Sciencing_Icons_Science SCIENCE
Sciencing_icons_biology biology, sciencing_icons_cells cells, sciencing_icons_molecular molecular, sciencing_icons_microorganisms microorganisms, sciencing_icons_genetics genetics, sciencing_icons_human body human body, sciencing_icons_ecology ecology, sciencing_icons_chemistry chemistry, sciencing_icons_atomic & molecular structure atomic & molecular structure, sciencing_icons_bonds bonds, sciencing_icons_reactions reactions, sciencing_icons_stoichiometry stoichiometry, sciencing_icons_solutions solutions, sciencing_icons_acids & bases acids & bases, sciencing_icons_thermodynamics thermodynamics, sciencing_icons_organic chemistry organic chemistry, sciencing_icons_physics physics, sciencing_icons_fundamentals-physics fundamentals, sciencing_icons_electronics electronics, sciencing_icons_waves waves, sciencing_icons_energy energy, sciencing_icons_fluid fluid, sciencing_icons_astronomy astronomy, sciencing_icons_geology geology, sciencing_icons_fundamentals-geology fundamentals, sciencing_icons_minerals & rocks minerals & rocks, sciencing_icons_earth scructure earth structure, sciencing_icons_fossils fossils, sciencing_icons_natural disasters natural disasters, sciencing_icons_nature nature, sciencing_icons_ecosystems ecosystems, sciencing_icons_environment environment, sciencing_icons_insects insects, sciencing_icons_plants & mushrooms plants & mushrooms, sciencing_icons_animals animals, sciencing_icons_math math, sciencing_icons_arithmetic arithmetic, sciencing_icons_addition & subtraction addition & subtraction, sciencing_icons_multiplication & division multiplication & division, sciencing_icons_decimals decimals, sciencing_icons_fractions fractions, sciencing_icons_conversions conversions, sciencing_icons_algebra algebra, sciencing_icons_working with units working with units, sciencing_icons_equations & expressions equations & expressions, sciencing_icons_ratios & proportions ratios & proportions, sciencing_icons_inequalities inequalities, sciencing_icons_exponents & logarithms exponents & logarithms, sciencing_icons_factorization factorization, sciencing_icons_functions functions, sciencing_icons_linear equations linear equations, sciencing_icons_graphs graphs, sciencing_icons_quadratics quadratics, sciencing_icons_polynomials polynomials, sciencing_icons_geometry geometry, sciencing_icons_fundamentals-geometry fundamentals, sciencing_icons_cartesian cartesian, sciencing_icons_circles circles, sciencing_icons_solids solids, sciencing_icons_trigonometry trigonometry, sciencing_icons_probability-statistics probability & statistics, sciencing_icons_mean-median-mode mean/median/mode, sciencing_icons_independent-dependent variables independent/dependent variables, sciencing_icons_deviation deviation, sciencing_icons_correlation correlation, sciencing_icons_sampling sampling, sciencing_icons_distributions distributions, sciencing_icons_probability probability, sciencing_icons_calculus calculus, sciencing_icons_differentiation-integration differentiation/integration, sciencing_icons_application application, sciencing_icons_projects projects, sciencing_icons_news news.
- Share Tweet Email Print
- Home ⋅
- Math ⋅
- Probability & Statistics ⋅
- Distributions

How to Write a Hypothesis for Correlation
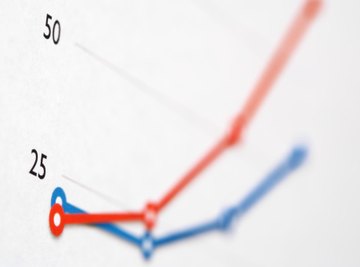
How to Calculate a P-Value
A hypothesis is a testable statement about how something works in the natural world. While some hypotheses predict a causal relationship between two variables, other hypotheses predict a correlation between them. According to the Research Methods Knowledge Base, a correlation is a single number that describes the relationship between two variables. If you do not predict a causal relationship or cannot measure one objectively, state clearly in your hypothesis that you are merely predicting a correlation.
Research the topic in depth before forming a hypothesis. Without adequate knowledge about the subject matter, you will not be able to decide whether to write a hypothesis for correlation or causation. Read the findings of similar experiments before writing your own hypothesis.
Identify the independent variable and dependent variable. Your hypothesis will be concerned with what happens to the dependent variable when a change is made in the independent variable. In a correlation, the two variables undergo changes at the same time in a significant number of cases. However, this does not mean that the change in the independent variable causes the change in the dependent variable.
Construct an experiment to test your hypothesis. In a correlative experiment, you must be able to measure the exact relationship between two variables. This means you will need to find out how often a change occurs in both variables in terms of a specific percentage.
Establish the requirements of the experiment with regard to statistical significance. Instruct readers exactly how often the variables must correlate to reach a high enough level of statistical significance. This number will vary considerably depending on the field. In a highly technical scientific study, for instance, the variables may need to correlate 98 percent of the time; but in a sociological study, 90 percent correlation may suffice. Look at other studies in your particular field to determine the requirements for statistical significance.
State the null hypothesis. The null hypothesis gives an exact value that implies there is no correlation between the two variables. If the results show a percentage equal to or lower than the value of the null hypothesis, then the variables are not proven to correlate.
Record and summarize the results of your experiment. State whether or not the experiment met the minimum requirements of your hypothesis in terms of both percentage and significance.
Related Articles
How to determine the sample size in a quantitative..., how to calculate a two-tailed test, how to interpret a student's t-test results, how to know if something is significant using spss, quantitative vs. qualitative data and laboratory testing, similarities of univariate & multivariate statistical..., what is the meaning of sample size, distinguishing between descriptive & causal studies, how to calculate cv values, how to determine your practice clep score, what are the different types of correlations, how to calculate p-hat, how to calculate percentage error, how to calculate percent relative range, how to calculate a sample size population, how to calculate bias, how to calculate the percentage of another number, how to find y value for the slope of a line, advantages & disadvantages of finding variance.
- University of New England; Steps in Hypothesis Testing for Correlation; 2000
- Research Methods Knowledge Base; Correlation; William M.K. Trochim; 2006
- Science Buddies; Hypothesis
About the Author
Brian Gabriel has been a writer and blogger since 2009, contributing to various online publications. He earned his Bachelor of Arts in history from Whitworth University.
Photo Credits
Thinkstock/Comstock/Getty Images
Find Your Next Great Science Fair Project! GO
psychologyrocks
Hypotheses; directional and non-directional, what is the difference between an experimental and an alternative hypothesis.
Nothing much! If the study is a true experiment then we can call the hypothesis “an experimental hypothesis”, a prediction is made about how the IV causes an effect on the DV. In a study which does not involve the direct manipulation of an IV, i.e. a natural or quasi-experiment or any other quantitative research method (e.g. survey) has been used, then we call it an “alternative hypothesis”, it is the alternative to the null.
Directional hypothesis: A directional (or one-tailed hypothesis) states which way you think the results are going to go, for example in an experimental study we might say…”Participants who have been deprived of sleep for 24 hours will have more cold symptoms the week after exposure to a virus than participants who have not been sleep deprived”; the hypothesis compares the two groups/conditions and states which one will ….have more/less, be quicker/slower, etc.
If we had a correlational study, the directional hypothesis would state whether we expect a positive or a negative correlation, we are stating how the two variables will be related to each other, e.g. there will be a positive correlation between the number of stressful life events experienced in the last year and the number of coughs and colds suffered, whereby the more life events you have suffered the more coughs and cold you will have had”. The directional hypothesis can also state a negative correlation, e.g. the higher the number of face-book friends, the lower the life satisfaction score “
Non-directional hypothesis: A non-directional (or two tailed hypothesis) simply states that there will be a difference between the two groups/conditions but does not say which will be greater/smaller, quicker/slower etc. Using our example above we would say “There will be a difference between the number of cold symptoms experienced in the following week after exposure to a virus for those participants who have been sleep deprived for 24 hours compared with those who have not been sleep deprived for 24 hours.”
When the study is correlational, we simply state that variables will be correlated but do not state whether the relationship will be positive or negative, e.g. there will be a significant correlation between variable A and variable B.
Null hypothesis The null hypothesis states that the alternative or experimental hypothesis is NOT the case, if your experimental hypothesis was directional you would say…
Participants who have been deprived of sleep for 24 hours will NOT have more cold symptoms in the following week after exposure to a virus than participants who have not been sleep deprived and any difference that does arise will be due to chance alone.
or with a directional correlational hypothesis….
There will NOT be a positive correlation between the number of stress life events experienced in the last year and the number of coughs and colds suffered, whereby the more life events you have suffered the more coughs and cold you will have had”
With a non-directional or two tailed hypothesis…
There will be NO difference between the number of cold symptoms experienced in the following week after exposure to a virus for those participants who have been sleep deprived for 24 hours compared with those who have not been sleep deprived for 24 hours.
or for a correlational …
there will be NO correlation between variable A and variable B.
When it comes to conducting an inferential stats test, if you have a directional hypothesis , you must do a one tailed test to find out whether your observed value is significant. If you have a non-directional hypothesis , you must do a two tailed test .
Exam Techniques/Advice
- Remember, a decent hypothesis will contain two variables, in the case of an experimental hypothesis there will be an IV and a DV; in a correlational hypothesis there will be two co-variables
- both variables need to be fully operationalised to score the marks, that is you need to be very clear and specific about what you mean by your IV and your DV; if someone wanted to repeat your study, they should be able to look at your hypothesis and know exactly what to change between the two groups/conditions and exactly what to measure (including any units/explanation of rating scales etc, e.g. “where 1 is low and 7 is high”)
- double check the question, did it ask for a directional or non-directional hypothesis?
- if you were asked for a null hypothesis, make sure you always include the phrase “and any difference/correlation (is your study experimental or correlational?) that does arise will be due to chance alone”
Practice Questions:
- Mr Faraz wants to compare the levels of attendance between his psychology group and those of Mr Simon, who teaches a different psychology group. Which of the following is a suitable directional (one tailed) hypothesis for Mr Faraz’s investigation?
A There will be a difference in the levels of attendance between the two psychology groups.
B Students’ level of attendance will be higher in Mr Faraz’s group than Mr Simon’s group.
C Any difference in the levels of attendance between the two psychology groups is due to chance.
D The level of attendance of the students will depend upon who is teaching the groups.
2. Tracy works for the local council. The council is thinking about reducing the number of people it employs to pick up litter from the street. Tracy has been asked to carry out a study to see if having the streets cleaned at less regular intervals will affect the amount of litter the public will drop. She studies a street to compare how much litter is dropped at two different times, once when it has just been cleaned and once after it has not been cleaned for a month.
Write a fully operationalised non-directional (two-tailed) hypothesis for Tracy’s study. (2)
3. Jamila is conducting a practical investigation to look at gender differences in carrying out visuo-spatial tasks. She decides to give males and females a jigsaw puzzle and will time them to see who completes it the fastest. She uses a random sample of pupils from a local school to get her participants.
(a) Write a fully operationalised directional (one tailed) hypothesis for Jamila’s study. (2) (b) Outline one strength and one weakness of the random sampling method. You may refer to Jamila’s use of this type of sampling in your answer. (4)
4. Which of the following is a non-directional (two tailed) hypothesis?
A There is a difference in driving ability with men being better drivers than women
B Women are better at concentrating on more than one thing at a time than men
C Women spend more time doing the cooking and cleaning than men
D There is a difference in the number of men and women who participate in sports
Revision Activities
writing-hypotheses-revision-sheet
Quizizz link for teachers: https://quizizz.com/admin/quiz/5bf03f51add785001bc5a09e
By Psychstix by Mandy wood
Share this:
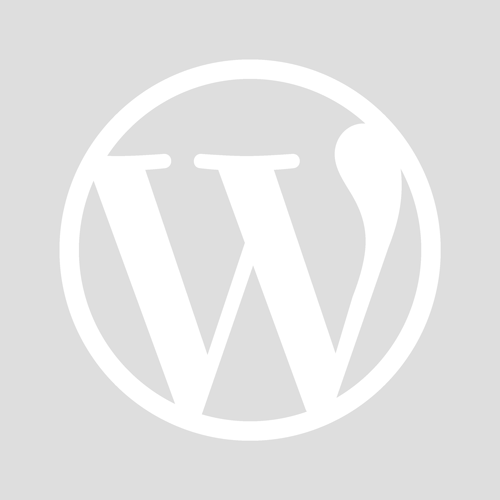
- Already have a WordPress.com account? Log in now.
- Subscribe Subscribed
- Copy shortlink
- Report this content
- View post in Reader
- Manage subscriptions
- Collapse this bar

