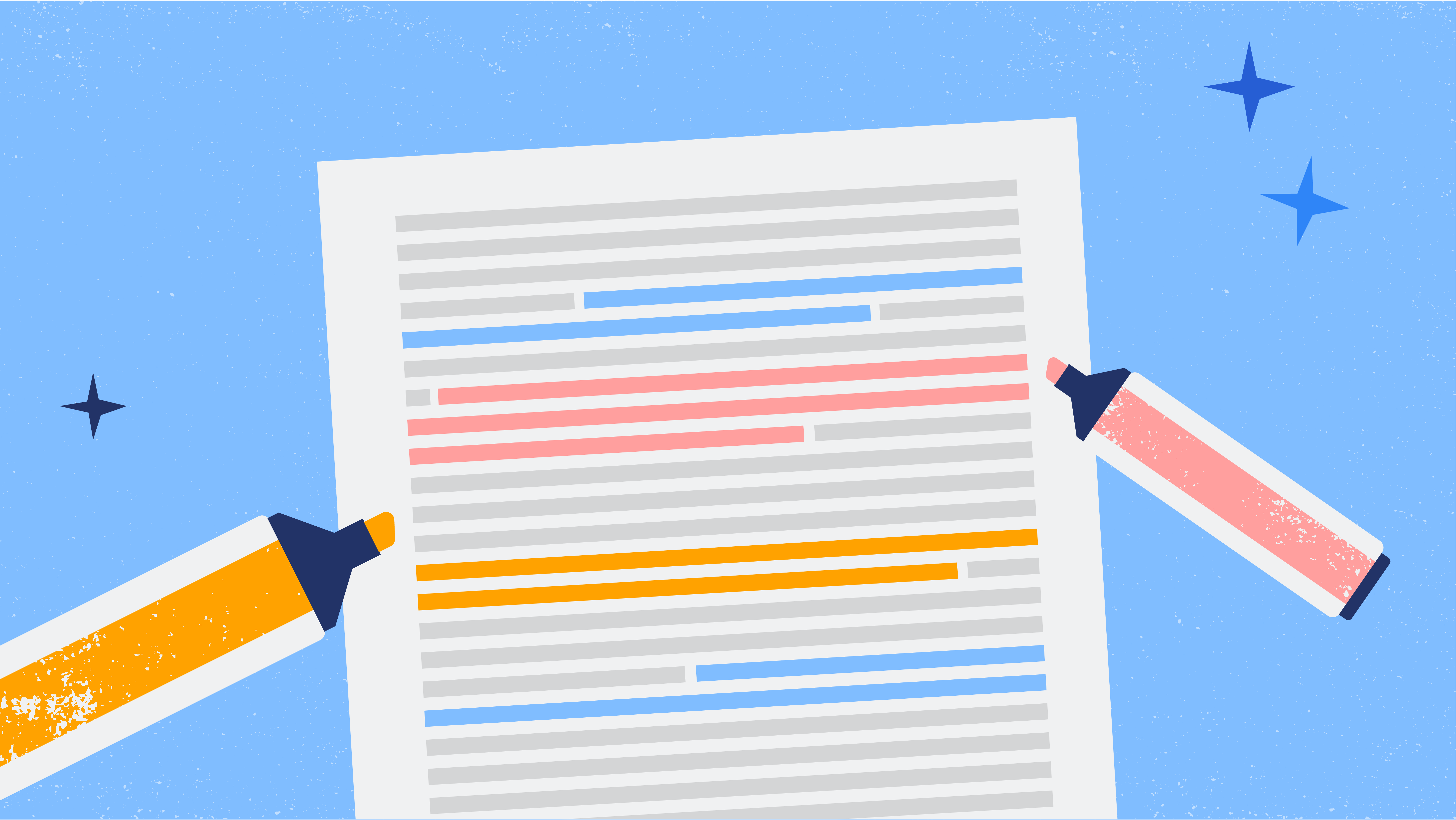
Case Study: Iterative Design for Skimming Support
How might we help researchers quickly assess the relevance of scientific literature? Take a closer look at Skimming, Semantic Reader’s latest AI feature, and the collaborative design process behind it.

Behind the Scenes of Semantic Scholar’s New Author Influence Design
We released a new version of Author Influence interface to help scholars better discover other scholars in their fields. Here's how we identified user insights and made those design choices.
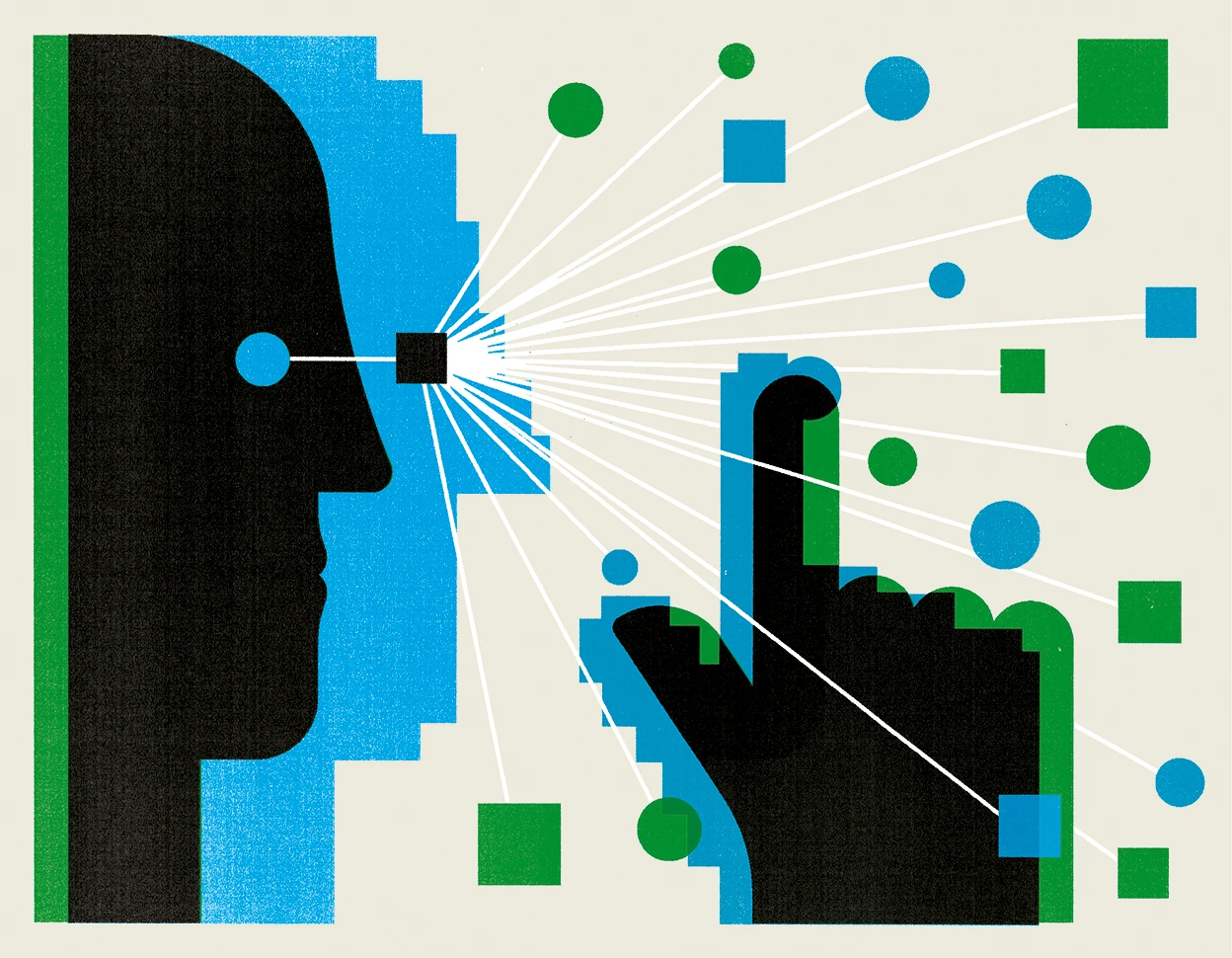
Artificial-intelligence search engines wrangle academic literature
Nature had a chat with Dan Weld, Chief Scientist at Semantic Scholar, to discuss how search engines are helping scientists explore and innovate by making it easier to draw connections from a massive collection of scientific literature.
Stay Connected With Semantic Scholar
Thank you for visiting nature.com. You are using a browser version with limited support for CSS. To obtain the best experience, we recommend you use a more up to date browser (or turn off compatibility mode in Internet Explorer). In the meantime, to ensure continued support, we are displaying the site without styles and JavaScript.
- View all journals
- Explore content
- About the journal
- Publish with us
- Sign up for alerts
- Open access
- Published: 31 August 2024
Knowledge mapping and evolution of research on older adults’ technology acceptance: a bibliometric study from 2013 to 2023
- Xianru Shang ORCID: orcid.org/0009-0000-8906-3216 1 ,
- Zijian Liu 1 ,
- Chen Gong 1 ,
- Zhigang Hu 1 ,
- Yuexuan Wu 1 &
- Chengliang Wang ORCID: orcid.org/0000-0003-2208-3508 2
Humanities and Social Sciences Communications volume 11 , Article number: 1115 ( 2024 ) Cite this article
Metrics details
- Science, technology and society
The rapid expansion of information technology and the intensification of population aging are two prominent features of contemporary societal development. Investigating older adults’ acceptance and use of technology is key to facilitating their integration into an information-driven society. Given this context, the technology acceptance of older adults has emerged as a prioritized research topic, attracting widespread attention in the academic community. However, existing research remains fragmented and lacks a systematic framework. To address this gap, we employed bibliometric methods, utilizing the Web of Science Core Collection to conduct a comprehensive review of literature on older adults’ technology acceptance from 2013 to 2023. Utilizing VOSviewer and CiteSpace for data assessment and visualization, we created knowledge mappings of research on older adults’ technology acceptance. Our study employed multidimensional methods such as co-occurrence analysis, clustering, and burst analysis to: (1) reveal research dynamics, key journals, and domains in this field; (2) identify leading countries, their collaborative networks, and core research institutions and authors; (3) recognize the foundational knowledge system centered on theoretical model deepening, emerging technology applications, and research methods and evaluation, uncovering seminal literature and observing a shift from early theoretical and influential factor analyses to empirical studies focusing on individual factors and emerging technologies; (4) moreover, current research hotspots are primarily in the areas of factors influencing technology adoption, human-robot interaction experiences, mobile health management, and aging-in-place technology, highlighting the evolutionary context and quality distribution of research themes. Finally, we recommend that future research should deeply explore improvements in theoretical models, long-term usage, and user experience evaluation. Overall, this study presents a clear framework of existing research in the field of older adults’ technology acceptance, providing an important reference for future theoretical exploration and innovative applications.
Similar content being viewed by others
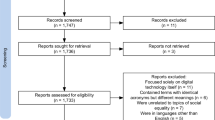
Research progress and intellectual structure of design for digital equity (DDE): A bibliometric analysis based on citespace
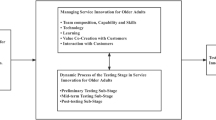
Exploring the role of interaction in older-adult service innovation: insights from the testing stage
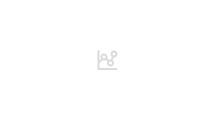
Smart device interest, perceived usefulness, and preferences in rural Alabama seniors
Introduction.
In contemporary society, the rapid development of information technology has been intricately intertwined with the intensifying trend of population aging. According to the latest United Nations forecast, by 2050, the global population aged 65 and above is expected to reach 1.6 billion, representing about 16% of the total global population (UN 2023 ). Given the significant challenges of global aging, there is increasing evidence that emerging technologies have significant potential to maintain health and independence for older adults in their home and healthcare environments (Barnard et al. 2013 ; Soar 2010 ; Vancea and Solé-Casals 2016 ). This includes, but is not limited to, enhancing residential safety with smart home technologies (Touqeer et al. 2021 ; Wang et al. 2022 ), improving living independence through wearable technologies (Perez et al. 2023 ), and increasing medical accessibility via telehealth services (Kruse et al. 2020 ). Technological innovations are redefining the lifestyles of older adults, encouraging a shift from passive to active participation (González et al. 2012 ; Mostaghel 2016 ). Nevertheless, the effective application and dissemination of technology still depends on user acceptance and usage intentions (Naseri et al. 2023 ; Wang et al. 2023a ; Xia et al. 2024 ; Yu et al. 2023 ). Particularly, older adults face numerous challenges in accepting and using new technologies. These challenges include not only physical and cognitive limitations but also a lack of technological experience, along with the influences of social and economic factors (Valk et al. 2018 ; Wilson et al. 2021 ).
User acceptance of technology is a significant focus within information systems (IS) research (Dai et al. 2024 ), with several models developed to explain and predict user behavior towards technology usage, including the Technology Acceptance Model (TAM) (Davis 1989 ), TAM2, TAM3, and the Unified Theory of Acceptance and Use of Technology (UTAUT) (Venkatesh et al. 2003 ). Older adults, as a group with unique needs, exhibit different behavioral patterns during technology acceptance than other user groups, and these uniquenesses include changes in cognitive abilities, as well as motivations, attitudes, and perceptions of the use of new technologies (Chen and Chan 2011 ). The continual expansion of technology introduces considerable challenges for older adults, rendering the understanding of their technology acceptance a research priority. Thus, conducting in-depth research into older adults’ acceptance of technology is critically important for enhancing their integration into the information society and improving their quality of life through technological advancements.
