Home Blog Design Understanding Data Presentations (Guide + Examples)

Understanding Data Presentations (Guide + Examples)
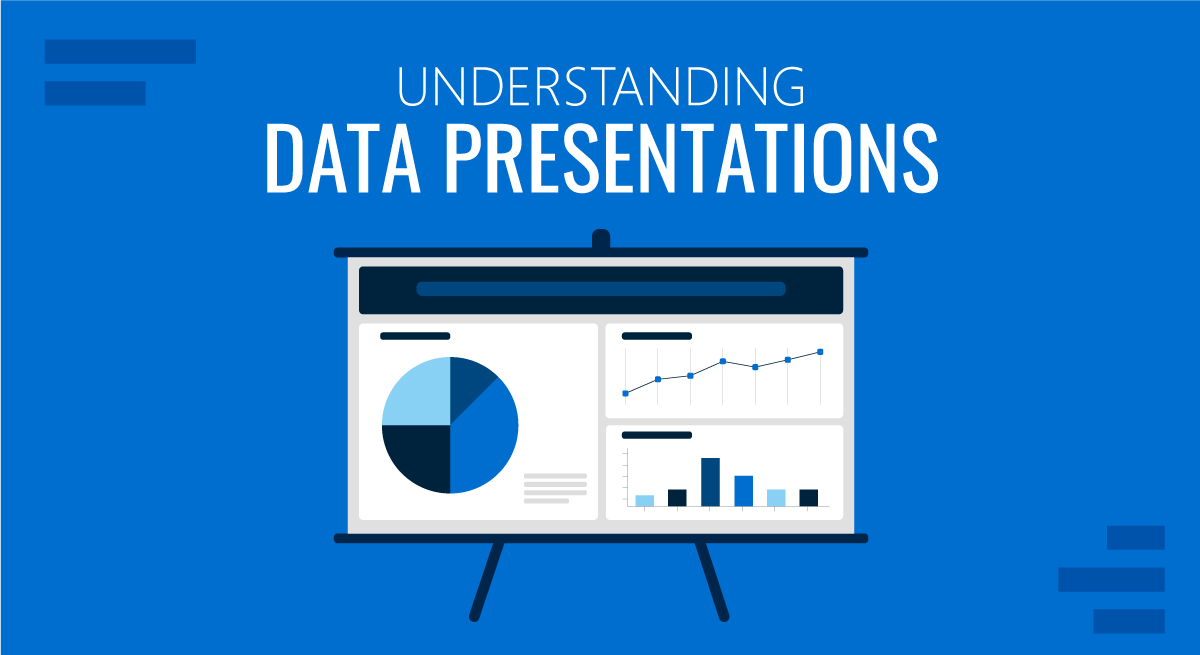
In this age of overwhelming information, the skill to effectively convey data has become extremely valuable. Initiating a discussion on data presentation types involves thoughtful consideration of the nature of your data and the message you aim to convey. Different types of visualizations serve distinct purposes. Whether you’re dealing with how to develop a report or simply trying to communicate complex information, how you present data influences how well your audience understands and engages with it. This extensive guide leads you through the different ways of data presentation.
Table of Contents
What is a Data Presentation?
What should a data presentation include, line graphs, treemap chart, scatter plot, how to choose a data presentation type, recommended data presentation templates, common mistakes done in data presentation.
A data presentation is a slide deck that aims to disclose quantitative information to an audience through the use of visual formats and narrative techniques derived from data analysis, making complex data understandable and actionable. This process requires a series of tools, such as charts, graphs, tables, infographics, dashboards, and so on, supported by concise textual explanations to improve understanding and boost retention rate.
Data presentations require us to cull data in a format that allows the presenter to highlight trends, patterns, and insights so that the audience can act upon the shared information. In a few words, the goal of data presentations is to enable viewers to grasp complicated concepts or trends quickly, facilitating informed decision-making or deeper analysis.
Data presentations go beyond the mere usage of graphical elements. Seasoned presenters encompass visuals with the art of data storytelling , so the speech skillfully connects the points through a narrative that resonates with the audience. Depending on the purpose – inspire, persuade, inform, support decision-making processes, etc. – is the data presentation format that is better suited to help us in this journey.
To nail your upcoming data presentation, ensure to count with the following elements:
- Clear Objectives: Understand the intent of your presentation before selecting the graphical layout and metaphors to make content easier to grasp.
- Engaging introduction: Use a powerful hook from the get-go. For instance, you can ask a big question or present a problem that your data will answer. Take a look at our guide on how to start a presentation for tips & insights.
- Structured Narrative: Your data presentation must tell a coherent story. This means a beginning where you present the context, a middle section in which you present the data, and an ending that uses a call-to-action. Check our guide on presentation structure for further information.
- Visual Elements: These are the charts, graphs, and other elements of visual communication we ought to use to present data. This article will cover one by one the different types of data representation methods we can use, and provide further guidance on choosing between them.
- Insights and Analysis: This is not just showcasing a graph and letting people get an idea about it. A proper data presentation includes the interpretation of that data, the reason why it’s included, and why it matters to your research.