Directional Hypothesis: Definition and 10 Examples
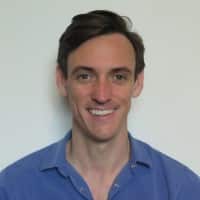
Chris Drew (PhD)
Dr. Chris Drew is the founder of the Helpful Professor. He holds a PhD in education and has published over 20 articles in scholarly journals. He is the former editor of the Journal of Learning Development in Higher Education. [Image Descriptor: Photo of Chris]
Learn about our Editorial Process
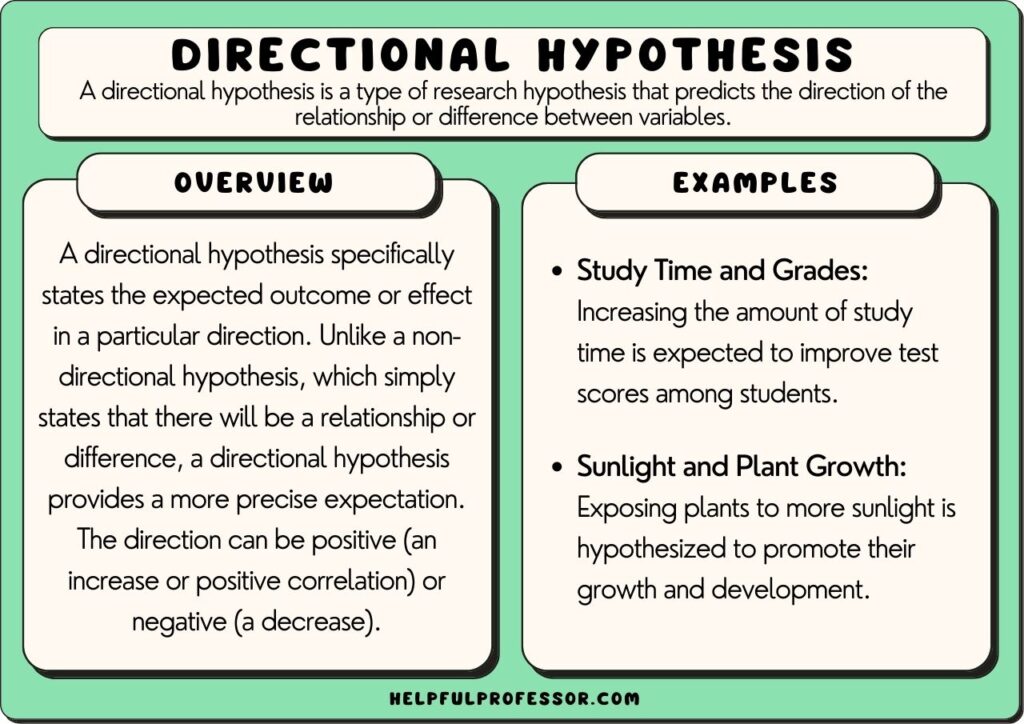
A directional hypothesis refers to a type of hypothesis used in statistical testing that predicts a particular direction of the expected relationship between two variables.
In simpler terms, a directional hypothesis is an educated, specific guess about the direction of an outcome—whether an increase, decrease, or a proclaimed difference in variable sets.
For example, in a study investigating the effects of sleep deprivation on cognitive performance, a directional hypothesis might state that as sleep deprivation (Independent Variable) increases, cognitive performance (Dependent Variable) decreases (Killgore, 2010). Such a hypothesis offers a clear, directional relationship whereby a specific increase or decrease is anticipated.
Global warming provides another notable example of a directional hypothesis. A researcher might hypothesize that as carbon dioxide (CO2) levels increase, global temperatures also increase (Thompson, 2010). In this instance, the hypothesis clearly articulates an upward trend for both variables.
In any given circumstance, it’s imperative that a directional hypothesis is grounded on solid evidence. For instance, the CO2 and global temperature relationship is based on substantial scientific evidence, and not on a random guess or mere speculation (Florides & Christodoulides, 2009).
Directional vs Non-Directional vs Null Hypotheses
A directional hypothesis is generally contrasted to a non-directional hypothesis. Here’s how they compare:
- Directional hypothesis: A directional hypothesis provides a perspective of the expected relationship between variables, predicting the direction of that relationship (either positive, negative, or a specific difference).
- Non-directional hypothesis: A non-directional hypothesis denotes the possibility of a relationship between two variables ( the independent and dependent variables ), although this hypothesis does not venture a prediction as to the direction of this relationship (Ali & Bhaskar, 2016). For example, a non-directional hypothesis might state that there exists a relationship between a person’s diet (independent variable) and their mood (dependent variable), without indicating whether improvement in diet enhances mood positively or negatively. Overall, the choice between a directional or non-directional hypothesis depends on the known or anticipated link between the variables under consideration in research studies.
Another very important type of hypothesis that we need to know about is a null hypothesis :
- Null hypothesis : The null hypothesis stands as a universality—the hypothesis that there is no observed effect in the population under study, meaning there is no association between variables (or that the differences are down to chance). For instance, a null hypothesis could be constructed around the idea that changing diet (independent variable) has no discernible effect on a person’s mood (dependent variable) (Yan & Su, 2016). This proposition is the one that we aim to disprove in an experiment.
While directional and non-directional hypotheses involve some integrated expectations about the outcomes (either distinct direction or a vague relationship), a null hypothesis operates on the premise of negating such relationships or effects.
The null hypotheses is typically proposed to be negated or disproved by statistical tests, paving way for the acceptance of an alternate hypothesis (either directional or non-directional).
Directional Hypothesis Examples
1. exercise and heart health.
Research suggests that as regular physical exercise (independent variable) increases, the risk of heart disease (dependent variable) decreases (Jakicic, Davis, Rogers, King, Marcus, Helsel, Rickman, Wahed, Belle, 2016). In this example, a directional hypothesis anticipates that the more individuals maintain routine workouts, the lesser would be their odds of developing heart-related disorders. This assumption is based on the underlying fact that routine exercise can help reduce harmful cholesterol levels, regulate blood pressure, and bring about overall health benefits. Thus, a direction – a decrease in heart disease – is expected in relation with an increase in exercise.
2. Screen Time and Sleep Quality
Another classic instance of a directional hypothesis can be seen in the relationship between the independent variable, screen time (especially before bed), and the dependent variable, sleep quality. This hypothesis predicts that as screen time before bed increases, sleep quality decreases (Chang, Aeschbach, Duffy, Czeisler, 2015). The reasoning behind this hypothesis is the disruptive effect of artificial light (especially blue light from screens) on melatonin production, a hormone needed to regulate sleep. As individuals spend more time exposed to screens before bed, it is predictably hypothesized that their sleep quality worsens.
3. Job Satisfaction and Employee Turnover
A typical scenario in organizational behavior research posits that as job satisfaction (independent variable) increases, the rate of employee turnover (dependent variable) decreases (Cheng, Jiang, & Riley, 2017). This directional hypothesis emphasizes that an increased level of job satisfaction would lead to a reduced rate of employees leaving the company. The theoretical basis for this hypothesis is that satisfied employees often tend to be more committed to the organization and are less likely to seek employment elsewhere, thus reducing turnover rates.
4. Healthy Eating and Body Weight
Healthy eating, as the independent variable, is commonly thought to influence body weight, the dependent variable, in a positive way. For example, the hypothesis might state that as consumption of healthy foods increases, an individual’s body weight decreases (Framson, Kristal, Schenk, Littman, Zeliadt, & Benitez, 2009). This projection is based on the premise that healthier foods, such as fruits and vegetables, are generally lower in calories than junk food, assisting in weight management.
5. Sun Exposure and Skin Health
The association between sun exposure (independent variable) and skin health (dependent variable) allows for a definitive hypothesis declaring that as sun exposure increases, the risk of skin damage or skin cancer increases (Whiteman, Whiteman, & Green, 2001). The premise aligns with the understanding that overexposure to the sun’s ultraviolet rays can deteriorate skin health, leading to conditions like sunburn or, in extreme cases, skin cancer.
6. Study Hours and Academic Performance
A regularly assessed relationship in academia suggests that as the number of study hours (independent variable) rises, so too does academic performance (dependent variable) (Nonis, Hudson, Logan, Ford, 2013). The hypothesis proposes a positive correlation , with an increase in study time expected to contribute to enhanced academic outcomes.
7. Screen Time and Eye Strain
It’s commonly hypothesized that as screen time (independent variable) increases, the likelihood of experiencing eye strain (dependent variable) also increases (Sheppard & Wolffsohn, 2018). This is based on the idea that prolonged engagement with digital screens—computers, tablets, or mobile phones—can cause discomfort or fatigue in the eyes, attributing to symptoms of eye strain.
8. Physical Activity and Stress Levels
In the sphere of mental health, it’s often proposed that as physical activity (independent variable) increases, levels of stress (dependent variable) decrease (Stonerock, Hoffman, Smith, Blumenthal, 2015). Regular exercise is known to stimulate the production of endorphins, the body’s natural mood elevators, helping to alleviate stress.
9. Water Consumption and Kidney Health
A common health-related hypothesis might predict that as water consumption (independent variable) increases, the risk of kidney stones (dependent variable) decreases (Curhan, Willett, Knight, & Stampfer, 2004). Here, an increase in water intake is inferred to reduce the risk of kidney stones by diluting the substances that lead to stone formation.
10. Traffic Noise and Sleep Quality
In urban planning research, it’s often supposed that as traffic noise (independent variable) increases, sleep quality (dependent variable) decreases (Muzet, 2007). Increased noise levels, particularly during the night, can result in sleep disruptions, thus, leading to poor sleep quality.
11. Sugar Consumption and Dental Health
In the field of dental health, an example might be stating as one’s sugar consumption (independent variable) increases, dental health (dependent variable) decreases (Sheiham, & James, 2014). This stems from the fact that sugar is a major factor in tooth decay, and increased consumption of sugary foods or drinks leads to a decline in dental health due to the high likelihood of cavities.
See 15 More Examples of Hypotheses Here
A directional hypothesis plays a critical role in research, paving the way for specific predicted outcomes based on the relationship between two variables. These hypotheses clearly illuminate the expected direction—the increase or decrease—of an effect. From predicting the impacts of healthy eating on body weight to forecasting the influence of screen time on sleep quality, directional hypotheses allow for targeted and strategic examination of phenomena. In essence, directional hypotheses provide the crucial path for inquiry, shaping the trajectory of research studies and ultimately aiding in the generation of insightful, relevant findings.
Ali, S., & Bhaskar, S. (2016). Basic statistical tools in research and data analysis. Indian Journal of Anaesthesia, 60 (9), 662-669. doi: https://doi.org/10.4103%2F0019-5049.190623
Chang, A. M., Aeschbach, D., Duffy, J. F., & Czeisler, C. A. (2015). Evening use of light-emitting eReaders negatively affects sleep, circadian timing, and next-morning alertness. Proceeding of the National Academy of Sciences, 112 (4), 1232-1237. doi: https://doi.org/10.1073/pnas.1418490112
Cheng, G. H. L., Jiang, D., & Riley, J. H. (2017). Organizational commitment and intrinsic motivation of regular and contractual primary school teachers in China. New Psychology, 19 (3), 316-326. Doi: https://doi.org/10.4103%2F2249-4863.184631
Curhan, G. C., Willett, W. C., Knight, E. L., & Stampfer, M. J. (2004). Dietary factors and the risk of incident kidney stones in younger women: Nurses’ Health Study II. Archives of Internal Medicine, 164 (8), 885–891.
Florides, G. A., & Christodoulides, P. (2009). Global warming and carbon dioxide through sciences. Environment international , 35 (2), 390-401. doi: https://doi.org/10.1016/j.envint.2008.07.007
Framson, C., Kristal, A. R., Schenk, J. M., Littman, A. J., Zeliadt, S., & Benitez, D. (2009). Development and validation of the mindful eating questionnaire. Journal of the American Dietetic Association, 109 (8), 1439-1444. doi: https://doi.org/10.1016/j.jada.2009.05.006
Jakicic, J. M., Davis, K. K., Rogers, R. J., King, W. C., Marcus, M. D., Helsel, D., … & Belle, S. H. (2016). Effect of wearable technology combined with a lifestyle intervention on long-term weight loss: The IDEA randomized clinical trial. JAMA, 316 (11), 1161-1171.
Khan, S., & Iqbal, N. (2013). Study of the relationship between study habits and academic achievement of students: A case of SPSS model. Higher Education Studies, 3 (1), 14-26.
Killgore, W. D. (2010). Effects of sleep deprivation on cognition. Progress in brain research , 185 , 105-129. doi: https://doi.org/10.1016/B978-0-444-53702-7.00007-5
Marczinski, C. A., & Fillmore, M. T. (2014). Dissociative antagonistic effects of caffeine on alcohol-induced impairment of behavioral control. Experimental and Clinical Psychopharmacology, 22 (4), 298–311. doi: https://psycnet.apa.org/doi/10.1037/1064-1297.11.3.228
Muzet, A. (2007). Environmental Noise, Sleep and Health. Sleep Medicine Reviews, 11 (2), 135-142. doi: https://doi.org/10.1016/j.smrv.2006.09.001
Nonis, S. A., Hudson, G. I., Logan, L. B., & Ford, C. W. (2013). Influence of perceived control over time on college students’ stress and stress-related outcomes. Research in Higher Education, 54 (5), 536-552. doi: https://doi.org/10.1023/A:1018753706925
Sheiham, A., & James, W. P. (2014). A new understanding of the relationship between sugars, dental caries and fluoride use: implications for limits on sugars consumption. Public health nutrition, 17 (10), 2176-2184. Doi: https://doi.org/10.1017/S136898001400113X
Sheppard, A. L., & Wolffsohn, J. S. (2018). Digital eye strain: prevalence, measurement and amelioration. BMJ open ophthalmology , 3 (1), e000146. doi: http://dx.doi.org/10.1136/bmjophth-2018-000146
Stonerock, G. L., Hoffman, B. M., Smith, P. J., & Blumenthal, J. A. (2015). Exercise as Treatment for Anxiety: Systematic Review and Analysis. Annals of Behavioral Medicine, 49 (4), 542–556. doi: https://doi.org/10.1007/s12160-014-9685-9
Thompson, L. G. (2010). Climate change: The evidence and our options. The Behavior Analyst , 33 , 153-170. Doi: https://doi.org/10.1007/BF03392211
Whiteman, D. C., Whiteman, C. A., & Green, A. C. (2001). Childhood sun exposure as a risk factor for melanoma: a systematic review of epidemiologic studies. Cancer Causes & Control, 12 (1), 69-82. doi: https://doi.org/10.1023/A:1008980919928
Yan, X., & Su, X. (2009). Linear regression analysis: theory and computing . New Jersey: World Scientific.
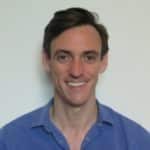
- Chris Drew (PhD) https://helpfulprofessor.com/author/chris-drew-phd-2/ 10 Reasons you’re Perpetually Single
- Chris Drew (PhD) https://helpfulprofessor.com/author/chris-drew-phd-2/ 20 Montessori Toddler Bedrooms (Design Inspiration)
- Chris Drew (PhD) https://helpfulprofessor.com/author/chris-drew-phd-2/ 21 Montessori Homeschool Setups
- Chris Drew (PhD) https://helpfulprofessor.com/author/chris-drew-phd-2/ 101 Hidden Talents Examples
Leave a Comment Cancel Reply
Your email address will not be published. Required fields are marked *
Directional and non-directional hypothesis: A Comprehensive Guide
Karolina Konopka
Customer support manager
Want to talk with us?
In the world of research and statistical analysis, hypotheses play a crucial role in formulating and testing scientific claims. Understanding the differences between directional and non-directional hypothesis is essential for designing sound experiments and drawing accurate conclusions. Whether you’re a student, researcher, or simply curious about the foundations of hypothesis testing, this guide will equip you with the knowledge and tools to navigate this fundamental aspect of scientific inquiry.
Understanding Directional Hypothesis
Understanding directional hypotheses is crucial for conducting hypothesis-driven research, as they guide the selection of appropriate statistical tests and aid in the interpretation of results. By incorporating directional hypotheses, researchers can make more precise predictions, contribute to scientific knowledge, and advance their fields of study.
Definition of directional hypothesis
Directional hypotheses, also known as one-tailed hypotheses, are statements in research that make specific predictions about the direction of a relationship or difference between variables. Unlike non-directional hypotheses, which simply state that there is a relationship or difference without specifying its direction, directional hypotheses provide a focused and precise expectation.
A directional hypothesis predicts either a positive or negative relationship between variables or predicts that one group will perform better than another. It asserts a specific direction of effect or outcome. For example, a directional hypothesis could state that “increased exposure to sunlight will lead to an improvement in mood” or “participants who receive the experimental treatment will exhibit higher levels of cognitive performance compared to the control group.”
Directional hypotheses are formulated based on existing theory, prior research, or logical reasoning, and they guide the researcher’s expectations and analysis. They allow for more targeted predictions and enable researchers to test specific hypotheses using appropriate statistical tests.
The role of directional hypothesis in research
Directional hypotheses also play a significant role in research surveys. Let’s explore their role specifically in the context of survey research:
- Objective-driven surveys : Directional hypotheses help align survey research with specific objectives. By formulating directional hypotheses, researchers can focus on gathering data that directly addresses the predicted relationship or difference between variables of interest.
- Question design and measurement : Directional hypotheses guide the design of survey question types and the selection of appropriate measurement scales. They ensure that the questions are tailored to capture the specific aspects related to the predicted direction, enabling researchers to obtain more targeted and relevant data from survey respondents.
- Data analysis and interpretation : Directional hypotheses assist in data analysis by directing researchers towards appropriate statistical tests and methods. Researchers can analyze the survey data to specifically test the predicted relationship or difference, enhancing the accuracy and reliability of their findings. The results can then be interpreted within the context of the directional hypothesis, providing more meaningful insights.
- Practical implications and decision-making : Directional hypotheses in surveys often have practical implications. When the predicted relationship or difference is confirmed, it informs decision-making processes, program development, or interventions. The survey findings based on directional hypotheses can guide organizations, policymakers, or practitioners in making informed choices to achieve desired outcomes.
- Replication and further research : Directional hypotheses in survey research contribute to the replication and extension of studies. Researchers can replicate the survey with different populations or contexts to assess the generalizability of the predicted relationships. Furthermore, if the directional hypothesis is supported, it encourages further research to explore underlying mechanisms or boundary conditions.
By incorporating directional hypotheses in survey research, researchers can align their objectives, design effective surveys, conduct focused data analysis, and derive practical insights. They provide a framework for organizing survey research and contribute to the accumulation of knowledge in the field.
Examples of research questions for directional hypothesis
Here are some examples of research questions that lend themselves to directional hypotheses:
- Does increased daily exercise lead to a decrease in body weight among sedentary adults?
- Is there a positive relationship between study hours and academic performance among college students?
- Does exposure to violent video games result in an increase in aggressive behavior among adolescents?
- Does the implementation of a mindfulness-based intervention lead to a reduction in stress levels among working professionals?
- Is there a difference in customer satisfaction between Product A and Product B, with Product A expected to have higher satisfaction ratings?
- Does the use of social media influence self-esteem levels, with higher social media usage associated with lower self-esteem?
- Is there a negative relationship between job satisfaction and employee turnover, indicating that lower job satisfaction leads to higher turnover rates?
- Does the administration of a specific medication result in a decrease in symptoms among individuals with a particular medical condition?
- Does increased access to early childhood education lead to improved cognitive development in preschool-aged children?
- Is there a difference in purchase intention between advertisements with celebrity endorsements and advertisements without, with celebrity endorsements expected to have a higher impact?
These research questions generate specific predictions about the direction of the relationship or difference between variables and can be tested using appropriate research methods and statistical analyses.
Definition of non-directional hypothesis
Non-directional hypotheses, also known as two-tailed hypotheses, are statements in research that indicate the presence of a relationship or difference between variables without specifying the direction of the effect. Instead of making predictions about the specific direction of the relationship or difference, non-directional hypotheses simply state that there is an association or distinction between the variables of interest.
Non-directional hypotheses are often used when there is no prior theoretical basis or clear expectation about the direction of the relationship. They leave the possibility open for either a positive or negative relationship, or for both groups to differ in some way without specifying which group will perform better or worse.
Advantages and utility of non-directional hypothesis
Non-directional hypotheses in survey s offer several advantages and utilities, providing flexibility and comprehensive analysis of survey data. Here are some of the key advantages and utilities of using non-directional hypotheses in surveys:
- Exploration of Relationships : Non-directional hypotheses allow researchers to explore and examine relationships between variables without assuming a specific direction. This is particularly useful in surveys where the relationship between variables may not be well-known or there may be conflicting evidence regarding the direction of the effect.
- Flexibility in Question Design : With non-directional hypotheses, survey questions can be designed to measure the relationship between variables without being biased towards a particular outcome. This flexibility allows researchers to collect data and analyze the results more objectively.
- Open to Unexpected Findings : Non-directional hypotheses enable researchers to be open to unexpected or surprising findings in survey data. By not committing to a specific direction of the effect, researchers can identify and explore relationships that may not have been initially anticipated, leading to new insights and discoveries.
- Comprehensive Analysis : Non-directional hypotheses promote comprehensive analysis of survey data by considering the possibility of an effect in either direction. Researchers can assess the magnitude and significance of relationships without limiting their analysis to only one possible outcome.
- S tatistical Validity : Non-directional hypotheses in surveys allow for the use of two-tailed statistical tests, which provide a more conservative and robust assessment of significance. Two-tailed tests consider both positive and negative deviations from the null hypothesis, ensuring accurate and reliable statistical analysis of survey data.
- Exploratory Research : Non-directional hypotheses are particularly useful in exploratory research, where the goal is to gather initial insights and generate hypotheses. Surveys with non-directional hypotheses can help researchers explore various relationships and identify patterns that can guide further research or hypothesis development.
It is worth noting that the choice between directional and non-directional hypotheses in surveys depends on the research objectives, existing knowledge, and the specific variables being investigated. Researchers should carefully consider the advantages and limitations of each approach and select the one that aligns best with their research goals and survey design.
- Share with others
- Twitter Twitter Icon
- LinkedIn LinkedIn Icon
Related posts
Microsurveys: complete guide, recurring surveys: the ultimate guide, close-ended questions: definition, types, and examples, top 10 typeform alternatives and competitors, 15 best google forms alternatives and competitors, top 10 qualtrics alternatives and competitors, get answers today.
- No credit card required
- No time limit on Free plan
You can modify this template in every possible way.
All templates work great on every device.
5.2 - Writing Hypotheses
The first step in conducting a hypothesis test is to write the hypothesis statements that are going to be tested. For each test you will have a null hypothesis (\(H_0\)) and an alternative hypothesis (\(H_a\)).
When writing hypotheses there are three things that we need to know: (1) the parameter that we are testing (2) the direction of the test (non-directional, right-tailed or left-tailed), and (3) the value of the hypothesized parameter.
- At this point we can write hypotheses for a single mean (\(\mu\)), paired means(\(\mu_d\)), a single proportion (\(p\)), the difference between two independent means (\(\mu_1-\mu_2\)), the difference between two proportions (\(p_1-p_2\)), a simple linear regression slope (\(\beta\)), and a correlation (\(\rho\)).
- The research question will give us the information necessary to determine if the test is two-tailed (e.g., "different from," "not equal to"), right-tailed (e.g., "greater than," "more than"), or left-tailed (e.g., "less than," "fewer than").
- The research question will also give us the hypothesized parameter value. This is the number that goes in the hypothesis statements (i.e., \(\mu_0\) and \(p_0\)). For the difference between two groups, regression, and correlation, this value is typically 0.
Hypotheses are always written in terms of population parameters (e.g., \(p\) and \(\mu\)). The tables below display all of the possible hypotheses for the parameters that we have learned thus far. Note that the null hypothesis always includes the equality (i.e., =).
Research Question | Is the population mean different from \( \mu_{0} \)? | Is the population mean greater than \(\mu_{0}\)? | Is the population mean less than \(\mu_{0}\)? |
---|---|---|---|
Null Hypothesis, \(H_{0}\) | \(\mu=\mu_{0} \) | \(\mu=\mu_{0} \) | \(\mu=\mu_{0} \) |
Alternative Hypothesis, \(H_{a}\) | \(\mu\neq \mu_{0} \) | \(\mu> \mu_{0} \) | \(\mu<\mu_{0} \) |
Type of Hypothesis Test | Two-tailed, non-directional | Right-tailed, directional | Left-tailed, directional |
Research Question | Is there a difference in the population? | Is there a mean increase in the population? | Is there a mean decrease in the population? |
---|---|---|---|
Null Hypothesis, \(H_{0}\) | \(\mu_d=0 \) | \(\mu_d =0 \) | \(\mu_d=0 \) |
Alternative Hypothesis, \(H_{a}\) | \(\mu_d \neq 0 \) | \(\mu_d> 0 \) | \(\mu_d<0 \) |
Type of Hypothesis Test | Two-tailed, non-directional | Right-tailed, directional | Left-tailed, directional |
Research Question | Is the population proportion different from \(p_0\)? | Is the population proportion greater than \(p_0\)? | Is the population proportion less than \(p_0\)? |
---|---|---|---|
Null Hypothesis, \(H_{0}\) | \(p=p_0\) | \(p= p_0\) | \(p= p_0\) |
Alternative Hypothesis, \(H_{a}\) | \(p\neq p_0\) | \(p> p_0\) | \(p< p_0\) |
Type of Hypothesis Test | Two-tailed, non-directional | Right-tailed, directional | Left-tailed, directional |
Research Question | Are the population means different? | Is the population mean in group 1 greater than the population mean in group 2? | Is the population mean in group 1 less than the population mean in groups 2? |
---|---|---|---|
Null Hypothesis, \(H_{0}\) | \(\mu_1=\mu_2\) | \(\mu_1 = \mu_2 \) | \(\mu_1 = \mu_2 \) |
Alternative Hypothesis, \(H_{a}\) | \(\mu_1 \ne \mu_2 \) | \(\mu_1 \gt \mu_2 \) | \(\mu_1 \lt \mu_2\) |
Type of Hypothesis Test | Two-tailed, non-directional | Right-tailed, directional | Left-tailed, directional |
Research Question | Are the population proportions different? | Is the population proportion in group 1 greater than the population proportion in groups 2? | Is the population proportion in group 1 less than the population proportion in group 2? |
---|---|---|---|
Null Hypothesis, \(H_{0}\) | \(p_1 = p_2 \) | \(p_1 = p_2 \) | \(p_1 = p_2 \) |
Alternative Hypothesis, \(H_{a}\) | \(p_1 \ne p_2\) | \(p_1 \gt p_2 \) | \(p_1 \lt p_2\) |
Type of Hypothesis Test | Two-tailed, non-directional | Right-tailed, directional | Left-tailed, directional |
Research Question | Is the slope in the population different from 0? | Is the slope in the population positive? | Is the slope in the population negative? |
---|---|---|---|
Null Hypothesis, \(H_{0}\) | \(\beta =0\) | \(\beta= 0\) | \(\beta = 0\) |
Alternative Hypothesis, \(H_{a}\) | \(\beta\neq 0\) | \(\beta> 0\) | \(\beta< 0\) |
Type of Hypothesis Test | Two-tailed, non-directional | Right-tailed, directional | Left-tailed, directional |
Research Question | Is the correlation in the population different from 0? | Is the correlation in the population positive? | Is the correlation in the population negative? |
---|---|---|---|
Null Hypothesis, \(H_{0}\) | \(\rho=0\) | \(\rho= 0\) | \(\rho = 0\) |
Alternative Hypothesis, \(H_{a}\) | \(\rho \neq 0\) | \(\rho > 0\) | \(\rho< 0\) |
Type of Hypothesis Test | Two-tailed, non-directional | Right-tailed, directional | Left-tailed, directional |
5.2.1 - Examples
Example: rent.
Research question : Is the average monthly rent of a one-bedroom apartment in State College, Pennsylvania less than \$900?
In this question we are comparing the mean of all State College one-bedroom apartments (i.e. \(\mu\)) to the value of \$900. This is a single sample mean test. We want to know if the population mean is less than \$900, so this is a left-tailed test. Our hypotheses are:
- \(H_0:\mu=\$900\)
- \(H_a: \mu < \$900\)
Example: IQ Scores
Research question : Is the average IQ score of all World Campus STAT 200 students higher than the national average of 100?
In this question we are comparing the mean of all World Campus STAT 200 students (i.e. \(\mu\)) to the given value of 100. This is a single sample mean test. We want to know if the population mean is greater than 100, so this is a right-tailed test. Our hypotheses are:
- \(H_0:\mu = 100\)
- \(H_a: \mu > 100\)
Example: Weight Loss
Research question: Do participants lose weight following a weight-loss intervention?
Data were collected from one group of participants before and after a weight-loss intervention. Data were paired by participant. Assuming that \(x_1\) is an individual's weight before the intervention and \(x_2\) is their weight at the end of the study, if they lost weight then \(x_1-x_2\) would be a positive number (i.e., greater than 0). Thus, this is a right-tailed test. Because we are testing their mean difference, the parameter that we should write in our hypotheses is \(\mu_d\) where \(\mu_d\) is the mean weight change (before-after) in the population.
Our hypotheses are:
- \(H_0: \mu_d=0\)
- \(H_a:\mu_d > 0 \)
Example: Gender of College of Science Students
Research question : Is the percent of students enrolled in Penn State's College of Science who identify as women different from 50%?
In this question we are comparing the proportion of all Penn State College of Science students (i.e. \(p\)) to the given value of 0.5. This is a single sample proportion test. We want to know if the population proportion is different from 0.5, so this is a two-tailed test. Our hypotheses are:
- \(H_0:p=0.5\)
- \(H_a: p ≠ 0.5\)
Example: Dog Ownership
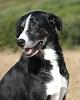
Research question : Do the majority of all World Campus STAT 200 students own a dog?
If the majority of all students own a dog, then more than 50% own a dog. In this question we are comparing the population proportion for all World Campus STAT 200 students (i.e. \(p\)) to the value of 0.5. This is a single sampling proportion test. We want to know if the proportion is greater than 0.5, so this is a right-tailed test. Our hypotheses are:
- \(H_a: p > 0.5\)
Example: Weights of Boys and Girls
Research question : In preschool, are the weights of boys and girls different?
We are comparing the weights of two independent groups: boys and girls. Weight is a quantitative variable so the parameter we are testing is \(\mu\). Our research question does not hypothesize which group has the larger weight, so this is a two-tailed test. Our hypotheses are:
- \(H_0: \mu_b = \mu_g\)
- \(H_a: \mu_b \ne \mu_g\)
Note: This is equivalent to \(H_0: \mu_b - \mu_g = 0\) and \(H_a: \mu_b - \mu_g \ne 0\).
Example: Smoking by Gender
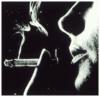
Research question : Is the proportion of men who smoke cigarettes different from the proportion of women who smoke cigarettes in the United States?
In this question we are comparing two independent groups: men and women. The response variable, smoking, is categorical therefore we are comparing proportions. Our research question does not suggest which group smokes more, so we have a two-tailed test. Our hypotheses are:
- \(H_0: p_1=p_2\)
- \(H_a: p_1 \ne p_2\)
Note: This is equivalent to \(H_0: p_1 - p_2 =0\) and \(H_a: p_1 - p_2 \ne 0\)
Example: Predicting SAT-Math using IQ
Research question : Can IQ scores be used to predict SAT-Math scores in the population of all American high school seniors?
SAT-Math and IQ scores are both quantitative variables. Our research question is about prediction, so we are going to use simple linear regression. The parameter we are testing is \(\beta\). Our research question does not state whether we expect the slope to be positive or negative, therefore this is a two-tailed test. Our hypotheses are:
- \(H_0: \beta = 0\)
- \(H_a: \beta \ne 0\)
Example: Relation Between Height and Weight
Research question : Is there a positive relationship between height and weight in the population of all American adults age 25 and older?
The relationship between two quantitative variables is measured using correlation (Pearson's r). The parameter we are testing is \(\rho\). A positive relationship would be indicated by a positive correlation coefficient, therefore this is a right-tailed test. Our hypotheses are:
- \(H_0: \rho = 0\)
- \(H_a: \rho > 0\)
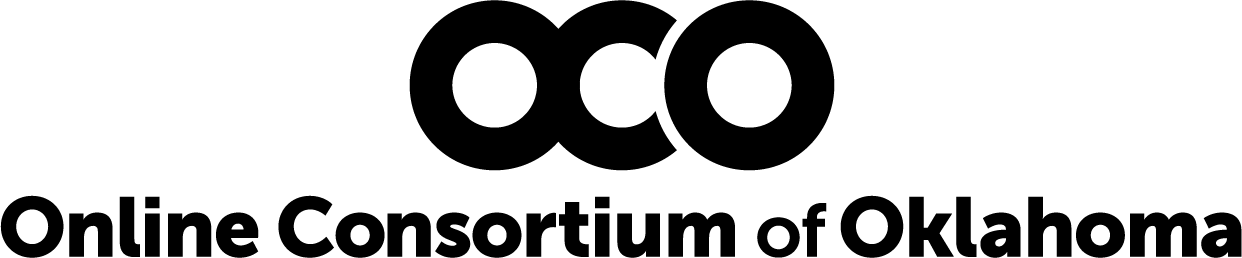
16 Correlation
Learning outcomes.
In this chapter, you will learn how to:
- Describe the concept of the correlation coefficient and its interpretation
- Conduct a hypothesis test for a correlation between two continuous variables
- Compute the Pearson correlation as a test statistic
- Evaluate effect size for a Pearson correlation
- Identify type of correlation based on the data (Pearson vs Spearman)
- Describe the effect of outlier data points and how to address them
Hypothesis testing beyond t-tests and ANOVAs
Correlations or relationships are measured by correlation coefficients. A correlation coefficient is a measure that varies from -1 to 1, where a value of 1 represents a perfect positive relationship between the variables, 0 represents no relationship, and -1 represents a perfect negative relationship.
In this chapter we will focus on Pearson’s r , which is a measure of the strength of the linear relationship between two continuous variables . Pearson’s r was developed by Karl Pearson in the early 1900s.
Figure 1 shows examples of various levels of correlation using randomly generated data for two continuous variables (reporting Pearson’s r s). We will learn more about interpreting a correlation coefficient when we discuss direction and magnitude later in the chapter.
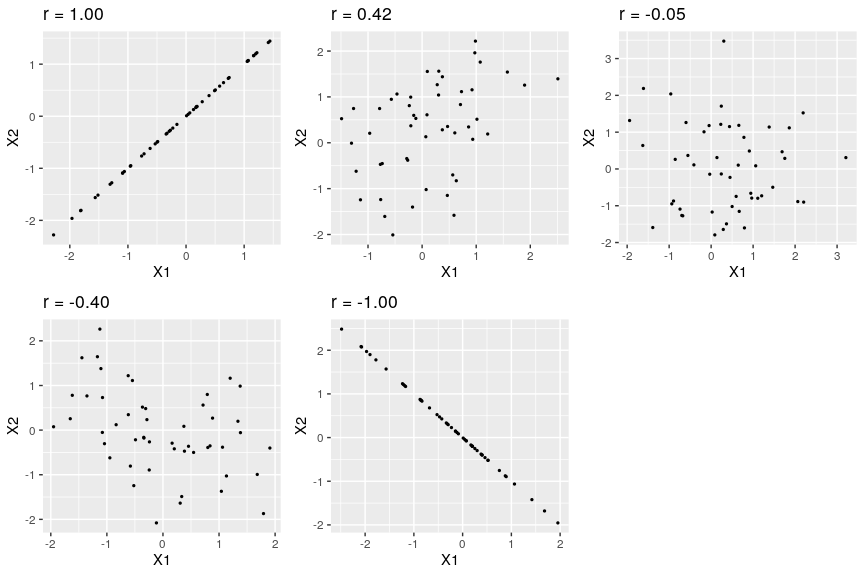
Figure 1. Examples of various levels of Pearson’s r.
Variability and Covariance
A common theme throughout statistics is the notion that individuals will differ on different characteristics and traits, which we call variance. In inferential statistics and hypothesis testing, our goal is to find systematic reasons for differences and rule out random chance as the cause. By doing this, we are using information from a different variable – which so far has been group membership like in ANOVA – to explain this variance. In correlations, we will instead use a continuous variable to account for the variance. Because we have two continuous variables, we will have two characteristics or scores on which people will vary. What we want to know is do people vary on the scores together. That is, as one score changes, does the other score also change in a predictable or consistent way? This notion of variables differing together is called covariance (the prefix “co” meaning “together”).
To be able to understand the covariance between two variables, we must first remember how we get to the variance of a single variable.
[latex]s^2=\frac{SS}{df}=\frac{SS}{n-1}[/latex]
We should also review our two formulas for SS. The first formula is our definitional formula, and the second is our computational formula.
[latex]SS=\Sigma(X-M)^2=\Sigma(X^2)-\frac{(\Sigma X)^2}{n}[/latex]
[latex]cov_{XY}=\frac{SP}{df}=\frac{SP}{n-1}[/latex]
Notice that we now have an SP in the numerator rather than an SS. We won’t be squaring our deviation scores anymore. Rather, we will now be multiplying the deviation scores for X by the deviation scores for Y and summing them up. Thus, this is the sum of the products of deviation scores. Or the sum of products of deviations (SP). And, just like the sum of squared deviation scores, we have a definitional formula and a computational version of the formula available for us to use.
Definitional Formula for SP
[latex]SP=\Sigma(X-M_X)(Y-M_Y)[/latex]
Computational Formula for SP
[latex]SP=\Sigma(XY)-\frac{(\Sigma X)(\Sigma Y)}{n}[/latex]
X | (X −M ) | (X − M ) | Y | (Y −M ) | (Y − M ) | (X −M )(Y −M ) |
|
| (if need s ) |
|
| (if need s ) |
|
… | … | … | … | … | … | … |
|
| ∑ (total up for SS ) |
|
| ∑ (total up for SS ) | ∑ (total up for SP) |
Table 1. Example for calculating Sum of Products
The previous paragraph brings us to an important definition about relations between variables. What we are looking for in a relationship is a consistent or predictable pattern. That is, the variables change together, either in the same direction or opposite directions, in the same way each time. It doesn’t matter if this relationship is positive or negative, only that it is not zero. If there is no consistency in how the variables change within a person, then the relationship is zero and does not exist. We will revisit this notion of direction versus zero relationship later on.
Visualizing Relations
Chapter 3 covered many different forms of data visualization, and visualizing data remains an important first step in understanding and describing our data before we move into inferential statistics. Nowhere is this more important than in correlation. Correlations are visualized by a scatterplot , where our X variable values are plotted on the X-axis, the Y variable values are plotted on the Y-axis, and each point or marker in the plot represents a single person’s score on X and Y. Figure 2 shows a scatterplot for hypothetical scores on job satisfaction (X) and worker well-being (Y). We can see from the axes that each of these variables is measured on a 10- point scale, with 10 being the highest on both variables (high satisfaction and good health and well-being) and 1 being the lowest (dissatisfaction and poor health). When we look at this plot, we can see that the variables do seem to be related. The higher scores on job satisfaction tend to also be the higher scores on well-being, and the same is true of the lower scores.
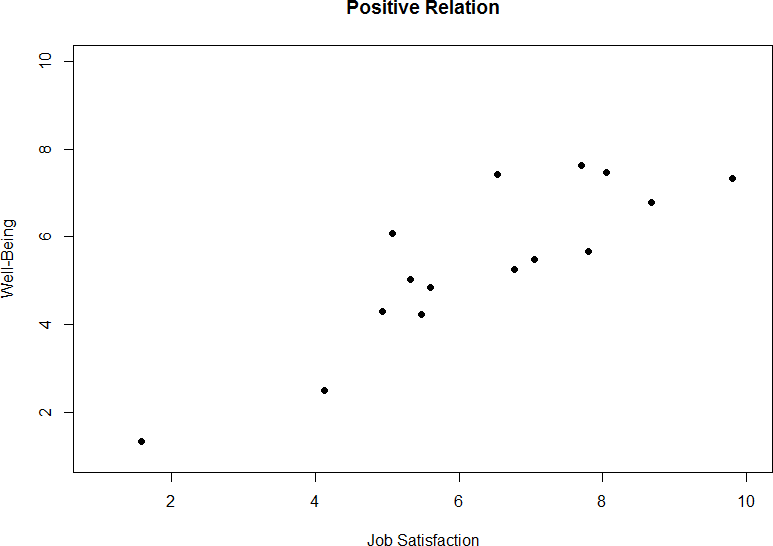
Figure 2. Plotting satisfaction and well-being scores.
Figure 2 demonstrates a positive relation. As scores on X increase, scores on Y also tend to increase. Although this is not a perfect relationship (if it were, the points would form a single straight line), it is nonetheless very clearly positive. This is one of the key benefits to scatterplots: they make it very easy to see the direction of the relationship. As another example, figure 3 shows a negative relationship between job satisfaction (X) and burnout (Y). As we can see from this plot, higher scores on job satisfaction tend to correspond to lower scores on burnout, which is how stressed, unenergetic, and unhappy someone is at their job. As with figure 2, this is not a perfect relationship, but it is still a clear one. As these figures show, points in a positive relationship move from the bottom left of the plot to the top right, and points in a negative relationship move from the top left to the bottom right.
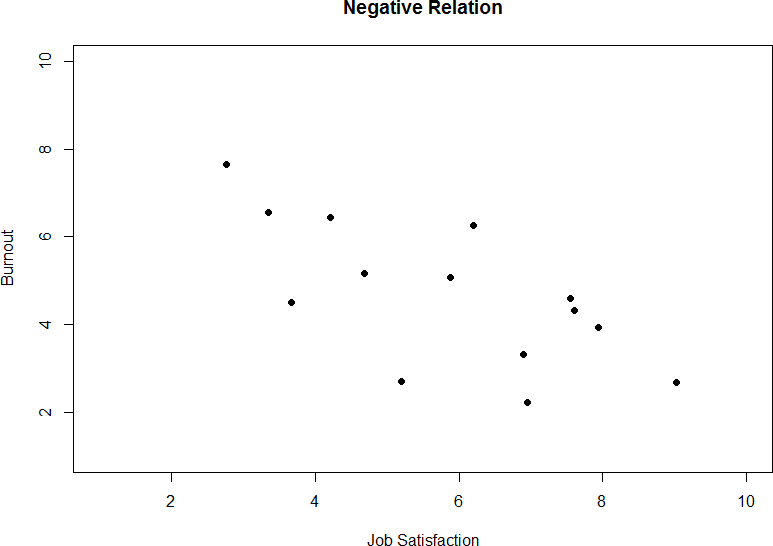
Figure 3. Plotting satisfaction and burnout scores.
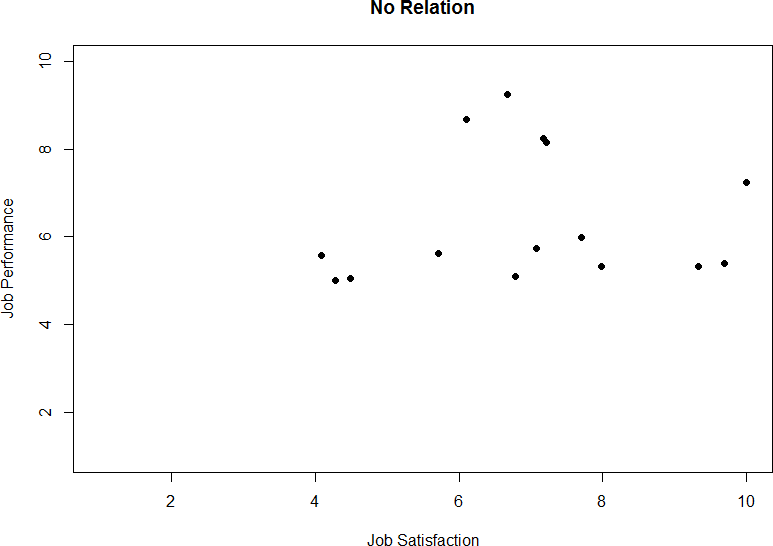
Figure 4. Plotting no relation between satisfaction and job performance.
As we can see, scatterplots are very useful for giving us an approximate idea of whether or not there is a relationship between the two variables and, if there is, if that relation is positive or negative. They are also useful for another reason: they are the only way to determine one of the characteristics of correlations that are discussed next: form.
Three Characteristics
When we talk about correlations, there are three characteristics that we need to know in order to truly understand the relationship (or lack of relationship) between X and Y: form , direction , and magnitude . We will discuss each of them in turn.
The first characteristic of relationship between variables is their form. The form of a relationship is the shape it takes in a scatterplot, and a scatterplot is the only way it is possible to assess the form of a relationship. there are three forms we look for: linear, curvilinear , or no relationship . A linear relationship is what we saw in figures 1, 2, and 3. If we drew a line through the middle points in the any of the scatterplots, we would be best suited with a straight line. The term “linear” comes from the word “line”. A linear relationship is what we will always assume when we calculate correlations. All of the correlations presented here are only valid for linear relationships . Thus, it is important to plot our data to make sure we meet this assumption.
The relationship between two variables can also be curvilinear . As the name suggests, a curvilinear relationship is one in which a line through the middle of the points in a scatterplot will be curved rather than straight. Two examples are presented in figures 5 and 6.
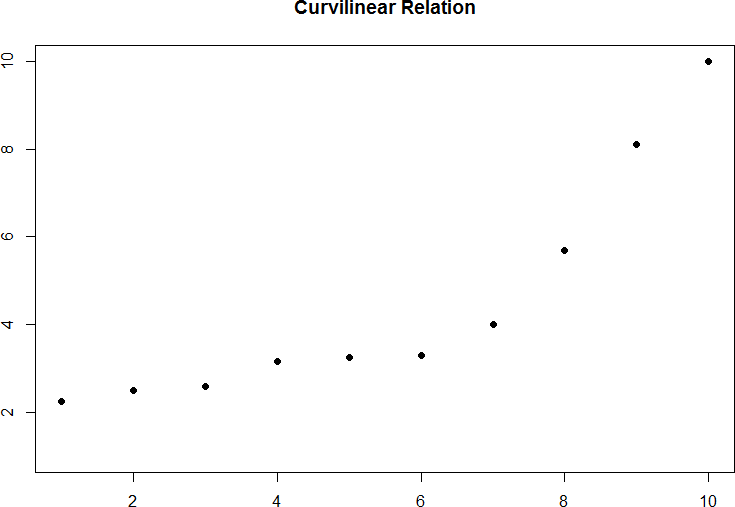
Figure 5. Exponentially increasing curvilinear relationship.
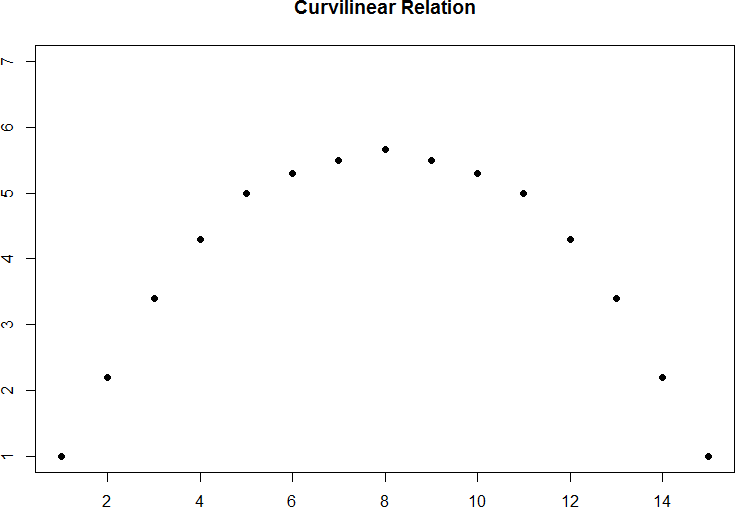
Figure 6. Inverted-U curvilinear relationship.
Curvilinear relationships can take many shapes, and the two examples above are only a small sample of the possibilities. What they have in common is that they both have a very clear pattern but that pattern is not a straight line. If we try to draw a straight line through them, we would get a result similar to what is shown in figure 7.
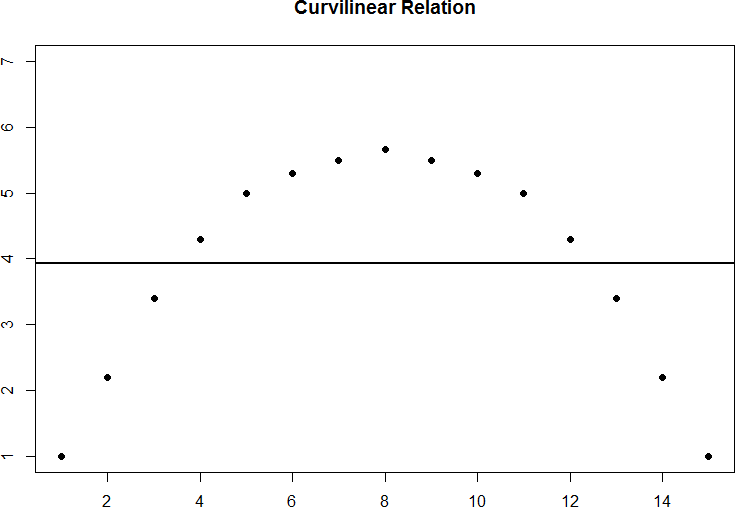
Figure 7. Overlaying a straight line on a curvilinear relationship.
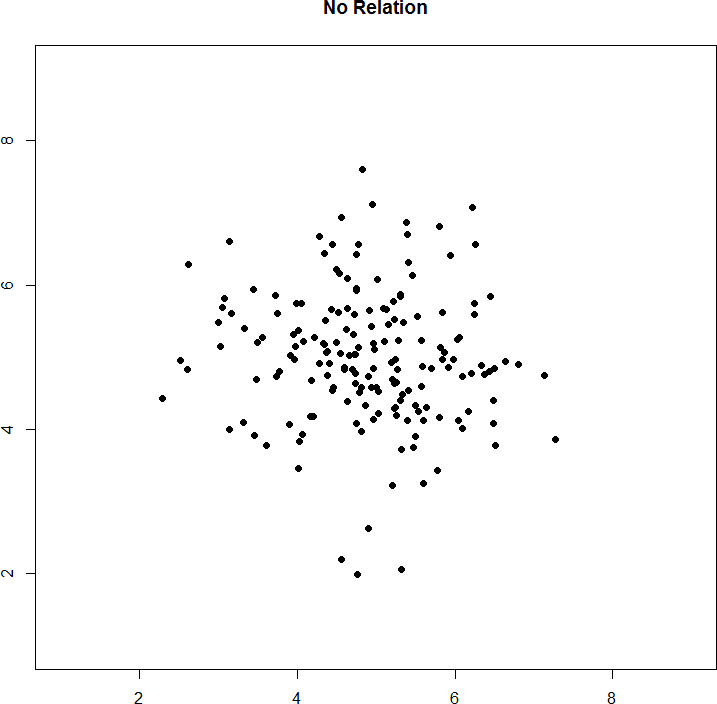
Figure 8. No relation
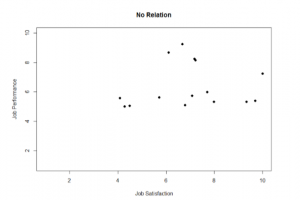
Figure 9. No relationship fictional data scatterplot between job satisfaction and job performance
Sometimes when we look at scatterplots, it is tempting to get biased by a few points that fall far away from the rest of the points and seem to imply that there may be some sort of relation. These points are called outliers, and we will discuss them in more detail later in the chapter. These can be common, so it is important to formally test for a relationship between our variables, not just rely on visualization. This is the point of hypothesis testing with correlations, and we will go in depth on it soon. First, however, we need to describe the other two characteristics of relationships: direction and magnitude .
The direction of the relationship between two variables tells us whether the variables change in the same way at the same time or in opposite ways at the same time. We saw this concept earlier when first discussing scatterplots, and we used the terms positive and negative. A positive relationship is one in which X and Y change in the same direction: as X goes up, Y goes up, and as X goes down, Y also goes down. A negative relationship is just the opposite: X and Y change together in opposite directions: as X goes up, Y goes down, and vice versa.
As we will see soon, when we calculate a correlation coefficient, we are quantifying the relationship demonstrated in a scatterplot. That is, we are putting a number to it. That number will be either positive, negative, or zero, and we interpret the sign of the number as our direction. If the number is positive, it is a positive relationship, and if it is negative, it is a negative relationship. If it is zero, then there is no relationship. The direction of the relationship corresponds directly to the slope of the hypothetical line we draw through scatterplots when assessing the form of the relation. If the line has a positive slope that moves from bottom left to top right, it is positive. If it has a line that goes from top left to bottom right it is considered negative. If the line it flat, that means it has no slope, and there is no relationship, which will in turn yield a zero for our correlation coefficient.
The number we calculate for our correlation coefficient, which we will describe in detail below, corresponds to the magnitude of the relationship between the two variables. The magnitude is how strong or how consistent the relationship between the variables is. Higher numbers mean greater magnitude, which means a stronger relationship. Our correlation coefficients will take on any value between -1.00 and 1.00, with 0.00 in the middle, which again represents no relationship. A correlation of -1.00 is a perfect negative relationship; as X goes up by some amount, Y goes down by the same amount, consistently. Likewise, a correlation of 1.00 indicates a perfect positive relationship; as X goes up by some amount, Y also goes up by the same amount. Finally, a correlation of 0.00, which indicates no relationship, means that as X goes up by some amount, Y may or may not change by any amount, and it does so inconsistently.
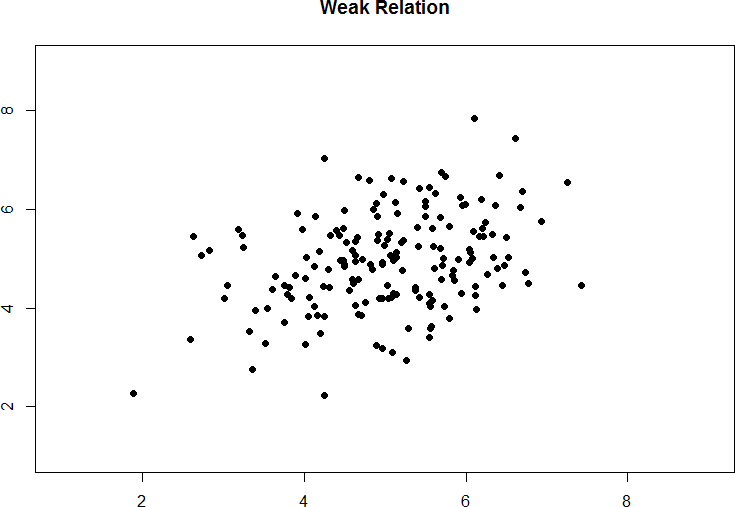
Figure 10. Weak positive correlation.
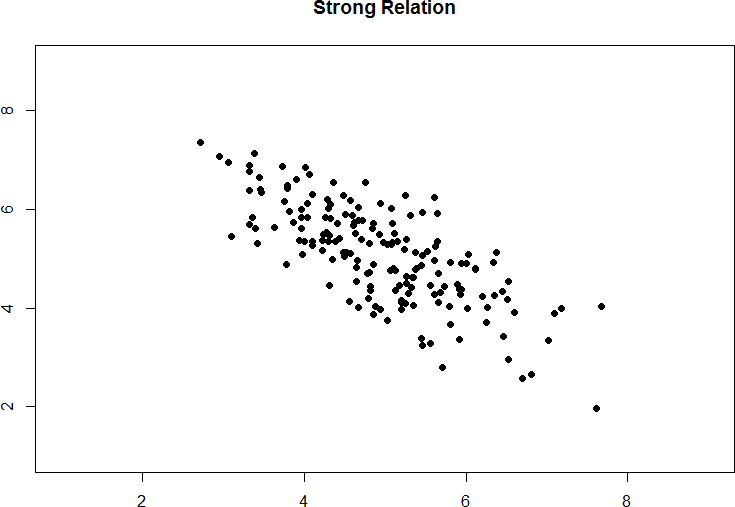
Figure 11. Strong negative correlation.
Pearson’s r
There are several different types of correlation coefficients, but we will only focus on the most common: Pearson’s r . Pearson’s r is a very popular correlation coefficient for assessing linear relationships between two continuous variables, and it serves as both a descriptive statistic (like M ) and as a test statistic (like t ). It is descriptive because it describes what is happening in the scatterplot; r will have both a sign (+/–) for the direction and a number (0 – 1 in absolute value) for the magnitude. As noted above, to use r we assume a linear relation, so nothing about r will suggest what the form is – it will only tell what the direction and magnitude would be if the form is linear. (Remember: always make a scatterplot first!) Pearson’s r also works as a test statistic because the magnitude of r will correspond directly to a t value as the specific degrees of freedom, which can then be compared to a critical value. Luckily, we do not need to do this conversion by hand. Instead, we will have a table of r critical values that looks very similar to our t table, and we can compare our r directly to those. We calculate Pearson’s r using equations we’ve already established earlier in this chapter.
Pearson’s r
[latex]r=\frac{cov_{XY}}{(s_X)(s_Y)}[/latex]
Because of the duplication of information found in the denominators of the formulas for covariance and standard deviations for X and Y, we can simplify this formula a bit.
[latex]r=\frac{SP}{\sqrt{(SS_X)(SS_Y)}}[/latex]
Now, let’s look at an example.
Example: Anxiety and Depression
Anxiety and depression are often reported to be highly linked (or “comorbid”) psychological disorders. In this example we will ask the question: is there a significant positive relationship between anxiety and depression? That is, as symptoms of anxiety increase, do symptoms of depression also increase? We will see in this example that our hypothesis testing procedure follows the same four-step process as before, starting with our null and alternative hypotheses.
Step 1: State the Hypotheses
First, we need to decide if we are looking for directional or non-directional hypotheses. Remember that the null hypothesis is the idea that there is nothing interesting, notable, or impactful represented in our dataset. In a correlation, that takes the form of ‘no relationship’. Thus, our null hypothesis for can take one of three forms:
H 0 : There is no relationship
H 0 : ρ = 0
H 0 : There is not a positive relationship
H 0 : ρ < 0
H 0 : There is not a negative relationship
H 0 : ρ > 0
As with our other null hypotheses, we express the null hypothesis for a Pearson correlation in both words and mathematical notation. The exact wording of the written-out version should be changed to match whatever research question we are addressing (e.g. “ There is no relationship between anxiety and depression”). However, the mathematical version of the null hypothesis is always exactly the same: the lack of relationship is equal to zero. Our population parameter for the correlation is represented by ρ (“rho”), the Greek letter for lower case r . Obviously, correlational values can go up or down, but the null hypothesis states that these positive or negative values are just random chance and that the true correlation across all people is 0. Remember that if there is no relation between variables, the magnitude will be 0, which is where we get the null and alternative hypothesis values.
Our alternative hypotheses will also follow the same format that they did before: they can be directional if we suspect a relationship in a specific direction, or we can use an inequality sign to test for a relationship in any direction. Thus, our alternative hypothesis could take one of these three forms:
H A : There is a relationship
H A : ρ ≠ 0
H A : There is a positive relationship
H A : ρ > 0
H A : There is a negative relationship
H A : ρ < 0
As before, your choice of which alternative hypothesis to use should be specified before you collect data based on your research question and any evidence you might have that would indicate a specific directional (or non-directional) relationship. For our example, based on our research question, our null and alternative hypotheses would be:
H 0 : There is not a positive relationship between anxiety and depression
H A : There is a positive relationship between anxiety and depression
H A : ρ > 0
Step 2: Find the Critical Values
The critical values for correlations come from a critical value table just like before, some look very similar to a t -table (see figure 12). Just like a t -table, in the correlation table below, the column of critical values is based on our significance level (α) and the directionality of our test. The row is determined by our degrees of freedom. For correlations, we have n– 2 degrees of freedom, rather than n – 1 (why this is the case is not important at the moment). For our example, we have 10 people, so our degrees of freedom = 10 – 2 = 8. Some correlation tables use n instead of df so make sure you are paying attention to what information is required to find a critical value on the table that you are using.
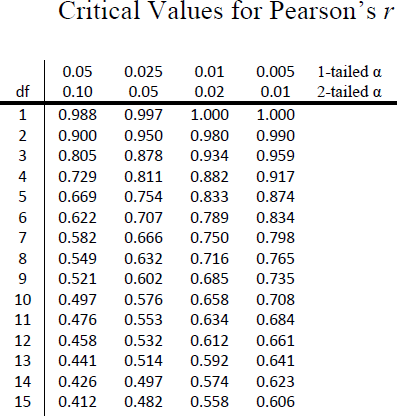
Figure 12. Example Correlation Critical Value Table
We were not given any information about the level of significance at which we should test our hypothesis, so we will assume α = 0.05 as default. From our table, we can see that a one-tailed test (because we expect only a positive relationship) at the α = 0.05 level with df = 8 has a critical value of r crit = 0.549. Thus, if our r test is greater than 0.549, it will be statistically significant. This is a rather high bar (remember, the guideline for a strong relationship is r = 0.50); this is because we have so few people. Larger samples make it much easier to find significant relationships (remember the law of large numbers?).
Step 3: Calculate the Test Statistic
We have laid out our hypotheses and the criteria we will use to assess them, so now we can move on to our test statistic. Before we do that, we must first create a scatterplot of the data to make sure that the most likely form of our relationship is in fact linear. Figure 13 below shows our data plotted out, and it looks like they are, in fact, linearly related, so Pearson’s r is appropriate.
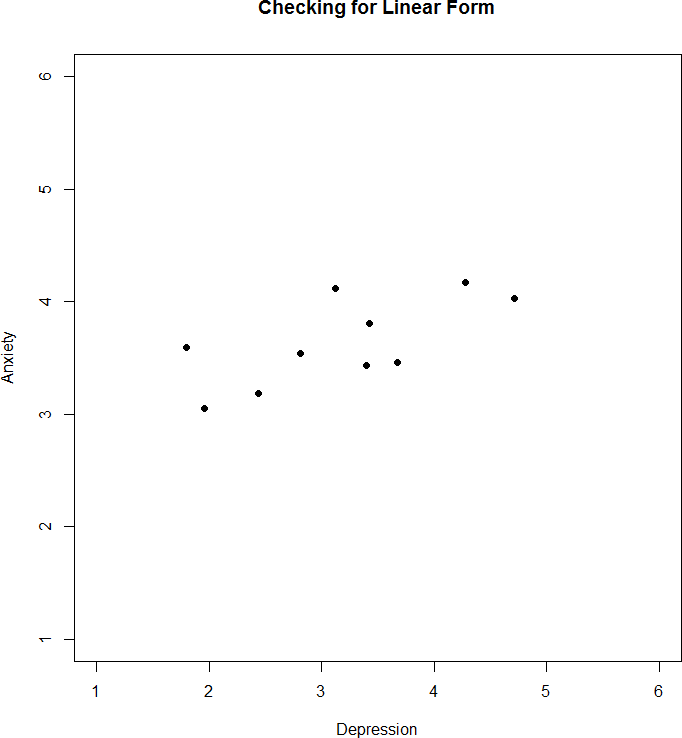
Figure 13. Scatterplot of anxiety and depression
The data we gather from our participants (n = 10) is as follows:
Dep (X) | 2.81 | 1.96 | 3.43 | 3.40 | 4.71 | 1.80 | 4.27 | 3.68 | 2.44 | 3.13 | M = 3.16 | s =0.89 | SS = 7.97 |
Anx (Y) | 3.54 | 3.05 | 3.81 | 3.43 | 4.03 | 3.59 | 4.17 | 3.46 | 3.19 | 4.12 | M = 3.64 | s = 0.37 | SS =1.33 |
Table 2. Data for step 3 to calculate r.
We will need to put these values into our Sum of Products table to calculate the standard deviation and covariance of our variables. We will use X for depression and Y for anxiety to keep track of our data, but be aware that this choice is arbitrary and the math will work out the same if we decided to do the opposite. Our table is thus:
X | (X −M ) | (X − M ) | Y | (Y − M ) | (Y − M ) | (X − M )(Y − M ) |
2.81 | -0.35 | 0.12 | 3.54 | -0.10 | 0.01 | 0.04 |
1.96 | -1.20 | 1.44 | 3.05 | -0.59 | 0.35 | 0.71 |
3.43 | 0.27 | 0.07 | 3.81 | 0.17 | 0.03 | 0.05 |
3.40 | 0.24 | 0.06 | 3.43 | -0.21 | 0.04 | -0.05 |
4.71 | 1.55 | 2.40 | 4.03 | 0.39 | 0.15 | 0.60 |
1.80 | -1.36 | 1.85 | 3.59 | -0.05 | 0.00 | 0.07 |
4.27 | 1.11 | 1.23 | 4.17 | 0.53 | 0.28 | 0.59 |
3.68 | 0.52 | 0.27 | 3.46 | -0.18 | 0.03 | -0.09 |
2.44 | -0.72 | 0.52 | 3.19 | -0.45 | 0.20 | 0.32 |
3.13 | -0.03 | 0.00 | 4.12 | 0.48 | 0.23 | -0.01 |
31.63 | 0.03 | = 7.97 | 36.39 | -0.01 | = 1.33 |
|
Table 3. Data for step 3 to calculate r using the definitional formula.
The bottom row is the sum of each column. We can see from this that the sum of the X observations is 31.63, which makes the mean of the X variable M X = 3.16. The deviation scores for X sum to 0.03, which is very close to 0, given rounding error, so everything looks right so far. The next column is the squared deviations for X, so we can see that the sum of squares for X is SS X = 7.97. The same is true of the Y columns, with an average of M Y = 3.64, deviations that sum to zero within rounding error, and a sum of squares as SS Y = 1.33. The final column is the product of our deviation scores (NOT of our squared deviations), which gives us a sum of products of SP = 2.22.
If we were to instead use the computational formula we would have the following table instead:
X | X | Y | Y | X*Y |
2.81 | 7.90 | 3.54 | 12.53 | 9.95 |
1.96 | 3.84 | 3.05 | 9.30 | 5.98 |
3.43 | 11.76 | 3.81 | 14.52 | 13.07 |
3.40 | 11.56 | 3.43 | 11.76 | 11.66 |
4.71 | 22.18 | 4.03 | 16.24 | 18.98 |
1.80 | 3.24 | 3.59 | 12.89 | 6.47 |
4.27 | 18.23 | 4.17 | 17.39 | 17.81 |
3.68 | 13.54 | 3.46 | 11.97 | 12.73 |
2.44 | 5.95 | 3.19 | 10.18 | 7.78 |
3.13 | 9.80 | 4.12 | 16.97 | 12.90 |
31.63 | 108.00 | 36.39 | 133.75 | 117.32 |
Table 4. Data for step 3 to calculate r using the computational formula.
To find the sum of product deviations using the computational formula we would now do the following:
[latex]SP=\Sigma(XY)-\frac{(\Sigma X)(\Sigma Y)}{n}=117.32-\frac{(31.63)(36.39)}{10}[/latex]
[latex]SP=117.32-\frac{1151.02}{10}=117.32-115.10=2.22[/latex]
We can also calculate our SS X and SS Y computationally using this single table.
[latex]SS_X=\Sigma(X^2)-\frac{(\Sigma X)^2}{n}=108.00-\frac{(31.63)^2}{10}[/latex]
[latex]SS_X=108.00-\frac{1000.46}{10}=108.00-100.05=7.95[/latex]
[latex]SS_Y=\Sigma(Y^2)-\frac{(\Sigma Y)^2}{n}=133.75-\frac{(36.39)^2}{10}[/latex]
[latex]SS_Y=133.75-\frac{1324.23}{10}=133.75-132.42=1.33[/latex]
As stated before and shown here, you will get the same (or at least really close to depending on rounding differences) answer for SP, SS X , and SS Y using the definitional or computational formulas.
We now have the three pieces of information we need to calculate our correlation coefficient, r: the covariance of X and Y and the variability of X and Y separately.
[latex]r=\frac{SP}{\sqrt{(SS_X)(SS_Y)}}=\frac{2.22}{\sqrt{(7.97)(1.33)}}[/latex]
[latex]r=\frac{2.22}{\sqrt{10.60}}=\frac{2.22}{3.26}=0.68[/latex]
So our observed correlation between anxiety and depression is r test = 0.68, which, based on sign and magnitude, is a strong, positive correlation. Now we need to compare it to our critical value to see if it is also statistically significant.
Step 4: Make a Decision
Our critical value was r crit = 0.549 and our obtained value was r = 0.68. Our test statistic value was larger than our critical value placing it in the critical region, so we can reject the null hypothesis.
Notice in our interpretation that, because we already know the magnitude and direction of our correlation, we can interpret that. We also report the degrees of freedom, just like with t , and we know that p < α because we rejected the null hypothesis. As we can see, even though we are dealing with a very different type of data, our process of hypothesis testing has remained unchanged.
Effect Size
Pearson’s r is an incredibly flexible and useful statistic. Not only is it both descriptive and inferential, as we saw above, but because it is on a standardized metric (always between -1.00 and 1.00), it can also serve as its own effect size. In general, we use r = 0.10, r = 0.30, and r = 0.50 as our guidelines for small, medium, and large effects. Just like with Cohen’s d , these guidelines are not absolutes, but they do serve as useful indicators in most situations. Notice as well that these are the same guidelines we used earlier to interpret the magnitude of the relation based on the correlation coefficient.
The similarities between η 2 and r 2 in interpretation and magnitude should clue you in to the fact that they are similar analyses, even if they look nothing alike. That is because, behind the scenes, they actually are! In the next chapter, we will learn a technique called Linear Regression , which will formally link the two analyses together.
Correlation versus Causation
We cover a great deal of material in introductory statistics and, as mentioned chapter 1, many of the principles underlying what we do in statistics can be used in your day-to-day life to help you interpret information objectively and make better decisions. We now come to what may be the most important lesson in introductory statistics: the difference between correlation and causation.
A Reminder about Experimental Design
When we say that one thing causes another, what do we mean? There is a long history in philosophy of discussion about the meaning of causality, but in statistics one way that we commonly think of causation is in terms of experimental control. That is, if we think that factor X causes factor Y, then manipulating the value of X should also change the value of Y.
Often we would like to test causal hypotheses but we can’t actually do an experiment, either because it’s impossible (“What is the relationship between human carbon emissions and the earth’s climate?”) or unethical (“What are the effects of severe abuse on child brain development?”). However, we can still collect data that might be relevant to those questions. For example, we can potentially collect data from children who have been abused as well as those who have not, and we can then ask whether their brain development differs.
Let’s say that we did such an analysis, and we found that abused children had poorer brain development than non-abused children. Would this demonstrate that abuse causes poorer brain development? No. Whenever we observe a statistical association between two variables, it is certainly possible that one of those two variables causes the other. However, it is also possible that both of the variables are being influenced by a third variable; in this example, it could be that child abuse is associated with family stress, which could also cause poorer brain development through less intellectual engagement, food stress, or many other possible avenues. The point is that a correlation between two variables generally tells us that something is probably causing something else, but it doesn’t tell us what is causing what.
Final Considerations
Correlations, although simple to calculate, can be very complex, and there are many additional issues we should consider. We will look at two of the most common issues that affect our correlations, as well as discuss some other correlations and reporting methods you may encounter.
Range Restriction
The strength of a correlation depends on how much variability is in each of the variables X and Y. This is evident in the formula for Pearson’s r , which uses both covariance (based on the sum of products, which comes from deviation scores) and the standard deviation of both variables (which are based on the sums of squares, which also come from deviation scores). Thus, if we reduce the amount of variability in one or both variables, our correlation will go down. Failure to capture the full variability of a variable is called range restriction.
Take a look at figures 14 and 15 below. The first shows a strong relationship ( r = 0.67) between two variables. The second shows the same data, but the bottom half of the X variable (all scores below 5) have been removed, which causes our relationship to become much weaker ( r = 0.38). Thus range restriction has truncated (made smaller) our observed correlation.
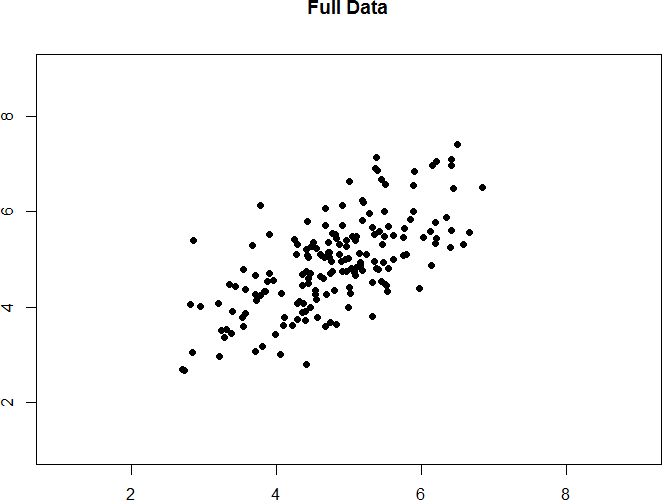
Figure 14. Strong, positive correlation.
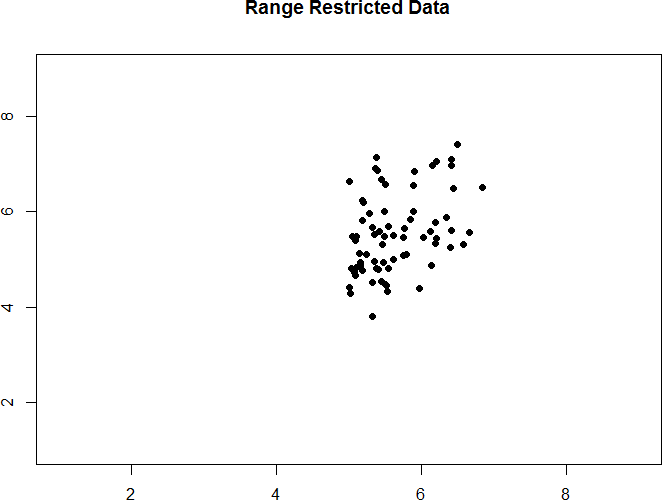
Figure 15. Effect of range restriction.
Sometimes range restriction happens by design. For example, we rarely hire people who do poorly on job applications, so we would not have the lower range of those predictor variables. Other times, we inadvertently cause range restriction by not properly sampling our population. Although there are ways to correct for range restriction, they are complicated and require much information that may not be known, so it is best to be very careful during the data collection process to avoid it.
Another issue that can cause the observed size of our correlation to be inappropriately large or small is the presence of outliers. An outlier is a data point that falls far away from the rest of the observations in the dataset. Sometimes outliers are the result of incorrect data entry, poor or intentionally misleading responses, or simple random chance. Other times, however, they represent real people with meaningful values on our variables. The distinction between meaningful and accidental outliers is a difficult one that is based on the expert judgment of the researcher. Sometimes, we will remove the outlier (if we think it is an accident) or we may decide to keep it (if we find the scores to still be meaningful even though they are different).
Pearson’s r is sensitive to outliers. For example, in Figure 16 we can see how a single outlying data point can cause a positive correlation value, even when the actual relationship between the other data points is negative.
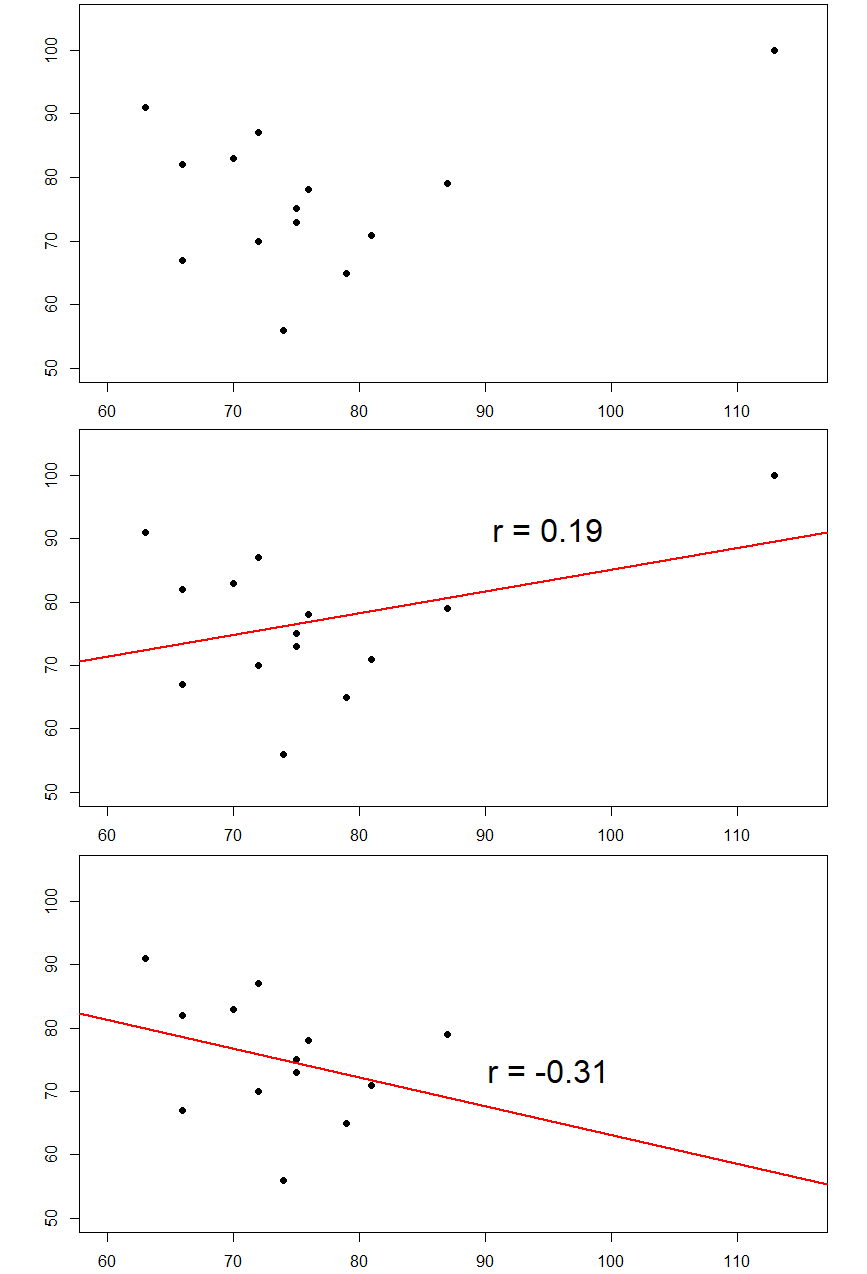
Figure 16. Three plots showing correlations with and without outliers.
In general, there are three effects that an outlier can have on a correlation: it can change the magnitude (make it stronger or weaker), it can change the significance (make a non-significant correlation significant or vice versa), and/or it can change the direction (make a positive relation negative or vice versa). Outliers are a big issue in small datasets where a single observation can have a strong weight compared to the rest. However, as our samples sizes get very large (into the hundreds), the effects of outliers diminishes because they are outweighed by the rest of the data. Nevertheless, no matter how large a dataset you have, it is always a good idea to screen for outliers, both statistically (using analyses that we do not cover here) and/or visually (using scatterplots).
Other Correlation Coefficients
In this chapter we have focused on Pearson’s r as our correlation coefficient because it very common and very useful. There are, however, many other correlations out there, each of which is designed for a different type of data. The most common of these is Spearman’s rho (ρ), which is designed to be used on ordinal data rather than continuous data. This is a very useful analysis if we have ranked data or our data. It can also be used when our continuous data do not conform to the normality assumption and our sample sizes are small. There are even more correlations for ordered categories, but they are much less common and beyond the scope of this chapter.
Additionally, the principles of correlations underlie many other advanced analyses. In the next chapter, we will learn about regression, which is a formal way of running and analyzing a correlation that can be extended to more than two variables. Regression is a very powerful technique that serves as the basis for even our most advanced statistical models, so what we have learned in this chapter will open the door to an entire world of possibilities in data analysis.
An misreported media example: Hate crimes and income inequality
In 2017, the web site Fivethirtyeight.com published a story titled Higher Rates Of Hate Crimes Are Tied To Income Inequality which discussed the relationship between the prevalence of hate crimes and income inequality in the wake of the 2016 Presidential election. The story reported an analysis of hate crime data from the FBI and the Southern Poverty Law Center, on the basis of which they report:
“we found that income inequality was the most significant determinant of population-adjusted hate crimes and hate incidents across the United States”.
The analysis reported in the story focused on the relationship between income inequality (defined by a quantity called the Gini index — see Appendix for more details) and the prevalence of hate crimes in each state.
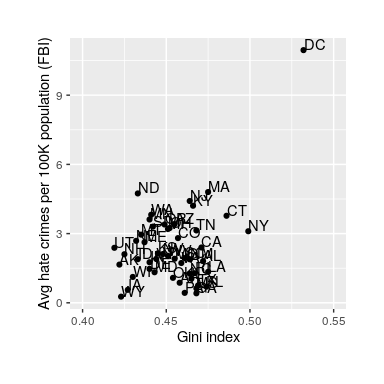
Figure 17: Plot of rates of hate crimes vs. Gini index.
The relationship between income inequality and rates of hate crimes is shown in Figure 17. Looking at the data, it seems that there may be a positive relationship between the two variables. The correlation value of 0.42 between hate crimes and income inequality seems to indicate a reasonably strong relationship between the two, but we can also imagine that this could occur by chance even if there is no relationship. We can test the null hypothesis that the correlation is zero using a statistical program (similar to our step 2). We get r (48) = .42, [.16,.63], p = .002. The numbers reported in the brackets are the 95% confidence interval for r. This test shows that the likelihood of an r value this extreme or more is quite low under the null hypothesis, so we would reject the null hypothesis that there is no relationship. Note that this test assumes that both variables are normally distributed. However, y ou may have noticed something a bit odd in Figure 17 – one of the datapoints (the one for the District of Columbia) seemed to be quite separate from the others. We refer to this as an outlier , and the standard correlation coefficient is very sensitive to outliers. When we calculate Spearman’s rho (ρ) (less sensitive to outliers), ρ = .033, p = .08. Now we see that the correlation is no longer significant (and in fact is very near zero), suggesting that the claims of the FiveThirtyEight blog post may have been incorrect due to the effect of the outlier.
Correlation Matrices
Many research studies look at the relationships between more than two continuous variables. In such situations, we could simply list all of our correlations, but that would take up a lot of space and make it difficult to quickly find the relationships we are looking for. Instead, we create correlation matrices so that we can quickly and simply display our results. A matrix is like a grid that contains our values. There is one row and one column for each of our variables, and the intersections of the rows and columns for different variables contain the correlation for those two variables. At the beginning of the chapter, we saw scatterplots presenting data for correlations between job satisfaction, well-being, burnout, and job performance. We can create a correlation matrix to quickly display the numerical values of each. Such a matrix is shown below.
Satisfaction | Well-Being | Burnout | Performance | |
Satisfaction | 1.00 | |||
Well-Being | 0.41 | 1.00 | ||
Burnout | -0.54 | -0.87 | 1.00 | |
Performance | 0.08 | 0.21 | -0.33 | 1.00 |
Table 5. Example Correlation Matrix
Notice that there are values of 1.00 where each row and column of the same variable intersect. This is because a variable correlates perfectly with itself, so the value is always exactly 1.00. Also notice that the upper cells are left blank and only the cells below the diagonal of 1s are filled in. This is because correlation matrices are symmetrical: they have the same values above the diagonal as below it. Filling in both sides would provide redundant information and make it a bit harder to read the matrix, so we leave the upper triangle blank. Correlation matrices are a very condensed way of presenting many results quickly, so they appear in almost all research studies that use continuous variables. Many matrices also include columns that show the variable means and standard deviations, as well as asterisks showing whether or not each correlation is statistically significant. Just remember that these can get quite big the more variables you have in your study.
Value of the correlation coefficient (r)
- The value of r is always between –1 and +1
- r = 1 represents a perfect positive correlation. A correlation of 1 indicates a perfect linear relationship.
- r = –1 represents a perfect negative correlation. A correlation of -1 indicates a perfect negative relationship.
- If r = 0 there is absolutely no linear relationship between x and y. A correlation of zero indicates no linear relationship.
Direction of the correlation coefficient ( r )
- A positive value of r means that when X increases, Y tends to increase; and when X decreases, Y tends to decrease (positive correlation).
- A negative value of r means that when X increases, Y tends to decrease; and when X decreases, Y tends to increase (negative correlation).
If two variables have a significant linear correlation we normally might assume that there is something causing them to go together. However, we cannot know the direction of causality (what is causing what) just from the fact that the two variables are correlated.
Consider this example, the relationship between doing exciting activities with your significant other and satisfaction with the relationship. There are three possible directions of causality for these two variables:
- X could be causing Y
- Y could be causing X
- Some third factor could be causing both X and Y
These three possible directions of causality are shown in the figure below (b).
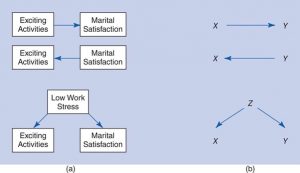
- Correlation is a relationship that has established that X and Y are related – if we know one then the other can be predicted but we cannot conclude that one variable causes the other.
- For example, when we apply heat (X) the temperature of water (Y) increases and when we remove heat (X) the temperature of water (Y) decreases.
characterized by two variables or attributes
consisting of or otherwise involving a number of distinct variables.
the value obtained by multiplying each pair of numbers in a set and then adding the individual totals.
a graphical representation of the relationship between two continuously measured variables in which one variable is arrayed on each axis and a dot or other symbol is placed at each point where the values of the variables intersect. The overall pattern of dots provides an indication of the extent to which there is a linear relationship between variables.
an association between two variables that when subjected to regression analysis and plotted on a graph forms a straight line. In linear relationships, the direction and rate of change in one variable are constant with respect to changes in the other variable.
describing an association between variables that does not consistently follow an increasing or decreasing pattern but rather changes direction after a certain point (i.e., it involves a curve in the set of data points).
an association between two variables such that they rise and fall in value together.
an association in which one variable decreases as the other variable increases, or vice versa. Also called inverse relationship.
a regression analysis in which the predictor or independent variables ( x s) are assumed to be related to the criterion or dependent variable ( y ) in such a manner that increases in an x variable result in consistent increases in the y variable. In other words, the direction and rate of change of one variable is constant with respect to changes in the other variable.
A confounding variable is a type of extraneous variable that will influence the outcome of the study. And so, researchers do their best to control these variables.
a situation in which variables are associated through their common relationship with one or more other variables but do not have a causal relationship with one another.
Observation or data point that does not fit the pattern of the rest of the data. Sometimes called an extreme value.
Introduction to Statistics for the Social Sciences Copyright © 2021 by Jennifer Ivie; Alicia MacKay is licensed under a Creative Commons Attribution-NonCommercial-ShareAlike 4.0 International License , except where otherwise noted.
Share This Book
Research Hypothesis In Psychology: Types, & Examples
Saul McLeod, PhD
Editor-in-Chief for Simply Psychology
BSc (Hons) Psychology, MRes, PhD, University of Manchester
Saul McLeod, PhD., is a qualified psychology teacher with over 18 years of experience in further and higher education. He has been published in peer-reviewed journals, including the Journal of Clinical Psychology.
Learn about our Editorial Process
Olivia Guy-Evans, MSc
Associate Editor for Simply Psychology
BSc (Hons) Psychology, MSc Psychology of Education
Olivia Guy-Evans is a writer and associate editor for Simply Psychology. She has previously worked in healthcare and educational sectors.
On This Page:
A research hypothesis, in its plural form “hypotheses,” is a specific, testable prediction about the anticipated results of a study, established at its outset. It is a key component of the scientific method .
Hypotheses connect theory to data and guide the research process towards expanding scientific understanding
Some key points about hypotheses:
- A hypothesis expresses an expected pattern or relationship. It connects the variables under investigation.
- It is stated in clear, precise terms before any data collection or analysis occurs. This makes the hypothesis testable.
- A hypothesis must be falsifiable. It should be possible, even if unlikely in practice, to collect data that disconfirms rather than supports the hypothesis.
- Hypotheses guide research. Scientists design studies to explicitly evaluate hypotheses about how nature works.
- For a hypothesis to be valid, it must be testable against empirical evidence. The evidence can then confirm or disprove the testable predictions.
- Hypotheses are informed by background knowledge and observation, but go beyond what is already known to propose an explanation of how or why something occurs.
Predictions typically arise from a thorough knowledge of the research literature, curiosity about real-world problems or implications, and integrating this to advance theory. They build on existing literature while providing new insight.
Types of Research Hypotheses
Alternative hypothesis.
The research hypothesis is often called the alternative or experimental hypothesis in experimental research.
It typically suggests a potential relationship between two key variables: the independent variable, which the researcher manipulates, and the dependent variable, which is measured based on those changes.
The alternative hypothesis states a relationship exists between the two variables being studied (one variable affects the other).
A hypothesis is a testable statement or prediction about the relationship between two or more variables. It is a key component of the scientific method. Some key points about hypotheses:
- Important hypotheses lead to predictions that can be tested empirically. The evidence can then confirm or disprove the testable predictions.
In summary, a hypothesis is a precise, testable statement of what researchers expect to happen in a study and why. Hypotheses connect theory to data and guide the research process towards expanding scientific understanding.
An experimental hypothesis predicts what change(s) will occur in the dependent variable when the independent variable is manipulated.
It states that the results are not due to chance and are significant in supporting the theory being investigated.
The alternative hypothesis can be directional, indicating a specific direction of the effect, or non-directional, suggesting a difference without specifying its nature. It’s what researchers aim to support or demonstrate through their study.
Null Hypothesis
The null hypothesis states no relationship exists between the two variables being studied (one variable does not affect the other). There will be no changes in the dependent variable due to manipulating the independent variable.
It states results are due to chance and are not significant in supporting the idea being investigated.
The null hypothesis, positing no effect or relationship, is a foundational contrast to the research hypothesis in scientific inquiry. It establishes a baseline for statistical testing, promoting objectivity by initiating research from a neutral stance.
Many statistical methods are tailored to test the null hypothesis, determining the likelihood of observed results if no true effect exists.
This dual-hypothesis approach provides clarity, ensuring that research intentions are explicit, and fosters consistency across scientific studies, enhancing the standardization and interpretability of research outcomes.
Nondirectional Hypothesis
A non-directional hypothesis, also known as a two-tailed hypothesis, predicts that there is a difference or relationship between two variables but does not specify the direction of this relationship.
It merely indicates that a change or effect will occur without predicting which group will have higher or lower values.
For example, “There is a difference in performance between Group A and Group B” is a non-directional hypothesis.
Directional Hypothesis
A directional (one-tailed) hypothesis predicts the nature of the effect of the independent variable on the dependent variable. It predicts in which direction the change will take place. (i.e., greater, smaller, less, more)
It specifies whether one variable is greater, lesser, or different from another, rather than just indicating that there’s a difference without specifying its nature.
For example, “Exercise increases weight loss” is a directional hypothesis.
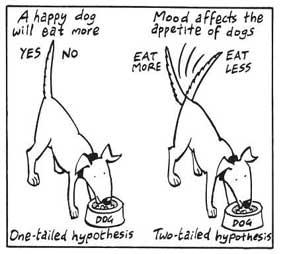
Falsifiability
The Falsification Principle, proposed by Karl Popper , is a way of demarcating science from non-science. It suggests that for a theory or hypothesis to be considered scientific, it must be testable and irrefutable.
Falsifiability emphasizes that scientific claims shouldn’t just be confirmable but should also have the potential to be proven wrong.
It means that there should exist some potential evidence or experiment that could prove the proposition false.
However many confirming instances exist for a theory, it only takes one counter observation to falsify it. For example, the hypothesis that “all swans are white,” can be falsified by observing a black swan.
For Popper, science should attempt to disprove a theory rather than attempt to continually provide evidence to support a research hypothesis.
Can a Hypothesis be Proven?
Hypotheses make probabilistic predictions. They state the expected outcome if a particular relationship exists. However, a study result supporting a hypothesis does not definitively prove it is true.
All studies have limitations. There may be unknown confounding factors or issues that limit the certainty of conclusions. Additional studies may yield different results.
In science, hypotheses can realistically only be supported with some degree of confidence, not proven. The process of science is to incrementally accumulate evidence for and against hypothesized relationships in an ongoing pursuit of better models and explanations that best fit the empirical data. But hypotheses remain open to revision and rejection if that is where the evidence leads.
- Disproving a hypothesis is definitive. Solid disconfirmatory evidence will falsify a hypothesis and require altering or discarding it based on the evidence.
- However, confirming evidence is always open to revision. Other explanations may account for the same results, and additional or contradictory evidence may emerge over time.
We can never 100% prove the alternative hypothesis. Instead, we see if we can disprove, or reject the null hypothesis.
If we reject the null hypothesis, this doesn’t mean that our alternative hypothesis is correct but does support the alternative/experimental hypothesis.
Upon analysis of the results, an alternative hypothesis can be rejected or supported, but it can never be proven to be correct. We must avoid any reference to results proving a theory as this implies 100% certainty, and there is always a chance that evidence may exist which could refute a theory.
How to Write a Hypothesis
- Identify variables . The researcher manipulates the independent variable and the dependent variable is the measured outcome.
- Operationalized the variables being investigated . Operationalization of a hypothesis refers to the process of making the variables physically measurable or testable, e.g. if you are about to study aggression, you might count the number of punches given by participants.
- Decide on a direction for your prediction . If there is evidence in the literature to support a specific effect of the independent variable on the dependent variable, write a directional (one-tailed) hypothesis. If there are limited or ambiguous findings in the literature regarding the effect of the independent variable on the dependent variable, write a non-directional (two-tailed) hypothesis.
- Make it Testable : Ensure your hypothesis can be tested through experimentation or observation. It should be possible to prove it false (principle of falsifiability).
- Clear & concise language . A strong hypothesis is concise (typically one to two sentences long), and formulated using clear and straightforward language, ensuring it’s easily understood and testable.
Consider a hypothesis many teachers might subscribe to: students work better on Monday morning than on Friday afternoon (IV=Day, DV= Standard of work).
Now, if we decide to study this by giving the same group of students a lesson on a Monday morning and a Friday afternoon and then measuring their immediate recall of the material covered in each session, we would end up with the following:
- The alternative hypothesis states that students will recall significantly more information on a Monday morning than on a Friday afternoon.
- The null hypothesis states that there will be no significant difference in the amount recalled on a Monday morning compared to a Friday afternoon. Any difference will be due to chance or confounding factors.
More Examples
- Memory : Participants exposed to classical music during study sessions will recall more items from a list than those who studied in silence.
- Social Psychology : Individuals who frequently engage in social media use will report higher levels of perceived social isolation compared to those who use it infrequently.
- Developmental Psychology : Children who engage in regular imaginative play have better problem-solving skills than those who don’t.
- Clinical Psychology : Cognitive-behavioral therapy will be more effective in reducing symptoms of anxiety over a 6-month period compared to traditional talk therapy.
- Cognitive Psychology : Individuals who multitask between various electronic devices will have shorter attention spans on focused tasks than those who single-task.
- Health Psychology : Patients who practice mindfulness meditation will experience lower levels of chronic pain compared to those who don’t meditate.
- Organizational Psychology : Employees in open-plan offices will report higher levels of stress than those in private offices.
- Behavioral Psychology : Rats rewarded with food after pressing a lever will press it more frequently than rats who receive no reward.