Reviewing relevant literature to identify research gaps helps further solidify the theoretical foundation of the research topic. However, many existing literature reviews primarily focus on the factors influencing older adults’ acceptance or intentions to use technology. For instance, Ma et al. ( 2021 ) conducted a comprehensive analysis of the determinants of older adults’ behavioral intentions to use technology; Liu et al. ( 2022 ) categorized key variables in studies of older adults’ technology acceptance, noting a shift in focus towards social and emotional factors; Yap et al. ( 2022 ) identified seven categories of antecedents affecting older adults’ use of technology from an analysis of 26 articles, including technological, psychological, social, personal, cost, behavioral, and environmental factors; Schroeder et al. ( 2023 ) extracted 119 influencing factors from 59 articles and further categorized these into six themes covering demographics, health status, and emotional awareness. Additionally, some studies focus on the application of specific technologies, such as Ferguson et al. ( 2021 ), who explored barriers and facilitators to older adults using wearable devices for heart monitoring, and He et al. ( 2022 ) and Baer et al. ( 2022 ), who each conducted in-depth investigations into the acceptance of social assistive robots and mobile nutrition and fitness apps, respectively. In summary, current literature reviews on older adults’ technology acceptance exhibit certain limitations. Due to the interdisciplinary nature and complex knowledge structure of this field, traditional literature reviews often rely on qualitative analysis, based on literature analysis and periodic summaries, which lack sufficient objectivity and comprehensiveness. Additionally, systematic research is relatively limited, lacking a macroscopic description of the research trajectory from a holistic perspective. Over the past decade, research on older adults’ technology acceptance has experienced rapid growth, with a significant increase in literature, necessitating the adoption of new methods to review and examine the developmental trends in this field (Chen 2006 ; Van Eck and Waltman 2010 ). Bibliometric analysis, as an effective quantitative research method, analyzes published literature through visualization, offering a viable approach to extracting patterns and insights from a large volume of papers, and has been widely applied in numerous scientific research fields (Achuthan et al. 2023 ; Liu and Duffy 2023 ). Therefore, this study will employ bibliometric methods to systematically analyze research articles related to older adults’ technology acceptance published in the Web of Science Core Collection from 2013 to 2023, aiming to understand the core issues and evolutionary trends in the field, and to provide valuable references for future related research. Specifically, this study aims to explore and answer the following questions:
RQ1: What are the research dynamics in the field of older adults’ technology acceptance over the past decade? What are the main academic journals and fields that publish studies related to older adults’ technology acceptance?
RQ2: How is the productivity in older adults’ technology acceptance research distributed among countries, institutions, and authors?
RQ3: What are the knowledge base and seminal literature in older adults’ technology acceptance research? How has the research theme progressed?
RQ4: What are the current hot topics and their evolutionary trajectories in older adults’ technology acceptance research? How is the quality of research distributed?
Methodology and materials
Research method.
In recent years, bibliometrics has become one of the crucial methods for analyzing literature reviews and is widely used in disciplinary and industrial intelligence analysis (Jing et al. 2023 ; Lin and Yu 2024a ; Wang et al. 2024a ; Xu et al. 2021 ). Bibliometric software facilitates the visualization analysis of extensive literature data, intuitively displaying the network relationships and evolutionary processes between knowledge units, and revealing the underlying knowledge structure and potential information (Chen et al. 2024 ; López-Robles et al. 2018 ; Wang et al. 2024c ). This method provides new insights into the current status and trends of specific research areas, along with quantitative evidence, thereby enhancing the objectivity and scientific validity of the research conclusions (Chen et al. 2023 ; Geng et al. 2024 ). VOSviewer and CiteSpace are two widely used bibliometric software tools in academia (Pan et al. 2018 ), recognized for their robust functionalities based on the JAVA platform. Although each has its unique features, combining these two software tools effectively constructs mapping relationships between literature knowledge units and clearly displays the macrostructure of the knowledge domains. Particularly, VOSviewer, with its excellent graphical representation capabilities, serves as an ideal tool for handling large datasets and precisely identifying the focal points and hotspots of research topics. Therefore, this study utilizes VOSviewer (version 1.6.19) and CiteSpace (version 6.1.R6), combined with in-depth literature analysis, to comprehensively examine and interpret the research theme of older adults’ technology acceptance through an integrated application of quantitative and qualitative methods.
Data source
Web of Science is a comprehensively recognized database in academia, featuring literature that has undergone rigorous peer review and editorial scrutiny (Lin and Yu 2024b ; Mongeon and Paul-Hus 2016 ; Pranckutė 2021 ). This study utilizes the Web of Science Core Collection as its data source, specifically including three major citation indices: Science Citation Index Expanded (SCIE), Social Sciences Citation Index (SSCI), and Arts & Humanities Citation Index (A&HCI). These indices encompass high-quality research literature in the fields of science, social sciences, and arts and humanities, ensuring the comprehensiveness and reliability of the data. We combined “older adults” with “technology acceptance” through thematic search, with the specific search strategy being: TS = (elder OR elderly OR aging OR ageing OR senile OR senior OR old people OR “older adult*”) AND TS = (“technology acceptance” OR “user acceptance” OR “consumer acceptance”). The time span of literature search is from 2013 to 2023, with the types limited to “Article” and “Review” and the language to “English”. Additionally, the search was completed by October 27, 2023, to avoid data discrepancies caused by database updates. The initial search yielded 764 journal articles. Given that searches often retrieve articles that are superficially relevant but actually non-compliant, manual screening post-search was essential to ensure the relevance of the literature (Chen et al. 2024 ). Through manual screening, articles significantly deviating from the research theme were eliminated and rigorously reviewed. Ultimately, this study obtained 500 valid sample articles from the Web of Science Core Collection. The complete PRISMA screening process is illustrated in Fig. 1 .
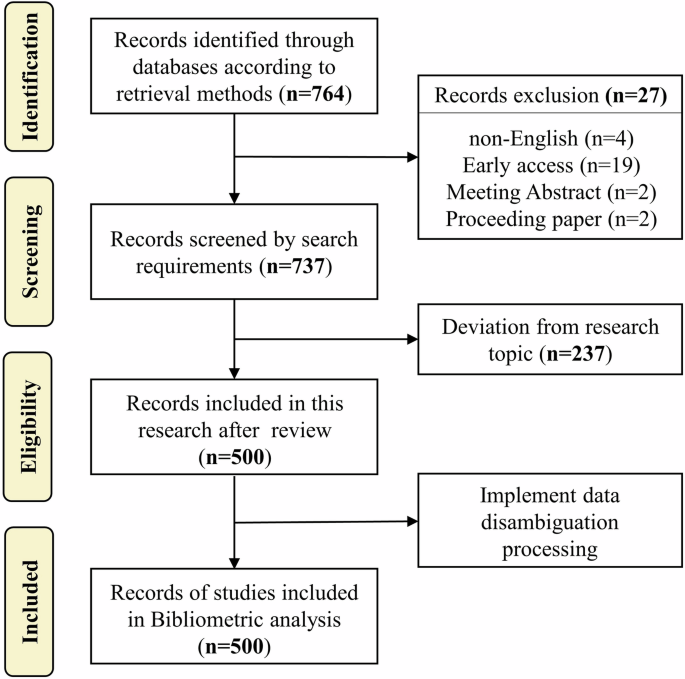
Presentation of the data culling process in detail.
Data standardization
Raw data exported from databases often contain multiple expressions of the same terminology (Nguyen and Hallinger 2020 ). To ensure the accuracy and consistency of data, it is necessary to standardize the raw data (Strotmann and Zhao 2012 ). This study follows the data standardization process proposed by Taskin and Al ( 2019 ), mainly executing the following operations:
(1) Standardization of author and institution names is conducted to address different name expressions for the same author. For instance, “Chan, Alan Hoi Shou” and “Chan, Alan H. S.” are considered the same author, and distinct authors with the same name are differentiated by adding identifiers. Diverse forms of institutional names are unified to address variations caused by name changes or abbreviations, such as standardizing “FRANKFURT UNIV APPL SCI” and “Frankfurt University of Applied Sciences,” as well as “Chinese University of Hong Kong” and “University of Hong Kong” to consistent names.
(2) Different expressions of journal names are unified. For example, “International Journal of Human-Computer Interaction” and “Int J Hum Comput Interact” are standardized to a single name. This ensures consistency in journal names and prevents misclassification of literature due to differing journal names. Additionally, it involves checking if the journals have undergone name changes in the past decade to prevent any impact on the analysis due to such changes.
(3) Keywords data are cleansed by removing words that do not directly pertain to specific research content (e.g., people, review), merging synonyms (e.g., “UX” and “User Experience,” “aging-in-place” and “aging in place”), and standardizing plural forms of keywords (e.g., “assistive technologies” and “assistive technology,” “social robots” and “social robot”). This reduces redundant information in knowledge mapping.
Bibliometric results and analysis
Distribution power (rq1), literature descriptive statistical analysis.
Table 1 presents a detailed descriptive statistical overview of the literature in the field of older adults’ technology acceptance. After deduplication using the CiteSpace software, this study confirmed a valid sample size of 500 articles. Authored by 1839 researchers, the documents encompass 792 research institutions across 54 countries and are published in 217 different academic journals. As of the search cutoff date, these articles have accumulated 13,829 citations, with an annual average of 1156 citations, and an average of 27.66 citations per article. The h-index, a composite metric of quantity and quality of scientific output (Kamrani et al. 2021 ), reached 60 in this study.
Trends in publications and disciplinary distribution
The number of publications and citations are significant indicators of the research field’s development, reflecting its continuity, attention, and impact (Ale Ebrahim et al. 2014 ). The ranking of annual publications and citations in the field of older adults’ technology acceptance studies is presented chronologically in Fig. 2A . The figure shows a clear upward trend in the amount of literature in this field. Between 2013 and 2017, the number of publications increased slowly and decreased in 2018. However, in 2019, the number of publications increased rapidly to 52 and reached a peak of 108 in 2022, which is 6.75 times higher than in 2013. In 2022, the frequency of document citations reached its highest point with 3466 citations, reflecting the widespread recognition and citation of research in this field. Moreover, the curve of the annual number of publications fits a quadratic function, with a goodness-of-fit R 2 of 0.9661, indicating that the number of future publications is expected to increase even more rapidly.
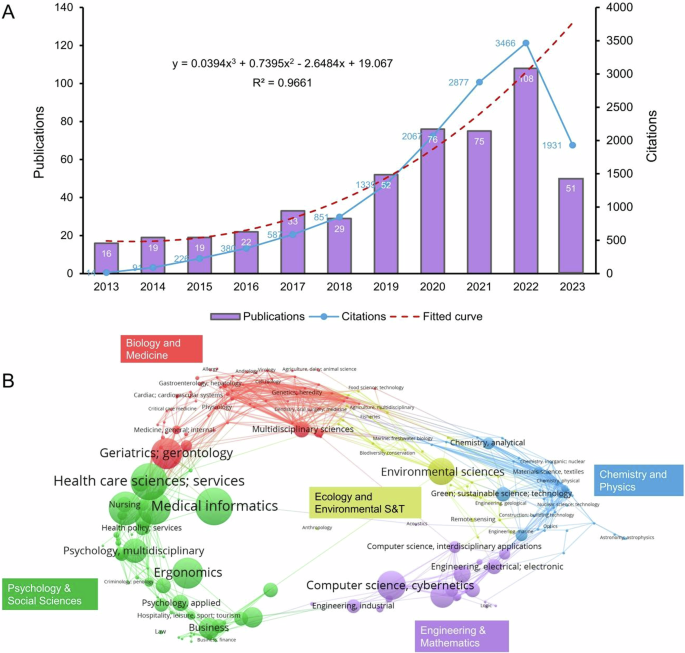
A Trends in trends in annual publications and citations (2013–2023). B Overlay analysis of the distribution of discipline fields.
Figure 2B shows that research on older adults’ technology acceptance involves the integration of multidisciplinary knowledge. According to Web of Science Categories, these 500 articles are distributed across 85 different disciplines. We have tabulated the top ten disciplines by publication volume (Table 2 ), which include Medical Informatics (75 articles, 15.00%), Health Care Sciences & Services (71 articles, 14.20%), Gerontology (61 articles, 12.20%), Public Environmental & Occupational Health (57 articles, 11.40%), and Geriatrics & Gerontology (52 articles, 10.40%), among others. The high output in these disciplines reflects the concentrated global academic interest in this comprehensive research topic. Additionally, interdisciplinary research approaches provide diverse perspectives and a solid theoretical foundation for studies on older adults’ technology acceptance, also paving the way for new research directions.
Knowledge flow analysis
A dual-map overlay is a CiteSpace map superimposed on top of a base map, which shows the interrelationships between journals in different domains, representing the publication and citation activities in each domain (Chen and Leydesdorff 2014 ). The overlay map reveals the link between the citing domain (on the left side) and the cited domain (on the right side), reflecting the knowledge flow of the discipline at the journal level (Leydesdorff and Rafols 2012 ). We utilize the in-built Z-score algorithm of the software to cluster the graph, as shown in Fig. 3 .
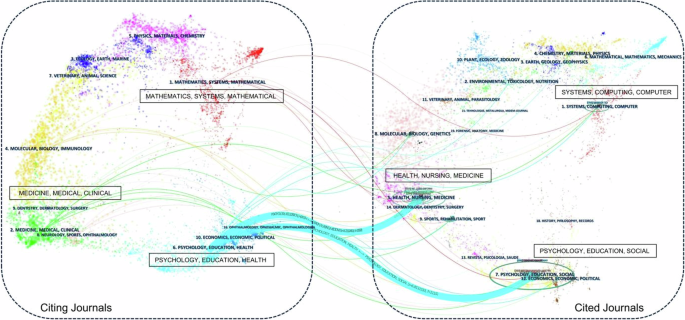
The left side shows the citing journal, and the right side shows the cited journal.
Figure 3 shows the distribution of citing journals clusters for older adults’ technology acceptance on the left side, while the right side refers to the main cited journals clusters. Two knowledge flow citation trajectories were obtained; they are presented by the color of the cited regions, and the thickness of these trajectories is proportional to the Z-score scaled frequency of citations (Chen et al. 2014 ). Within the cited regions, the most popular fields with the most records covered are “HEALTH, NURSING, MEDICINE” and “PSYCHOLOGY, EDUCATION, SOCIAL”, and the elliptical aspect ratio of these two fields stands out. Fields have prominent elliptical aspect ratios, highlighting their significant influence on older adults’ technology acceptance research. Additionally, the major citation trajectories originate in these two areas and progress to the frontier research area of “PSYCHOLOGY, EDUCATION, HEALTH”. It is worth noting that the citation trajectory from “PSYCHOLOGY, EDUCATION, SOCIAL” has a significant Z-value (z = 6.81), emphasizing the significance and impact of this development path. In the future, “MATHEMATICS, SYSTEMS, MATHEMATICAL”, “MOLECULAR, BIOLOGY, IMMUNOLOGY”, and “NEUROLOGY, SPORTS, OPHTHALMOLOGY” may become emerging fields. The fields of “MEDICINE, MEDICAL, CLINICAL” may be emerging areas of cutting-edge research.