- Skip to secondary menu
- Skip to main content
- Skip to primary sidebar
Statistics By Jim
Making statistics intuitive
One-Tailed and Two-Tailed Hypothesis Tests Explained
By Jim Frost 61 Comments
Choosing whether to perform a one-tailed or a two-tailed hypothesis test is one of the methodology decisions you might need to make for your statistical analysis. This choice can have critical implications for the types of effects it can detect, the statistical power of the test, and potential errors.
In this post, you’ll learn about the differences between one-tailed and two-tailed hypothesis tests and their advantages and disadvantages. I include examples of both types of statistical tests. In my next post, I cover the decision between one and two-tailed tests in more detail.
What Are Tails in a Hypothesis Test?
First, we need to cover some background material to understand the tails in a test. Typically, hypothesis tests take all of the sample data and convert it to a single value, which is known as a test statistic. You’re probably already familiar with some test statistics. For example, t-tests calculate t-values . F-tests, such as ANOVA, generate F-values . The chi-square test of independence and some distribution tests produce chi-square values. All of these values are test statistics. For more information, read my post about Test Statistics .
These test statistics follow a sampling distribution. Probability distribution plots display the probabilities of obtaining test statistic values when the null hypothesis is correct. On a probability distribution plot, the portion of the shaded area under the curve represents the probability that a value will fall within that range.
The graph below displays a sampling distribution for t-values. The two shaded regions cover the two-tails of the distribution.

Keep in mind that this t-distribution assumes that the null hypothesis is correct for the population. Consequently, the peak (most likely value) of the distribution occurs at t=0, which represents the null hypothesis in a t-test. Typically, the null hypothesis states that there is no effect. As t-values move further away from zero, it represents larger effect sizes. When the null hypothesis is true for the population, obtaining samples that exhibit a large apparent effect becomes less likely, which is why the probabilities taper off for t-values further from zero.
Related posts : How t-Tests Work and Understanding Probability Distributions
Critical Regions in a Hypothesis Test
In hypothesis tests, critical regions are ranges of the distributions where the values represent statistically significant results. Analysts define the size and location of the critical regions by specifying both the significance level (alpha) and whether the test is one-tailed or two-tailed.
Consider the following two facts:
- The significance level is the probability of rejecting a null hypothesis that is correct.
- The sampling distribution for a test statistic assumes that the null hypothesis is correct.
Consequently, to represent the critical regions on the distribution for a test statistic, you merely shade the appropriate percentage of the distribution. For the common significance level of 0.05, you shade 5% of the distribution.
Related posts : Significance Levels and P-values and T-Distribution Table of Critical Values
Two-Tailed Hypothesis Tests
Two-tailed hypothesis tests are also known as nondirectional and two-sided tests because you can test for effects in both directions. When you perform a two-tailed test, you split the significance level percentage between both tails of the distribution. In the example below, I use an alpha of 5% and the distribution has two shaded regions of 2.5% (2 * 2.5% = 5%).
When a test statistic falls in either critical region, your sample data are sufficiently incompatible with the null hypothesis that you can reject it for the population.
In a two-tailed test, the generic null and alternative hypotheses are the following:
- Null : The effect equals zero.
- Alternative : The effect does not equal zero.
The specifics of the hypotheses depend on the type of test you perform because you might be assessing means, proportions, or rates.

Example of a two-tailed 1-sample t-test
Suppose we perform a two-sided 1-sample t-test where we compare the mean strength (4.1) of parts from a supplier to a target value (5). We use a two-tailed test because we care whether the mean is greater than or less than the target value.
To interpret the results, simply compare the p-value to your significance level. If the p-value is less than the significance level, you know that the test statistic fell into one of the critical regions, but which one? Just look at the estimated effect. In the output below, the t-value is negative, so we know that the test statistic fell in the critical region in the left tail of the distribution, indicating the mean is less than the target value. Now we know this difference is statistically significant.

We can conclude that the population mean for part strength is less than the target value. However, the test had the capacity to detect a positive difference as well. You can also assess the confidence interval. With a two-tailed hypothesis test, you’ll obtain a two-sided confidence interval. The confidence interval tells us that the population mean is likely to fall between 3.372 and 4.828. This range excludes the target value (5), which is another indicator of significance.
Advantages of two-tailed hypothesis tests
You can detect both positive and negative effects. Two-tailed tests are standard in scientific research where discovering any type of effect is usually of interest to researchers.
One-Tailed Hypothesis Tests
One-tailed hypothesis tests are also known as directional and one-sided tests because you can test for effects in only one direction. When you perform a one-tailed test, the entire significance level percentage goes into the extreme end of one tail of the distribution.
In the examples below, I use an alpha of 5%. Each distribution has one shaded region of 5%. When you perform a one-tailed test, you must determine whether the critical region is in the left tail or the right tail. The test can detect an effect only in the direction that has the critical region. It has absolutely no capacity to detect an effect in the other direction.
In a one-tailed test, you have two options for the null and alternative hypotheses, which corresponds to where you place the critical region.
You can choose either of the following sets of generic hypotheses:
- Null : The effect is less than or equal to zero.
- Alternative : The effect is greater than zero.

- Null : The effect is greater than or equal to zero.
- Alternative : The effect is less than zero.

Again, the specifics of the hypotheses depend on the type of test you perform.
Notice how for both possible null hypotheses the tests can’t distinguish between zero and an effect in a particular direction. For example, in the example directly above, the null combines “the effect is greater than or equal to zero” into a single category. That test can’t differentiate between zero and greater than zero.
Example of a one-tailed 1-sample t-test
Suppose we perform a one-tailed 1-sample t-test. We’ll use a similar scenario as before where we compare the mean strength of parts from a supplier (102) to a target value (100). Imagine that we are considering a new parts supplier. We will use them only if the mean strength of their parts is greater than our target value. There is no need for us to differentiate between whether their parts are equally strong or less strong than the target value—either way we’d just stick with our current supplier.
Consequently, we’ll choose the alternative hypothesis that states the mean difference is greater than zero (Population mean – Target value > 0). The null hypothesis states that the difference between the population mean and target value is less than or equal to zero.

To interpret the results, compare the p-value to your significance level. If the p-value is less than the significance level, you know that the test statistic fell into the critical region. For this study, the statistically significant result supports the notion that the population mean is greater than the target value of 100.
Confidence intervals for a one-tailed test are similarly one-sided. You’ll obtain either an upper bound or a lower bound. In this case, we get a lower bound, which indicates that the population mean is likely to be greater than or equal to 100.631. There is no upper limit to this range.
A lower-bound matches our goal of determining whether the new parts are stronger than our target value. The fact that the lower bound (100.631) is higher than the target value (100) indicates that these results are statistically significant.
This test is unable to detect a negative difference even when the sample mean represents a very negative effect.
Advantages and disadvantages of one-tailed hypothesis tests
One-tailed tests have more statistical power to detect an effect in one direction than a two-tailed test with the same design and significance level. One-tailed tests occur most frequently for studies where one of the following is true:
- Effects can exist in only one direction.
- Effects can exist in both directions but the researchers only care about an effect in one direction. There is no drawback to failing to detect an effect in the other direction. (Not recommended.)
The disadvantage of one-tailed tests is that they have no statistical power to detect an effect in the other direction.
As part of your pre-study planning process, determine whether you’ll use the one- or two-tailed version of a hypothesis test. To learn more about this planning process, read 5 Steps for Conducting Scientific Studies with Statistical Analyses .
This post explains the differences between one-tailed and two-tailed statistical hypothesis tests. How these forms of hypothesis tests function is clear and based on mathematics. However, there is some debate about when you can use one-tailed tests. My next post explores this decision in much more depth and explains the different schools of thought and my opinion on the matter— When Can I Use One-Tailed Hypothesis Tests .
If you’re learning about hypothesis testing and like the approach I use in my blog, check out my Hypothesis Testing book! You can find it at Amazon and other retailers.

Share this:

Reader Interactions
August 23, 2024 at 1:28 pm
Thank so much. This is very helpfull
June 26, 2022 at 12:14 pm
Hi, Can help me with figuring out the null and alternative hypothesis of the following statement? Some claimed that the real average expenditure on beverage by general people is at least $10.
February 19, 2022 at 6:02 am
thank you for the thoroughly explanation, I’m still strugling to wrap my mind around the t-table and the relation between the alpha values for one or two tail probability and the confidence levels on the bottom (I’m understanding it so wrongly that for me it should be the oposite, like one tail 0,05 should correspond 95% CI and two tailed 0,025 should correspond to 95% because then you got the 2,5% on each side). In my mind if I picture the one tail diagram with an alpha of 0,05 I see the rest 95% inside the diagram, but for a one tail I only see 90% CI paired with a 5% alpha… where did the other 5% go? I tried to understand when you said we should just double the alpha for a one tail probability in order to find the CI but I still cant picture it. I have been trying to understand this. Like if you only have one tail and there is 0,05, shouldn’t the rest be on the other side? why is it then 90%… I know I’m missing a point and I can’t figure it out and it’s so frustrating…
February 23, 2022 at 10:01 pm
The alpha is the total shaded area. So, if the alpha = 0.05, you know that 5% of the distribution is shaded. The number of tails tells you how to divide the shaded areas. Is it all in one region (1-tailed) or do you split the shaded regions in two (2-tailed)?
So, for a one-tailed test with an alpha of 0.05, the 5% shading is all in one tail. If alpha = 0.10, then it’s 10% on one side. If it’s two-tailed, then you need to split that 10% into two–5% in both tails. Hence, the 5% in a one-tailed test is the same as a two-tailed test with an alpha of 0.10 because that test has the same 5% on one side (but there’s another 5% in the other tail).
It’s similar for CIs. However, for CIs, you shade the middle rather than the extremities. I write about that in one my articles about hypothesis testing and confidence intervals .
I’m not sure if I’m answering your question or not.
February 17, 2022 at 1:46 pm
I ran a post hoc Dunnett’s test alpha=0.05 after a significant Anova test in Proc Mixed using SAS. I want to determine if the means for treatment (t1, t2, t3) is significantly less than the means for control (p=pathogen). The code for the dunnett’s test is – LSmeans trt / diff=controll (‘P’) adjust=dunnett CL plot=control; I think the lower bound one tailed test is the correct test to run but I’m not 100% sure. I’m finding conflicting information online. In the output table for the dunnett’s test the mean difference between the control and the treatments is t1=9.8, t2=64.2, and t3=56.5. The control mean estimate is 90.5. The adjusted p-value by treatment is t1(p=0.5734), t2 (p=.0154) and t3(p=.0245). The adjusted lower bound confidence limit in order from t1-t3 is -38.8, 13.4, and 7.9. The adjusted upper bound for all test is infinity. The graphical output for the dunnett’s test in SAS is difficult to understand for those of us who are beginner SAS users. All treatments appear as a vertical line below the the horizontal line for control at 90.5 with t2 and t3 in the shaded area. For treatment 1 the shaded area is above the line for control. Looking at just the output table I would say that t2 and t3 are significantly lower than the control. I guess I would like to know if my interpretation of the outputs is correct that treatments 2 and 3 are statistically significantly lower than the control? Should I have used an upper bound one tailed test instead?
November 10, 2021 at 1:00 am
Thanks Jim. Please help me understand how a two tailed testing can be used to minimize errors in research
July 1, 2021 at 9:19 am
Hi Jim, Thanks for posting such a thorough and well-written explanation. It was extremely useful to clear up some doubts.
May 7, 2021 at 4:27 pm
Hi Jim, I followed your instructions for the Excel add-in. Thank you. I am very new to statistics and sort of enjoy it as I enter week number two in my class. I am to select if three scenarios call for a one or two-tailed test is required and why. The problem is stated:
30% of mole biopsies are unnecessary. Last month at his clinic, 210 out of 634 had benign biopsy results. Is there enough evidence to reject the dermatologist’s claim?
Part two, the wording changes to “more than of 30% of biopsies,” and part three, the wording changes to “less than 30% of biopsies…”
I am not asking for the problem to be solved for me, but I cannot seem to find direction needed. I know the elements i am dealing with are =30%, greater than 30%, and less than 30%. 210 and 634. I just don’t know what to with the information. I can’t seem to find an example of a similar problem to work with.
May 9, 2021 at 9:22 pm
As I detail in this post, a two-tailed test tells you whether an effect exists in either direction. Or, is it different from the null value in either direction. For the first example, the wording suggests you’d need a two-tailed test to determine whether the population proportion is ≠ 30%. Whenever you just need to know ≠, it suggests a two-tailed test because you’re covering both directions.
For part two, because it’s in one direction (greater than), you need a one-tailed test. Same for part three but it’s less than. Look in this blog post to see how you’d construct the null and alternative hypotheses for these cases. Note that you’re working with a proportion rather than the mean, but the principles are the same! Just plug your scenario and the concept of proportion into the wording I use for the hypotheses.
I hope that helps!
April 11, 2021 at 9:30 am
Hello Jim, great website! I am using a statistics program (SPSS) that does NOT compute one-tailed t-tests. I am trying to compare two independent groups and have justifiable reasons why I only care about one direction. Can I do the following? Use SPSS for two-tailed tests to calculate the t & p values. Then report the p-value as p/2 when it is in the predicted direction (e.g , SPSS says p = .04, so I report p = .02), and report the p-value as 1 – (p/2) when it is in the opposite direction (e.g., SPSS says p = .04, so I report p = .98)? If that is incorrect, what do you suggest (hopefully besides changing statistics programs)? Also, if I want to report confidence intervals, I realize that I would only have an upper or lower bound, but can I use the CI’s from SPSS to compute that? Thank you very much!
April 11, 2021 at 5:42 pm
Yes, for p-values, that’s absolutely correct for both cases.
For confidence intervals, if you take one endpoint of a two-side CI, it becomes a one-side bound with half the confidence level.
Consequently, to obtain a one-sided bound with your desired confidence level, you need to take your desired significance level (e.g., 0.05) and double it. Then subtract it from 1. So, if you’re using a significance level of 0.05, double that to 0.10 and then subtract from 1 (1 – 0.10 = 0.90). 90% is the confidence level you want to use for a two-sided test. After obtaining the two-sided CI, use one of the endpoints depending on the direction of your hypothesis (i.e., upper or lower bound). That’s produces the one-sided the bound with the confidence level that you want. For our example, we calculated a 95% one-sided bound.
March 3, 2021 at 8:27 am
Hi Jim. I used the one-tailed(right) statistical test to determine an anomaly in the below problem statement: On a daily basis, I calculate the (mapped_%) in a common field between two tables.
The way I used the t-test is: On any particular day, I calculate the sample_mean, S.D and sample_count (n=30) for the last 30 days including the current day. My null hypothesis, H0 (pop. mean)=95 and H1>95 (alternate hypothesis). So, I calculate the t-stat based on the sample_mean, pop.mean, sample S.D and n. I then choose the t-crit value for 0.05 from my t-ditribution table for dof(n-1). On the current day if my abs.(t-stat)>t-crit, then I reject the null hypothesis and I say the mapped_pct on that day has passed the t-test.
I get some weird results here, where if my mapped_pct is as low as 6%-8% in all the past 30 days, the t-test still gets a “pass” result. Could you help on this? If my hypothesis needs to be changed.
I would basically look for the mapped_pct >95, if it worked on a static trigger. How can I use the t-test effectively in this problem statement?
December 18, 2020 at 8:23 pm
Hello Dr. Jim, I am wondering if there is evidence in one of your books or other source you could provide, which supports that it is OK not to divide alpha level by 2 in one-tailed hypotheses. I need the source for supporting evidence in a Portfolio exercise and couldn’t find one.
I am grateful for your reply and for your statistics knowledge sharing!
November 27, 2020 at 10:31 pm
If I did a one directional F test ANOVA(one tail ) and wanted to calculate a confidence interval for each individual groups (3) mean . Would I use a one tailed or two tailed t , within my confidence interval .
November 29, 2020 at 2:36 am
Hi Bashiru,
F-tests for ANOVA will always be one-tailed for the reasons I discuss in this post. To learn more about, read my post about F-tests in ANOVA .
For the differences between my groups, I would not use t-tests because the family-wise error rate quickly grows out of hand. To learn more about how to compare group means while controlling the familywise error rate, read my post about using post hoc tests with ANOVA . Typically, these are two-side intervals but you’d be able to use one-sided.
November 26, 2020 at 10:51 am
Hi Jim, I had a question about the formulation of the hypotheses. When you want to test if a beta = 1 or a beta = 0. What will be the null hypotheses? I’m having trouble with finding out. Because in most cases beta = 0 is the null hypotheses but in this case you want to test if beta = 0. so i’m having my doubts can it in this case be the alternative hypotheses or is it still the null hypotheses?
Kind regards, Noa
November 27, 2020 at 1:21 am
Typically, the null hypothesis represents no effect or no relationship. As an analyst, you’re hoping that your data have enough evidence to reject the null and favor the alternative.
Assuming you’re referring to beta as in regression coefficients, zero represents no relationship. Consequently, beta = 0 is the null hypothesis.
You might hope that beta = 1, but you don’t usually include that in your alternative hypotheses. The alternative hypothesis usually states that it does not equal no effect. In other words, there is an effect but it doesn’t state what it is.
There are some exceptions to the above but I’m writing about the standard case.
November 22, 2020 at 8:46 am
Your articles are a help to intro to econometrics students. Keep up the good work! More power to you!
November 6, 2020 at 11:25 pm
Hello Jim. Can you help me with these please?
Write the null and alternative hypothesis using a 1-tailed and 2-tailed test for each problem. (In paragraph and symbols)
A teacher wants to know if there is a significant difference in the performance in MAT C313 between her morning and afternoon classes.
It is known that in our university canteen, the average waiting time for a customer to receive and pay for his/her order is 20 minutes. Additional personnel has been added and now the management wants to know if the average waiting time had been reduced.
November 8, 2020 at 12:29 am
I cover how to write the hypotheses for the different types of tests in this post. So, you just need to figure which type of test you need to use. In your case, you want to determine whether the mean waiting time is less than the target value of 20 minutes. That’s a 1-sample t-test because you’re comparing a mean to a target value (20 minutes). You specifically want to determine whether the mean is less than the target value. So, that’s a one-tailed test. And, you’re looking for a mean that is “less than” the target.
So, go to the one-tailed section in the post and look for the hypotheses for the effect being less than. That’s the one with the critical region on the left side of the curve.
Now, you need include your own information. In your case, you’re comparing the sample estimate to a population mean of 20. The 20 minutes is your null hypothesis value. Use the symbol mu μ to represent the population mean.
You put all that together and you get the following:
Null: μ ≥ 20 Alternative: μ 0 to denote the null hypothesis and H 1 or H A to denote the alternative hypothesis if that’s what you been using in class.
October 17, 2020 at 12:11 pm
I was just wondering if you could please help with clarifying what the hypothesises would be for say income for gamblers and, age of gamblers. I am struggling to find which means would be compared.
October 17, 2020 at 7:05 pm
Those are both continuous variables, so you’d use either correlation or regression for them. For both of those analyses, the hypotheses are the following:
Null : The correlation or regression coefficient equals zero (i.e., there is no relationship between the variables) Alternative : The coefficient does not equal zero (i.e., there is a relationship between the variables.)
When the p-value is less than your significance level, you reject the null and conclude that a relationship exists.
October 17, 2020 at 3:05 am
I was ask to choose and justify the reason between a one tailed and two tailed test for dummy variables, how do I do that and what does it mean?
October 17, 2020 at 7:11 pm
I don’t have enough information to answer your question. A dummy variable is also known as an indicator variable, which is a binary variable that indicates the presence or absence of a condition or characteristic. If you’re using this variable in a hypothesis test, I’d presume that you’re using a proportions test, which is based on the binomial distribution for binary data.
Choosing between a one-tailed or two-tailed test depends on subject area issues and, possibly, your research objectives. Typically, use a two-tailed test unless you have a very good reason to use a one-tailed test. To understand when you might use a one-tailed test, read my post about when to use a one-tailed hypothesis test .
October 16, 2020 at 2:07 pm
In your one-tailed example, Minitab describes the hypotheses as “Test of mu = 100 vs > 100”. Any idea why Minitab says the null is “=” rather than “= or less than”? No ASCII character for it?
October 16, 2020 at 4:20 pm
I’m not entirely sure even though I used to work there! I know we had some discussions about how to represent that hypothesis but I don’t recall the exact reasoning. I suspect that it has to do with the conclusions that you can draw. Let’s focus on the failing to reject the null hypothesis. If the test statistic falls in that region (i.e., it is not significant), you fail to reject the null. In this case, all you know is that you have insufficient evidence to say it is different than 100. I’m pretty sure that’s why they use the equal sign because it might as well be one.
Mathematically, I think using ≤ is more accurate, which you can really see when you look at the distribution plots. That’s why I phrase the hypotheses using ≤ or ≥ as needed. However, in terms of the interpretation, the “less than” portion doesn’t really add anything of importance. You can conclude that its equal to 100 or greater than 100, but not less than 100.
October 15, 2020 at 5:46 am
Thank you so much for your timely feedback. It helps a lot
October 14, 2020 at 10:47 am
How can i use one tailed test at 5% alpha on this problem?
A manufacturer of cellular phone batteries claims that when fully charged, the mean life of his product lasts for 26 hours with a standard deviation of 5 hours. Mr X, a regular distributor, randomly picked and tested 35 of the batteries. His test showed that the average life of his sample is 25.5 hours. Is there a significant difference between the average life of all the manufacturer’s batteries and the average battery life of his sample?
October 14, 2020 at 8:22 pm
I don’t think you’d want to use a one-tailed test. The goal is to determine whether the sample is significantly different than the manufacturer’s population average. You’re not saying significantly greater than or less than, which would be a one-tailed test. As phrased, you want a two-tailed test because it can detect a difference in either direct.
It sounds like you need to use a 1-sample t-test to test the mean. During this test, enter 26 as the test mean. The procedure will tell you if the sample mean of 25.5 hours is a significantly different from that test mean. Similarly, you’d need a one variance test to determine whether the sample standard deviation is significantly different from the test value of 5 hours.
For both of these tests, compare the p-value to your alpha of 0.05. If the p-value is less than this value, your results are statistically significant.
September 22, 2020 at 4:16 am
Hi Jim, I didn’t get an idea that when to use two tail test and one tail test. Will you please explain?
September 22, 2020 at 10:05 pm
I have a complete article dedicated to that: When Can I Use One-Tailed Tests .
Basically, start with the assumption that you’ll use a two-tailed test but then consider scenarios where a one-tailed test can be appropriate. I talk about all of that in the article.
If you have questions after reading that, please don’t hesitate to ask!
July 31, 2020 at 12:33 pm
Thank you so so much for this webpage.
I have two scenarios that I need some clarification. I will really appreciate it if you can take a look:
So I have several of materials that I know when they are tested after production. My hypothesis is that the earlier they are tested after production, the higher the mean value I should expect. At the same time, the later they are tested after production, the lower the mean value. Since this is more like a “greater or lesser” situation, I should use one tail. Is that the correct approach?
On the other hand, I have several mix of materials that I don’t know when they are tested after production. I only know the mean values of the test. And I only want to know whether one mean value is truly higher or lower than the other, I guess I want to know if they are only significantly different. Should I use two tail for this? If they are not significantly different, I can judge based on the mean values of test alone. And if they are significantly different, then I will need to do other type of analysis. Also, when I get my P-value for two tail, should I compare it to 0.025 or 0.05 if my confidence level is 0.05?
Thank you so much again.
July 31, 2020 at 11:19 pm
For your first, if you absolutely know that the mean must be lower the later the material is tested, that it cannot be higher, that would be a situation where you can use a one-tailed test. However, if that’s not a certainty, you’re just guessing, use a two-tail test. If you’re measuring different items at the different times, use the independent 2-sample t-test. However, if you’re measuring the same items at two time points, use the paired t-test. If it’s appropriate, using the paired t-test will give you more statistical power because it accounts for the variability between items. For more information, see my post about when it’s ok to use a one-tailed test .
For the mix of materials, use a two-tailed test because the effect truly can go either direction.
Always compare the p-value to your full significance level regardless of whether it’s a one or two-tailed test. Don’t divide the significance level in half.
June 17, 2020 at 2:56 pm
Is it possible that we reach to opposite conclusions if we use a critical value method and p value method Secondly if we perform one tail test and use p vale method to conclude our Ho, then do we need to convert sig value of 2 tail into sig value of one tail. That can be done just by dividing it with 2
June 18, 2020 at 5:17 pm
The p-value method and critical value method will always agree as long as you’re not changing anything about how the methodology.
If you’re using statistical software, you don’t need to make any adjustments. The software will do that for you.
However, if you calculating it by hand, you’ll need to take your significance level and then look in the table for your test statistic for a one-tailed test. For example, you’ll want to look up 5% for a one-tailed test rather than a two-tailed test. That’s not as simple as dividing by two. In this article, I show examples of one-tailed and two-tailed tests for the same degrees of freedom. The t critical value for the two-tailed test is +/- 2.086 while for the one-sided test it is 1.725. It is true that probability associated with those critical values doubles for the one-tailed test (2.5% -> 5%), but the critical value itself is not half (2.086 -> 1.725). Study the first several graphs in this article to see why that is true.
For the p-value, you can take a two-tailed p-value and divide by 2 to determine the one-sided p-value. However, if you’re using statistical software, it does that for you.
June 11, 2020 at 3:46 pm
Hello Jim, if you have the time I’d be grateful if you could shed some clarity on this scenario:
“A researcher believes that aromatherapy can relieve stress but wants to determine whether it can also enhance focus. To test this, the researcher selected a random sample of students to take an exam in which the average score in the general population is 77. Prior to the exam, these students studied individually in a small library room where a lavender scent was present. If students in this group scored significantly above the average score in general population [is this one-tailed or two-tailed hypothesis?], then this was taken as evidence that the lavender scent enhanced focus.”
Thank you for your time if you do decide to respond.
June 11, 2020 at 4:00 pm
It’s unclear from the information provided whether the researchers used a one-tailed or two-tailed test. It could be either. A two-tailed test can detect effects in both directions, so it could definitely detect an average group score above the population score. However, you could also detect that effect using a one-tailed test if it was set up correctly. So, there’s not enough information in what you provided to know for sure. It could be either.
However, that’s irrelevant to answering the question. The tricky part, as I see it, is that you’re not entirely sure about why the scores are higher. Are they higher because the lavender scent increased concentration or are they higher because the subjects have lower stress from the lavender? Or, maybe it’s not even related to the scent but some other characteristic of the room or testing conditions in which they took the test. You just know the scores are higher but not necessarily why they’re higher.
I’d say that, no, it’s not necessarily evidence that the lavender scent enhanced focus. There are competing explanations for why the scores are higher. Also, it would be best do this as an experiment with a control and treatment group where subjects are randomly assigned to either group. That process helps establish causality rather than just correlation and helps rules out competing explanations for why the scores are higher.
By the way, I spend a lot of time on these issues in my Introduction to Statistics ebook .
June 9, 2020 at 1:47 pm
If a left tail test has an alpha value of 0.05 how will you find the value in the table
April 19, 2020 at 10:35 am
Hi Jim, My question is in regards to the results in the table in your example of the one-sample T (Two-Tailed) test. above. What about the P-value? The P-value listed is .018. I assuming that is compared to and alpha of 0.025, correct?
In regression analysis, when I get a test statistic for the predictive variable of -2.099 and a p-value of 0.039. Am I comparing the p-value to an alpha of 0.025 or 0.05? Now if I run a Bootstrap for coefficients analysis, the results say the sig (2-tail) is 0.098. What are the critical values and alpha in this case? I’m trying to reconcile what I am seeing in both tables.
Thanks for your help.
April 20, 2020 at 3:24 am
Hi Marvalisa,
For one-tailed tests, you don’t need to divide alpha in half. If you can tell your software to perform a one-tailed test, it’ll do all the calculations necessary so you don’t need to adjust anything. So, if you’re using an alpha of 0.05 for a one-tailed test and your p-value is 0.04, it is significant. The procedures adjust the p-values automatically and it all works out. So, whether you’re using a one-tailed or two-tailed test, you always compare the p-value to the alpha with no need to adjust anything. The procedure does that for you!
The exception would be if for some reason your software doesn’t allow you to specify that you want to use a one-tailed test instead of a two-tailed test. Then, you divide the p-value from a two-tailed test in half to get the p-value for a one tailed test. You’d still compare it to your original alpha.
For regression, the same thing applies. If you want to use a one-tailed test for a cofficient, just divide the p-value in half if you can’t tell the software that you want a one-tailed test. The default is two-tailed. If your software has the option for one-tailed tests for any procedure, including regression, it’ll adjust the p-value for you. So, in the normal course of things, you won’t need to adjust anything.
March 26, 2020 at 12:00 pm
Hey Jim, for a one-tailed hypothesis test with a .05 confidence level, should I use a 95% confidence interval or a 90% confidence interval? Thanks
March 26, 2020 at 5:05 pm
You should use a one-sided 95% confidence interval. One-sided CIs have either an upper OR lower bound but remains unbounded on the other side.
March 16, 2020 at 4:30 pm
This is not applicable to the subject but… When performing tests of equivalence, we look at the confidence interval of the difference between two groups, and we perform two one-sided t-tests for equivalence..
March 15, 2020 at 7:51 am
Thanks for this illustrative blogpost. I had a question on one of your points though.
By definition of H1 and H0, a two-sided alternate hypothesis is that there is a difference in means between the test and control. Not that anything is ‘better’ or ‘worse’.
Just because we observed a negative result in your example, does not mean we can conclude it’s necessarily worse, but instead just ‘different’.
Therefore while it enables us to spot the fact that there may be differences between test and control, we cannot make claims about directional effects. So I struggle to see why they actually need to be used instead of one-sided tests.
What’s your take on this?
March 16, 2020 at 3:02 am
Hi Dominic,
If you’ll notice, I carefully avoid stating better or worse because in a general sense you’re right. However, given the context of a specific experiment, you can conclude whether a negative value is better or worse. As always in statistics, you have to use your subject-area knowledge to help interpret the results. In some cases, a negative value is a bad result. In other cases, it’s not. Use your subject-area knowledge!
I’m not sure why you think that you can’t make claims about directional effects? Of course you can!
As for why you shouldn’t use one-tailed tests for most cases, read my post When Can I Use One-Tailed Tests . That should answer your questions.
May 10, 2019 at 12:36 pm
Your website is absolutely amazing Jim, you seem like the nicest guy for doing this and I like how there’s no ulterior motive, (I wasn’t automatically signed up for emails or anything when leaving this comment). I study economics and found econometrics really difficult at first, but your website explains it so clearly its been a big asset to my studies, keep up the good work!
May 10, 2019 at 2:12 pm
Thank you so much, Jack. Your kind words mean a lot!
April 26, 2019 at 5:05 am
Hy Jim I really need your help now pls
One-tailed and two- tailed hypothesis, is it the same or twice, half or unrelated pls
April 26, 2019 at 11:41 am
Hi Anthony,
I describe how the hypotheses are different in this post. You’ll find your answers.
February 8, 2019 at 8:00 am
Thank you for your blog Jim, I have a Statistics exam soon and your articles let me understand a lot!
February 8, 2019 at 10:52 am
You’re very welcome! I’m happy to hear that it’s been helpful. Best of luck on your exam!
January 12, 2019 at 7:06 am
Hi Jim, When you say target value is 5. Do you mean to say the population mean is 5 and we are trying to validate it with the help of sample mean 4.1 using Hypo tests ?.. If it is so.. How can we measure a population parameter as 5 when it is almost impossible o measure a population parameter. Please clarify
January 12, 2019 at 6:57 pm
When you set a target for a one-sample test, it’s based on a value that is important to you. It’s not a population parameter or anything like that. The example in this post uses a case where we need parts that are stronger on average than a value of 5. We derive the value of 5 by using our subject area knowledge about what is required for a situation. Given our product knowledge for the hypothetical example, we know it should be 5 or higher. So, we use that in the hypothesis test and determine whether the population mean is greater than that target value.
When you perform a one-sample test, a target value is optional. If you don’t supply a target value, you simply obtain a confidence interval for the range of values that the parameter is likely to fall within. But, sometimes there is meaningful number that you want to test for specifically.
I hope that clarifies the rational behind the target value!
November 15, 2018 at 8:08 am
I understand that in Psychology a one tailed hypothesis is preferred. Is that so
November 15, 2018 at 11:30 am
No, there’s no overall preference for one-tailed hypothesis tests in statistics. That would be a study-by-study decision based on the types of possible effects. For more information about this decision, read my post: When Can I Use One-Tailed Tests?
November 6, 2018 at 1:14 am
I’m grateful to you for the explanations on One tail and Two tail hypothesis test. This opens my knowledge horizon beyond what an average statistics textbook can offer. Please include more examples in future posts. Thanks
November 5, 2018 at 10:20 am
Thank you. I will search it as well.
Stan Alekman
November 4, 2018 at 8:48 pm
Jim, what is the difference between the central and non-central t-distributions w/respect to hypothesis testing?
November 5, 2018 at 10:12 am
Hi Stan, this is something I will need to look into. I know central t-distribution is the common Student t-distribution, but I don’t have experience using non-central t-distributions. There might well be a blog post in that–after I learn more!
November 4, 2018 at 7:42 pm
this is awesome.
Comments and Questions Cancel reply
Aims And Hypotheses, Directional And Non-Directional
March 7, 2021 - paper 2 psychology in context | research methods.
In Psychology, hypotheses are predictions made by the researcher about the outcome of a study. The research can chose to make a specific prediction about what they feel will happen in their research (a directional hypothesis) or they can make a ‘general,’ ‘less specific’ prediction about the outcome of their research (a non-directional hypothesis). The type of prediction that a researcher makes is usually dependent on whether or not any previous research has also investigated their research aim.
Variables Recap:
The independent variable (IV) is the variable that psychologists manipulate/change to see if changing this variable has an effect on the depen dent variable (DV).
The dependent variable (DV) is the variable that the psychologists measures (to see if the IV has had an effect).
Research/Experimental Aim(S):