Main research journals analysis
Table 3 provides statistics for the top ten journals by publication volume in the field of older adults’ technology acceptance. Together, these journals have published 137 articles, accounting for 27.40% of the total publications, indicating that there is no highly concentrated core group of journals in this field, with publications being relatively dispersed. Notably, Computers in Human Behavior , Journal of Medical Internet Research , and International Journal of Human-Computer Interaction each lead with 15 publications. In terms of citation metrics, International Journal of Medical Informatics and Computers in Human Behavior stand out significantly, with the former accumulating a total of 1,904 citations, averaging 211.56 citations per article, and the latter totaling 1,449 citations, with an average of 96.60 citations per article. These figures emphasize the academic authority and widespread impact of these journals within the research field.
Research power (RQ2)
Countries and collaborations analysis.
The analysis revealed the global research pattern for country distribution and collaboration (Chen et al. 2019 ). Figure 4A shows the network of national collaborations on older adults’ technology acceptance research. The size of the bubbles represents the amount of publications in each country, while the thickness of the connecting lines expresses the closeness of the collaboration among countries. Generally, this research subject has received extensive international attention, with China and the USA publishing far more than any other countries. China has established notable research collaborations with the USA, UK and Malaysia in this field, while other countries have collaborations, but the closeness is relatively low and scattered. Figure 4B shows the annual publication volume dynamics of the top ten countries in terms of total publications. Since 2017, China has consistently increased its annual publications, while the USA has remained relatively stable. In 2019, the volume of publications in each country increased significantly, this was largely due to the global outbreak of the COVID-19 pandemic, which has led to increased reliance on information technology among the elderly for medical consultations, online socialization, and health management (Sinha et al. 2021 ). This phenomenon has led to research advances in technology acceptance among older adults in various countries. Table 4 shows that the top ten countries account for 93.20% of the total cumulative number of publications, with each country having published more than 20 papers. Among these ten countries, all of them except China are developed countries, indicating that the research field of older adults’ technology acceptance has received general attention from developed countries. Currently, China and the USA were the leading countries in terms of publications with 111 and 104 respectively, accounting for 22.20% and 20.80%. The UK, Germany, Italy, and the Netherlands also made significant contributions. The USA and China ranked first and second in terms of the number of citations, while the Netherlands had the highest average citations, indicating the high impact and quality of its research. The UK has shown outstanding performance in international cooperation, while the USA highlights its significant academic influence in this field with the highest h-index value.
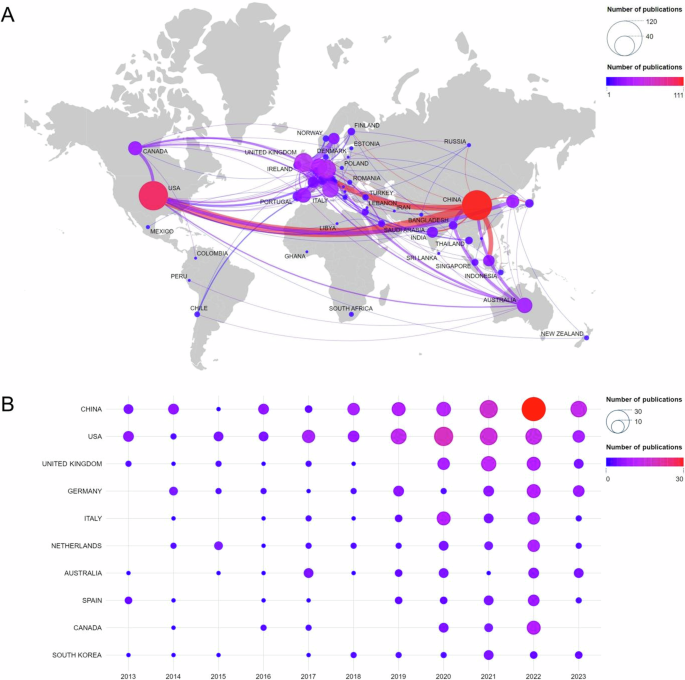
A National collaboration network. B Annual volume of publications in the top 10 countries.
Institutions and authors analysis
Analyzing the number of publications and citations can reveal an institution’s or author’s research strength and influence in a particular research area (Kwiek 2021 ). Tables 5 and 6 show the statistics of the institutions and authors whose publication counts are in the top ten, respectively. As shown in Table 5 , higher education institutions hold the main position in this research field. Among the top ten institutions, City University of Hong Kong and The University of Hong Kong from China lead with 14 and 9 publications, respectively. City University of Hong Kong has the highest h-index, highlighting its significant influence in the field. It is worth noting that Tilburg University in the Netherlands is not among the top five in terms of publications, but the high average citation count (130.14) of its literature demonstrates the high quality of its research.
After analyzing the authors’ output using Price’s Law (Redner 1998 ), the highest number of publications among the authors counted ( n = 10) defines a publication threshold of 3 for core authors in this research area. As a result of quantitative screening, a total of 63 core authors were identified. Table 6 shows that Chen from Zhejiang University, China, Ziefle from RWTH Aachen University, Germany, and Rogers from Macquarie University, Australia, were the top three authors in terms of the number of publications, with 10, 9, and 8 articles, respectively. In terms of average citation rate, Peek and Wouters, both scholars from the Netherlands, have significantly higher rates than other scholars, with 183.2 and 152.67 respectively. This suggests that their research is of high quality and widely recognized. Additionally, Chen and Rogers have high h-indices in this field.
Knowledge base and theme progress (RQ3)
Research knowledge base.
Co-citation relationships occur when two documents are cited together (Zhang and Zhu 2022 ). Co-citation mapping uses references as nodes to represent the knowledge base of a subject area (Min et al. 2021). Figure 5A illustrates co-occurrence mapping in older adults’ technology acceptance research, where larger nodes signify higher co-citation frequencies. Co-citation cluster analysis can be used to explore knowledge structure and research boundaries (Hota et al. 2020 ; Shiau et al. 2023 ). The co-citation clustering mapping of older adults’ technology acceptance research literature (Fig. 5B ) shows that the Q value of the clustering result is 0.8129 (>0.3), and the average value of the weight S is 0.9391 (>0.7), indicating that the clusters are uniformly distributed with a significant and credible structure. This further proves that the boundaries of the research field are clear and there is significant differentiation in the field. The figure features 18 cluster labels, each associated with thematic color blocks corresponding to different time slices. Highlighted emerging research themes include #2 Smart Home Technology, #7 Social Live, and #10 Customer Service. Furthermore, the clustering labels extracted are primarily classified into three categories: theoretical model deepening, emerging technology applications, research methods and evaluation, as detailed in Table 7 .
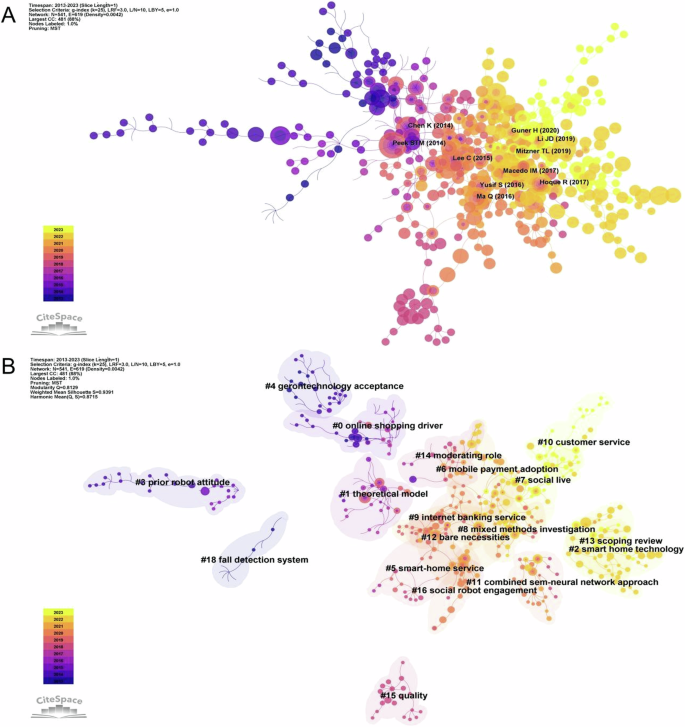
A Co-citation analysis of references. B Clustering network analysis of references.
Seminal literature analysis
The top ten nodes in terms of co-citation frequency were selected for further analysis. Table 8 displays the corresponding node information. Studies were categorized into four main groups based on content analysis. (1) Research focusing on specific technology usage by older adults includes studies by Peek et al. ( 2014 ), Ma et al. ( 2016 ), Hoque and Sorwar ( 2017 ), and Li et al. ( 2019 ), who investigated the factors influencing the use of e-technology, smartphones, mHealth, and smart wearables, respectively. (2) Concerning the development of theoretical models of technology acceptance, Chen and Chan ( 2014 ) introduced the Senior Technology Acceptance Model (STAM), and Macedo ( 2017 ) analyzed the predictive power of UTAUT2 in explaining older adults’ intentional behaviors and information technology usage. (3) In exploring older adults’ information technology adoption and behavior, Lee and Coughlin ( 2015 ) emphasized that the adoption of technology by older adults is a multifactorial process that includes performance, price, value, usability, affordability, accessibility, technical support, social support, emotion, independence, experience, and confidence. Yusif et al. ( 2016 ) conducted a literature review examining the key barriers affecting older adults’ adoption of assistive technology, including factors such as privacy, trust, functionality/added value, cost, and stigma. (4) From the perspective of research into older adults’ technology acceptance, Mitzner et al. ( 2019 ) assessed the long-term usage of computer systems designed for the elderly, whereas Guner and Acarturk ( 2020 ) compared information technology usage and acceptance between older and younger adults. The breadth and prevalence of this literature make it a vital reference for researchers in the field, also providing new perspectives and inspiration for future research directions.
Research thematic progress
Burst citation is a node of literature that guides the sudden change in dosage, which usually represents a prominent development or major change in a particular field, with innovative and forward-looking qualities. By analyzing the emergent literature, it is often easy to understand the dynamics of the subject area, mapping the emerging thematic change (Chen et al. 2022 ). Figure 6 shows the burst citation mapping in the field of older adults’ technology acceptance research, with burst citations represented by red nodes (Fig. 6A ). For the ten papers with the highest burst intensity (Fig. 6B ), this study will conduct further analysis in conjunction with literature review.
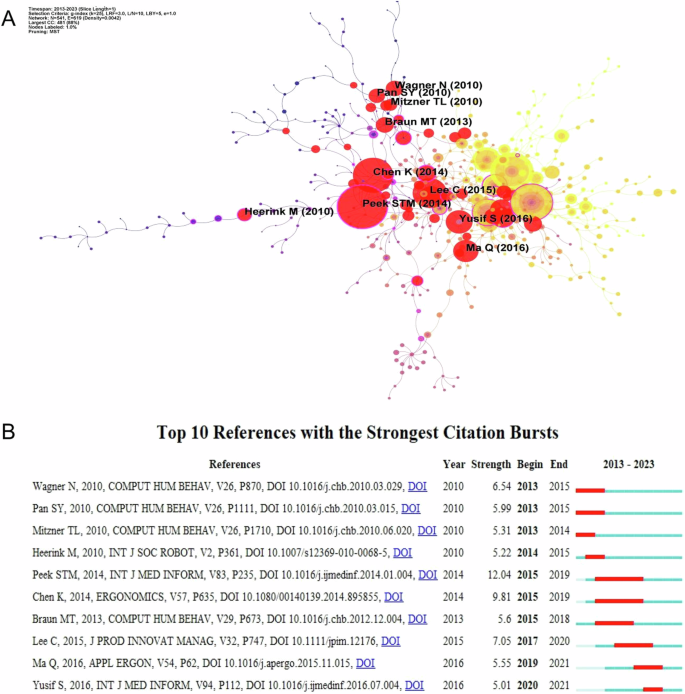
A Burst detection of co-citation. B The top 10 references with the strongest citation bursts.
As shown in Fig. 6 , Mitzner et al. ( 2010 ) broke the stereotype that older adults are fearful of technology, found that they actually have positive attitudes toward technology, and emphasized the centrality of ease of use and usefulness in the process of technology acceptance. This finding provides an important foundation for subsequent research. During the same period, Wagner et al. ( 2010 ) conducted theory-deepening and applied research on technology acceptance among older adults. The research focused on older adults’ interactions with computers from the perspective of Social Cognitive Theory (SCT). This expanded the understanding of technology acceptance, particularly regarding the relationship between behavior, environment, and other SCT elements. In addition, Pan and Jordan-Marsh ( 2010 ) extended the TAM to examine the interactions among predictors of perceived usefulness, perceived ease of use, subjective norm, and convenience conditions when older adults use the Internet, taking into account the moderating roles of gender and age. Heerink et al. ( 2010 ) adapted and extended the UTAUT, constructed a technology acceptance model specifically designed for older users’ acceptance of assistive social agents, and validated it using controlled experiments and longitudinal data, explaining intention to use by combining functional assessment and social interaction variables.
Then the research theme shifted to an in-depth analysis of the factors influencing technology acceptance among older adults. Two papers with high burst strengths emerged during this period: Peek et al. ( 2014 ) (Strength = 12.04), Chen and Chan ( 2014 ) (Strength = 9.81). Through a systematic literature review and empirical study, Peek STM and Chen K, among others, identified multidimensional factors that influence older adults’ technology acceptance. Peek et al. ( 2014 ) analyzed literature on the acceptance of in-home care technology among older adults and identified six factors that influence their acceptance: concerns about technology, expected benefits, technology needs, technology alternatives, social influences, and older adult characteristics, with a focus on differences between pre- and post-implementation factors. Chen and Chan ( 2014 ) constructed the STAM by administering a questionnaire to 1012 older adults and adding eight important factors, including technology anxiety, self-efficacy, cognitive ability, and physical function, based on the TAM. This enriches the theoretical foundation of the field. In addition, Braun ( 2013 ) highlighted the role of perceived usefulness, trust in social networks, and frequency of Internet use in older adults’ use of social networks, while ease of use and social pressure were not significant influences. These findings contribute to the study of older adults’ technology acceptance within specific technology application domains.