An aim is a clear and precise statement of the purpose of the study. It is a statement of why a research study is taking place. This should include what is being studied and what the study is trying to achieve. (e.g. “This study aims to investigate the effects of alcohol on reaction times”.
Hypotheses:
This is a testable statement that predicts what the researcher expects to happen in their research. The research study itself is therefore a means of testing whether or not the hypothesis is supported by the findings. If the findings do support the hypothesis then the hypothesis can be retained (i.e., accepted), but if not, then it must be rejected.

(1) Directional Hypothesis: states that the IV will have an effect on the DV and what that effect will be (the direction of results). For example, eating smarties will significantly improve an individual’s dancing ability. When writing a directional hypothesis, it is important that you state exactly how the IV will influence the DV.
(3) A Null Hypothesis: states that the IV will have no significant effect on the DV, for example, ‘eating smarties will have no effect in an individuals dancing ability.’

Non Directional Hypothesis
Ai generator.
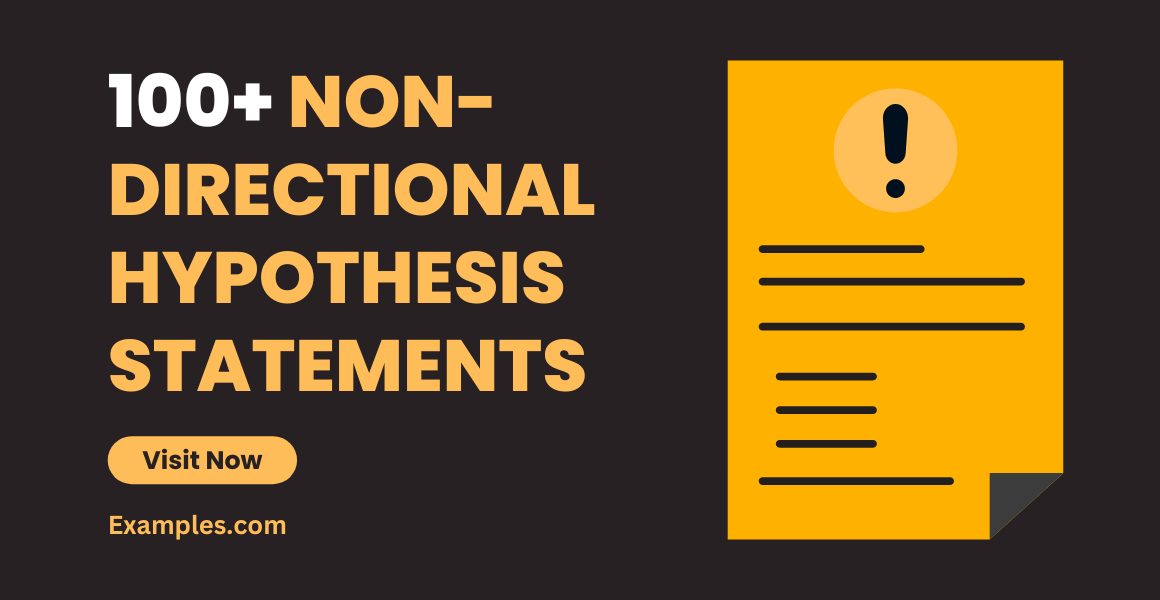
In the realm of hypothesis formulation, non-directional hypotheses offer a distinct perspective. These hypotheses suggest a relationship between variables without specifying the nature or direction of that relationship. This guide delves into non-directional hypothesis examples across various fields, outlines a step-by-step approach to crafting them, and provides expert tips to ensure your non-directional hypotheses are robust and insightful. Explore the world of Thesis statement hypotheses that explore connections without predetermined expectations.
What is the Non-Directional Hypothesis? – Definition
A non-directional hypothesis, also known as a two tailed hypothesis , is a type of hypothesis that predicts a relationship between variables without specifying the direction of that relationship. Unlike directional hypotheses that predict a specific outcome, non-directional hypotheses simply suggest that a relationship exists without indicating whether one variable will increase or decrease in response to changes in the other variable.
What is an Example of a Non-Directional Hypothesis Statement?
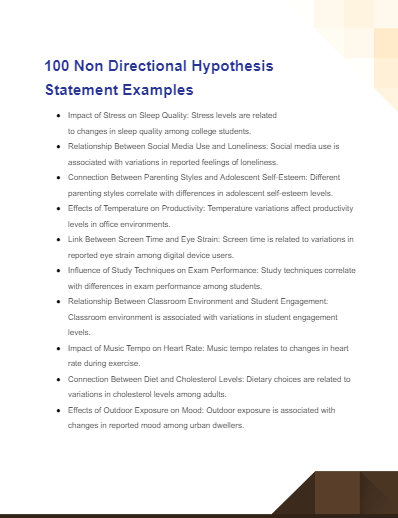
Size: 219 KB
“An increase in exercise frequency is associated with changes in weight.”
In this non-directional hypothesis, the statement suggests that a relationship exists between exercise frequency and weight changes but doesn’t specify whether increased exercise will lead to weight loss or weight gain. It leaves the direction of the relationship open for empirical investigation and data analysis.
100 Non Directional Hypothesis Statement Examples
Non-directional hypotheses explore relationships between variables without predicting the specific outcome. These simple hypothesis offer flexibility, allowing researchers to uncover unforeseen connections. Discover a range of non-directional hypothesis examples that span disciplines, enabling empirical exploration and evidence-based conclusions.
- Impact of Stress on Sleep Quality : Stress levels are related to changes in sleep quality among college students.
- Relationship Between Social Media Use and Loneliness : Social media use is associated with variations in reported feelings of loneliness.
- Connection Between Parenting Styles and Adolescent Self-Esteem : Different parenting styles correlate with differences in adolescent self-esteem levels.
- Effects of Temperature on Productivity : Temperature variations affect productivity levels in office environments.
- Link Between Screen Time and Eye Strain : Screen time is related to variations in reported eye strain among digital device users.
- Influence of Study Techniques on Exam Performance : Study techniques correlate with differences in exam performance among students.
- Relationship Between Classroom Environment and Student Engagement : Classroom environment is associated with variations in student engagement levels.
- Impact of Music Tempo on Heart Rate : Music tempo relates to changes in heart rate during exercise.
- Connection Between Diet and Cholesterol Levels : Dietary choices are related to variations in cholesterol levels among adults.
- Effects of Outdoor Exposure on Mood : Outdoor exposure is associated with changes in reported mood among urban dwellers.
- Relationship Between Personality Traits and Leadership Styles : Personality traits are associated with differences in preferred leadership styles among professionals.
- Impact of Time Management Strategies on Academic Performance : Time management strategies correlate with variations in academic performance among college students.
- Connection Between Cultural Exposure and Empathy Levels : Cultural exposure relates to changes in reported empathy levels among individuals.
- Effects of Nutrition Education on Dietary Choices : Nutrition education is associated with variations in dietary choices among adolescents.
- Link Between Social Support and Stress Levels : Social support is related to differences in reported stress levels among working adults.
- Influence of Exercise Intensity on Mood : Exercise intensity correlates with variations in reported mood among fitness enthusiasts.
- Relationship Between Parental Involvement and Academic Achievement : Parental involvement is associated with differences in academic achievement among schoolchildren.
- Impact of Sleep Duration on Cognitive Function : Sleep duration is related to changes in cognitive function among older adults.
- Connection Between Environmental Factors and Creativity : Environmental factors correlate with variations in reported creative thinking abilities among artists.
- Effects of Communication Styles on Conflict Resolution : Communication styles are associated with differences in conflict resolution outcomes among couples.
- Relationship Between Social Interaction and Life Satisfaction : Social interaction is related to variations in reported life satisfaction among elderly individuals.
- Impact of Classroom Seating Arrangements on Participation : Classroom seating arrangements correlate with differences in student participation levels.
- Connection Between Smartphone Use and Sleep Quality : Smartphone use is associated with changes in reported sleep quality among young adults.
- Effects of Mindfulness Practices on Stress Reduction : Mindfulness practices relate to variations in reported stress levels among participants.
- Link Between Gender and Communication Styles : Gender is related to differences in communication styles among individuals in group discussions.
- Influence of Advertising Exposure on Purchase Decisions : Advertising exposure correlates with variations in reported purchase decisions among consumers.
- Relationship Between Job Satisfaction and Employee Productivity : Job satisfaction is associated with differences in employee productivity levels.
- Impact of Social Support on Coping Mechanisms : Social support relates to variations in reported coping mechanisms among individuals facing challenges.
- Connection Between Classroom Environment and Student Creativity : Classroom environment is related to changes in student creativity levels.
- Effects of Exercise on Mood : Exercise is associated with variations in reported mood levels among participants.
- Relationship Between Music Preferences and Stress Levels : Music preferences are related to variations in reported stress levels among individuals.
- Impact of Nutrition Education on Food Choices : Nutrition education correlates with differences in dietary food choices among adolescents.
- Connection Between Physical Activity and Cognitive Function : Physical activity is associated with changes in cognitive function among older adults.
- Effects of Color Exposure on Mood : Color exposure relates to variations in reported mood levels among participants.
- Link Between Personality Traits and Career Choice : Personality traits are related to differences in career choices among individuals.
- Influence of Outdoor Recreation on Mental Well-being : Outdoor recreation is associated with variations in reported mental well-being among participants.
- Relationship Between Social Media Use and Self-Esteem : Social media use correlates with changes in reported self-esteem levels among young adults.
- Impact of Parenting Styles on Adolescent Risk Behavior : Parenting styles are related to variations in reported risk behaviors among adolescents.
- Connection Between Sleep Quality and Cognitive Performance : Sleep quality relates to changes in cognitive performance among students.
- Effects of Art Exposure on Creativity : Art exposure is associated with differences in reported creative thinking abilities among participants.
- Relationship Between Social Support and Mental Health : Social support is related to variations in reported mental health outcomes among individuals.
- Impact of Technology Use on Interpersonal Communication : Technology use correlates with differences in reported interpersonal communication skills among individuals.
- Connection Between Parental Attachment and Romantic Relationships : Parental attachment is associated with variations in the quality of romantic relationships among adults.
- Effects of Environmental Noise on Concentration : Environmental noise relates to changes in reported concentration levels among students.
- Link Between Music Exposure and Memory Performance : Music exposure is related to differences in memory performance among participants.
- Influence of Nutrition on Physical Fitness : Nutrition choices correlate with variations in reported physical fitness levels among athletes.
- Relationship Between Stress and Health Outcomes : Stress levels are associated with changes in reported health outcomes among individuals.
- Impact of Workplace Environment on Job Satisfaction : Workplace environment relates to differences in reported job satisfaction among employees.
- Connection Between Humor and Stress Reduction : Humor is related to variations in reported stress reduction among participants.
- Effects of Social Interaction on Emotional Well-being : Social interaction correlates with changes in reported emotional well-being among participants.
- Relationship Between Cultural Exposure and Cognitive Flexibility : Cultural exposure is related to variations in reported cognitive flexibility among individuals.
- Impact of Parent-Child Communication on Academic Achievement : Parent-child communication correlates with differences in academic achievement levels among students.
- Connection Between Personality Traits and Prosocial Behavior : Personality traits are associated with variations in reported prosocial behaviors among individuals.
- Effects of Nature Exposure on Stress Reduction : Nature exposure relates to changes in reported stress reduction among participants.
- Link Between Sleep Duration and Cognitive Performance : Sleep duration is related to differences in cognitive performance among participants.
- Influence of Social Media Use on Body Image : Social media use correlates with variations in reported body image satisfaction among young adults.
- Relationship Between Exercise and Mental Well-being : Exercise levels are associated with changes in reported mental well-being among participants.
- Impact of Cultural Competency Training on Patient Care : Cultural competency training relates to differences in patient care outcomes among healthcare professionals.
- Connection Between Perceived Social Support and Resilience : Perceived social support is related to variations in reported resilience levels among individuals.
- Effects of Environmental Factors on Mood : Environmental factors correlate with changes in reported mood levels among participants.
- Relationship Between Cultural Diversity and Team Performance : Cultural diversity is related to variations in reported team performance outcomes among professionals.
- Impact of Parental Involvement on Academic Motivation : Parental involvement correlates with differences in academic motivation levels among schoolchildren.
- Connection Between Mindfulness Practices and Anxiety Reduction : Mindfulness practices are associated with changes in reported anxiety levels among participants.
- Effects of Nutrition Education on Eating Habits : Nutrition education relates to variations in dietary eating habits among adolescents.
- Link Between Personality Traits and Learning Styles : Personality traits are related to differences in preferred learning styles among students.
- Influence of Nature Exposure on Creativity : Nature exposure correlates with variations in reported creative thinking abilities among individuals.
- Relationship Between Extracurricular Activities and Social Skills : Extracurricular activities are associated with changes in reported social skills among adolescents.
- Impact of Cultural Awareness Training on Stereotypes : Cultural awareness training relates to differences in perceived stereotypes among participants.
- Connection Between Sleep Quality and Emotional Regulation : Sleep quality is related to variations in reported emotional regulation skills among individuals.
- Effects of Music Exposure on Mood : Music exposure correlates with changes in reported mood levels among participants.
- Relationship Between Cultural Sensitivity and Cross-Cultural Communication : Cultural sensitivity is related to variations in reported cross-cultural communication skills among professionals.
- Impact of Parent-Child Bonding on Emotional Well-being : Parent-child bonding correlates with differences in reported emotional well-being levels among individuals.
- Connection Between Personality Traits and Conflict Resolution Styles : Personality traits are associated with variations in preferred conflict resolution styles among individuals.
- Effects of Mindfulness Practices on Focus and Concentration : Mindfulness practices relate to changes in reported focus and concentration levels among participants.
- Link Between Gender Identity and Career Aspirations : Gender identity is related to differences in reported career aspirations among individuals.
- Influence of Art Exposure on Emotional Expression : Art exposure correlates with variations in reported emotional expression abilities among participants.
- Relationship Between Peer Influence and Risky Behavior : Peer influence is associated with changes in reported engagement in risky behaviors among adolescents.
- Impact of Diversity Training on Workplace Harmony : Diversity training relates to differences in perceived workplace harmony among employees.
- Connection Between Sleep Patterns and Cognitive Performance : Sleep patterns are related to variations in cognitive performance among students.
- Effects of Exercise on Self-Esteem : Exercise correlates with changes in reported self-esteem levels among participants.
- Relationship Between Social Interaction and Well-being : Social interaction is related to variations in reported well-being levels among individuals.
- Impact of Parenting Styles on Adolescent Peer Relationships : Parenting styles correlate with differences in peer relationship quality among adolescents.
- Connection Between Personality Traits and Communication Effectiveness : Personality traits are associated with variations in communication effectiveness among professionals.
- Effects of Outdoor Activities on Stress Reduction : Outdoor activities relate to changes in reported stress reduction among participants.
- Link Between Music Exposure and Emotional Regulation : Music exposure is related to differences in reported emotional regulation skills among individuals.
- Influence of Family Dynamics on Academic Achievement : Family dynamics correlate with variations in academic achievement levels among students.
- Relationship Between Cultural Engagement and Empathy : Cultural engagement is associated with changes in reported empathy levels among individuals.
- Impact of Conflict Resolution Strategies on Relationship Satisfaction : Conflict resolution strategies relate to differences in reported relationship satisfaction levels among couples.
- Connection Between Sleep Quality and Physical Health : Sleep quality is related to variations in reported physical health outcomes among individuals.
- Effects of Social Support on Coping with Stress : Social support correlates with changes in reported coping strategies for stress among participants.
- Relationship Between Cultural Sensitivity and Patient Care : Cultural sensitivity is related to variations in reported patient care outcomes among healthcare professionals.
- Impact of Family Communication on Adolescent Well-being : Family communication correlates with differences in reported well-being levels among adolescents.
- Connection Between Personality Traits and Leadership Styles : Personality traits are associated with variations in preferred leadership styles among professionals.
- Effects of Nature Exposure on Attention Span : Nature exposure relates to changes in reported attention span among participants.
- Link Between Music Preference and Emotional Expression : Music preference is related to differences in reported emotional expression abilities among individuals.
- Influence of Peer Support on Academic Success : Peer support correlates with variations in reported academic success levels among students.
- Relationship Between Cultural Engagement and Creativity : Cultural engagement is associated with changes in reported creative thinking abilities among individuals.
- Impact of Conflict Resolution Skills on Relationship Satisfaction : Conflict resolution skills relate to differences in reported relationship satisfaction levels among couples.
- Connection Between Sleep Patterns and Stress Levels : Sleep patterns are related to variations in reported stress levels among individuals.
- Effects of Social Interaction on Happiness : Social interaction correlates with changes in reported happiness levels among participants.
Non-Directional Hypothesis Statement Examples for Psychology
These examples pertain to psychological studies and cover various relationships between psychological hypothesis concepts. For instance, the first example suggests that attachment styles might be related to romantic satisfaction, but it doesn’t specify whether attachment styles would increase or decrease satisfaction.
- Relationship Between Attachment Styles and Romantic Satisfaction : Attachment styles are related to variations in reported romantic satisfaction levels among individuals in psychology studies.
- Impact of Personality Traits on Career Success : Personality traits correlate with differences in reported career success outcomes among psychology study participants.
- Connection Between Parenting Styles and Adolescent Self-Esteem : Parenting styles are associated with variations in reported self-esteem levels among adolescents in psychological research.
- Effects of Social Media Use on Body Image : Social media use relates to changes in reported body image satisfaction among young adults in psychology experiments.
- Link Between Sleep Patterns and Emotional Well-being : Sleep patterns are related to differences in reported emotional well-being levels among psychology research participants.
- Influence of Mindfulness Practices on Stress Reduction : Mindfulness practices correlate with variations in reported stress reduction among psychology study participants.
- Relationship Between Social Interaction and Mental Health : Social interaction is associated with changes in reported mental health outcomes among individuals in psychology studies.
- Impact of Parent-Child Bonding on Emotional Resilience : Parent-child bonding relates to differences in reported emotional resilience levels among psychology research participants.
- Connection Between Cultural Sensitivity and Empathy : Cultural sensitivity is related to variations in reported empathy levels among individuals in psychology experiments.
- Effects of Exercise on Mood : Exercise correlates with changes in reported mood levels among psychology study participants.
Non-Directional Hypothesis Statement Examples in Research
These research hypothesis examples focus on research studies in general, covering a wide range of topics and relationships. For instance, the second example suggests that employee training might be related to workplace productivity, without indicating whether the training would lead to higher or lower productivity.
- Relationship Between Time Management and Academic Performance : Time management is related to variations in academic performance levels among research participants.
- Impact of Employee Training on Workplace Productivity : Employee training correlates with differences in reported workplace productivity outcomes among research subjects.
- Connection Between Media Exposure and Political Knowledge : Media exposure is associated with variations in reported political knowledge levels among research participants.
- Effects of Environmental Factors on Children’s Cognitive Development : Environmental factors relate to changes in reported cognitive development among research subjects.
- Link Between Parental Involvement and Student Motivation : Parental involvement is related to differences in reported student motivation levels among research participants.
- Influence of Cultural Immersion on Language Proficiency : Cultural immersion correlates with variations in reported language proficiency levels among research subjects.
- Relationship Between Leadership Styles and Team Performance : Leadership styles are associated with changes in reported team performance outcomes among research participants.
- Impact of Financial Literacy Education on Savings Habits : Financial literacy education relates to differences in reported savings habits among research subjects.
- Connection Between Stress Levels and Physical Health : Stress levels are related to variations in reported physical health outcomes among research participants.
- Effects of Music Exposure on Concentration : Music exposure correlates with changes in reported concentration levels among research subjects.
Non-Directional Hypothesis Statement Examples for Research Methodology
These examples are specific to the methods used in conducting research. The eighth example states that randomization might relate to group equivalence, but it doesn’t specify whether randomization would lead to more equivalent or less equivalent groups.
- Relationship Between Sampling Techniques and Research Validity : Sampling techniques are related to variations in research validity outcomes in studies of research methodology.
- Impact of Data Collection Methods on Data Accuracy : Data collection methods correlate with differences in reported data accuracy in research methodology experiments.
- Connection Between Research Design and Study Reproducibility : Research design is associated with variations in reported study reproducibility in research methodology studies.
- Effects of Questionnaire Format on Response Consistency : Questionnaire format relates to changes in reported response consistency in research methodology research.
- Link Between Ethical Considerations and Research Credibility : Ethical considerations are related to differences in reported research credibility in studies of research methodology.
- Influence of Measurement Scales on Data Precision : Measurement scales correlate with variations in reported data precision in research methodology experiments.
- Relationship Between Experimental Controls and Internal Validity : Experimental controls are associated with changes in internal validity outcomes in research methodology studies.
- Impact of Randomization on Group Equivalence : Randomization relates to differences in reported group equivalence in research methodology research.
- Connection Between Qualitative Data Analysis Methods and Data Richness : Qualitative data analysis methods are related to variations in reported data richness in studies of research methodology.
- Effects of Hypothesis Formulation on Research Focus : Hypothesis formulation correlates with changes in reported research focus in research methodology experiments.
These non-directional hypothesis statement examples offer insights into the diverse array of relationships explored in psychology, research, and research methodology studies, fostering empirical discovery and contributing to the advancement of knowledge across various fields.
Difference between Directional & Non-Directional Hypothesis
Directional and non-directional hypotheses are distinct approaches used in formulating hypotheses for research studies. Understanding the differences between them is essential for researchers to choose the appropriate type of causal hypothesis based on their study’s goals and prior knowledge.
- Direction: Directional hypotheses predict a specific relationship direction, while non-directional hypotheses do not specify a direction.
- Specificity: Directional hypotheses are more specific, while non-directional hypotheses are more general.
- Flexibility: Non-directional hypotheses allow for open-ended exploration, while directional hypotheses focus on confirming or refuting specific expectations.
How to Write a Non-Directional Hypothesis Statement – Step by Step Guide
- Identify Variables: Clearly define the variables you’re investigating—usually, an independent variable (the one manipulated) and a dependent variable (the one measured).
- Indicate Relationship: State that a relationship exists between the variables without predicting a specific direction.
- Use General Language: Craft the statement in a way that encompasses various possible outcomes.
- Avoid Biased Language: Do not include words that suggest a stronger effect or specific outcome for either variable.
- Connect to Research: If applicable, link the hypothesis to existing research or theories that justify exploring the relationship.
Tips for Writing a Non-Directional Hypothesis
- Start with Inquiry: Frame your hypothesis as an answer to a research question.
- Embrace Openness: Non-directional hypotheses are ideal when no strong expectation exists.
- Be Succinct: Keep the hypothesis statement concise and clear.
- Stay Neutral: Avoid implying that one variable will have a stronger impact.
- Allow Exploration: Leave room for various potential outcomes without preconceived notions.
- Tailor to Context: Ensure the hypothesis aligns with your research context and goals.
Non-directional hypotheses are particularly useful in exploratory research, where researchers aim to discover relationships without imposing specific expectations. They allow for unbiased investigation and the potential to uncover unexpected patterns or connections.
Remember that whether you choose a directional or non-directional hypothesis, both play critical roles in shaping the research process, guiding study design, data collection, and analysis. The choice depends on the research’s nature, goals, and existing knowledge in the field. You may also be interested in our science hypothesis .
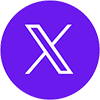
Text prompt
- Instructive
- Professional
10 Examples of Public speaking
20 Examples of Gas lighting