Recent research has focused on empirical studies of personal factors and emerging technologies. Ma et al. ( 2016 ) identified key personal factors affecting smartphone acceptance among older adults through structured questionnaires and face-to-face interviews with 120 participants. The study found that cost, self-satisfaction, and convenience were important factors influencing perceived usefulness and ease of use. This study offers empirical evidence to comprehend the main factors that drive smartphone acceptance among Chinese older adults. Additionally, Yusif et al. ( 2016 ) presented an overview of the obstacles that hinder older adults’ acceptance of assistive technologies, focusing on privacy, trust, and functionality.
In summary, research on older adults’ technology acceptance has shifted from early theoretical deepening and analysis of influencing factors to empirical studies in the areas of personal factors and emerging technologies, which have greatly enriched the theoretical basis of older adults’ technology acceptance and provided practical guidance for the design of emerging technology products.
Research hotspots, evolutionary trends, and quality distribution (RQ4)
Core keywords analysis.
Keywords concise the main idea and core of the literature, and are a refined summary of the research content (Huang et al. 2021 ). In CiteSpace, nodes with a centrality value greater than 0.1 are considered to be critical nodes. Analyzing keywords with high frequency and centrality helps to visualize the hot topics in the research field (Park et al. 2018 ). The merged keywords were imported into CiteSpace, and the top 10 keywords were counted and sorted by frequency and centrality respectively, as shown in Table 9 . The results show that the keyword “TAM” has the highest frequency (92), followed by “UTAUT” (24), which reflects that the in-depth study of the existing technology acceptance model and its theoretical expansion occupy a central position in research related to older adults’ technology acceptance. Furthermore, the terms ‘assistive technology’ and ‘virtual reality’ are both high-frequency and high-centrality terms (frequency = 17, centrality = 0.10), indicating that the research on assistive technology and virtual reality for older adults is the focus of current academic attention.
Research hotspots analysis
Using VOSviewer for keyword co-occurrence analysis organizes keywords into groups or clusters based on their intrinsic connections and frequencies, clearly highlighting the research field’s hot topics. The connectivity among keywords reveals correlations between different topics. To ensure accuracy, the analysis only considered the authors’ keywords. Subsequently, the keywords were filtered by setting the keyword frequency to 5 to obtain the keyword clustering map of the research on older adults’ technology acceptance research keyword clustering mapping (Fig. 7 ), combined with the keyword co-occurrence clustering network (Fig. 7A ) and the corresponding density situation (Fig. 7B ) to make a detailed analysis of the following four groups of clustered themes.
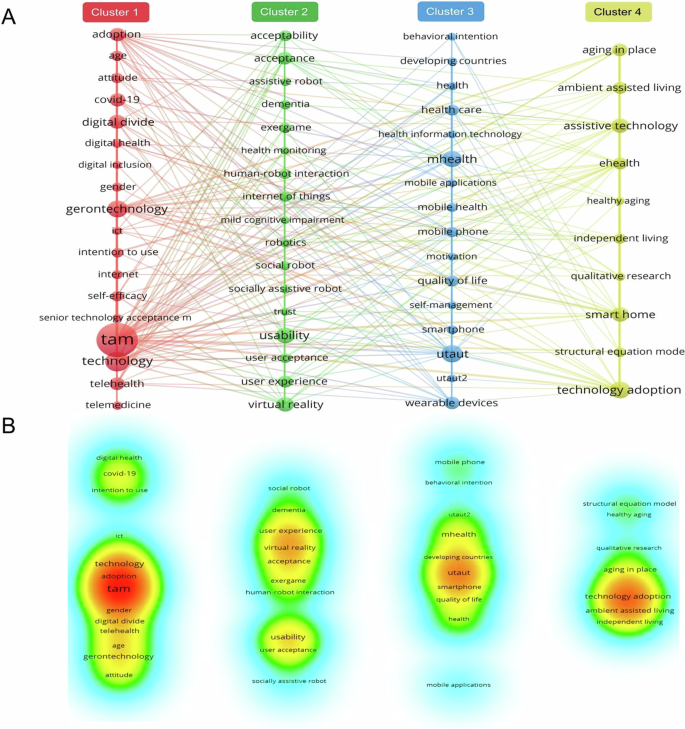
A Co-occurrence clustering network. B Keyword density.
Cluster #1—Research on the factors influencing technology adoption among older adults is a prominent topic, covering age, gender, self-efficacy, attitude, and and intention to use (Berkowsky et al. 2017 ; Wang et al. 2017 ). It also examined older adults’ attitudes towards and acceptance of digital health technologies (Ahmad and Mozelius, 2022 ). Moreover, the COVID-19 pandemic, significantly impacting older adults’ technology attitudes and usage, has underscored the study’s importance and urgency. Therefore, it is crucial to conduct in-depth studies on how older adults accept, adopt, and effectively use new technologies, to address their needs and help them overcome the digital divide within digital inclusion. This will improve their quality of life and healthcare experiences.
Cluster #2—Research focuses on how older adults interact with assistive technologies, especially assistive robots and health monitoring devices, emphasizing trust, usability, and user experience as crucial factors (Halim et al. 2022 ). Moreover, health monitoring technologies effectively track and manage health issues common in older adults, like dementia and mild cognitive impairment (Lussier et al. 2018 ; Piau et al. 2019 ). Interactive exercise games and virtual reality have been deployed to encourage more physical and cognitive engagement among older adults (Campo-Prieto et al. 2021 ). Personalized and innovative technology significantly enhances older adults’ participation, improving their health and well-being.
Cluster #3—Optimizing health management for older adults using mobile technology. With the development of mobile health (mHealth) and health information technology, mobile applications, smartphones, and smart wearable devices have become effective tools to help older users better manage chronic conditions, conduct real-time health monitoring, and even receive telehealth services (Dupuis and Tsotsos 2018 ; Olmedo-Aguirre et al. 2022 ; Kim et al. 2014 ). Additionally, these technologies can mitigate the problem of healthcare resource inequality, especially in developing countries. Older adults’ acceptance and use of these technologies are significantly influenced by their behavioral intentions, motivational factors, and self-management skills. These internal motivational factors, along with external factors, jointly affect older adults’ performance in health management and quality of life.
Cluster #4—Research on technology-assisted home care for older adults is gaining popularity. Environmentally assisted living enhances older adults’ independence and comfort at home, offering essential support and security. This has a crucial impact on promoting healthy aging (Friesen et al. 2016 ; Wahlroos et al. 2023 ). The smart home is a core application in this field, providing a range of solutions that facilitate independent living for the elderly in a highly integrated and user-friendly manner. This fulfills different dimensions of living and health needs (Majumder et al. 2017 ). Moreover, eHealth offers accurate and personalized health management and healthcare services for older adults (Delmastro et al. 2018 ), ensuring their needs are met at home. Research in this field often employs qualitative methods and structural equation modeling to fully understand older adults’ needs and experiences at home and analyze factors influencing technology adoption.
Evolutionary trends analysis
To gain a deeper understanding of the evolutionary trends in research hotspots within the field of older adults’ technology acceptance, we conducted a statistical analysis of the average appearance times of keywords, using CiteSpace to generate the time-zone evolution mapping (Fig. 8 ) and burst keywords. The time-zone mapping visually displays the evolution of keywords over time, intuitively reflecting the frequency and initial appearance of keywords in research, commonly used to identify trends in research topics (Jing et al. 2024a ; Kumar et al. 2021 ). Table 10 lists the top 15 keywords by burst strength, with the red sections indicating high-frequency citations and their burst strength in specific years. These burst keywords reveal the focus and trends of research themes over different periods (Kleinberg 2002 ). Combining insights from the time-zone mapping and burst keywords provides more objective and accurate research insights (Wang et al. 2023b ).
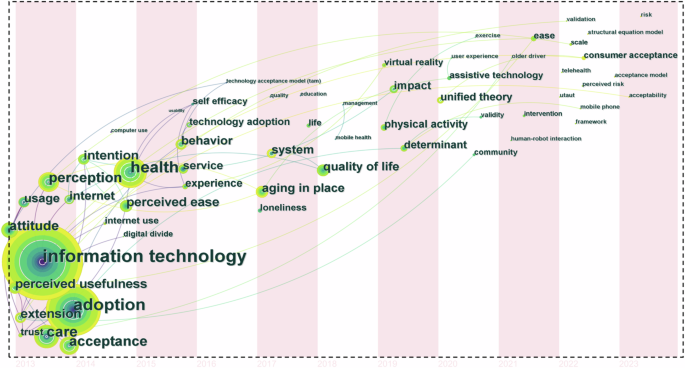
Reflecting the frequency and time of first appearance of keywords in the study.
An integrated analysis of Fig. 8 and Table 10 shows that early research on older adults’ technology acceptance primarily focused on factors such as perceived usefulness, ease of use, and attitudes towards information technology, including their use of computers and the internet (Pan and Jordan-Marsh 2010 ), as well as differences in technology use between older adults and other age groups (Guner and Acarturk 2020 ). Subsequently, the research focus expanded to improving the quality of life for older adults, exploring how technology can optimize health management and enhance the possibility of independent living, emphasizing the significant role of technology in improving the quality of life for the elderly. With ongoing technological advancements, recent research has shifted towards areas such as “virtual reality,” “telehealth,” and “human-robot interaction,” with a focus on the user experience of older adults (Halim et al. 2022 ). The appearance of keywords such as “physical activity” and “exercise” highlights the value of technology in promoting physical activity and health among older adults. This phase of research tends to make cutting-edge technology genuinely serve the practical needs of older adults, achieving its widespread application in daily life. Additionally, research has focused on expanding and quantifying theoretical models of older adults’ technology acceptance, involving keywords such as “perceived risk”, “validation” and “UTAUT”.
In summary, from 2013 to 2023, the field of older adults’ technology acceptance has evolved from initial explorations of influencing factors, to comprehensive enhancements in quality of life and health management, and further to the application and deepening of theoretical models and cutting-edge technologies. This research not only reflects the diversity and complexity of the field but also demonstrates a comprehensive and in-depth understanding of older adults’ interactions with technology across various life scenarios and needs.
Research quality distribution
To reveal the distribution of research quality in the field of older adults’ technology acceptance, a strategic diagram analysis is employed to calculate and illustrate the internal development and interrelationships among various research themes (Xie et al. 2020 ). The strategic diagram uses Centrality as the X-axis and Density as the Y-axis to divide into four quadrants, where the X-axis represents the strength of the connection between thematic clusters and other themes, with higher values indicating a central position in the research field; the Y-axis indicates the level of development within the thematic clusters, with higher values denoting a more mature and widely recognized field (Li and Zhou 2020 ).
Through cluster analysis and manual verification, this study categorized 61 core keywords (Frequency ≥5) into 11 thematic clusters. Subsequently, based on the keywords covered by each thematic cluster, the research themes and their directions for each cluster were summarized (Table 11 ), and the centrality and density coordinates for each cluster were precisely calculated (Table 12 ). Finally, a strategic diagram of the older adults’ technology acceptance research field was constructed (Fig. 9 ). Based on the distribution of thematic clusters across the quadrants in the strategic diagram, the structure and developmental trends of the field were interpreted.
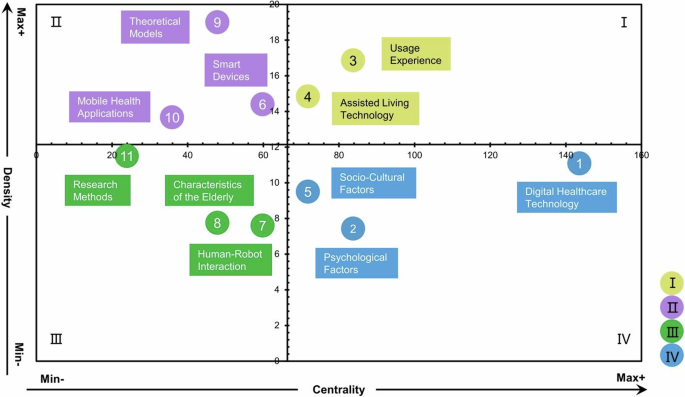
Classification and visualization of theme clusters based on density and centrality.
As illustrated in Fig. 9 , (1) the theme clusters of #3 Usage Experience and #4 Assisted Living Technology are in the first quadrant, characterized by high centrality and density. Their internal cohesion and close links with other themes indicate their mature development, systematic research content or directions have been formed, and they have a significant influence on other themes. These themes play a central role in the field of older adults’ technology acceptance and have promising prospects. (2) The theme clusters of #6 Smart Devices, #9 Theoretical Models, and #10 Mobile Health Applications are in the second quadrant, with higher density but lower centrality. These themes have strong internal connections but weaker external links, indicating that these three themes have received widespread attention from researchers and have been the subject of related research, but more as self-contained systems and exhibit independence. Therefore, future research should further explore in-depth cooperation and cross-application with other themes. (3) The theme clusters of #7 Human-Robot Interaction, #8 Characteristics of the Elderly, and #11 Research Methods are in the third quadrant, with lower centrality and density. These themes are loosely connected internally and have weak links with others, indicating their developmental immaturity. Compared to other topics, they belong to the lower attention edge and niche themes, and there is a need for further investigation. (4) The theme clusters of #1 Digital Healthcare Technology, #2 Psychological Factors, and #5 Socio-Cultural Factors are located in the fourth quadrant, with high centrality but low density. Although closely associated with other research themes, the internal cohesion within these clusters is relatively weak. This suggests that while these themes are closely linked to other research areas, their own development remains underdeveloped, indicating a core immaturity. Nevertheless, these themes are crucial within the research domain of elderly technology acceptance and possess significant potential for future exploration.