An official website of the United States government
The .gov means it’s official. Federal government websites often end in .gov or .mil. Before sharing sensitive information, make sure you’re on a federal government site.
The site is secure. The https:// ensures that you are connecting to the official website and that any information you provide is encrypted and transmitted securely.
- Publications
- Account settings
Preview improvements coming to the PMC website in October 2024. Learn More or Try it out now .
- Advanced Search
- Journal List
- J Korean Med Sci
- v.37(16); 2022 Apr 25

A Practical Guide to Writing Quantitative and Qualitative Research Questions and Hypotheses in Scholarly Articles
Edward barroga.
1 Department of General Education, Graduate School of Nursing Science, St. Luke’s International University, Tokyo, Japan.
Glafera Janet Matanguihan
2 Department of Biological Sciences, Messiah University, Mechanicsburg, PA, USA.
The development of research questions and the subsequent hypotheses are prerequisites to defining the main research purpose and specific objectives of a study. Consequently, these objectives determine the study design and research outcome. The development of research questions is a process based on knowledge of current trends, cutting-edge studies, and technological advances in the research field. Excellent research questions are focused and require a comprehensive literature search and in-depth understanding of the problem being investigated. Initially, research questions may be written as descriptive questions which could be developed into inferential questions. These questions must be specific and concise to provide a clear foundation for developing hypotheses. Hypotheses are more formal predictions about the research outcomes. These specify the possible results that may or may not be expected regarding the relationship between groups. Thus, research questions and hypotheses clarify the main purpose and specific objectives of the study, which in turn dictate the design of the study, its direction, and outcome. Studies developed from good research questions and hypotheses will have trustworthy outcomes with wide-ranging social and health implications.
INTRODUCTION
Scientific research is usually initiated by posing evidenced-based research questions which are then explicitly restated as hypotheses. 1 , 2 The hypotheses provide directions to guide the study, solutions, explanations, and expected results. 3 , 4 Both research questions and hypotheses are essentially formulated based on conventional theories and real-world processes, which allow the inception of novel studies and the ethical testing of ideas. 5 , 6
It is crucial to have knowledge of both quantitative and qualitative research 2 as both types of research involve writing research questions and hypotheses. 7 However, these crucial elements of research are sometimes overlooked; if not overlooked, then framed without the forethought and meticulous attention it needs. Planning and careful consideration are needed when developing quantitative or qualitative research, particularly when conceptualizing research questions and hypotheses. 4
There is a continuing need to support researchers in the creation of innovative research questions and hypotheses, as well as for journal articles that carefully review these elements. 1 When research questions and hypotheses are not carefully thought of, unethical studies and poor outcomes usually ensue. Carefully formulated research questions and hypotheses define well-founded objectives, which in turn determine the appropriate design, course, and outcome of the study. This article then aims to discuss in detail the various aspects of crafting research questions and hypotheses, with the goal of guiding researchers as they develop their own. Examples from the authors and peer-reviewed scientific articles in the healthcare field are provided to illustrate key points.
DEFINITIONS AND RELATIONSHIP OF RESEARCH QUESTIONS AND HYPOTHESES
A research question is what a study aims to answer after data analysis and interpretation. The answer is written in length in the discussion section of the paper. Thus, the research question gives a preview of the different parts and variables of the study meant to address the problem posed in the research question. 1 An excellent research question clarifies the research writing while facilitating understanding of the research topic, objective, scope, and limitations of the study. 5
On the other hand, a research hypothesis is an educated statement of an expected outcome. This statement is based on background research and current knowledge. 8 , 9 The research hypothesis makes a specific prediction about a new phenomenon 10 or a formal statement on the expected relationship between an independent variable and a dependent variable. 3 , 11 It provides a tentative answer to the research question to be tested or explored. 4
Hypotheses employ reasoning to predict a theory-based outcome. 10 These can also be developed from theories by focusing on components of theories that have not yet been observed. 10 The validity of hypotheses is often based on the testability of the prediction made in a reproducible experiment. 8
Conversely, hypotheses can also be rephrased as research questions. Several hypotheses based on existing theories and knowledge may be needed to answer a research question. Developing ethical research questions and hypotheses creates a research design that has logical relationships among variables. These relationships serve as a solid foundation for the conduct of the study. 4 , 11 Haphazardly constructed research questions can result in poorly formulated hypotheses and improper study designs, leading to unreliable results. Thus, the formulations of relevant research questions and verifiable hypotheses are crucial when beginning research. 12
CHARACTERISTICS OF GOOD RESEARCH QUESTIONS AND HYPOTHESES
Excellent research questions are specific and focused. These integrate collective data and observations to confirm or refute the subsequent hypotheses. Well-constructed hypotheses are based on previous reports and verify the research context. These are realistic, in-depth, sufficiently complex, and reproducible. More importantly, these hypotheses can be addressed and tested. 13
There are several characteristics of well-developed hypotheses. Good hypotheses are 1) empirically testable 7 , 10 , 11 , 13 ; 2) backed by preliminary evidence 9 ; 3) testable by ethical research 7 , 9 ; 4) based on original ideas 9 ; 5) have evidenced-based logical reasoning 10 ; and 6) can be predicted. 11 Good hypotheses can infer ethical and positive implications, indicating the presence of a relationship or effect relevant to the research theme. 7 , 11 These are initially developed from a general theory and branch into specific hypotheses by deductive reasoning. In the absence of a theory to base the hypotheses, inductive reasoning based on specific observations or findings form more general hypotheses. 10
TYPES OF RESEARCH QUESTIONS AND HYPOTHESES
Research questions and hypotheses are developed according to the type of research, which can be broadly classified into quantitative and qualitative research. We provide a summary of the types of research questions and hypotheses under quantitative and qualitative research categories in Table 1 .
Quantitative research questions | Quantitative research hypotheses |
---|---|
Descriptive research questions | Simple hypothesis |
Comparative research questions | Complex hypothesis |
Relationship research questions | Directional hypothesis |
Non-directional hypothesis | |
Associative hypothesis | |
Causal hypothesis | |
Null hypothesis | |
Alternative hypothesis | |
Working hypothesis | |
Statistical hypothesis | |
Logical hypothesis | |
Hypothesis-testing | |
Qualitative research questions | Qualitative research hypotheses |
Contextual research questions | Hypothesis-generating |
Descriptive research questions | |
Evaluation research questions | |
Explanatory research questions | |
Exploratory research questions | |
Generative research questions | |
Ideological research questions | |
Ethnographic research questions | |
Phenomenological research questions | |
Grounded theory questions | |
Qualitative case study questions |
Research questions in quantitative research
In quantitative research, research questions inquire about the relationships among variables being investigated and are usually framed at the start of the study. These are precise and typically linked to the subject population, dependent and independent variables, and research design. 1 Research questions may also attempt to describe the behavior of a population in relation to one or more variables, or describe the characteristics of variables to be measured ( descriptive research questions ). 1 , 5 , 14 These questions may also aim to discover differences between groups within the context of an outcome variable ( comparative research questions ), 1 , 5 , 14 or elucidate trends and interactions among variables ( relationship research questions ). 1 , 5 We provide examples of descriptive, comparative, and relationship research questions in quantitative research in Table 2 .
Quantitative research questions | |
---|---|
Descriptive research question | |
- Measures responses of subjects to variables | |
- Presents variables to measure, analyze, or assess | |
What is the proportion of resident doctors in the hospital who have mastered ultrasonography (response of subjects to a variable) as a diagnostic technique in their clinical training? | |
Comparative research question | |
- Clarifies difference between one group with outcome variable and another group without outcome variable | |
Is there a difference in the reduction of lung metastasis in osteosarcoma patients who received the vitamin D adjunctive therapy (group with outcome variable) compared with osteosarcoma patients who did not receive the vitamin D adjunctive therapy (group without outcome variable)? | |
- Compares the effects of variables | |
How does the vitamin D analogue 22-Oxacalcitriol (variable 1) mimic the antiproliferative activity of 1,25-Dihydroxyvitamin D (variable 2) in osteosarcoma cells? | |
Relationship research question | |
- Defines trends, association, relationships, or interactions between dependent variable and independent variable | |
Is there a relationship between the number of medical student suicide (dependent variable) and the level of medical student stress (independent variable) in Japan during the first wave of the COVID-19 pandemic? |
Hypotheses in quantitative research
In quantitative research, hypotheses predict the expected relationships among variables. 15 Relationships among variables that can be predicted include 1) between a single dependent variable and a single independent variable ( simple hypothesis ) or 2) between two or more independent and dependent variables ( complex hypothesis ). 4 , 11 Hypotheses may also specify the expected direction to be followed and imply an intellectual commitment to a particular outcome ( directional hypothesis ) 4 . On the other hand, hypotheses may not predict the exact direction and are used in the absence of a theory, or when findings contradict previous studies ( non-directional hypothesis ). 4 In addition, hypotheses can 1) define interdependency between variables ( associative hypothesis ), 4 2) propose an effect on the dependent variable from manipulation of the independent variable ( causal hypothesis ), 4 3) state a negative relationship between two variables ( null hypothesis ), 4 , 11 , 15 4) replace the working hypothesis if rejected ( alternative hypothesis ), 15 explain the relationship of phenomena to possibly generate a theory ( working hypothesis ), 11 5) involve quantifiable variables that can be tested statistically ( statistical hypothesis ), 11 6) or express a relationship whose interlinks can be verified logically ( logical hypothesis ). 11 We provide examples of simple, complex, directional, non-directional, associative, causal, null, alternative, working, statistical, and logical hypotheses in quantitative research, as well as the definition of quantitative hypothesis-testing research in Table 3 .
Quantitative research hypotheses | |
---|---|
Simple hypothesis | |
- Predicts relationship between single dependent variable and single independent variable | |
If the dose of the new medication (single independent variable) is high, blood pressure (single dependent variable) is lowered. | |
Complex hypothesis | |
- Foretells relationship between two or more independent and dependent variables | |
The higher the use of anticancer drugs, radiation therapy, and adjunctive agents (3 independent variables), the higher would be the survival rate (1 dependent variable). | |
Directional hypothesis | |
- Identifies study direction based on theory towards particular outcome to clarify relationship between variables | |
Privately funded research projects will have a larger international scope (study direction) than publicly funded research projects. | |
Non-directional hypothesis | |
- Nature of relationship between two variables or exact study direction is not identified | |
- Does not involve a theory | |
Women and men are different in terms of helpfulness. (Exact study direction is not identified) | |
Associative hypothesis | |
- Describes variable interdependency | |
- Change in one variable causes change in another variable | |
A larger number of people vaccinated against COVID-19 in the region (change in independent variable) will reduce the region’s incidence of COVID-19 infection (change in dependent variable). | |
Causal hypothesis | |
- An effect on dependent variable is predicted from manipulation of independent variable | |
A change into a high-fiber diet (independent variable) will reduce the blood sugar level (dependent variable) of the patient. | |
Null hypothesis | |
- A negative statement indicating no relationship or difference between 2 variables | |
There is no significant difference in the severity of pulmonary metastases between the new drug (variable 1) and the current drug (variable 2). | |
Alternative hypothesis | |
- Following a null hypothesis, an alternative hypothesis predicts a relationship between 2 study variables | |
The new drug (variable 1) is better on average in reducing the level of pain from pulmonary metastasis than the current drug (variable 2). | |
Working hypothesis | |
- A hypothesis that is initially accepted for further research to produce a feasible theory | |
Dairy cows fed with concentrates of different formulations will produce different amounts of milk. | |
Statistical hypothesis | |
- Assumption about the value of population parameter or relationship among several population characteristics | |
- Validity tested by a statistical experiment or analysis | |
The mean recovery rate from COVID-19 infection (value of population parameter) is not significantly different between population 1 and population 2. | |
There is a positive correlation between the level of stress at the workplace and the number of suicides (population characteristics) among working people in Japan. | |
Logical hypothesis | |
- Offers or proposes an explanation with limited or no extensive evidence | |
If healthcare workers provide more educational programs about contraception methods, the number of adolescent pregnancies will be less. | |
Hypothesis-testing (Quantitative hypothesis-testing research) | |
- Quantitative research uses deductive reasoning. | |
- This involves the formation of a hypothesis, collection of data in the investigation of the problem, analysis and use of the data from the investigation, and drawing of conclusions to validate or nullify the hypotheses. |
Research questions in qualitative research
Unlike research questions in quantitative research, research questions in qualitative research are usually continuously reviewed and reformulated. The central question and associated subquestions are stated more than the hypotheses. 15 The central question broadly explores a complex set of factors surrounding the central phenomenon, aiming to present the varied perspectives of participants. 15
There are varied goals for which qualitative research questions are developed. These questions can function in several ways, such as to 1) identify and describe existing conditions ( contextual research question s); 2) describe a phenomenon ( descriptive research questions ); 3) assess the effectiveness of existing methods, protocols, theories, or procedures ( evaluation research questions ); 4) examine a phenomenon or analyze the reasons or relationships between subjects or phenomena ( explanatory research questions ); or 5) focus on unknown aspects of a particular topic ( exploratory research questions ). 5 In addition, some qualitative research questions provide new ideas for the development of theories and actions ( generative research questions ) or advance specific ideologies of a position ( ideological research questions ). 1 Other qualitative research questions may build on a body of existing literature and become working guidelines ( ethnographic research questions ). Research questions may also be broadly stated without specific reference to the existing literature or a typology of questions ( phenomenological research questions ), may be directed towards generating a theory of some process ( grounded theory questions ), or may address a description of the case and the emerging themes ( qualitative case study questions ). 15 We provide examples of contextual, descriptive, evaluation, explanatory, exploratory, generative, ideological, ethnographic, phenomenological, grounded theory, and qualitative case study research questions in qualitative research in Table 4 , and the definition of qualitative hypothesis-generating research in Table 5 .
Qualitative research questions | |
---|---|
Contextual research question | |
- Ask the nature of what already exists | |
- Individuals or groups function to further clarify and understand the natural context of real-world problems | |
What are the experiences of nurses working night shifts in healthcare during the COVID-19 pandemic? (natural context of real-world problems) | |
Descriptive research question | |
- Aims to describe a phenomenon | |
What are the different forms of disrespect and abuse (phenomenon) experienced by Tanzanian women when giving birth in healthcare facilities? | |
Evaluation research question | |
- Examines the effectiveness of existing practice or accepted frameworks | |
How effective are decision aids (effectiveness of existing practice) in helping decide whether to give birth at home or in a healthcare facility? | |
Explanatory research question | |
- Clarifies a previously studied phenomenon and explains why it occurs | |
Why is there an increase in teenage pregnancy (phenomenon) in Tanzania? | |
Exploratory research question | |
- Explores areas that have not been fully investigated to have a deeper understanding of the research problem | |
What factors affect the mental health of medical students (areas that have not yet been fully investigated) during the COVID-19 pandemic? | |
Generative research question | |
- Develops an in-depth understanding of people’s behavior by asking ‘how would’ or ‘what if’ to identify problems and find solutions | |
How would the extensive research experience of the behavior of new staff impact the success of the novel drug initiative? | |
Ideological research question | |
- Aims to advance specific ideas or ideologies of a position | |
Are Japanese nurses who volunteer in remote African hospitals able to promote humanized care of patients (specific ideas or ideologies) in the areas of safe patient environment, respect of patient privacy, and provision of accurate information related to health and care? | |
Ethnographic research question | |
- Clarifies peoples’ nature, activities, their interactions, and the outcomes of their actions in specific settings | |
What are the demographic characteristics, rehabilitative treatments, community interactions, and disease outcomes (nature, activities, their interactions, and the outcomes) of people in China who are suffering from pneumoconiosis? | |
Phenomenological research question | |
- Knows more about the phenomena that have impacted an individual | |
What are the lived experiences of parents who have been living with and caring for children with a diagnosis of autism? (phenomena that have impacted an individual) | |
Grounded theory question | |
- Focuses on social processes asking about what happens and how people interact, or uncovering social relationships and behaviors of groups | |
What are the problems that pregnant adolescents face in terms of social and cultural norms (social processes), and how can these be addressed? | |
Qualitative case study question | |
- Assesses a phenomenon using different sources of data to answer “why” and “how” questions | |
- Considers how the phenomenon is influenced by its contextual situation. | |
How does quitting work and assuming the role of a full-time mother (phenomenon assessed) change the lives of women in Japan? |
Qualitative research hypotheses | |
---|---|
Hypothesis-generating (Qualitative hypothesis-generating research) | |
- Qualitative research uses inductive reasoning. | |
- This involves data collection from study participants or the literature regarding a phenomenon of interest, using the collected data to develop a formal hypothesis, and using the formal hypothesis as a framework for testing the hypothesis. | |
- Qualitative exploratory studies explore areas deeper, clarifying subjective experience and allowing formulation of a formal hypothesis potentially testable in a future quantitative approach. |
Qualitative studies usually pose at least one central research question and several subquestions starting with How or What . These research questions use exploratory verbs such as explore or describe . These also focus on one central phenomenon of interest, and may mention the participants and research site. 15
Hypotheses in qualitative research
Hypotheses in qualitative research are stated in the form of a clear statement concerning the problem to be investigated. Unlike in quantitative research where hypotheses are usually developed to be tested, qualitative research can lead to both hypothesis-testing and hypothesis-generating outcomes. 2 When studies require both quantitative and qualitative research questions, this suggests an integrative process between both research methods wherein a single mixed-methods research question can be developed. 1
FRAMEWORKS FOR DEVELOPING RESEARCH QUESTIONS AND HYPOTHESES
Research questions followed by hypotheses should be developed before the start of the study. 1 , 12 , 14 It is crucial to develop feasible research questions on a topic that is interesting to both the researcher and the scientific community. This can be achieved by a meticulous review of previous and current studies to establish a novel topic. Specific areas are subsequently focused on to generate ethical research questions. The relevance of the research questions is evaluated in terms of clarity of the resulting data, specificity of the methodology, objectivity of the outcome, depth of the research, and impact of the study. 1 , 5 These aspects constitute the FINER criteria (i.e., Feasible, Interesting, Novel, Ethical, and Relevant). 1 Clarity and effectiveness are achieved if research questions meet the FINER criteria. In addition to the FINER criteria, Ratan et al. described focus, complexity, novelty, feasibility, and measurability for evaluating the effectiveness of research questions. 14
The PICOT and PEO frameworks are also used when developing research questions. 1 The following elements are addressed in these frameworks, PICOT: P-population/patients/problem, I-intervention or indicator being studied, C-comparison group, O-outcome of interest, and T-timeframe of the study; PEO: P-population being studied, E-exposure to preexisting conditions, and O-outcome of interest. 1 Research questions are also considered good if these meet the “FINERMAPS” framework: Feasible, Interesting, Novel, Ethical, Relevant, Manageable, Appropriate, Potential value/publishable, and Systematic. 14
As we indicated earlier, research questions and hypotheses that are not carefully formulated result in unethical studies or poor outcomes. To illustrate this, we provide some examples of ambiguous research question and hypotheses that result in unclear and weak research objectives in quantitative research ( Table 6 ) 16 and qualitative research ( Table 7 ) 17 , and how to transform these ambiguous research question(s) and hypothesis(es) into clear and good statements.
Variables | Unclear and weak statement (Statement 1) | Clear and good statement (Statement 2) | Points to avoid |
---|---|---|---|
Research question | Which is more effective between smoke moxibustion and smokeless moxibustion? | “Moreover, regarding smoke moxibustion versus smokeless moxibustion, it remains unclear which is more effective, safe, and acceptable to pregnant women, and whether there is any difference in the amount of heat generated.” | 1) Vague and unfocused questions |
2) Closed questions simply answerable by yes or no | |||
3) Questions requiring a simple choice | |||
Hypothesis | The smoke moxibustion group will have higher cephalic presentation. | “Hypothesis 1. The smoke moxibustion stick group (SM group) and smokeless moxibustion stick group (-SLM group) will have higher rates of cephalic presentation after treatment than the control group. | 1) Unverifiable hypotheses |
Hypothesis 2. The SM group and SLM group will have higher rates of cephalic presentation at birth than the control group. | 2) Incompletely stated groups of comparison | ||
Hypothesis 3. There will be no significant differences in the well-being of the mother and child among the three groups in terms of the following outcomes: premature birth, premature rupture of membranes (PROM) at < 37 weeks, Apgar score < 7 at 5 min, umbilical cord blood pH < 7.1, admission to neonatal intensive care unit (NICU), and intrauterine fetal death.” | 3) Insufficiently described variables or outcomes | ||
Research objective | To determine which is more effective between smoke moxibustion and smokeless moxibustion. | “The specific aims of this pilot study were (a) to compare the effects of smoke moxibustion and smokeless moxibustion treatments with the control group as a possible supplement to ECV for converting breech presentation to cephalic presentation and increasing adherence to the newly obtained cephalic position, and (b) to assess the effects of these treatments on the well-being of the mother and child.” | 1) Poor understanding of the research question and hypotheses |
2) Insufficient description of population, variables, or study outcomes |
a These statements were composed for comparison and illustrative purposes only.
b These statements are direct quotes from Higashihara and Horiuchi. 16
Variables | Unclear and weak statement (Statement 1) | Clear and good statement (Statement 2) | Points to avoid |
---|---|---|---|
Research question | Does disrespect and abuse (D&A) occur in childbirth in Tanzania? | How does disrespect and abuse (D&A) occur and what are the types of physical and psychological abuses observed in midwives’ actual care during facility-based childbirth in urban Tanzania? | 1) Ambiguous or oversimplistic questions |
2) Questions unverifiable by data collection and analysis | |||
Hypothesis | Disrespect and abuse (D&A) occur in childbirth in Tanzania. | Hypothesis 1: Several types of physical and psychological abuse by midwives in actual care occur during facility-based childbirth in urban Tanzania. | 1) Statements simply expressing facts |
Hypothesis 2: Weak nursing and midwifery management contribute to the D&A of women during facility-based childbirth in urban Tanzania. | 2) Insufficiently described concepts or variables | ||
Research objective | To describe disrespect and abuse (D&A) in childbirth in Tanzania. | “This study aimed to describe from actual observations the respectful and disrespectful care received by women from midwives during their labor period in two hospitals in urban Tanzania.” | 1) Statements unrelated to the research question and hypotheses |
2) Unattainable or unexplorable objectives |
a This statement is a direct quote from Shimoda et al. 17
The other statements were composed for comparison and illustrative purposes only.
CONSTRUCTING RESEARCH QUESTIONS AND HYPOTHESES
To construct effective research questions and hypotheses, it is very important to 1) clarify the background and 2) identify the research problem at the outset of the research, within a specific timeframe. 9 Then, 3) review or conduct preliminary research to collect all available knowledge about the possible research questions by studying theories and previous studies. 18 Afterwards, 4) construct research questions to investigate the research problem. Identify variables to be accessed from the research questions 4 and make operational definitions of constructs from the research problem and questions. Thereafter, 5) construct specific deductive or inductive predictions in the form of hypotheses. 4 Finally, 6) state the study aims . This general flow for constructing effective research questions and hypotheses prior to conducting research is shown in Fig. 1 .

Research questions are used more frequently in qualitative research than objectives or hypotheses. 3 These questions seek to discover, understand, explore or describe experiences by asking “What” or “How.” The questions are open-ended to elicit a description rather than to relate variables or compare groups. The questions are continually reviewed, reformulated, and changed during the qualitative study. 3 Research questions are also used more frequently in survey projects than hypotheses in experiments in quantitative research to compare variables and their relationships.
Hypotheses are constructed based on the variables identified and as an if-then statement, following the template, ‘If a specific action is taken, then a certain outcome is expected.’ At this stage, some ideas regarding expectations from the research to be conducted must be drawn. 18 Then, the variables to be manipulated (independent) and influenced (dependent) are defined. 4 Thereafter, the hypothesis is stated and refined, and reproducible data tailored to the hypothesis are identified, collected, and analyzed. 4 The hypotheses must be testable and specific, 18 and should describe the variables and their relationships, the specific group being studied, and the predicted research outcome. 18 Hypotheses construction involves a testable proposition to be deduced from theory, and independent and dependent variables to be separated and measured separately. 3 Therefore, good hypotheses must be based on good research questions constructed at the start of a study or trial. 12
In summary, research questions are constructed after establishing the background of the study. Hypotheses are then developed based on the research questions. Thus, it is crucial to have excellent research questions to generate superior hypotheses. In turn, these would determine the research objectives and the design of the study, and ultimately, the outcome of the research. 12 Algorithms for building research questions and hypotheses are shown in Fig. 2 for quantitative research and in Fig. 3 for qualitative research.