Discussion on distribution power (RQ1)
Over the past decade, academic interest and influence in the area of older adults’ technology acceptance have significantly increased. This trend is evidenced by a quantitative analysis of publication and citation volumes, particularly noticeable in 2019 and 2022, where there was a substantial rise in both metrics. The rise is closely linked to the widespread adoption of emerging technologies such as smart homes, wearable devices, and telemedicine among older adults. While these technologies have enhanced their quality of life, they also pose numerous challenges, sparking extensive research into their acceptance, usage behaviors, and influencing factors among the older adults (Pirzada et al. 2022 ; Garcia Reyes et al. 2023 ). Furthermore, the COVID-19 pandemic led to a surge in technology demand among older adults, especially in areas like medical consultation, online socialization, and health management, further highlighting the importance and challenges of technology. Health risks and social isolation have compelled older adults to rely on technology for daily activities, accelerating its adoption and application within this demographic. This phenomenon has made technology acceptance a critical issue, driving societal and academic focus on the study of technology acceptance among older adults.
The flow of knowledge at the level of high-output disciplines and journals, along with the primary publishing outlets, indicates the highly interdisciplinary nature of research into older adults’ technology acceptance. This reflects the complexity and breadth of issues related to older adults’ technology acceptance, necessitating the integration of multidisciplinary knowledge and approaches. Currently, research is primarily focused on medical health and human-computer interaction, demonstrating academic interest in improving health and quality of life for older adults and addressing the urgent needs related to their interactions with technology. In the field of medical health, research aims to provide advanced and innovative healthcare technologies and services to meet the challenges of an aging population while improving the quality of life for older adults (Abdi et al. 2020 ; Wilson et al. 2021 ). In the field of human-computer interaction, research is focused on developing smarter and more user-friendly interaction models to meet the needs of older adults in the digital age, enabling them to actively participate in social activities and enjoy a higher quality of life (Sayago, 2019 ). These studies are crucial for addressing the challenges faced by aging societies, providing increased support and opportunities for the health, welfare, and social participation of older adults.
Discussion on research power (RQ2)
This study analyzes leading countries and collaboration networks, core institutions and authors, revealing the global research landscape and distribution of research strength in the field of older adults’ technology acceptance, and presents quantitative data on global research trends. From the analysis of country distribution and collaborations, China and the USA hold dominant positions in this field, with developed countries like the UK, Germany, Italy, and the Netherlands also excelling in international cooperation and research influence. The significant investment in technological research and the focus on the technological needs of older adults by many developed countries reflect their rapidly aging societies, policy support, and resource allocation.
China is the only developing country that has become a major contributor in this field, indicating its growing research capabilities and high priority given to aging societies and technological innovation. Additionally, China has close collaborations with countries such as USA, the UK, and Malaysia, driven not only by technological research needs but also by shared challenges and complementarities in aging issues among these nations. For instance, the UK has extensive experience in social welfare and aging research, providing valuable theoretical guidance and practical experience. International collaborations, aimed at addressing the challenges of aging, integrate the strengths of various countries, advancing in-depth and widespread development in the research of technology acceptance among older adults.
At the institutional and author level, City University of Hong Kong leads in publication volume, with research teams led by Chan and Chen demonstrating significant academic activity and contributions. Their research primarily focuses on older adults’ acceptance and usage behaviors of various technologies, including smartphones, smart wearables, and social robots (Chen et al. 2015 ; Li et al. 2019 ; Ma et al. 2016 ). These studies, targeting specific needs and product characteristics of older adults, have developed new models of technology acceptance based on existing frameworks, enhancing the integration of these technologies into their daily lives and laying a foundation for further advancements in the field. Although Tilburg University has a smaller publication output, it holds significant influence in the field of older adults’ technology acceptance. Particularly, the high citation rate of Peek’s studies highlights their excellence in research. Peek extensively explored older adults’ acceptance and usage of home care technologies, revealing the complexity and dynamics of their technology use behaviors. His research spans from identifying systemic influencing factors (Peek et al. 2014 ; Peek et al. 2016 ), emphasizing familial impacts (Luijkx et al. 2015 ), to constructing comprehensive models (Peek et al. 2017 ), and examining the dynamics of long-term usage (Peek et al. 2019 ), fully reflecting the evolving technology landscape and the changing needs of older adults. Additionally, the ongoing contributions of researchers like Ziefle, Rogers, and Wouters in the field of older adults’ technology acceptance demonstrate their research influence and leadership. These researchers have significantly enriched the knowledge base in this area with their diverse perspectives. For instance, Ziefle has uncovered the complex attitudes of older adults towards technology usage, especially the trade-offs between privacy and security, and how different types of activities affect their privacy needs (Maidhof et al. 2023 ; Mujirishvili et al. 2023 ; Schomakers and Ziefle 2023 ; Wilkowska et al. 2022 ), reflecting a deep exploration and ongoing innovation in the field of older adults’ technology acceptance.
Discussion on knowledge base and thematic progress (RQ3)
Through co-citation analysis and systematic review of seminal literature, this study reveals the knowledge foundation and thematic progress in the field of older adults’ technology acceptance. Co-citation networks and cluster analyses illustrate the structural themes of the research, delineating the differentiation and boundaries within this field. Additionally, burst detection analysis offers a valuable perspective for understanding the thematic evolution in the field of technology acceptance among older adults. The development and innovation of theoretical models are foundational to this research. Researchers enhance the explanatory power of constructed models by deepening and expanding existing technology acceptance theories to address theoretical limitations. For instance, Heerink et al. ( 2010 ) modified and expanded the UTAUT model by integrating functional assessment and social interaction variables to create the almere model. This model significantly enhances the ability to explain the intentions of older users in utilizing assistive social agents and improves the explanation of actual usage behaviors. Additionally, Chen and Chan ( 2014 ) extended the TAM to include age-related health and capability features of older adults, creating the STAM, which substantially improves predictions of older adults’ technology usage behaviors. Personal attributes, health and capability features, and facilitating conditions have a direct impact on technology acceptance. These factors more effectively predict older adults’ technology usage behaviors than traditional attitudinal factors.
With the advancement of technology and the application of emerging technologies, new research topics have emerged, increasingly focusing on older adults’ acceptance and use of these technologies. Prior to this, the study by Mitzner et al. ( 2010 ) challenged the stereotype of older adults’ conservative attitudes towards technology, highlighting the central roles of usability and usefulness in the technology acceptance process. This discovery laid an important foundation for subsequent research. Research fields such as “smart home technology,” “social life,” and “customer service” are emerging, indicating a shift in focus towards the practical and social applications of technology in older adults’ lives. Research not only focuses on the technology itself but also on how these technologies integrate into older adults’ daily lives and how they can improve the quality of life through technology. For instance, studies such as those by Ma et al. ( 2016 ), Hoque and Sorwar ( 2017 ), and Li et al. ( 2019 ) have explored factors influencing older adults’ use of smartphones, mHealth, and smart wearable devices.
Furthermore, the diversification of research methodologies and innovation in evaluation techniques, such as the use of mixed methods, structural equation modeling (SEM), and neural network (NN) approaches, have enhanced the rigor and reliability of the findings, enabling more precise identification of the factors and mechanisms influencing technology acceptance. Talukder et al. ( 2020 ) employed an effective multimethodological strategy by integrating SEM and NN to leverage the complementary strengths of both approaches, thus overcoming their individual limitations and more accurately analyzing and predicting older adults’ acceptance of wearable health technologies (WHT). SEM is utilized to assess the determinants’ impact on the adoption of WHT, while neural network models validate SEM outcomes and predict the significance of key determinants. This combined approach not only boosts the models’ reliability and explanatory power but also provides a nuanced understanding of the motivations and barriers behind older adults’ acceptance of WHT, offering deep research insights.
Overall, co-citation analysis of the literature in the field of older adults’ technology acceptance has uncovered deeper theoretical modeling and empirical studies on emerging technologies, while emphasizing the importance of research methodological and evaluation innovations in understanding complex social science issues. These findings are crucial for guiding the design and marketing strategies of future technology products, especially in the rapidly growing market of older adults.
Discussion on research hotspots and evolutionary trends (RQ4)
By analyzing core keywords, we can gain deep insights into the hot topics, evolutionary trends, and quality distribution of research in the field of older adults’ technology acceptance. The frequent occurrence of the keywords “TAM” and “UTAUT” indicates that the applicability and theoretical extension of existing technology acceptance models among older adults remain a focal point in academia. This phenomenon underscores the enduring influence of the studies by Davis ( 1989 ) and Venkatesh et al. ( 2003 ), whose models provide a robust theoretical framework for explaining and predicting older adults’ acceptance and usage of emerging technologies. With the widespread application of artificial intelligence (AI) and big data technologies, these theoretical models have incorporated new variables such as perceived risk, trust, and privacy issues (Amin et al. 2024 ; Chen et al. 2024 ; Jing et al. 2024b ; Seibert et al. 2021 ; Wang et al. 2024b ), advancing the theoretical depth and empirical research in this field.
Keyword co-occurrence cluster analysis has revealed multiple research hotspots in the field, including factors influencing technology adoption, interactive experiences between older adults and assistive technologies, the application of mobile health technology in health management, and technology-assisted home care. These studies primarily focus on enhancing the quality of life and health management of older adults through emerging technologies, particularly in the areas of ambient assisted living, smart health monitoring, and intelligent medical care. In these domains, the role of AI technology is increasingly significant (Qian et al. 2021 ; Ho 2020 ). With the evolution of next-generation information technologies, AI is increasingly integrated into elder care systems, offering intelligent, efficient, and personalized service solutions by analyzing the lifestyles and health conditions of older adults. This integration aims to enhance older adults’ quality of life in aspects such as health monitoring and alerts, rehabilitation assistance, daily health management, and emotional support (Lee et al. 2023 ). A survey indicates that 83% of older adults prefer AI-driven solutions when selecting smart products, demonstrating the increasing acceptance of AI in elder care (Zhao and Li 2024 ). Integrating AI into elder care presents both opportunities and challenges, particularly in terms of user acceptance, trust, and long-term usage effects, which warrant further exploration (Mhlanga 2023 ). These studies will help better understand the profound impact of AI technology on the lifestyles of older adults and provide critical references for optimizing AI-driven elder care services.
The Time-zone evolution mapping and burst keyword analysis further reveal the evolutionary trends of research hotspots. Early studies focused on basic technology acceptance models and user perceptions, later expanding to include quality of life and health management. In recent years, research has increasingly focused on cutting-edge technologies such as virtual reality, telehealth, and human-robot interaction, with a concurrent emphasis on the user experience of older adults. This evolutionary process demonstrates a deepening shift from theoretical models to practical applications, underscoring the significant role of technology in enhancing the quality of life for older adults. Furthermore, the strategic coordinate mapping analysis clearly demonstrates the development and mutual influence of different research themes. High centrality and density in the themes of Usage Experience and Assisted Living Technology indicate their mature research status and significant impact on other themes. The themes of Smart Devices, Theoretical Models, and Mobile Health Applications demonstrate self-contained research trends. The themes of Human-Robot Interaction, Characteristics of the Elderly, and Research Methods are not yet mature, but they hold potential for development. Themes of Digital Healthcare Technology, Psychological Factors, and Socio-Cultural Factors are closely related to other themes, displaying core immaturity but significant potential.
In summary, the research hotspots in the field of older adults’ technology acceptance are diverse and dynamic, demonstrating the academic community’s profound understanding of how older adults interact with technology across various life contexts and needs. Under the influence of AI and big data, research should continue to focus on the application of emerging technologies among older adults, exploring in depth how they adapt to and effectively use these technologies. This not only enhances the quality of life and healthcare experiences for older adults but also drives ongoing innovation and development in this field.
Research agenda
Based on the above research findings, to further understand and promote technology acceptance and usage among older adults, we recommend future studies focus on refining theoretical models, exploring long-term usage, and assessing user experience in the following detailed aspects:
Refinement and validation of specific technology acceptance models for older adults: Future research should focus on developing and validating technology acceptance models based on individual characteristics, particularly considering variations in technology acceptance among older adults across different educational levels and cultural backgrounds. This includes factors such as age, gender, educational background, and cultural differences. Additionally, research should examine how well specific technologies, such as wearable devices and mobile health applications, meet the needs of older adults. Building on existing theoretical models, this research should integrate insights from multiple disciplines such as psychology, sociology, design, and engineering through interdisciplinary collaboration to create more accurate and comprehensive models, which should then be validated in relevant contexts.
Deepening the exploration of the relationship between long-term technology use and quality of life among older adults: The acceptance and use of technology by users is a complex and dynamic process (Seuwou et al. 2016 ). Existing research predominantly focuses on older adults’ initial acceptance or short-term use of new technologies; however, the impact of long-term use on their quality of life and health is more significant. Future research should focus on the evolution of older adults’ experiences and needs during long-term technology usage, and the enduring effects of technology on their social interactions, mental health, and life satisfaction. Through longitudinal studies and qualitative analysis, this research reveals the specific needs and challenges of older adults in long-term technology use, providing a basis for developing technologies and strategies that better meet their requirements. This understanding aids in comprehensively assessing the impact of technology on older adults’ quality of life and guiding the optimization and improvement of technological products.
Evaluating the Importance of User Experience in Research on Older Adults’ Technology Acceptance: Understanding the mechanisms of information technology acceptance and use is central to human-computer interaction research. Although technology acceptance models and user experience models differ in objectives, they share many potential intersections. Technology acceptance research focuses on structured prediction and assessment, while user experience research concentrates on interpreting design impacts and new frameworks. Integrating user experience to assess older adults’ acceptance of technology products and systems is crucial (Codfrey et al. 2022 ; Wang et al. 2019 ), particularly for older users, where specific product designs should emphasize practicality and usability (Fisk et al. 2020 ). Researchers need to explore innovative age-appropriate design methods to enhance older adults’ usage experience. This includes studying older users’ actual usage preferences and behaviors, optimizing user interfaces, and interaction designs. Integrating feedback from older adults to tailor products to their needs can further promote their acceptance and continued use of technology products.
Conclusions
This study conducted a systematic review of the literature on older adults’ technology acceptance over the past decade through bibliometric analysis, focusing on the distribution power, research power, knowledge base and theme progress, research hotspots, evolutionary trends, and quality distribution. Using a combination of quantitative and qualitative methods, this study has reached the following conclusions:
Technology acceptance among older adults has become a hot topic in the international academic community, involving the integration of knowledge across multiple disciplines, including Medical Informatics, Health Care Sciences Services, and Ergonomics. In terms of journals, “PSYCHOLOGY, EDUCATION, HEALTH” represents a leading field, with key publications including Computers in Human Behavior , Journal of Medical Internet Research , and International Journal of Human-Computer Interaction . These journals possess significant academic authority and extensive influence in the field.