EXAMPLES OF RESEARCH QUESTIONS FROM PUBLISHED ARTICLES
- EXAMPLE 1. Descriptive research question (quantitative research)
- - Presents research variables to be assessed (distinct phenotypes and subphenotypes)
- “BACKGROUND: Since COVID-19 was identified, its clinical and biological heterogeneity has been recognized. Identifying COVID-19 phenotypes might help guide basic, clinical, and translational research efforts.
- RESEARCH QUESTION: Does the clinical spectrum of patients with COVID-19 contain distinct phenotypes and subphenotypes? ” 19
- EXAMPLE 2. Relationship research question (quantitative research)
- - Shows interactions between dependent variable (static postural control) and independent variable (peripheral visual field loss)
- “Background: Integration of visual, vestibular, and proprioceptive sensations contributes to postural control. People with peripheral visual field loss have serious postural instability. However, the directional specificity of postural stability and sensory reweighting caused by gradual peripheral visual field loss remain unclear.
- Research question: What are the effects of peripheral visual field loss on static postural control ?” 20
- EXAMPLE 3. Comparative research question (quantitative research)
- - Clarifies the difference among groups with an outcome variable (patients enrolled in COMPERA with moderate PH or severe PH in COPD) and another group without the outcome variable (patients with idiopathic pulmonary arterial hypertension (IPAH))
- “BACKGROUND: Pulmonary hypertension (PH) in COPD is a poorly investigated clinical condition.
- RESEARCH QUESTION: Which factors determine the outcome of PH in COPD?
- STUDY DESIGN AND METHODS: We analyzed the characteristics and outcome of patients enrolled in the Comparative, Prospective Registry of Newly Initiated Therapies for Pulmonary Hypertension (COMPERA) with moderate or severe PH in COPD as defined during the 6th PH World Symposium who received medical therapy for PH and compared them with patients with idiopathic pulmonary arterial hypertension (IPAH) .” 21
- EXAMPLE 4. Exploratory research question (qualitative research)
- - Explores areas that have not been fully investigated (perspectives of families and children who receive care in clinic-based child obesity treatment) to have a deeper understanding of the research problem
- “Problem: Interventions for children with obesity lead to only modest improvements in BMI and long-term outcomes, and data are limited on the perspectives of families of children with obesity in clinic-based treatment. This scoping review seeks to answer the question: What is known about the perspectives of families and children who receive care in clinic-based child obesity treatment? This review aims to explore the scope of perspectives reported by families of children with obesity who have received individualized outpatient clinic-based obesity treatment.” 22
- EXAMPLE 5. Relationship research question (quantitative research)
- - Defines interactions between dependent variable (use of ankle strategies) and independent variable (changes in muscle tone)
- “Background: To maintain an upright standing posture against external disturbances, the human body mainly employs two types of postural control strategies: “ankle strategy” and “hip strategy.” While it has been reported that the magnitude of the disturbance alters the use of postural control strategies, it has not been elucidated how the level of muscle tone, one of the crucial parameters of bodily function, determines the use of each strategy. We have previously confirmed using forward dynamics simulations of human musculoskeletal models that an increased muscle tone promotes the use of ankle strategies. The objective of the present study was to experimentally evaluate a hypothesis: an increased muscle tone promotes the use of ankle strategies. Research question: Do changes in the muscle tone affect the use of ankle strategies ?” 23
EXAMPLES OF HYPOTHESES IN PUBLISHED ARTICLES
- EXAMPLE 1. Working hypothesis (quantitative research)
- - A hypothesis that is initially accepted for further research to produce a feasible theory
- “As fever may have benefit in shortening the duration of viral illness, it is plausible to hypothesize that the antipyretic efficacy of ibuprofen may be hindering the benefits of a fever response when taken during the early stages of COVID-19 illness .” 24
- “In conclusion, it is plausible to hypothesize that the antipyretic efficacy of ibuprofen may be hindering the benefits of a fever response . The difference in perceived safety of these agents in COVID-19 illness could be related to the more potent efficacy to reduce fever with ibuprofen compared to acetaminophen. Compelling data on the benefit of fever warrant further research and review to determine when to treat or withhold ibuprofen for early stage fever for COVID-19 and other related viral illnesses .” 24
- EXAMPLE 2. Exploratory hypothesis (qualitative research)
- - Explores particular areas deeper to clarify subjective experience and develop a formal hypothesis potentially testable in a future quantitative approach
- “We hypothesized that when thinking about a past experience of help-seeking, a self distancing prompt would cause increased help-seeking intentions and more favorable help-seeking outcome expectations .” 25
- “Conclusion
- Although a priori hypotheses were not supported, further research is warranted as results indicate the potential for using self-distancing approaches to increasing help-seeking among some people with depressive symptomatology.” 25
- EXAMPLE 3. Hypothesis-generating research to establish a framework for hypothesis testing (qualitative research)
- “We hypothesize that compassionate care is beneficial for patients (better outcomes), healthcare systems and payers (lower costs), and healthcare providers (lower burnout). ” 26
- Compassionomics is the branch of knowledge and scientific study of the effects of compassionate healthcare. Our main hypotheses are that compassionate healthcare is beneficial for (1) patients, by improving clinical outcomes, (2) healthcare systems and payers, by supporting financial sustainability, and (3) HCPs, by lowering burnout and promoting resilience and well-being. The purpose of this paper is to establish a scientific framework for testing the hypotheses above . If these hypotheses are confirmed through rigorous research, compassionomics will belong in the science of evidence-based medicine, with major implications for all healthcare domains.” 26
- EXAMPLE 4. Statistical hypothesis (quantitative research)
- - An assumption is made about the relationship among several population characteristics ( gender differences in sociodemographic and clinical characteristics of adults with ADHD ). Validity is tested by statistical experiment or analysis ( chi-square test, Students t-test, and logistic regression analysis)
- “Our research investigated gender differences in sociodemographic and clinical characteristics of adults with ADHD in a Japanese clinical sample. Due to unique Japanese cultural ideals and expectations of women's behavior that are in opposition to ADHD symptoms, we hypothesized that women with ADHD experience more difficulties and present more dysfunctions than men . We tested the following hypotheses: first, women with ADHD have more comorbidities than men with ADHD; second, women with ADHD experience more social hardships than men, such as having less full-time employment and being more likely to be divorced.” 27
- “Statistical Analysis
- ( text omitted ) Between-gender comparisons were made using the chi-squared test for categorical variables and Students t-test for continuous variables…( text omitted ). A logistic regression analysis was performed for employment status, marital status, and comorbidity to evaluate the independent effects of gender on these dependent variables.” 27
EXAMPLES OF HYPOTHESIS AS WRITTEN IN PUBLISHED ARTICLES IN RELATION TO OTHER PARTS
- EXAMPLE 1. Background, hypotheses, and aims are provided
- “Pregnant women need skilled care during pregnancy and childbirth, but that skilled care is often delayed in some countries …( text omitted ). The focused antenatal care (FANC) model of WHO recommends that nurses provide information or counseling to all pregnant women …( text omitted ). Job aids are visual support materials that provide the right kind of information using graphics and words in a simple and yet effective manner. When nurses are not highly trained or have many work details to attend to, these job aids can serve as a content reminder for the nurses and can be used for educating their patients (Jennings, Yebadokpo, Affo, & Agbogbe, 2010) ( text omitted ). Importantly, additional evidence is needed to confirm how job aids can further improve the quality of ANC counseling by health workers in maternal care …( text omitted )” 28
- “ This has led us to hypothesize that the quality of ANC counseling would be better if supported by job aids. Consequently, a better quality of ANC counseling is expected to produce higher levels of awareness concerning the danger signs of pregnancy and a more favorable impression of the caring behavior of nurses .” 28
- “This study aimed to examine the differences in the responses of pregnant women to a job aid-supported intervention during ANC visit in terms of 1) their understanding of the danger signs of pregnancy and 2) their impression of the caring behaviors of nurses to pregnant women in rural Tanzania.” 28
- EXAMPLE 2. Background, hypotheses, and aims are provided
- “We conducted a two-arm randomized controlled trial (RCT) to evaluate and compare changes in salivary cortisol and oxytocin levels of first-time pregnant women between experimental and control groups. The women in the experimental group touched and held an infant for 30 min (experimental intervention protocol), whereas those in the control group watched a DVD movie of an infant (control intervention protocol). The primary outcome was salivary cortisol level and the secondary outcome was salivary oxytocin level.” 29
- “ We hypothesize that at 30 min after touching and holding an infant, the salivary cortisol level will significantly decrease and the salivary oxytocin level will increase in the experimental group compared with the control group .” 29
- EXAMPLE 3. Background, aim, and hypothesis are provided
- “In countries where the maternal mortality ratio remains high, antenatal education to increase Birth Preparedness and Complication Readiness (BPCR) is considered one of the top priorities [1]. BPCR includes birth plans during the antenatal period, such as the birthplace, birth attendant, transportation, health facility for complications, expenses, and birth materials, as well as family coordination to achieve such birth plans. In Tanzania, although increasing, only about half of all pregnant women attend an antenatal clinic more than four times [4]. Moreover, the information provided during antenatal care (ANC) is insufficient. In the resource-poor settings, antenatal group education is a potential approach because of the limited time for individual counseling at antenatal clinics.” 30
- “This study aimed to evaluate an antenatal group education program among pregnant women and their families with respect to birth-preparedness and maternal and infant outcomes in rural villages of Tanzania.” 30
- “ The study hypothesis was if Tanzanian pregnant women and their families received a family-oriented antenatal group education, they would (1) have a higher level of BPCR, (2) attend antenatal clinic four or more times, (3) give birth in a health facility, (4) have less complications of women at birth, and (5) have less complications and deaths of infants than those who did not receive the education .” 30
Research questions and hypotheses are crucial components to any type of research, whether quantitative or qualitative. These questions should be developed at the very beginning of the study. Excellent research questions lead to superior hypotheses, which, like a compass, set the direction of research, and can often determine the successful conduct of the study. Many research studies have floundered because the development of research questions and subsequent hypotheses was not given the thought and meticulous attention needed. The development of research questions and hypotheses is an iterative process based on extensive knowledge of the literature and insightful grasp of the knowledge gap. Focused, concise, and specific research questions provide a strong foundation for constructing hypotheses which serve as formal predictions about the research outcomes. Research questions and hypotheses are crucial elements of research that should not be overlooked. They should be carefully thought of and constructed when planning research. This avoids unethical studies and poor outcomes by defining well-founded objectives that determine the design, course, and outcome of the study.
Disclosure: The authors have no potential conflicts of interest to disclose.
Author Contributions:
- Conceptualization: Barroga E, Matanguihan GJ.
- Methodology: Barroga E, Matanguihan GJ.
- Writing - original draft: Barroga E, Matanguihan GJ.
- Writing - review & editing: Barroga E, Matanguihan GJ.
Reference Library
Collections
- See what's new
- All Resources
- Student Resources
- Assessment Resources
- Teaching Resources
- CPD Courses
- Livestreams
Study notes, videos, interactive activities and more!
Psychology news, insights and enrichment
Currated collections of free resources
Browse resources by topic
- All Psychology Resources
Resource Selections
Currated lists of resources
Non-Directional Hypothesis
A non-directional hypothesis is a two-tailed hypothesis that does not predict the direction of the difference or relationship (e.g. girls and boys are different in terms of helpfulness).
- Share on Facebook
- Share on Twitter
- Share by Email
Research Methods: MCQ Revision Test 1 for AQA A Level Psychology
Topic Videos
Example Answers for Research Methods: A Level Psychology, Paper 2, June 2018 (AQA)
Exam Support
Example Answer for Question 14 Paper 2: AS Psychology, June 2017 (AQA)
Model answer for question 11 paper 2: as psychology, june 2016 (aqa), a level psychology topic quiz - research methods.
Quizzes & Activities
Our subjects
- › Criminology
- › Economics
- › Geography
- › Health & Social Care
- › Psychology
- › Sociology
- › Teaching & learning resources
- › Student revision workshops
- › Online student courses
- › CPD for teachers
- › Livestreams
- › Teaching jobs
Boston House, 214 High Street, Boston Spa, West Yorkshire, LS23 6AD Tel: 01937 848885
- › Contact us
- › Terms of use
- › Privacy & cookies
© 2002-2024 Tutor2u Limited. Company Reg no: 04489574. VAT reg no 816865400.
Hypothesis ( AQA A Level Psychology )
Revision note.
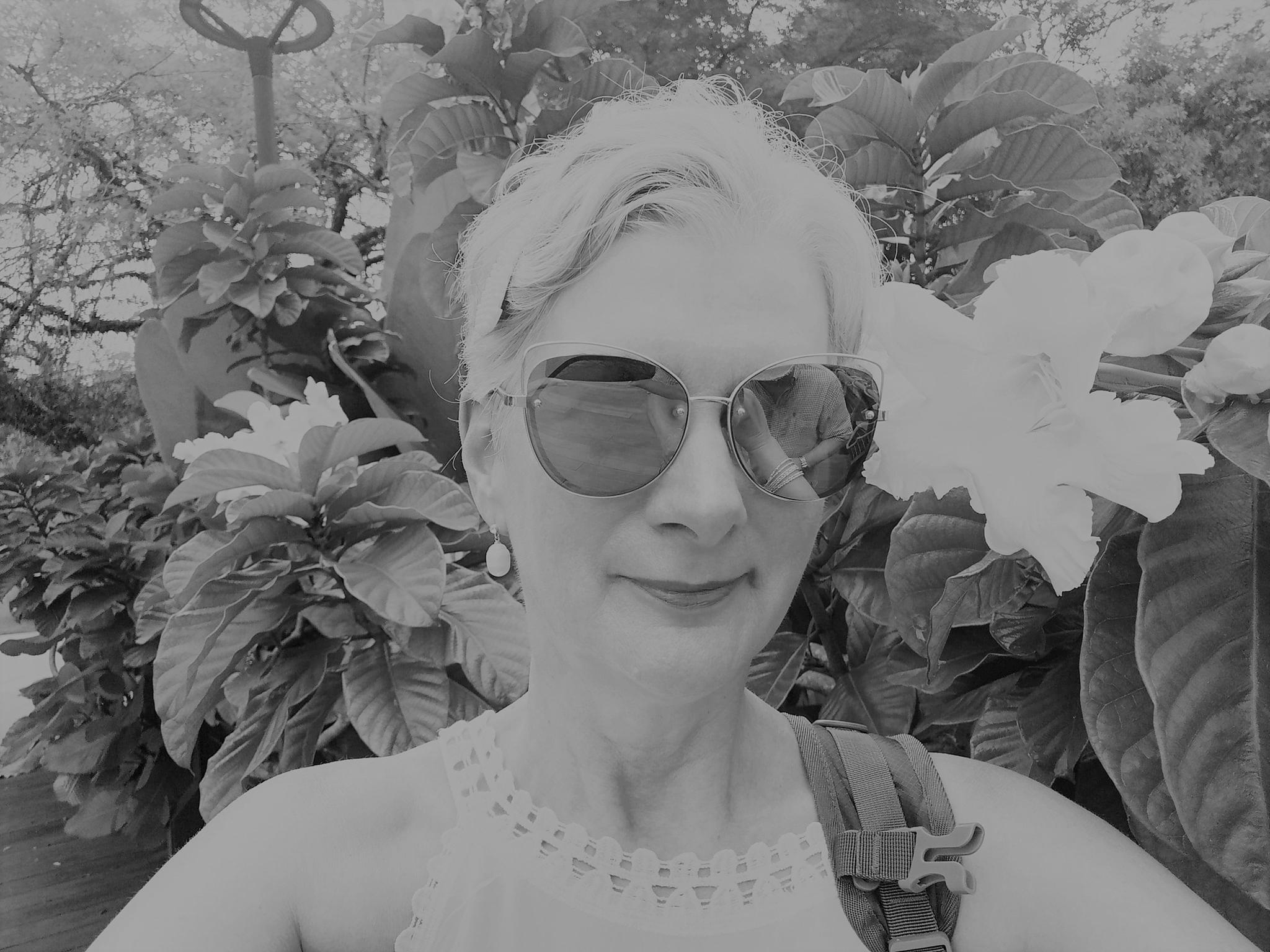
Psychology Content Creator
- A hypothesis is a testable statement written as a prediction of what the researcher expects to find as a result of their experiment
- A hypothesis should be no more than one sentence long
- The hypothesis needs to include the independent variable (IV) and the dependent variable (DV)
- For example - stating that you will measure ‘aggression’ is not enough ('aggression' has not been operationalised)
- by exposing some children to an aggressive adult model whilst other children are not exposed to an aggressive adult model (operationalisation of the IV)
- number of imitative and non-imitative acts of aggression performed by the child (operationalisation of the DV)
The Experimental Hypothesis
- Children who are exposed to an aggressive adult model will perform more acts of imitative and non-imitative aggression than children who have not been exposed to an aggressive adult model
- The experimental hypothesis can be written as a directional hypothesis or as a non-directional hypothesis
The Experimental Hypothesis: Directional
- A directional experimental hypothesis (also known as one-tailed) predicts the direction of the change/difference (it anticipates more specifically what might happen)
- A directional hypothesis is usually used when there is previous research which support a particular theory or outcome i.e. what a researcher might expect to happen
- Participants who drink 200ml of an energy drink 5 minutes before running 100m will be faster (in seconds) than participants who drink 200ml of water 5 minutes before running 100m
- Participants who learn a poem in a room in which loud music is playing will recall less of the poem's content than participants who learn the same poem in a silent room
The Experimental Hypothesis: Non-Directional
- A non-directional experimental hypothesis (also known as two -tailed) does not predict the direction of the change/difference (it is an 'open goal' i.e. anything could happen)
- A non-directional hypothesis is usually used when there is either no or little previous research which support a particular theory or outcome i.e. what the researcher cannot be confident as to what will happen
- There will be a difference in time taken (in seconds) to run 100m depending on whether participants have drunk 200ml of an energy drink or 200ml of water 5 minutes before running
- There will be a difference in recall of a poem depending on whether participants learn the poem in a room in which loud music is playing or in a silent room
The Null Hypothesis
- All published psychology research must include the null hypothesis
- There will be no difference in children's acts of imitative and non-imitative aggression depending on whether they have observed an aggressive adult model or a non-aggressive adult model
- The null hypothesis has to begin with the idea that the IV will have no effect on the DV because until the experiment is run and the results are analysed it is impossible to state anything else!
- To put this in 'laymen's terms: if you bought a lottery ticket you could not predict that you are going to win the jackpot: you have to wait for the results to find out (spoiler alert: the chances of this happening are soooo low that you might as well save your cash!)
- There will be no difference in time taken (in seconds) to run 100m depending on whether participants have drunk 200ml of an energy drink or 200ml of water 5 minutes before running
- There will be no difference in recall of a poem depending on whether participants learn the poem in a room in which loud music is playing or in a silent room
- (NB this is not quite so slick and easy with a directional hypothesis as this sort of hypothesis will never begin with 'There will be a difference')
- this is why the null hypothesis is so important - it tells the researcher whether or not their experiment has shown a difference in conditions (which is generally what they want to see, otherwise it's back to the drawing board...)
Worked example
Jim wants to test the theory that chocolate helps your ability to solve word-search puzzles
He believes that sugar helps memory as he has read some research on this in a text book
He puts up a poster in his sixth-form common room asking for people to take part after school one day and explains that they will be required to play two memory games, where eating chocolate will be involved
(a) Should Jim use a directional hypothesis in this study? Explain your answer (2 marks)
(b) Write a suitable hypothesis for this study. (4 marks)
a) Jim should use a directional hypothesis (1 mark)
because previous research exists that states what might happen (2 nd mark)
b) 'Participants will remember more items from a shopping list in a memory game within the hour after eating 50g of chocolate, compared to when they have not consumed any chocolate'
- 1 st mark for directional
- 2 nd mark for IV- eating chocolate
- 3 rd mark for DV- number of items remembered
- 4 th mark for operationalising both IV & DV
- If you write a non-directional or null hypothesis the mark is 0
- If you do not get the direction correct the mark is zero
- Remember to operationalise the IV & DV
You've read 0 of your 10 free revision notes
Get unlimited access.
to absolutely everything:
- Downloadable PDFs
- Unlimited Revision Notes
- Topic Questions
- Past Papers
- Model Answers
- Videos (Maths and Science)
Join the 100,000 + Students that ❤️ Save My Exams
the (exam) results speak for themselves:
Did this page help you?
Author: Claire Neeson
Claire has been teaching for 34 years, in the UK and overseas. She has taught GCSE, A-level and IB Psychology which has been a lot of fun and extremely exhausting! Claire is now a freelance Psychology teacher and content creator, producing textbooks, revision notes and (hopefully) exciting and interactive teaching materials for use in the classroom and for exam prep. Her passion (apart from Psychology of course) is roller skating and when she is not working (or watching 'Coronation Street') she can be found busting some impressive moves on her local roller rink.
Causality between autoimmune diseases and breast cancer: a two-sample Mendelian randomization study in a European population
- Open access
- Published: 01 September 2024
- Volume 15 , article number 396 , ( 2024 )
Cite this article
You have full access to this open access article
- Hengheng Zhang 1 , 2 na1 ,
- Guoshuang Shen 2 na1 ,
- Ping Yang 1 , 2 na1 ,
- Meijie Wu 1 , 2 ,
- Jinming Li 1 , 2 ,
- Zitao Li 2 ,
- Fuxing Zhao 2 ,
- Hongxia Liang 2 ,
- Mengting Da 2 ,
- Ronghua Wang 2 ,
- Chengrong Zhang 1 , 2 ,
- Jiuda Zhao 2 &
- Yi Zhao 2
The incidence of autoimmune diseases and breast cancer is significantly higher in women compared to men. Previous observational studies have not conclusively determined the relationship between these two conditions. This study utilizes the Mendelian randomization approach to investigate the genetic association between autoimmune diseases and breast cancer.
Two-sample Mendelian randomization was conducted on a European population using the GWAS database. The inverse variance-weighted method served as the primary analytical approach. The MR-PRESSO test was applied to detect horizontal pleiotropy. To ensure result robustness, the FDR correction method was used.
The study revealed that Sjögren’s syndrome lowers the overall risk of breast cancer (OR 0.96, 95% CI [0.93–0.99], p = 0.011). Idiopathic inflammatory myopathy shows a protective effect against overall breast cancer (OR 0.98, 95% CI [0.97–0.99], p = 0.035). An association was identified between rheumatoid arthritis and overall breast cancer (OR 0.98, 95% CI [0.96–1.00], p = 0.050). No causal link was found between systemic lupus erythematosus, systemic sclerosis, and overall breast cancer. The study also suggests that Sjögren’s syndrome, rheumatoid arthritis, and idiopathic inflammatory myopathy might reduce the risk of developing HER + breast cancer. Specifically, Sjögren’s syndrome (OR = 0.90, 95% CI [0.83–0.98], p = 0.02), rheumatoid arthritis (OR = 0.94, 95% CI [0.91–0.98], p = 0.006), and idiopathic inflammatory myopathy (OR = 0.96, 95% CI [0.93–0.99], p = 0.036). Additionally, systemic lupus erythematosus was found to lower the risk of HER- breast cancer (OR = 0.95, 95% CI [0.91–0.99], p = 0.046). The study did not establish a causal relationship between these five autoimmune diseases and ER + or ER- breast cancer.
This study found that autoimmune diseases may act as protective factors against breast cancer risk.
Avoid common mistakes on your manuscript.
1 Introduction
Breast cancer ranks as one of the most common malignant tumors in women, exhibiting the highest incidence and mortality rates among female cancers [ 1 ]. Current data show about 2.26 million new cases of breast cancer diagnosed globally each year [ 1 , 2 ]. Breast cancer can be categorized into four subtypes based on hormone receptor (estrogen receptor, progesterone receptor) and human epidermal growth factor receptor 2 (HER2) status: HR + /HER2-, HR-/HER2-, HR + /HER2 + , and HR-/HER2 + . These subtypes are clinically important as receptor expression directly impacts treatment strategies [ 3 ]. Additionally, breast cancer is a highly heterogeneous disease. The molecular characteristics of breast cancer subtypes do not appear to be fixed but rather represent a dynamic entity that can change during tumor progression and metastasis. This presents a challenge for clinicians. Further research into the etiology of breast cancer and its subtypes will provide effective management strategies for disease prevention and treatment [ 4 , 5 , 6 ]. This presents a challenge for clinicians. Further research into the etiology of breast cancer and its subtypes will provide effective management strategies for disease prevention and treatment [ 7 ]. Studies have identified a clear link between diet, alcohol intake, and the development of breast cancer. Furthermore, there is a proposed connection between chronic inflammation and the progression of breast cancer [ 8 , 9 , 10 ].
Autoimmune diseases (ADs) are the most common chronic inflammatory conditions [ 11 ]. ADs occur when the immune system mistakenly attacks healthy cells, tissues, or organs, causing inflammatory damage [ 12 ]. As research delves into the molecular aspects of diseases, increasing attention is being paid to the relationship between autoimmune diseases and malignant tumors [ 13 ]. Nonetheless, the association between autoimmune diseases and breast cancer remains controversial. Some studies suggest that autoimmune diseases may increase the risk of breast cancer, while others indicate a possible reduction in this risk. For example, research by S. Bernatsky indicates a decreased risk of breast cancer among patients with SLE [ 14 ]. Similarly, Warren David Raymond’s findings align with this perspective, showing reduced odds of colorectal, breast, and skin cancers but higher rates of hematologic malignancies in SLE patients [ 15 ]. Meanwhile, Wenjie Li et al.’s research reports no causal relationship between SLE and overall breast cancer, ER + breast cancer, or ER- breast cancer in European cohorts, with a lower risk of breast cancer in East Asian SLE patients [ 16 ].
To better understand the relationship between autoimmune diseases and breast cancer, this study employed Mendelian randomization (MR) to investigate potential genetic correlations. MR is a causal inference method that uses genetic variations to study causal relationships, helping to reduce the influence of confounding factors [ 17 ]. Additionally, MR can lessen the chance of reverse causation since genetic variations are generally not affected by disease onset or progression [ 18 ]. This study considered five common autoimmune diseases as potential exposures and evaluated breast cancer and its various subtypes as outcome variables.
2.1 Study design
This study conducted two-sample Mendelian randomization (MR) analyses using summarized genetic data and IEU OpenGWAS database to evaluate the potential causal relationship between autoimmune diseases (ADs) and breast cancer risk. The instrumental variables (IVs) in this analysis adhered to three criteria: First, IVs had a strong association with ADs. Second, IVs were independent of potential confounders between ADs and cancer. Third, IVs influenced cancer risk solely through ADs without interference from other factors. Figure 1 illustrates this process.
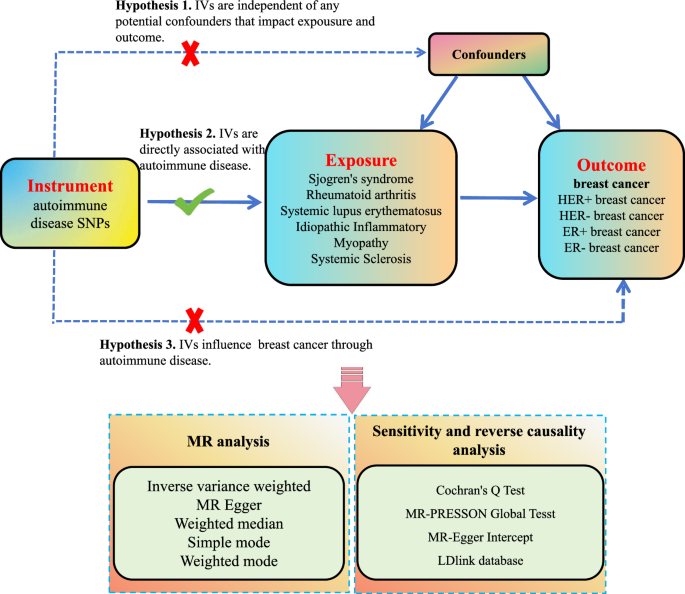
Flow diagram for study
2.2 GWAS data source for autoimmune diseases (ADs)
The study examined five common autoimmune diseases: Sjögren’s syndrome, rheumatoid arthritis, systemic lupus erythematosus, systemic sclerosis, and idiopathic inflammatory myopathy. Genetic data for these diseases were obtained from the IEU OpenGWAS database ( https://gwas.mrcieu.ac.uk/ ). Instrumental variables were selected based on a genome-wide significance threshold of p < 5 × 10 –8 , with an LD threshold of (LD r 2 < 0.001, kb = 10,000) to ensure effective linkage disequilibrium [ 19 ]. For idiopathic inflammatory myopathy and systemic sclerosis, a significance level of p < 1 × 10 –6 was used, with an LD r 2 < 0.01 criterion. These standards are consistent with previous studies [ 20 ]. To reduce bias from weak instrumental variables, the study calculated R 2 to determine the proportion of phenotypic variance each instrumental variable explained. The R 2 formula: R 2 = [2 × EAF × (1-EAF) × (β)^2]/[(2 × EAF × (1-EAF) × (β)^2) + (2 × EAF × (1-EAF) × N × se (β)^2)], where EAF is the effect allele frequency, β the effect size, N the sample size, and se(β) the standard error of the genetic effect [ 18 ]. F-statistics were then computed to assess the strength of each instrumental variable, using the formula: F = [r 2 × (N-k-1)]/[(1- R 2 ) × k], where k is the number of instrumental variables [ 21 ]. SNPs with an F-value < 10 were deemed weak and excluded from further analysis. For comprehensive details on the genetic instruments selected for each autoimmune disease, see Table 1 .
2.3 GWAS data source for breast cancer
For breast cancer data, this study used statistical information on European populations from the IEU OpenGWAS database ( https://gwas.mrcieu.ac.uk/ ) and previous studies. Data were mainly derived from the Breast Cancer Association Consortium (BCAC) GWAS, which included 228,951 European women, 122,977 of whom had breast cancer and 105,974 were controls [ 22 ]. Furthermore, GWAS data from Saori Sakaue's study, involving 17,389 breast cancer patients and 240,341 controls, were also incorporated [ 23 ]. Detailed information about the GWAS data for breast cancer is presented in Table 2 .
2.4 Statistical analysis
The statistical analysis in this research focused on determining the causal relationship between ADs and breast cancer, and we performed MR analyses using inverse variance weighting (IVW), weighted median, simple modal, weighted modal, and MR Egger methods. IVW is the primary MR analysis method, which includes the MR effects of individual snp to derive an overall weighted effect [ 24 ]. We used the other four methods as complementary methods to test for possible violations of the MR second and third hypotheses. When the Egger-intercept of linear regression approaches 0, there is no directional pleiotropy in IVs and the exclusion hypothesis can be considered valid [ 25 ]. The hypothesis can be considered valid. The analysis by the above method can make our results more robust.
We used the MR-PRESSO method to detect and remove outliers and then generated inverse variance-weighted estimates [ 26 ]. After removing the outliers, we calculated the inverse variance-weighted estimates again and used the P -value of the MR-PRESSO distortion test to assess whether the difference between the estimates before and after removal was significant. We then performed a sensitivity analysis on the remaining SNPs [ 27 ].
We searched the LDlink database ( https://ldlink.nih.gov/?tab=home ) for potentially causal SNPs to verify whether the instrumental variables (IVs) met the independence assumption [ 28 ]. The results of the search showed that seven SNPs were confounders, so we removed them when performing the MR analysis (Supplementary Table 1). In addition, we used Cochrane’s Q-test and MR multivariate residual sums to assess heterogeneity, thus increasing the reliability of our findings. egger_intercept of P _value and MR-egger intercept derived P_ value greater than 0.05 did not heterogeneity existed [ 29 ]. Leave-One-Out (LOO) analysis validated the MR estimates’ robustness and identified any SNP-driven associations.
Statistical analyses were conducted using R software version 4.3.1, with TwoSampleMR and MR-PRESSO packages. To ensure conclusion reliability, the study applied False Discovery Rate (FDR) correction, using q-values to manage the FDR. The FDR-corrected threshold was set at 0.05, allowing for up to 5% of positive findings to be false positives.
3.1 Association between autoimmune diseases and overall breast cancer risk
The study conducted a two-sample MR analysis using European population-based breast cancer GWAS data from Saori Sakaue's study to explore the link between autoimmune diseases and overall breast cancer risk. According to Fig. 2 , results from the Inverse Variance Weighted (IVW) method indicated a significant reduction in overall breast cancer risk for patients with Sjögren’s syndrome(OR 0.96, 95% CI [0.93–0.99], p = 0.011) and idiopathic inflammatory myopathy (OR 0.98, 95% CI [0.97–0.99], p = 0.035).Additionally, rheumatoid arthritis was found to have a correlated risk of breast cancer (OR 0.98, 95% CI [0.96–1.00], p = 0.050). The findings remained consistent after applying the FDR correction. The MR-Egger and Weighted Median method estimates aligned with the IVW results.
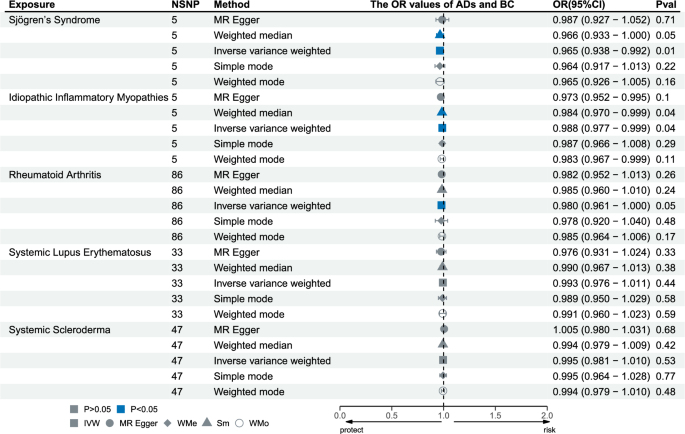
Forest plot of OR values between five autoimmune diseases and overall breast cancer
We analyzed exposure factors with breast cancer one by one. Two SNPs, rs3129962, rs3132487, were found to have palindromic sequences when analyzing desiccation syndrome with breast cancer, and we deleted them. No SNPs with horizontal pleiotropy were found when MR-PRESSO test was performed. rs3129767, rs9272305, two SNPs with palindromic sequences were found when analyzing idiopathic myositis myopathy with breast cancer, and we deleted them but did not find the presence of SNPs with horizontal pleiotropy. Analyzing the relationship between rheumatoid arthritis and overall breast cancer, we identified three SNPs, rs1858037, rs34536443, and rs7278257, contained palindromic sequences and therefore decided to remove them. In addition, when performing the MR-PRESSO test, we identified two SNPs, rs1950897 and rs3025669, as having horizontal pleiotropy, and therefore excluded them as well. Finally, the data after removing the SNPs were analyzed to obtain the above results.
Figures 3 , 4 and 5 include a scatter plot illustrating the relationship between autoimmune diseases and overall breast cancer, as well as a leave-one-out method analysis plot. The information on heterogeneity and MR-PRESSO test for autoimmune diseases and overall breast cancer in Table 3 . We provide SNP data for the analysis of exposure factors with breast cancer in Supplementary Tables 2–6.
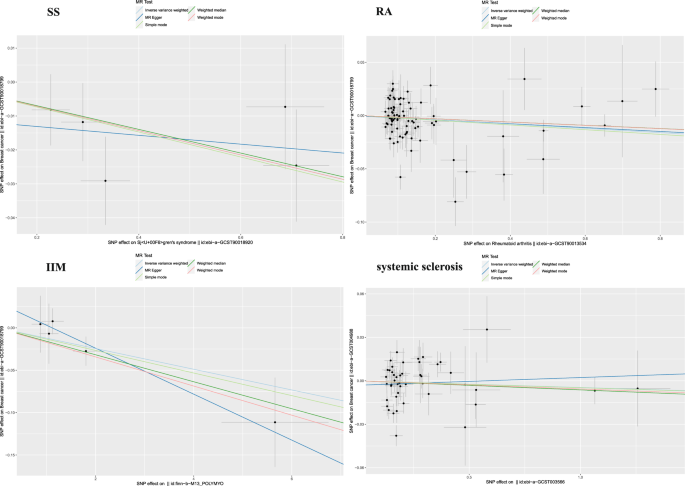
Scatter plot of association between five autoimmune diseases and overall breast cancer
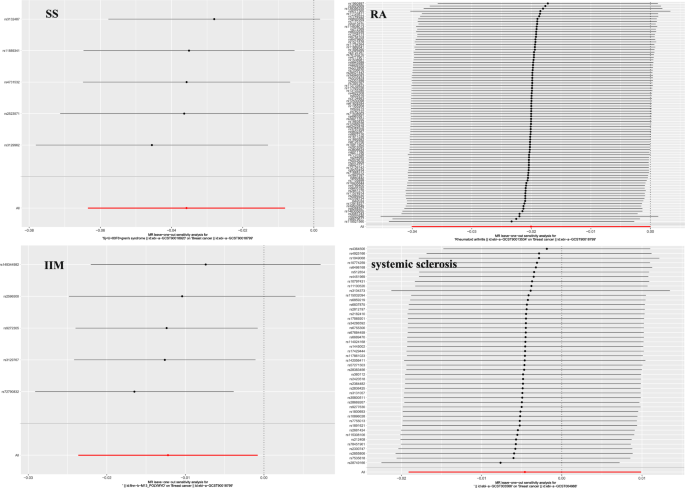
A forest plot illustrating ‘leave-one-out’ sensitivity analysis, showcasing individual SNP influences on results
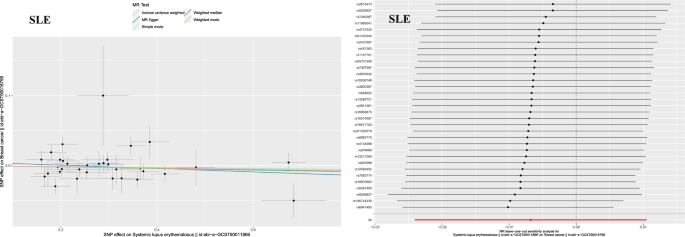
Scatter plot and Leave-one-out analysis of the causal relationship between systemic lupus erythematosus and overall breast cancer
3.2 Autoimmune diseases and HER- and HER + breast cancer risk
Research indicates a strong link between human epidermal growth factor receptor (HER) expression status in breast cancer and patient prognosis. Utilizing data from the Breast Cancer Association Consortium (BCAC) GWAS, this study explored the potential causal relationship between autoimmune diseases and HER- and HER + breast cancer. According to Fig. 6 , analysis via the Inverse Variance Weighted (IVW) method suggests that Sjögren's syndrome, rheumatoid arthritis, and idiopathic inflammatory myopathy might reduce the risk of developing HER + breast cancer. Specifically, Sjögren’s syndrome showed an odds ratio (OR) of 0.90(95% CI [0.83–0.98], p = 0.02), indicating a significant reduction in risk. Rheumatoid arthritis had an OR of 0.94 (95% CI [0.91–0.98], p = 0.006), suggesting a lower likelihood of HER + breast cancer in individuals with this condition. Idiopathic inflammatory myopathy was associated with a reduced risk (OR 0.96, 95% CI [0.93–0.99], p = 0.036). Additionally, systemic lupus erythematosus was linked to a slightly decreased risk of HER- breast cancer (OR 0.95, 95% CI [0.91–0.99], p = 0.046). After applying False Discovery Rate (FDR) corrections, the results indicated significant causal relationships between rheumatoid arthritis, idiopathic inflammatory myopathy, and HER + breast cancer, but not between Sjögren’s syndrome and HER + breast cancer. Also, a significant causal link was found between systemic lupus erythematosus and a reduced risk of HER- breast cancer.

Forest plot of OR values between five autoimmune diseases and breast cancer subtype
When analyzing exposure factors with HER + breast cancer, a palindrome sequence was found in the SNP (rs34536443) analyzed for rheumatoid arthritis with HER + breast cancer, which was removed. Also when we analyzed dry syndrome and idiopathic inflammatory myopathy with HER + breast cancer, no palindromic sequences were found. There was no horizontal pleiotropy when MR-presso test was performed ( P _value > 0.05).
The association between systemic lupus erythematosus (SLE) and HER-breast cancer was investigated. We found that rs11185603 was associated with Fasting glucose, and rs5749502 with Red blood cell traits, while rs4844538 was associated with type 2 diabetes. These SNPs may have confounding effects and were therefore excluded from the analysis. In addition, we found the presence of palindromic sequences for rs11059928 and rs11185603, which were also excluded for analytical accuracy considerations. Horizontal pleiotropy was not present at MR-presso test (P_value = 0.763).
Leave-One-Out analysis revealed that no instrumental variables significantly influenced the causal relationship between autoimmune diseases and HER + and HER- breast cancer. Supplementary Tables 7–10, we also provide SNP data that provide an analysis of exposure factors with HER + /HER- breast cancer.
3.3 Autoimmune diseases and ER- and ER + breast cancer risk
Other studies have highlighted differences in prognosis among breast cancer patients based on estrogen receptor (ER) expression status. This study reanalyzed data from the Breast Cancer Association Consortium (BCAC) GWAS dataset for ER + and ER- breast cancer. However, the analysis using the Inverse Variance Weighted (IVW) method found no causal relationship between the five autoimmune diseases and ER + or ER- breast cancer in Fig. 6 .
Supplementary Figs. 1 to 2 (eFig.1–2) include a scatter plot illustrating the relationship between autoimmune diseases and breast cancer subtype, as well as a leave-one-out method analysis plot. The information on heterogeneity and MR-PRESSO test for autoimmune diseases and breast cancer subtype in Supplementary Table 11 to 14 (eTable.11–14).
4 Discussion
Breast cancer is a major health challenge for women worldwide, and its etiology is complex and diverse [ 2 ]. Currently, clinical therapeutic strategies are mainly based on the classification of estrogen receptor (ER) and human epidermal growth factor receptor 2 (HER2) status [ 6 ]. The classification of breast cancer subtypes is closely related to the expression of ER and HER2 receptors, and it is important to explore the pathogenic factors of breast cancer subtypes for clinical prevention and treatment. Autoimmune diseases such as dry syndrome, rheumatoid arthritis and systemic lupus erythematosus have a high prevalence in women, while breast cancer is the most common malignant tumor in women [ 30 , 31 ]. To investigate whether there is a correlation between the two, we conducted the present study.
The findings indicate a reduced risk of breast cancer in patients with Sjögren’s syndrome, idiopathic inflammatory myopathy, and rheumatoid arthritis, showing odds ratios(OR) of 0.96 (95% CI [0.93–0.99], p = 0.011), 0.98 (95% CI [0.97–0.99], p = 0.035), and 0.98 (95% CI [0.96–1.00], p = 0.050), respectively. The observed protective effect of Sjögren's syndrome on breast cancer risk corroborates Jian Deng's research findings [ 32 ].
Moreover, the study delved into the association between autoimmune diseases and breast cancer across different HER and ER expression states. It revealed that systemic lupus erythematosus significantly reduces the risk of HER- breast cancer (OR = 0.95, 95% CI [0.91–0.99], p = 0.046). Idiopathic inflammatory myopathy significantly lowered the risk of HER + breast cancer (OR = 0.96, 95% CI [0.93–0.99], p = 0.036). Sjögren's syndrome and rheumatoid arthritis also appeared to act as protective factors against HER + breast cancer, with statistically significant differences observed (Sjögren's syndrome, OR = 0.90, 95% CI [0.83–0.98], p = 0.02; Rheumatoid arthritis, OR = 0.94, 95% CI [0.91–0.98], p = 0.006).
Notably, the analysis on the correlation between systemic sclerosis and HER- breast cancer yielded a p -value close to 0.05 ( p = 0.06), suggesting a non-significant statistical difference. However, this trend might still indicate a protective effect in patients with HER- breast cancer. The relationship between systemic sclerosis and breast cancer warrants further exploration in future studies as the database expands and more data become available.
According to the results of this study, autoimmune diseases may reduce the risk of developing breast cancer. We believe that the specific mechanisms are as follows. Firstly, the “immunosurveillance” hypothesis proposes that autoimmune diseases are due to an increase in immune tone, which improves immunosurveillance [ 33 ].
Immune surveillance refers to the immune system's process of recognizing and eliminating abnormal cells, such as cancer cells, which includes the phases of elimination, equilibrium, and escape [ 34 ]. Research indicates that tumors can be recognized by the immune system and controlled or prevented through immune surveillance processes [ 35 ]. The specificity of tumor immune responses lies in the recognition of tumor antigens. The immune system identifies and clears nascent tumors by recognizing tumor-specific neoantigens expressed on tumor cells, similar to allograft rejection, thus maintaining tissue homeostasis in complex multicellular organisms [ 34 , 36 ]. In clinical settings, the restoration of immune surveillance is considered a measure of the effectiveness of chemotherapy, targeted therapy, and radiotherapy in breast cancer patients [ 37 ].
Supporting this, studies have shown that individuals with broad autoantibody specificity can decrease their risk of breast cancer by 60% [ 38 ]. Additionally, research has reported a decreased risk of breast cancer in patients with systemic lupus erythematosus (SLE), which may be associated with anti-DNA antibodies. These antibodies exert direct anti-cancer effects on cells with DNA repair deficiencies [ 38 ]. This aligns with findings that systemic lupus erythematosus significantly lowers breast cancer risk, which is consistent with the results of this study [ 39 ]. Further mechanistic studies have revealed that a cell-penetrating lupus autoantibody (3E10) is synthetically lethal to BRCA2-deficient human cancer cells and can enhance the effectiveness of low doses of doxorubicin, thereby improving chemotherapy outcomes [ 40 ]. Additionally, James Gardner Thorpe's study reported that lupus-associated anti-ribosome P autoantibodies could directly induce apoptosis in cancer cells [ 41 ]. From the treatment perspective of autoimmune diseases, certain studies have noted that using non-steroidal anti-inflammatory drugs or aspirin can boost tumor immune surveillance and lower cancer risk [ 42 , 43 ]. On the contrary, glucocorticoid use may disrupt immune surveillance and elevate cancer risk [ 44 ].
Previous studies on Sjögren’s syndrome (SS) have consistently reported a decreased incidence of breast cancer, which aligns with our findings. Hemminki et al. compared 1516 Swedish SS patients with the general population, noting a standardized incidence ratio (SIR) for breast cancer of 0.46 (95% confidence interval: 0.26–0.75) [ 45 ]. Several factors may explain the lower risk of breast cancer in SS patients. SS primarily affects exocrine glands, especially the lacrimal and salivary glands, which share anatomical, histological, and immunological similarities with the breast. SS patients display different immune patterns in breast-associated mucosal tissues, with higher proportions of CD4 + T cells. This suggests a distinct immune response to local antigens, such as tumor cells, potentially establishing a more robust mucosal immune system dynamic that may reduce breast cancer incidence [ 45 , 46 ]. Additionally, studies have identified lower estrogen levels in SS patients, which significantly contribute to reducing breast cancer incidence [ 47 ]. Estrogen is a known risk factor for breast cancer development, so lower estrogen levels may provide protective effects against the disease.
Our findings suggest that rheumatoid arthritis may reduce the risk of developing HER + breast cancer. It has been shown that rheumatoid arthritis (RA) may reduce breast cancer risk, which is consistent with our findings [ 48 ]. The mechanisms may include the following: Firstly, the peak incidence of female RA often occurs during menopause, characterized by decreased hormone secretion, including estrogen. Lower estrogen levels are known to reduce breast cancer risk. Furthermore, studies have demonstrated significant differences in luteinizing hormone (LH) and follicle-stimulating hormone (FSH) levels between RA patients and healthy individuals. These hormones are crucial in regulating reproductive function and sex hormone levels, and their fluctuations may further influence breast cancer risk [ 49 ]. In cohorts of patients with idiopathic inflammatory myopathies, reports indicate that individuals with breast and ovarian cancers often carry anti-synthetase antibodies, which possess anticancer properties [ 50 , 51 ]. These observations suggest a complex interplay between autoimmunity and cancer incidence, necessitating further exploration through in vivo and in vitro studies. Overall, these findings highlight the significant role of the immune system and autoimmune diseases in regulating breast cancer risk.
However, to date, no significant link between ER + and ER- breast cancer and autoimmune diseases has been established in genome-wide association studies (GWAS) using European population samples. Future updates to these databases may provide opportunities for more in-depth exploration of this relationship. This study has several strengths: Primarily, it is the first to utilize Mendelian Randomization (MR) to investigate potential causality between autoimmune diseases and breast cancers with varying HER and ER states. MR methodology significantly minimizes the effects of confounding factors and reverse causation. Compared to traditional observational studies, the analysis of single nucleotide polymorphisms (SNPs) reduces the impact of multiple potential confounders, offering more robust research evidence.
Nonetheless, there are limitations to this study. First, the sample predominantly consisted of the European population. Whether these findings are applicable to other regions and populations remains uncertain, necessitating further validation in diverse populations and regions. Secondly, the potential for pleiotropy is an issue that cannot be entirely ruled out in MR studies and requires additional investigation and discussion. Furthermore, due to variations in data collection times, disease progression stages, and treatment phases in GWAS data, our study has some limitations. These factors may affect the research outcomes to a certain extent, but we can still derive valuable SNP data analysis results from them. Further, our study is based on genome-wide association study (GWAS) data, revealing potential genetic factors in relation to disease. However, these findings are limited to statistical associations and do not provide direct mechanistic causality. Therefore, our findings need to be validated by in vivo experiments in future studies to further reveal the underlying biological mechanisms.
5 Conclusion
The Mendelian Randomization (MR) analysis in this study indicates that autoimmune diseases may act as protective factors against breast cancer risk. Based on studies in European populations, there is a potential genetic correlation between desiccation syndrome, rheumatoid arthritis, idiopathic inflammatory myopathies and HER + breast cancer. In addition, we observed a potential genetic correlation between systemic lupus erythematosus and HER-breast cancer as well.
Availability of data and materials
The data used in this study was obtained from public databases and previous studies. Data sources and handing of these data were described in the Materials and Methods. Further information is available from the corresponding author upon request. All data involved in this study are available in the public database. For further information, please contact the corresponding authors. Data is provided in a manuscript or supplementary information file where our data is available.
Giaquinto AN, Sung H, Miller KD, Kramer JL, Newman LA, Minihan A, Siegel RL. Breast cancer statistics, 2022. CA A Cancer J Clin. 2022;72(6):524–41. https://doi.org/10.3322/caac.21754 .
Article Google Scholar
Arnold M, Morgan E, Rumgay H, Mafra A, Singh D, Laversanne M, Soerjomataram I. Current and future burden of breast cancer: global statistics for 2020 and 2040. Breast. 2022;66:15–23. https://doi.org/10.1016/j.breast.2022.08.010 .
Article PubMed PubMed Central Google Scholar
Howlader N, Altekruse SF, Li CI, Chen VW, Clarke CA, Ries LAG, Cronin KA. US incidence of breast cancer subtypes defined by joint hormone receptor and HER2 status. JNCI J Natl Cancer Inst. 2014. https://doi.org/10.1093/jnci/dju055 .
Article PubMed Google Scholar
Priedigkeit N, Hartmaier RJ, Chen Y, Vareslija D, Basudan A, Watters RJ, Lee AV. Intrinsic subtype switching and acquired ERBB2/HER2 amplifications and mutations in breast cancer brain metastases. JAMA Oncol. 2017;3(5):666–71. https://doi.org/10.1001/jamaoncol.2016.5630 .
Garcia-Recio S, Thennavan A, East MP, Parker JS, Cejalvo JM, Garay JP, Perou CM. FGFR4 regulates tumor subtype differentiation in luminal breast cancer and metastatic disease. J Clin Investig. 2020;130(9):4871–87. https://doi.org/10.1172/JCI130323 .
Jordan NV, Bardia A, Wittner BS, Benes C, Ligorio M, Zheng Y, Haber DA. HER2 expression identifies dynamic functional states within circulating breast cancer cells. Nature. 2016;537(7618):102–6. https://doi.org/10.1038/nature19328 .
Turner KM, Yeo SK, Holm TM, Shaughnessy E, Guan J-L. Heterogeneity within molecular subtypes of breast cancer. Am J Physiol Cell Physiol. 2021;321(2):C343–54. https://doi.org/10.1152/ajpcell.00109.2021 .
Campbell NJ, Barton C, Cutress RI, Copson ER. Impact of obesity, lifestyle factors and health interventions on breast cancer survivors. Proc Nutr Soc. 2023;82(1):47–57. https://doi.org/10.1017/S0029665122002816 .
De Visser KE, Eichten A, Coussens LM. Paradoxical roles of the immune system during cancer development. Nat Rev Cancer. 2006;6(1):24–37. https://doi.org/10.1038/nrc1782 .
Papadimitriou N, Markozannes G, Kanellopoulou A, Critselis E, Alhardan S, Karafousia V, Tsilidis KK. An umbrella review of the evidence associating diet and cancer risk at 11 anatomical sites. Nat Commun. 2021;12(1):4579. https://doi.org/10.1038/s41467-021-24861-8 .
Furman D, Campisi J, Verdin E, Carrera-Bastos P, Targ S, Franceschi C, Slavich GM. Chronic inflammation in the etiology of disease across the life span. Nat Med. 2019;25(12):1822–32. https://doi.org/10.1038/s41591-019-0675-0 .
Zhernakova A, Van Diemen CC, Wijmenga C. Detecting shared pathogenesis from the shared genetics of immune-related diseases. Nat Rev Genet. 2009;10(1):43–55. https://doi.org/10.1038/nrg2489 .
Hemminki K, Liu X, Ji J, Försti A, Sundquist J, Sundquist K. Effect of autoimmune diseases on risk and survival in female cancers. Gynecol Oncol. 2012;127(1):180–5. https://doi.org/10.1016/j.ygyno.2012.07.100 .
Bernatsky S, Ramsey-Goldman R, Foulkes WD, Gordon C, Clarke AE. Breast, ovarian, and endometrial malignancies in systemic lupus erythematosus: a meta-analysis. Br J Cancer. 2011;104(9):1478–81. https://doi.org/10.1038/bjc.2011.115 .
Raymond WD, Preen DB, Keen HI, Inderjeeth CA, Nossent JC. Cancer development in patients hospitalized with systemic lupus erythematosus: a population-level data linkage study. Int J Rheum Dis. 2023;26(8):1557–70. https://doi.org/10.1111/1756-185X.14784 .
Exploring the causality and pathogenesis of systemic lupus erythematosus in breast cancer based on Mendelian randomization and transcriptome data analyses—PubMed. (n.d.). https://pubmed.ncbi.nlm.nih.gov/36726984/ . Accessed 27 Jul 2024.
Davey Smith G, Ebrahim S. ‘Mendelian randomization’: can genetic epidemiology contribute to understanding environmental determinants of disease?*. Int J Epidemiol. 2003;32(1):1–22. https://doi.org/10.1093/ije/dyg070 .
Boef AGC, Dekkers OM, Le Cessie S. Mendelian randomization studies: a review of the approaches used and the quality of reporting. Int J Epidemiol. 2015;44(2):496–511. https://doi.org/10.1093/ije/dyv071 .
Papadimitriou N, Dimou N, Gill D, Tzoulaki I, Murphy N, Riboli E, Tsilidis KK. Genetically predicted circulating concentrations of micronutrients and risk of breast cancer: a Mendelian randomization study. Int J Cancer. 2021;148(3):646–53. https://doi.org/10.1002/ijc.33246 .
Chen F, Wen W, Long J, Shu X, Yang Y, Shu X, Zheng W. Mendelian randomization analyses of 23 known and suspected risk factors and biomarkers for breast cancer overall and by molecular subtypes. Int J Cancer. 2022;151(3):372–80. https://doi.org/10.1002/ijc.34026 .
Brion M-JA, Shakhbazov K, Visscher PM. Calculating statistical power in Mendelian randomization studies. Int J Epidemiol. 2013;42(5):1497–501. https://doi.org/10.1093/ije/dyt179 .
Michailidou K, Lindström S, Dennis J, Beesley J, Hui S, Kar S, Easton DF. Association analysis identifies 65 new breast cancer risk loci. Nature. 2017;551(7678):92–4. https://doi.org/10.1038/nature24284 .
Sakaue S, Kanai M, Tanigawa Y, Karjalainen J, Kurki M, Koshiba S, Okada Y. A cross-population atlas of genetic associations for 220 human phenotypes. Nat Genet. 2021;53(10):1415–24. https://doi.org/10.1038/s41588-021-00931-x .
Bowden J, Spiller W, Fabiola Del Greco M, Sheehan N, Thompson J, Minelli C, Smith GD. Improving the visualization, interpretation and analysis of two-sample summary data Mendelian randomization via the Radial plot and Radial regression. Int J Epidemiol. 2018;47(6):2100. https://doi.org/10.1093/ije/dyy265 .
Bowden J, Davey Smith G, Burgess S. Mendelian randomization with invalid instruments: effect estimation and bias detection through Egger regression. Int J Epidemiol. 2015;44(2):512–25. https://doi.org/10.1093/ije/dyv080 .
Evaluating the potential role of pleiotropy in Mendelian randomization studies—PubMed. (n.d.). https://pubmed.ncbi.nlm.nih.gov/29771313/ . Accessed 27 Jul 2024.
Verbanck M, Chen C-Y, Neale B, Do R. Detection of widespread horizontal pleiotropy in causal relationships inferred from Mendelian randomization between complex traits and diseases. Nat Genet. 2018;50(5):693–8. https://doi.org/10.1038/s41588-018-0099-7 .
Machiela MJ, Chanock SJ. LDassoc: an online tool for interactively exploring genome-wide association study results and prioritizing variants for functional investigation. Bioinformatics. 2018;34(5):887–9. https://doi.org/10.1093/bioinformatics/btx561 .
Burgess S, Thompson SG. Interpreting findings from Mendelian randomization using the MR-Egger method. Eur J Epidemiol. 2017;32(5):377–89. https://doi.org/10.1007/s10654-017-0255-x .
Qin B, Wang J, Yang Z, Yang M, Ma N, Huang F, Zhong R. Epidemiology of primary Sjögren’s syndrome: a systematic review and meta-analysis. Ann Rheum Dis. 2015;74(11):1983–9. https://doi.org/10.1136/annrheumdis-2014-205375 .
Association between comorbidities and extraglandular manifestations in primary Sjögren’s syndrome: a multicenter cross-sectional study—PubMed. (n.d.). https://pubmed.ncbi.nlm.nih.gov/32146615/ . Accessed 27 Jul 2024.
Deng J, Liu M, Xiao R, Wang J, Liao X, Ye Z, Sun Z. Risk, incidence, and mortality of breast cancer in primary sjögren’s syndrome: a systematic review and meta-analysis. Front Immunol. 2022;13:904682. https://doi.org/10.3389/fimmu.2022.904682 .
Pol J, Paillet J, Plantureux C, Kroemer G. Beneficial autoimmunity and maladaptive inflammation shape epidemiological links between cancer and immune-inflammatory diseases. OncoImmunology. 2022;11(1):2029299. https://doi.org/10.1080/2162402X.2022.2029299 .
Schreiber RD, Old LJ, Smyth MJ. Cancer immunoediting: integrating immunity’s roles in cancer suppression and promotion. Science. 2011;331(6024):1565–70. https://doi.org/10.1126/science.1203486 .
Finn OJ. Immuno-oncology: understanding the function and dysfunction of the immune system in cancer. Ann Oncol. 2012;23:viii6–9. https://doi.org/10.1093/annonc/mds256 .
Ribatti D. The concept of immune surveillance against tumors: the first theories. Oncotarget. 2017;8(4):7175–80. https://doi.org/10.1863/oncotarget.12739 .
Zitvogel L, Galluzzi L, Smyth MJ, Kroemer G. Mechanism of action of conventional and targeted anticancer therapies: reinstating immunosurveillance. Immunity. 2013;39(1):74–88. https://doi.org/10.1016/j.immuni.2013.06.014 .
Shah AA, Igusa T, Goldman D, Li J, Casciola-Rosen L, Rosen A, Petri M. Association of systemic lupus erythematosus autoantibody diversity with breast cancer protection. Arthritis Res Ther. 2021;23(1):64. https://doi.org/10.1186/s13075-021-02449-3 .
Bernatsky S, Ramsey-Goldman R, Labrecque J, Joseph L, Boivin J-F, Petri M, Clarke AE. Cancer risk in systemic lupus: an updated international multi-centre cohort study. J Autoimmunity. 2013;42:130–5. https://doi.org/10.1016/j.jaut.2012.12.009 .
Hansen JE, Chan G, Liu Y, Hegan DC, Dalal S, Dray E, Glazer MP. Targeting cancer with a lupus autoantibody. Sci Transl Med. 2012. https://doi.org/10.1126/scitranslmed.3004385 .
Gardner-Thorpe J, Ito H, Ashley SW, Whang EE. Autoantibody-mediated inhibition of pancreatic cancer cell growth in an athymic (Nude) mouse model. Pancreas. 2003;27(2):180–9. https://doi.org/10.1097/00006676-200308000-00012 .
Castoldi F, Humeau J, Martins I, Lachkar S, Loew D, Dingli F, Pietrocola F. Autophagy-mediated metabolic effects of aspirin. Cell Death Discov. 2020;6(1):129. https://doi.org/10.1038/s41420-020-00365-0 .
Zhang Y, Chen H, Chen S, Li Z, Chen J, Li W. The effect of concomitant use of statins, NSAIDs, low-dose aspirin, metformin and beta-blockers on outcomes in patients receiving immune checkpoint inhibitors: a systematic review and meta-analysis. OncoImmunology. 2021;10(1):1957605. https://doi.org/10.1080/2162402X.2021.1957605 .
Yang H, Xia L, Chen J, Zhang S, Martin V, Li Q, Ma Y. Stress–glucocorticoid–TSC22D3 axis compromises therapy-induced antitumor immunity. Nat Med. 2019;25(9):1428–41. https://doi.org/10.1038/s41591-019-0566-4 .
Goulabchand R, Malafaye N, Jacot W, Witkowski Durand Viel P, Morel J, Lukas C, Guilpain P. Cancer incidence in primary Sjögren’s syndrome: data from the French hospitalization database. Autoimmun Rev. 2021;20(12):102987. https://doi.org/10.1016/j.autrev.2021.102987 .
Goulabchand R, Hafidi A, Millet I, Morel J, Lukas C, Humbert S, Guilpain P. Mastitis associated with Sjögren’s syndrome: a series of nine cases. Immunol Res. 2017;65(1):218–29. https://doi.org/10.1007/s12026-016-8830-x .
McCoy SS, Sampene E, Baer AN. Association of Sjögren’s syndrome with reduced lifetime sex hormone exposure: a case-control study. Arthritis Care Res. 2020;72(9):1315–22. https://doi.org/10.1002/acr.24014 .
Incidence of malignancy in adult patients with rheumatoid arthritis: a meta-analysis—PubMed. (n.d.). https://pubmed.ncbi.nlm.nih.gov/26271620/ . Accessed 27 Jul 2024.
Cevik R, Em S, Gur A, Nas K, Sarac AJ, Çolpan L. Sex and thyroid hormone status in women with rheumatoid arthritis: are there any effects of menopausal state and disease activity on these hormones?: Sex and thyroid hormone status in women with RA. Int J Clin Pract. 2004;58(4):327–32. https://doi.org/10.1111/j.1368-5031.2004.00005.x .
Oldroyd AGS, Allard AB, Callen JP, Chinoy H, Chung L, Fiorentino D, Aggarwal R. A systematic review and meta-analysis to inform cancer screening guidelines in idiopathic inflammatory myopathies. Rheumatology. 2021;60(6):2615–28. https://doi.org/10.1093/rheumatology/keab166 .
Mecoli CA, Igusa T, Chen M, Wang X, Albayda J, Paik JJ, Shah AA. Subsets of idiopathic inflammatory myositis enriched for contemporaneous cancer relative to the general population. Arthr Rheumatol. 2023;75(4):620–9. https://doi.org/10.1002/art.42311 .
Download references
Acknowledgements
We are grateful to the investigators who shared the GWAS data. At the same time, I would like to thank the Central Government of Qinghai Province to guide the local Science and Technology Development Fund (approval number: 2022ZY009).
This study was supported by Central Government Guiding Local Scientific and Technological Development Funds for Qinghai Province in China (Grant ID: 2022ZY009).
Author information
Hengheng Zhang, Guoshuang Shen, Ping Yang have contributed equally (Co-first authors).
Authors and Affiliations
Qinghai University, Xining, China
Hengheng Zhang, Ping Yang, Meijie Wu, Jinming Li & Chengrong Zhang
The Center of Breast Disease Diagnosis and Treatment of Affiliated Hospital of Qinghai University & Affiliated Cancer Hospital of Qinghai University, Xining, 810000, China
Hengheng Zhang, Guoshuang Shen, Ping Yang, Meijie Wu, Jinming Li, Zitao Li, Fuxing Zhao, Hongxia Liang, Mengting Da, Ronghua Wang, Chengrong Zhang, Jiuda Zhao & Yi Zhao
You can also search for this author in PubMed Google Scholar
Contributions
H.h.Z and Y.Z orchestrated the conception and design of the study. Data collection and analysis were spearheaded by C.r.Z. R.h.w and J.m.L The initial manuscript was drafted by H.h.Z and M.j.Wu, M.t.D, H.x.L, P.Y and G.h.S significantly contributed to data analysis and interpretation. F.x.Z, Z.t.L, Y.Z and J.d.Z meticulously revised the manuscript. H.h.Z Drawing.Every author actively participated in writing the article and endorsed the final version submitted for publication. Furthermore, all authors have thoroughly reviewed and approved the published manuscript.
Corresponding authors
Correspondence to Jiuda Zhao or Yi Zhao .
Ethics declarations
Ethics approval and consent to participate.
Not needed.
Informed consent
The consent of the patients was not required because the informed consent had already been given in the original study.
Competing interests
On behalf of all authors, the corresponding author states that there is no conflict of interest.
Additional information
Publisher's note.
Springer Nature remains neutral with regard to jurisdictional claims in published maps and institutional affiliations.
Supplementary Information
Additional file 1., additional file 2., additional file 3., additional file 4., additional file 5., additional file 6., rights and permissions.
Open Access This article is licensed under a Creative Commons Attribution-NonCommercial-NoDerivatives 4.0 International License, which permits any non-commercial use, sharing, distribution and reproduction in any medium or format, as long as you give appropriate credit to the original author(s) and the source, provide a link to the Creative Commons licence, and indicate if you modified the licensed material. You do not have permission under this licence to share adapted material derived from this article or parts of it. The images or other third party material in this article are included in the article’s Creative Commons licence, unless indicated otherwise in a credit line to the material. If material is not included in the article’s Creative Commons licence and your intended use is not permitted by statutory regulation or exceeds the permitted use, you will need to obtain permission directly from the copyright holder. To view a copy of this licence, visit http://creativecommons.org/licenses/by-nc-nd/4.0/ .
Reprints and permissions
About this article
Zhang, H., Shen, G., Yang, P. et al. Causality between autoimmune diseases and breast cancer: a two-sample Mendelian randomization study in a European population. Discov Onc 15 , 396 (2024). https://doi.org/10.1007/s12672-024-01269-6
Download citation
Received : 10 January 2024
Accepted : 23 August 2024
Published : 01 September 2024
DOI : https://doi.org/10.1007/s12672-024-01269-6
Share this article
Anyone you share the following link with will be able to read this content:
Sorry, a shareable link is not currently available for this article.
Provided by the Springer Nature SharedIt content-sharing initiative
- Autoimmune diseases
- Breast cancer
- Mendelian randomization
- European population
Advertisement
- Find a journal
- Publish with us
- Track your research

IMAGES
VIDEO
COMMENTS
A hypothesis is a testable statement about how something works in the natural world. While some hypotheses predict a causal relationship between two variables, other hypotheses predict a correlation between them. According to the Research Methods Knowledge Base, a correlation is a single number that describes the ...
The directional hypothesis can also state a negative correlation, e.g. the higher the number of face-book friends, the lower the life satisfaction score ". Non-directional hypothesis: A non-directional (or two tailed hypothesis) simply states that there will be a difference between the two groups/conditions but does not say which will be ...
The alternative-hypothesis states that there is a significant correlation (there is a linear relation) between \ (x\) and \ (y\). The t-test is a statistical test for the correlation coefficient. It can be used when \ (x\) and \ (y\) are linearly related, the variables are random variables, and when the population of the variable \ (y\) is ...
Directional hypothesis: A directional hypothesis provides a perspective of the expected relationship between variables, predicting the direction of that relationship (either positive, negative, or a specific difference). Non-directional hypothesis: A non-directional hypothesis denotes the possibility of a relationship between two variables ...
Directional hypotheses, also known as one-tailed hypotheses, are statements in research that make specific predictions about the direction of a relationship or difference between variables. Unlike non-directional hypotheses, which simply state that there is a relationship or difference without specifying its direction, directional hypotheses ...
The correlation coefficient, r, tells us about the strength and direction of the linear relationship between x and y. However, the reliability of the linear model also depends on how many observed data points are in the sample. We need to look at both the value of the correlation coefficient r and the sample size n, together. We perform a hypothesis test of the "significance of the correlation ...
A hypothesis test can either contain a directional hypothesis or a non-directional hypothesis: Directional hypothesis: The alternative hypothesis contains the less than ("<") or greater than (">") sign. This indicates that we're testing whether or not there is a positive or negative effect.
9.4.1 - Hypothesis Testing for the Population Correlation In this section, we present the test for the population correlation using a test statistic based on the sample correlation.
When writing hypotheses there are three things that we need to know: (1) the parameter that we are testing (2) the direction of the test (non-directional, right-tailed or left-tailed), and (3) the value of the hypothesized parameter.
As before, your choice of which alternative hypothesis to use should be specified before you collect data based on your research question and any evidence you might have that would indicate a specific directional (or non-directional) relationship.
A non-directional hypothesis, also known as a two-tailed hypothesis, predicts that there is a difference or relationship between two variables but does not specify the direction of this relationship.
Sometimes a hypothesis states the direction in which the results are expected to go, for example 'studying improves exam marks', 'women are better drivers than men'. With a correlational study, a directional hypothesis states that there is a positive (or negative) correlation between two variables.
The Research Hypothesis. A research hypothesis is a mathematical way of stating a research question. A research hypothesis names the groups (we'll start with a sample and a population), what was measured, and which we think will have a higher mean. The last one gives the research hypothesis a direction. In other words, a research hypothesis ...
Learn how the choice between one and two-tailed hypothesis tests impacts the detectable effects, statistical power, and potential errors.
Three Different Hypotheses: (1) Directional Hypothesis: states that the IV will have an effect on the DV and what that effect will be (the direction of results). For example, eating smarties will significantly improve an individual's dancing ability. When writing a directional hypothesis, it is important that you state exactly how the IV will ...
A non-directional hypothesis, also known as a two tailed hypothesis, is a type of hypothesis that predicts a relationship between variables without specifying the direction of that relationship. Unlike directional hypotheses that predict a specific outcome, non-directional hypotheses simply suggest that a relationship exists without indicating ...
On the other hand, hypotheses may not predict the exact direction and are used in the absence of a theory, or when findings contradict previous studies ( non-directional hypothesis ). 4 In addition, hypotheses can 1) define interdependency between variables ( associative hypothesis ), 4 2) propose an effect on the dependent variable from ...
s: Directional or non-directional? handout number6.2Activity type ConsolidationA straightforward task identifying whether general The handout cove. s both experime. tal and correlational hypotheses are directional or non-directional. The hypotheses.handout summarises the difference between the two There is an extension task concerning what ...
A perfect positive correlation would be expressed as +1. A perfect negative correlation would be expressed as -1. No relationship would be expressed as 0. Both positive and negative coefficient correlations can be described as weak, moderate or strong e.g. a correlation coefficient of 0.03 is a weak positive correlation; a correlation ...
A non-directional hypothesis is a two-tailed hypothesis that does not predict the direction of the difference or relationship (e.g. girls and boys are different in terms of helpfulness).
Hypotheses can be directional (1-tailed) or non-directional (2-tailed); directional hypotheses predict a more specific result than non-directional ones. Cognitive researchers must also frame a null hypothesis which states they will not find the result they are looking for.
The Experimental Hypothesis: Non-Directional A non-directional experimental hypothesis (also known as two-tailed) does not predict the direction of the change/difference (it is an 'open goal' i.e. anything could happen) A non-directional hypothesis is usually used when there is either no or little previous research which support a particular theory or outcome i.e. what the researcher cannot be ...
For now, just remember this... Directional = previous research has found similar findings Non-directional = it is new research or previous findings have been inconclusive Task 4: write a hypothesis for each of these two scenarios, decide whether you need to write a directional or non-directional hypothesis first.
We used the other four methods as complementary methods to test for possible violations of the MR second and third hypotheses. When the Egger-intercept of linear regression approaches 0, there is no directional pleiotropy in IVs and the exclusion hypothesis can be considered valid . The hypothesis can be considered valid.
The complex characteristics of volatility and non-linearity of carbon price pose a serious challenge to accurately predict carbon price. Therefore, this study proposes a new hybrid model for multivariate carbon price forecasting, including feature selection, deep learning, intelligent optimization algorithms, model combination and evaluation indicators.