Research on technology acceptance among older adults is particularly active in developed countries, with China and USA publishing significantly more than other nations. The Netherlands leads in high average citation rates, indicating the depth and impact of its research. Meanwhile, the UK stands out in terms of international collaboration. At the institutional level, City University of Hong Kong and The University of Hong Kong in China are in leading positions. Tilburg University in the Netherlands demonstrates exceptional research quality through its high average citation count. At the author level, Chen from China has the highest number of publications, while Peek from the Netherlands has the highest average citation count.
Co-citation analysis of references indicates that the knowledge base in this field is divided into three main categories: theoretical model deepening, emerging technology applications, and research methods and evaluation. Seminal literature focuses on four areas: specific technology use by older adults, expansion of theoretical models of technology acceptance, information technology adoption behavior, and research perspectives. Research themes have evolved from initial theoretical deepening and analysis of influencing factors to empirical studies on individual factors and emerging technologies.
Keyword analysis indicates that TAM and UTAUT are the most frequently occurring terms, while “assistive technology” and “virtual reality” are focal points with high frequency and centrality. Keyword clustering analysis reveals that research hotspots are concentrated on the influencing factors of technology adoption, human-robot interaction experiences, mobile health management, and technology for aging in place. Time-zone evolution mapping and burst keyword analysis have revealed the research evolution from preliminary exploration of influencing factors, to enhancements in quality of life and health management, and onto advanced technology applications and deepening of theoretical models. Furthermore, analysis of research quality distribution indicates that Usage Experience and Assisted Living Technology have become core topics, while Smart Devices, Theoretical Models, and Mobile Health Applications point towards future research directions.
Through this study, we have systematically reviewed the dynamics, core issues, and evolutionary trends in the field of older adults’ technology acceptance, constructing a comprehensive Knowledge Mapping of the domain and presenting a clear framework of existing research. This not only lays the foundation for subsequent theoretical discussions and innovative applications in the field but also provides an important reference for relevant scholars.
Limitations
To our knowledge, this is the first bibliometric analysis concerning technology acceptance among older adults, and we adhered strictly to bibliometric standards throughout our research. However, this study relies on the Web of Science Core Collection, and while its authority and breadth are widely recognized, this choice may have missed relevant literature published in other significant databases such as PubMed, Scopus, and Google Scholar, potentially overlooking some critical academic contributions. Moreover, given that our analysis was confined to literature in English, it may not reflect studies published in other languages, somewhat limiting the global representativeness of our data sample.
It is noteworthy that with the rapid development of AI technology, its increasingly widespread application in elderly care services is significantly transforming traditional care models. AI is profoundly altering the lifestyles of the elderly, from health monitoring and smart diagnostics to intelligent home systems and personalized care, significantly enhancing their quality of life and health care standards. The potential for AI technology within the elderly population is immense, and research in this area is rapidly expanding. However, due to the restrictive nature of the search terms used in this study, it did not fully cover research in this critical area, particularly in addressing key issues such as trust, privacy, and ethics.
Consequently, future research should not only expand data sources, incorporating multilingual and multidatabase literature, but also particularly focus on exploring older adults’ acceptance of AI technology and its applications, in order to construct a more comprehensive academic landscape of older adults’ technology acceptance, thereby enriching and extending the knowledge system and academic trends in this field.
Data availability
The datasets analyzed during the current study are available in the Dataverse repository: https://doi.org/10.7910/DVN/6K0GJH .
Abdi S, de Witte L, Hawley M (2020) Emerging technologies with potential care and support applications for older people: review of gray literature. JMIR Aging 3(2):e17286. https://doi.org/10.2196/17286
Article PubMed PubMed Central Google Scholar
Achuthan K, Nair VK, Kowalski R, Ramanathan S, Raman R (2023) Cyberbullying research—Alignment to sustainable development and impact of COVID-19: Bibliometrics and science mapping analysis. Comput Human Behav 140:107566. https://doi.org/10.1016/j.chb.2022.107566
Article Google Scholar
Ahmad A, Mozelius P (2022) Human-Computer Interaction for Older Adults: a Literature Review on Technology Acceptance of eHealth Systems. J Eng Res Sci 1(4):119–126. https://doi.org/10.55708/js0104014
Ale Ebrahim N, Salehi H, Embi MA, Habibi F, Gholizadeh H, Motahar SM (2014) Visibility and citation impact. Int Educ Stud 7(4):120–125. https://doi.org/10.5539/ies.v7n4p120
Amin MS, Johnson VL, Prybutok V, Koh CE (2024) An investigation into factors affecting the willingness to disclose personal health information when using AI-enabled caregiver robots. Ind Manag Data Syst 124(4):1677–1699. https://doi.org/10.1108/IMDS-09-2023-0608
Baer NR, Vietzke J, Schenk L (2022) Middle-aged and older adults’ acceptance of mobile nutrition and fitness apps: a systematic mixed studies review. PLoS One 17(12):e0278879. https://doi.org/10.1371/journal.pone.0278879
Barnard Y, Bradley MD, Hodgson F, Lloyd AD (2013) Learning to use new technologies by older adults: Perceived difficulties, experimentation behaviour and usability. Comput Human Behav 29(4):1715–1724. https://doi.org/10.1016/j.chb.2013.02.006
Berkowsky RW, Sharit J, Czaja SJ (2017) Factors predicting decisions about technology adoption among older adults. Innov Aging 3(1):igy002. https://doi.org/10.1093/geroni/igy002
Braun MT (2013) Obstacles to social networking website use among older adults. Comput Human Behav 29(3):673–680. https://doi.org/10.1016/j.chb.2012.12.004
Article MathSciNet Google Scholar
Campo-Prieto P, Rodríguez-Fuentes G, Cancela-Carral JM (2021) Immersive virtual reality exergame promotes the practice of physical activity in older people: An opportunity during COVID-19. Multimodal Technol Interact 5(9):52. https://doi.org/10.3390/mti5090052
Chen C (2006) CiteSpace II: Detecting and visualizing emerging trends and transient patterns in scientific literature. J Am Soc Inf Sci Technol 57(3):359–377. https://doi.org/10.1002/asi.20317
Chen C, Dubin R, Kim MC (2014) Emerging trends and new developments in regenerative medicine: a scientometric update (2000–2014). Expert Opin Biol Ther 14(9):1295–1317. https://doi.org/10.1517/14712598.2014.920813
Article PubMed Google Scholar
Chen C, Leydesdorff L (2014) Patterns of connections and movements in dual‐map overlays: A new method of publication portfolio analysis. J Assoc Inf Sci Technol 65(2):334–351. https://doi.org/10.1002/asi.22968
Chen J, Wang C, Tang Y (2022) Knowledge mapping of volunteer motivation: A bibliometric analysis and cross-cultural comparative study. Front Psychol 13:883150. https://doi.org/10.3389/fpsyg.2022.883150
Chen JY, Liu YD, Dai J, Wang CL (2023) Development and status of moral education research: Visual analysis based on knowledge graph. Front Psychol 13:1079955. https://doi.org/10.3389/fpsyg.2022.1079955
Chen K, Chan AH (2011) A review of technology acceptance by older adults. Gerontechnology 10(1):1–12. https://doi.org/10.4017/gt.2011.10.01.006.00
Chen K, Chan AH (2014) Gerontechnology acceptance by elderly Hong Kong Chinese: a senior technology acceptance model (STAM). Ergonomics 57(5):635–652. https://doi.org/10.1080/00140139.2014.895855
Chen K, Zhang Y, Fu X (2019) International research collaboration: An emerging domain of innovation studies? Res Policy 48(1):149–168. https://doi.org/10.1016/j.respol.2018.08.005
Chen X, Hu Z, Wang C (2024) Empowering education development through AIGC: A systematic literature review. Educ Inf Technol 1–53. https://doi.org/10.1007/s10639-024-12549-7
Chen Y, Chen CM, Liu ZY, Hu ZG, Wang XW (2015) The methodology function of CiteSpace mapping knowledge domains. Stud Sci Sci 33(2):242–253. https://doi.org/10.16192/j.cnki.1003-2053.2015.02.009
Codfrey GS, Baharum A, Zain NHM, Omar M, Deris FD (2022) User Experience in Product Design and Development: Perspectives and Strategies. Math Stat Eng Appl 71(2):257–262. https://doi.org/10.17762/msea.v71i2.83
Dai J, Zhang X, Wang CL (2024) A meta-analysis of learners’ continuance intention toward online education platforms. Educ Inf Technol 1–36. https://doi.org/10.1007/s10639-024-12654-7
Davis FD (1989) Perceived usefulness, perceived ease of use, and user acceptance of information technology. MIS Q 13(3):319–340. https://doi.org/10.2307/249008
Delmastro F, Dolciotti C, Palumbo F, Magrini M, Di Martino F, La Rosa D, Barcaro U (2018) Long-term care: how to improve the quality of life with mobile and e-health services. In 2018 14th International Conference on Wireless and Mobile Computing, Networking and Communications (WiMob), pp. 12–19. IEEE. https://doi.org/10.1109/WiMOB.2018.8589157
Dupuis K, Tsotsos LE (2018) Technology for remote health monitoring in an older population: a role for mobile devices. Multimodal Technol Interact 2(3):43. https://doi.org/10.3390/mti2030043
Ferguson C, Hickman LD, Turkmani S, Breen P, Gargiulo G, Inglis SC (2021) Wearables only work on patients that wear them”: Barriers and facilitators to the adoption of wearable cardiac monitoring technologies. Cardiovasc Digit Health J 2(2):137–147. https://doi.org/10.1016/j.cvdhj.2021.02.001
Fisk AD, Czaja SJ, Rogers WA, Charness N, Sharit J (2020) Designing for older adults: Principles and creative human factors approaches. CRC Press. https://doi.org/10.1201/9781420080681
Friesen S, Brémault-Phillips S, Rudrum L, Rogers LG (2016) Environmental design that supports healthy aging: Evaluating a new supportive living facility. J Hous Elderly 30(1):18–34. https://doi.org/10.1080/02763893.2015.1129380
Garcia Reyes EP, Kelly R, Buchanan G, Waycott J (2023) Understanding Older Adults’ Experiences With Technologies for Health Self-management: Interview Study. JMIR Aging 6:e43197. https://doi.org/10.2196/43197
Geng Z, Wang J, Liu J, Miao J (2024) Bibliometric analysis of the development, current status, and trends in adult degenerative scoliosis research: A systematic review from 1998 to 2023. J Pain Res 17:153–169. https://doi.org/10.2147/JPR.S437575
González A, Ramírez MP, Viadel V (2012) Attitudes of the elderly toward information and communications technologies. Educ Gerontol 38(9):585–594. https://doi.org/10.1080/03601277.2011.595314
Guner H, Acarturk C (2020) The use and acceptance of ICT by senior citizens: a comparison of technology acceptance model (TAM) for elderly and young adults. Univ Access Inf Soc 19(2):311–330. https://doi.org/10.1007/s10209-018-0642-4
Halim I, Saptari A, Perumal PA, Abdullah Z, Abdullah S, Muhammad MN (2022) A Review on Usability and User Experience of Assistive Social Robots for Older Persons. Int J Integr Eng 14(6):102–124. https://penerbit.uthm.edu.my/ojs/index.php/ijie/article/view/8566
He Y, He Q, Liu Q (2022) Technology acceptance in socially assistive robots: Scoping review of models, measurement, and influencing factors. J Healthc Eng 2022(1):6334732. https://doi.org/10.1155/2022/6334732
Heerink M, Kröse B, Evers V, Wielinga B (2010) Assessing acceptance of assistive social agent technology by older adults: the almere model. Int J Soc Robot 2:361–375. https://doi.org/10.1007/s12369-010-0068-5
Ho A (2020) Are we ready for artificial intelligence health monitoring in elder care? BMC Geriatr 20(1):358. https://doi.org/10.1186/s12877-020-01764-9
Hoque R, Sorwar G (2017) Understanding factors influencing the adoption of mHealth by the elderly: An extension of the UTAUT model. Int J Med Inform 101:75–84. https://doi.org/10.1016/j.ijmedinf.2017.02.002
Hota PK, Subramanian B, Narayanamurthy G (2020) Mapping the intellectual structure of social entrepreneurship research: A citation/co-citation analysis. J Bus Ethics 166(1):89–114. https://doi.org/10.1007/s10551-019-04129-4
Huang R, Yan P, Yang X (2021) Knowledge map visualization of technology hotspots and development trends in China’s textile manufacturing industry. IET Collab Intell Manuf 3(3):243–251. https://doi.org/10.1049/cim2.12024
Article ADS Google Scholar
Jing Y, Wang C, Chen Y, Wang H, Yu T, Shadiev R (2023) Bibliometric mapping techniques in educational technology research: A systematic literature review. Educ Inf Technol 1–29. https://doi.org/10.1007/s10639-023-12178-6
Jing YH, Wang CL, Chen ZY, Shen SS, Shadiev R (2024a) A Bibliometric Analysis of Studies on Technology-Supported Learning Environments: Hotopics and Frontier Evolution. J Comput Assist Learn 1–16. https://doi.org/10.1111/jcal.12934
Jing YH, Wang HM, Chen XJ, Wang CL (2024b) What factors will affect the effectiveness of using ChatGPT to solve programming problems? A quasi-experimental study. Humanit Soc Sci Commun 11:319. https://doi.org/10.1057/s41599-024-02751-w
Kamrani P, Dorsch I, Stock WG (2021) Do researchers know what the h-index is? And how do they estimate its importance? Scientometrics 126(7):5489–5508. https://doi.org/10.1007/s11192-021-03968-1
Kim HS, Lee KH, Kim H, Kim JH (2014) Using mobile phones in healthcare management for the elderly. Maturitas 79(4):381–388. https://doi.org/10.1016/j.maturitas.2014.08.013
Article MathSciNet PubMed Google Scholar
Kleinberg J (2002) Bursty and hierarchical structure in streams. In Proceedings of the eighth ACM SIGKDD international conference on Knowledge discovery and data mining, pp. 91–101. https://doi.org/10.1145/775047.775061
Kruse C, Fohn J, Wilson N, Patlan EN, Zipp S, Mileski M (2020) Utilization barriers and medical outcomes commensurate with the use of telehealth among older adults: systematic review. JMIR Med Inform 8(8):e20359. https://doi.org/10.2196/20359
Kumar S, Lim WM, Pandey N, Christopher Westland J (2021) 20 years of electronic commerce research. Electron Commer Res 21:1–40. https://doi.org/10.1007/s10660-021-09464-1
Kwiek M (2021) What large-scale publication and citation data tell us about international research collaboration in Europe: Changing national patterns in global contexts. Stud High Educ 46(12):2629–2649. https://doi.org/10.1080/03075079.2020.1749254
Lee C, Coughlin JF (2015) PERSPECTIVE: Older adults’ adoption of technology: an integrated approach to identifying determinants and barriers. J Prod Innov Manag 32(5):747–759. https://doi.org/10.1111/jpim.12176
Lee CH, Wang C, Fan X, Li F, Chen CH (2023) Artificial intelligence-enabled digital transformation in elderly healthcare field: scoping review. Adv Eng Inform 55:101874. https://doi.org/10.1016/j.aei.2023.101874
Leydesdorff L, Rafols I (2012) Interactive overlays: A new method for generating global journal maps from Web-of-Science data. J Informetr 6(2):318–332. https://doi.org/10.1016/j.joi.2011.11.003
Li J, Ma Q, Chan AH, Man S (2019) Health monitoring through wearable technologies for older adults: Smart wearables acceptance model. Appl Ergon 75:162–169. https://doi.org/10.1016/j.apergo.2018.10.006
Article ADS PubMed Google Scholar
Li X, Zhou D (2020) Product design requirement information visualization approach for intelligent manufacturing services. China Mech Eng 31(07):871, http://www.cmemo.org.cn/EN/Y2020/V31/I07/871
Google Scholar
Lin Y, Yu Z (2024a) An integrated bibliometric analysis and systematic review modelling students’ technostress in higher education. Behav Inf Technol 1–25. https://doi.org/10.1080/0144929X.2024.2332458
Lin Y, Yu Z (2024b) A bibliometric analysis of artificial intelligence chatbots in educational contexts. Interact Technol Smart Educ 21(2):189–213. https://doi.org/10.1108/ITSE-12-2022-0165
Liu L, Duffy VG (2023) Exploring the future development of Artificial Intelligence (AI) applications in chatbots: a bibliometric analysis. Int J Soc Robot 15(5):703–716. https://doi.org/10.1007/s12369-022-00956-0
Liu R, Li X, Chu J (2022) Evolution of applied variables in the research on technology acceptance of the elderly. In: International Conference on Human-Computer Interaction, Cham: Springer International Publishing, pp 500–520. https://doi.org/10.1007/978-3-031-05581-23_5
Luijkx K, Peek S, Wouters E (2015) “Grandma, you should do it—It’s cool” Older Adults and the Role of Family Members in Their Acceptance of Technology. Int J Environ Res Public Health 12(12):15470–15485. https://doi.org/10.3390/ijerph121214999
Lussier M, Lavoie M, Giroux S, Consel C, Guay M, Macoir J, Bier N (2018) Early detection of mild cognitive impairment with in-home monitoring sensor technologies using functional measures: a systematic review. IEEE J Biomed Health Inform 23(2):838–847. https://doi.org/10.1109/JBHI.2018.2834317
López-Robles JR, Otegi-Olaso JR, Porto Gomez I, Gamboa-Rosales NK, Gamboa-Rosales H, Robles-Berumen H (2018) Bibliometric network analysis to identify the intellectual structure and evolution of the big data research field. In: International Conference on Intelligent Data Engineering and Automated Learning, Cham: Springer International Publishing, pp 113–120. https://doi.org/10.1007/978-3-030-03496-2_13
Ma Q, Chan AH, Chen K (2016) Personal and other factors affecting acceptance of smartphone technology by older Chinese adults. Appl Ergon 54:62–71. https://doi.org/10.1016/j.apergo.2015.11.015
Ma Q, Chan AHS, Teh PL (2021) Insights into Older Adults’ Technology Acceptance through Meta-Analysis. Int J Hum-Comput Interact 37(11):1049–1062. https://doi.org/10.1080/10447318.2020.1865005
Macedo IM (2017) Predicting the acceptance and use of information and communication technology by older adults: An empirical examination of the revised UTAUT2. Comput Human Behav 75:935–948. https://doi.org/10.1016/j.chb.2017.06.013
Maidhof C, Offermann J, Ziefle M (2023) Eyes on privacy: acceptance of video-based AAL impacted by activities being filmed. Front Public Health 11:1186944. https://doi.org/10.3389/fpubh.2023.1186944
Majumder S, Aghayi E, Noferesti M, Memarzadeh-Tehran H, Mondal T, Pang Z, Deen MJ (2017) Smart homes for elderly healthcare—Recent advances and research challenges. Sensors 17(11):2496. https://doi.org/10.3390/s17112496
Article ADS PubMed PubMed Central Google Scholar
Mhlanga D (2023) Artificial Intelligence in elderly care: Navigating ethical and responsible AI adoption for seniors. Available at SSRN 4675564. 4675564 min) Identifying citation patterns of scientific breakthroughs: A perspective of dynamic citation process. Inf Process Manag 58(1):102428. https://doi.org/10.1016/j.ipm.2020.102428
Mitzner TL, Boron JB, Fausset CB, Adams AE, Charness N, Czaja SJ, Sharit J (2010) Older adults talk technology: Technology usage and attitudes. Comput Human Behav 26(6):1710–1721. https://doi.org/10.1016/j.chb.2010.06.020
Mitzner TL, Savla J, Boot WR, Sharit J, Charness N, Czaja SJ, Rogers WA (2019) Technology adoption by older adults: Findings from the PRISM trial. Gerontologist 59(1):34–44. https://doi.org/10.1093/geront/gny113
Mongeon P, Paul-Hus A (2016) The journal coverage of Web of Science and Scopus: a comparative analysis. Scientometrics 106:213–228. https://doi.org/10.1007/s11192-015-1765-5
Mostaghel R (2016) Innovation and technology for the elderly: Systematic literature review. J Bus Res 69(11):4896–4900. https://doi.org/10.1016/j.jbusres.2016.04.049
Mujirishvili T, Maidhof C, Florez-Revuelta F, Ziefle M, Richart-Martinez M, Cabrero-García J (2023) Acceptance and privacy perceptions toward video-based active and assisted living technologies: Scoping review. J Med Internet Res 25:e45297. https://doi.org/10.2196/45297
Naseri RNN, Azis SN, Abas N (2023) A Review of Technology Acceptance and Adoption Models in Consumer Study. FIRM J Manage Stud 8(2):188–199. https://doi.org/10.33021/firm.v8i2.4536
Nguyen UP, Hallinger P (2020) Assessing the distinctive contributions of Simulation & Gaming to the literature, 1970–2019: A bibliometric review. Simul Gaming 51(6):744–769. https://doi.org/10.1177/1046878120941569
Olmedo-Aguirre JO, Reyes-Campos J, Alor-Hernández G, Machorro-Cano I, Rodríguez-Mazahua L, Sánchez-Cervantes JL (2022) Remote healthcare for elderly people using wearables: A review. Biosensors 12(2):73. https://doi.org/10.3390/bios12020073
Pan S, Jordan-Marsh M (2010) Internet use intention and adoption among Chinese older adults: From the expanded technology acceptance model perspective. Comput Human Behav 26(5):1111–1119. https://doi.org/10.1016/j.chb.2010.03.015
Pan X, Yan E, Cui M, Hua W (2018) Examining the usage, citation, and diffusion patterns of bibliometric map software: A comparative study of three tools. J Informetr 12(2):481–493. https://doi.org/10.1016/j.joi.2018.03.005
Park JS, Kim NR, Han EJ (2018) Analysis of trends in science and technology using keyword network analysis. J Korea Ind Inf Syst Res 23(2):63–73. https://doi.org/10.9723/jksiis.2018.23.2.063
Peek ST, Luijkx KG, Rijnaard MD, Nieboer ME, Van Der Voort CS, Aarts S, Wouters EJ (2016) Older adults’ reasons for using technology while aging in place. Gerontology 62(2):226–237. https://doi.org/10.1159/000430949
Peek ST, Luijkx KG, Vrijhoef HJ, Nieboer ME, Aarts S, van der Voort CS, Wouters EJ (2017) Origins and consequences of technology acquirement by independent-living seniors: Towards an integrative model. BMC Geriatr 17:1–18. https://doi.org/10.1186/s12877-017-0582-5
Peek ST, Wouters EJ, Van Hoof J, Luijkx KG, Boeije HR, Vrijhoef HJ (2014) Factors influencing acceptance of technology for aging in place: a systematic review. Int J Med Inform 83(4):235–248. https://doi.org/10.1016/j.ijmedinf.2014.01.004
Peek STM, Luijkx KG, Vrijhoef HJM, Nieboer ME, Aarts S, Van Der Voort CS, Wouters EJM (2019) Understanding changes and stability in the long-term use of technologies by seniors who are aging in place: a dynamical framework. BMC Geriatr 19:1–13. https://doi.org/10.1186/s12877-019-1241-9
Perez AJ, Siddiqui F, Zeadally S, Lane D (2023) A review of IoT systems to enable independence for the elderly and disabled individuals. Internet Things 21:100653. https://doi.org/10.1016/j.iot.2022.100653
Piau A, Wild K, Mattek N, Kaye J (2019) Current state of digital biomarker technologies for real-life, home-based monitoring of cognitive function for mild cognitive impairment to mild Alzheimer disease and implications for clinical care: systematic review. J Med Internet Res 21(8):e12785. https://doi.org/10.2196/12785
Pirzada P, Wilde A, Doherty GH, Harris-Birtill D (2022) Ethics and acceptance of smart homes for older adults. Inform Health Soc Care 47(1):10–37. https://doi.org/10.1080/17538157.2021.1923500
Pranckutė R (2021) Web of Science (WoS) and Scopus: The titans of bibliographic information in today’s academic world. Publications 9(1):12. https://doi.org/10.3390/publications9010012
Qian K, Zhang Z, Yamamoto Y, Schuller BW (2021) Artificial intelligence internet of things for the elderly: From assisted living to health-care monitoring. IEEE Signal Process Mag 38(4):78–88. https://doi.org/10.1109/MSP.2021.3057298
Redner S (1998) How popular is your paper? An empirical study of the citation distribution. Eur Phys J B-Condens Matter Complex Syst 4(2):131–134. https://doi.org/10.1007/s100510050359
Sayago S (ed.) (2019) Perspectives on human-computer interaction research with older people. Switzerland: Springer International Publishing. https://doi.org/10.1007/978-3-030-06076-3
Schomakers EM, Ziefle M (2023) Privacy vs. security: trade-offs in the acceptance of smart technologies for aging-in-place. Int J Hum Comput Interact 39(5):1043–1058. https://doi.org/10.1080/10447318.2022.2078463
Schroeder T, Dodds L, Georgiou A, Gewald H, Siette J (2023) Older adults and new technology: Mapping review of the factors associated with older adults’ intention to adopt digital technologies. JMIR Aging 6(1):e44564. https://doi.org/10.2196/44564
Seibert K, Domhoff D, Bruch D, Schulte-Althoff M, Fürstenau D, Biessmann F, Wolf-Ostermann K (2021) Application scenarios for artificial intelligence in nursing care: rapid review. J Med Internet Res 23(11):e26522. https://doi.org/10.2196/26522
Seuwou P, Banissi E, Ubakanma G (2016) User acceptance of information technology: A critical review of technology acceptance models and the decision to invest in Information Security. In: Global Security, Safety and Sustainability-The Security Challenges of the Connected World: 11th International Conference, ICGS3 2017, London, UK, January 18-20, 2017, Proceedings 11:230-251. Springer International Publishing. https://doi.org/10.1007/978-3-319-51064-4_19
Shiau WL, Wang X, Zheng F (2023) What are the trend and core knowledge of information security? A citation and co-citation analysis. Inf Manag 60(3):103774. https://doi.org/10.1016/j.im.2023.103774
Sinha S, Verma A, Tiwari P (2021) Technology: Saving and enriching life during COVID-19. Front Psychol 12:647681. https://doi.org/10.3389/fpsyg.2021.647681
Soar J (2010) The potential of information and communication technologies to support ageing and independent living. Ann Telecommun 65:479–483. https://doi.org/10.1007/s12243-010-0167-1
Strotmann A, Zhao D (2012) Author name disambiguation: What difference does it make in author‐based citation analysis? J Am Soc Inf Sci Technol 63(9):1820–1833. https://doi.org/10.1002/asi.22695
Talukder MS, Sorwar G, Bao Y, Ahmed JU, Palash MAS (2020) Predicting antecedents of wearable healthcare technology acceptance by elderly: A combined SEM-Neural Network approach. Technol Forecast Soc Change 150:119793. https://doi.org/10.1016/j.techfore.2019.119793
Taskin Z, Al U (2019) Natural language processing applications in library and information science. Online Inf Rev 43(4):676–690. https://doi.org/10.1108/oir-07-2018-0217
Touqeer H, Zaman S, Amin R, Hussain M, Al-Turjman F, Bilal M (2021) Smart home security: challenges, issues and solutions at different IoT layers. J Supercomput 77(12):14053–14089. https://doi.org/10.1007/s11227-021-03825-1
United Nations Department of Economic and Social Affairs (2023) World population ageing 2023: Highlights. https://www.un.org/zh/193220
Valk CAL, Lu Y, Randriambelonoro M, Jessen J (2018) Designing for technology acceptance of wearable and mobile technologies for senior citizen users. In: 21st DMI: Academic Design Management Conference (ADMC 2018), Design Management Institute, pp 1361–1373. https://www.dmi.org/page/ADMC2018
Van Eck N, Waltman L (2010) Software survey: VOSviewer, a computer program for bibliometric mapping. Scientometrics 84(2):523–538. https://doi.org/10.1007/s11192-009-0146-3
Vancea M, Solé-Casals J (2016) Population aging in the European Information Societies: towards a comprehensive research agenda in eHealth innovations for elderly. Aging Dis 7(4):526. https://doi.org/10.14336/AD.2015.1214
Venkatesh V, Morris MG, Davis GB, Davis FD (2003) User acceptance of information technology: Toward a unified view. MIS Q 27(3):425–478. https://doi.org/10.2307/30036540
Wagner N, Hassanein K, Head M (2010) Computer use by older adults: A multi-disciplinary review. Comput Human Behav 26(5):870–882. https://doi.org/10.1016/j.chb.2010.03.029
Wahlroos N, Narsakka N, Stolt M, Suhonen R (2023) Physical environment maintaining independence and self-management of older people in long-term care settings—An integrative literature review. J Aging Environ 37(3):295–313. https://doi.org/10.1080/26892618.2022.2092927
Wang CL, Chen XJ, Yu T, Liu YD, Jing YH (2024a) Education reform and change driven by digital technology: a bibliometric study from a global perspective. Humanit Soc Sci Commun 11(1):1–17. https://doi.org/10.1057/s41599-024-02717-y
Wang CL, Dai J, Zhu KK, Yu T, Gu XQ (2023a) Understanding the Continuance Intention of College Students Toward New E-learning Spaces Based on an Integrated Model of the TAM and TTF. Int J Hum-comput Int 1–14. https://doi.org/10.1080/10447318.2023.2291609
Wang CL, Wang HM, Li YY, Dai J, Gu XQ, Yu T (2024b) Factors Influencing University Students’ Behavioral Intention to Use Generative Artificial Intelligence: Integrating the Theory of Planned Behavior and AI Literacy. Int J Hum-comput Int 1–23. https://doi.org/10.1080/10447318.2024.2383033
Wang J, Zhao W, Zhang Z, Liu X, Xie T, Wang L, Zhang Y (2024c) A journey of challenges and victories: a bibliometric worldview of nanomedicine since the 21st century. Adv Mater 36(15):2308915. https://doi.org/10.1002/adma.202308915
Wang J, Chen Y, Huo S, Mai L, Jia F (2023b) Research hotspots and trends of social robot interaction design: A bibliometric analysis. Sensors 23(23):9369. https://doi.org/10.3390/s23239369
Wang KH, Chen G, Chen HG (2017) A model of technology adoption by older adults. Soc Behav Personal 45(4):563–572. https://doi.org/10.2224/sbp.5778
Wang S, Bolling K, Mao W, Reichstadt J, Jeste D, Kim HC, Nebeker C (2019) Technology to Support Aging in Place: Older Adults’ Perspectives. Healthcare 7(2):60. https://doi.org/10.3390/healthcare7020060
Wang Z, Liu D, Sun Y, Pang X, Sun P, Lin F, Ren K (2022) A survey on IoT-enabled home automation systems: Attacks and defenses. IEEE Commun Surv Tutor 24(4):2292–2328. https://doi.org/10.1109/COMST.2022.3201557
Wilkowska W, Offermann J, Spinsante S, Poli A, Ziefle M (2022) Analyzing technology acceptance and perception of privacy in ambient assisted living for using sensor-based technologies. PloS One 17(7):e0269642. https://doi.org/10.1371/journal.pone.0269642
Wilson J, Heinsch M, Betts D, Booth D, Kay-Lambkin F (2021) Barriers and facilitators to the use of e-health by older adults: a scoping review. BMC Public Health 21:1–12. https://doi.org/10.1186/s12889-021-11623-w
Xia YQ, Deng YL, Tao XY, Zhang SN, Wang CL (2024) Digital art exhibitions and psychological well-being in Chinese Generation Z: An analysis based on the S-O-R framework. Humanit Soc Sci Commun 11:266. https://doi.org/10.1057/s41599-024-02718-x
Xie H, Zhang Y, Duan K (2020) Evolutionary overview of urban expansion based on bibliometric analysis in Web of Science from 1990 to 2019. Habitat Int 95:102100. https://doi.org/10.1016/j.habitatint.2019.10210
Xu Z, Ge Z, Wang X, Skare M (2021) Bibliometric analysis of technology adoption literature published from 1997 to 2020. Technol Forecast Soc Change 170:120896. https://doi.org/10.1016/j.techfore.2021.120896
Yap YY, Tan SH, Choon SW (2022) Elderly’s intention to use technologies: a systematic literature review. Heliyon 8(1). https://doi.org/10.1016/j.heliyon.2022.e08765
Yu T, Dai J, Wang CL (2023) Adoption of blended learning: Chinese university students’ perspectives. Humanit Soc Sci Commun 10:390. https://doi.org/10.1057/s41599-023-01904-7
Yusif S, Soar J, Hafeez-Baig A (2016) Older people, assistive technologies, and the barriers to adoption: A systematic review. Int J Med Inform 94:112–116. https://doi.org/10.1016/j.ijmedinf.2016.07.004
Zhang J, Zhu L (2022) Citation recommendation using semantic representation of cited papers’ relations and content. Expert Syst Appl 187:115826. https://doi.org/10.1016/j.eswa.2021.115826
Zhao Y, Li J (2024) Opportunities and challenges of integrating artificial intelligence in China’s elderly care services. Sci Rep 14(1):9254. https://doi.org/10.1038/s41598-024-60067-w
Article ADS MathSciNet PubMed PubMed Central Google Scholar
Download references
Acknowledgements
This research was supported by the Social Science Foundation of Shaanxi Province in China (Grant No. 2023J014).
Author information
Authors and affiliations.
School of Art and Design, Shaanxi University of Science and Technology, Xi’an, China
Xianru Shang, Zijian Liu, Chen Gong, Zhigang Hu & Yuexuan Wu
Department of Education Information Technology, Faculty of Education, East China Normal University, Shanghai, China
Chengliang Wang
You can also search for this author in PubMed Google Scholar
Contributions
Conceptualization, XS, YW, CW; methodology, XS, ZL, CG, CW; software, XS, CG, YW; writing-original draft preparation, XS, CW; writing-review and editing, XS, CG, ZH, CW; supervision, ZL, ZH, CW; project administration, ZL, ZH, CW; funding acquisition, XS, CG. All authors read and approved the final manuscript. All authors have read and approved the re-submission of the manuscript.
Corresponding author
Correspondence to Chengliang Wang .
Ethics declarations
Competing interests.
The authors declare no competing interests.
Ethical approval
Ethical approval was not required as the study did not involve human participants.
Informed consent
Informed consent was not required as the study did not involve human participants.
Additional information
Publisher’s note Springer Nature remains neutral with regard to jurisdictional claims in published maps and institutional affiliations.
Rights and permissions
Open Access This article is licensed under a Creative Commons Attribution-NonCommercial-NoDerivatives 4.0 International License, which permits any non-commercial use, sharing, distribution and reproduction in any medium or format, as long as you give appropriate credit to the original author(s) and the source, provide a link to the Creative Commons licence, and indicate if you modified the licensed material. You do not have permission under this licence to share adapted material derived from this article or parts of it. The images or other third party material in this article are included in the article’s Creative Commons licence, unless indicated otherwise in a credit line to the material. If material is not included in the article’s Creative Commons licence and your intended use is not permitted by statutory regulation or exceeds the permitted use, you will need to obtain permission directly from the copyright holder. To view a copy of this licence, visit http://creativecommons.org/licenses/by-nc-nd/4.0/ .
Reprints and permissions
About this article
Cite this article.
Shang, X., Liu, Z., Gong, C. et al. Knowledge mapping and evolution of research on older adults’ technology acceptance: a bibliometric study from 2013 to 2023. Humanit Soc Sci Commun 11 , 1115 (2024). https://doi.org/10.1057/s41599-024-03658-2
Download citation
Received : 20 June 2024
Accepted : 21 August 2024
Published : 31 August 2024
DOI : https://doi.org/10.1057/s41599-024-03658-2
Share this article
Anyone you share the following link with will be able to read this content:
Sorry, a shareable link is not currently available for this article.
Provided by the Springer Nature SharedIt content-sharing initiative
Quick links
- Explore articles by subject
- Guide to authors
- Editorial policies

- DOI: 10.37075/rp.2021.5.01
- Corpus ID: 245194093
Theoretical Foundations of the First Purchase in Marketing
- Published in Research Papers 10 December 2021
- Research Papers
Related Papers
Showing 1 through 3 of 0 Related Papers

IMAGES
VIDEO
COMMENTS
About the journal. Journal of Semantics covers all areas in the study of meaning, with a focus on formal and experimental methods. It welcomes submissions on semantics, pragmatics, the syntax/semantics interface, cross-linguistic semantics, experimental studies of meaning, and semantically informed philosophy of language. Find out more.
What Is Semantic Scholar? Semantic Scholar is a free, AI-powered research tool for scientific literature, based at Ai2. Semantic Scholar uses groundbreaking AI and engineering to understand the semantics of scientific literature to help Scholars discover relevant research.
Semantics and Pragmatics, founded in 2007 and first published in 2008, is a Diamond Open Access journal published by the Linguistic Society of America. Current Issue Vol. 17 (2024) Published: 2024-01-05 Main Articles. The semantics and probabilistic pragmatics of deadjectival intensifiers Rick Nouwen 2:1-45 ...
Explore the latest full-text research PDFs, articles, conference papers, preprints and more on SEMANTICS. Find methods information, sources, references or conduct a literature review on SEMANTICS
The current special issue of Axiomathes is intended to address variety of foundational issues in semantics and pragmatics. The idea of the special issue has its origins at the second Context, Cognition and Communication conference held at the University of Warsaw in June 2018. After the conference we have decided to invite the conference participants to submit their papers for the special ...
Summarily, the report has been a critical review of over 12 published research papers on semantic analysis [13, 22, 30, 42,43,44,45,46,47,48,49,50]. Some papers have been scanned quickly to check the relevance of the topic and the interesting findings revealed by the authors.
The Routledge Handbook of Semantics provides a broad and state-of-the-art survey of this field, covering semantic research at both word and sentence level. It presents a synoptic view of the most important areas of semantic investigation, including contemporary methodologies and debates, and indicating possible future directions in the field.
The Stanford semantics and pragmatics community encompasses a broad range of interests including: Lexical semantics. Formal semantics and pragmatics, and their interfaces with syntax. Psycholinguistics. Numerous sub-areas of psychology, philosophy, and computer science. We share the goal of grounding theories of meaning in diverse research ...
Abstract. Understanding semantics of data on the Web and thus enabling meaningful processing of it has been at the core of Semantic Web research for over the past decade and a half. The early promise of enabling software agents on the Web to talk to one another in a meaningful way spawned research in a number of areas and has been adopted by ...
semantics (Pires de Oliveira et al, 2020). Contemporary Issues in Syntax. and Semantics, the theme of the 3 EISSI, was the open call for paper. answered by all the papers in this volume. For this ...
Topics in Semantics and Pragmatics. Topics in Semantics and Pragmatics. This course will provide a comprehensive overview of the empirical patterns, analytical challenges and broader theoretical issues surrounding a particular topic, such as information structure, presupposition, scalar implicature, binding, aspectual composition, nominal ...
PDF | The study of Semantics is an important area of word meaning, references, senses, logic, and perlocutions and illocutions. ... to write a 5-6-page research paper on a topic that they think i ...
8.1.2 Developing conceptual semantics analyses 265 8.1.3 Problems with conceptual semantics 269 8.2 Semantics and computation 270 8.2.1 The lexicon in computational linguistics 272 8.2.2 Word sense disambiguation 277 8.2.3 Pustejovskian semantics 280 Summary 283 Further reading 284 Exercises 285 9Meaning and morphosyntax I: the semantics
The birth of dynamic semantics: File Change Potentials. Week 4 2/16 Harvard Chierchia "Standard" Dynamic Semantics of the 90's. Indefinites as Dynamic Generalized Quantifiers, weak and strong readings of donkey pronouns, existential disclosure. Week 7 3/9 MIT Chierchia The debate on long distance indefinites: Non canonical scope ...
As text semantics has an important role in text meaning, the term semantics has been seen in a vast sort of text mining studies. However, there is a lack of studies that integrate the different research branches and summarize the developed works. This paper reports a systematic mapping about semantics-concerned text mining studies. This systematic mapping study followed a well-defined protocol.
Summary. Lexical semantics is the study of word meaning. Descriptively speaking, the main topics studied within lexical semantics involve either the internal semantic structure of words, or the semantic relations that occur within the vocabulary. Within the first set, major phenomena include polysemy (in contrast with vagueness), metonymy ...
Search 220,445,154 papers from all fields of science. Search. Sign In Create Free Account. Stay Connected With Semantic Scholar. Sign Up. What Is Semantic Scholar? Semantic Scholar is a free, AI-powered research tool for scientific literature, based at Ai2. ... Research Publications Researchers Research Careers Prototypes Resources. Help
First, we analyze semantic web and those domains that increase the growth of the semantic web. Second, we discuss all domains where semantic web technologies play a vital role. Third, we emphasize those domains that go hand in hand with semantic web technologies. This review paper will be utilized as an unbiased direction for the researchers.
This document discusses writing a research paper on semantics and provides guidance on choosing a topic. It notes that crafting a thesis in semantics requires extensive research into language meaning and linguistic structures. However, navigating semantic theories can feel overwhelming for students. The organization aims to help students at every step of writing a thesis, from narrowing a ...
Semantic image segmentation (SiS) plays a fundamental role in a broad variety of computer vision applications, providing key information for the global understanding of an image. This survey is an effort to summarize two decades of research in the field of SiS, where we propose a literature review of solutions starting from early historical methods followed by an overview of more recent deep ...
Experience a smarter way to search and discover scholarly research. Create Your Account. Semantic Scholar provides free, AI-driven research tools and open resources for all researchers. Search and cite any papers, manage your reading lists in your personal library, and get AI-powered paper recommendations just for you.
Research method. In recent years, bibliometrics has become one of the crucial methods for analyzing literature reviews and is widely used in disciplinary and industrial intelligence analysis (Jing ...
Consumer decision making as an important process in marketing sphere has been discussed in detail, but so far researchers as a rule have not focused the attention on how the purchase happens for the very first time. The current text is an attempt to develop the foundations and to make a conceptual framework of the first purchase in marketing and to outline its significance for current or ...