- Conclusion & CTA: Ending your presentation with a call to action is necessary. Whether you intend to wow your audience into acquiring your services, inspire them to change the world, or whatever the purpose of your presentation, there must be a stage in which you convey all that you shared and show the path to staying in touch. Plan ahead whether you want to use a thank-you slide, a video presentation, or which method is apt and tailored to the kind of presentation you deliver.
- Q&A Session: After your speech is concluded, allocate 3-5 minutes for the audience to raise any questions about the information you disclosed. This is an extra chance to establish your authority on the topic. Check our guide on questions and answer sessions in presentations here.
Bar charts are a graphical representation of data using rectangular bars to show quantities or frequencies in an established category. They make it easy for readers to spot patterns or trends. Bar charts can be horizontal or vertical, although the vertical format is commonly known as a column chart. They display categorical, discrete, or continuous variables grouped in class intervals [1] . They include an axis and a set of labeled bars horizontally or vertically. These bars represent the frequencies of variable values or the values themselves. Numbers on the y-axis of a vertical bar chart or the x-axis of a horizontal bar chart are called the scale.
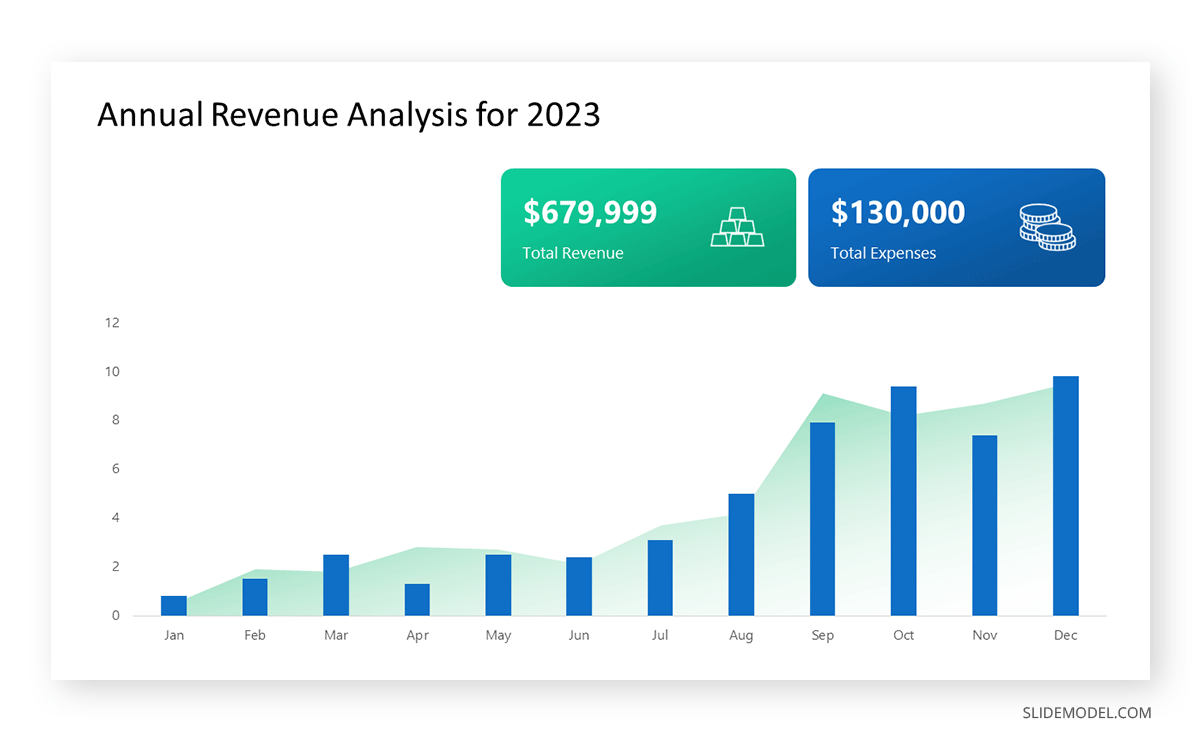
Real-Life Application of Bar Charts
Let’s say a sales manager is presenting sales to their audience. Using a bar chart, he follows these steps.
Step 1: Selecting Data
The first step is to identify the specific data you will present to your audience.
The sales manager has highlighted these products for the presentation.
- Product A: Men’s Shoes
- Product B: Women’s Apparel
- Product C: Electronics
- Product D: Home Decor
Step 2: Choosing Orientation
Opt for a vertical layout for simplicity. Vertical bar charts help compare different categories in case there are not too many categories [1] . They can also help show different trends. A vertical bar chart is used where each bar represents one of the four chosen products. After plotting the data, it is seen that the height of each bar directly represents the sales performance of the respective product.
It is visible that the tallest bar (Electronics – Product C) is showing the highest sales. However, the shorter bars (Women’s Apparel – Product B and Home Decor – Product D) need attention. It indicates areas that require further analysis or strategies for improvement.
Step 3: Colorful Insights
Different colors are used to differentiate each product. It is essential to show a color-coded chart where the audience can distinguish between products.
- Men’s Shoes (Product A): Yellow
- Women’s Apparel (Product B): Orange
- Electronics (Product C): Violet
- Home Decor (Product D): Blue
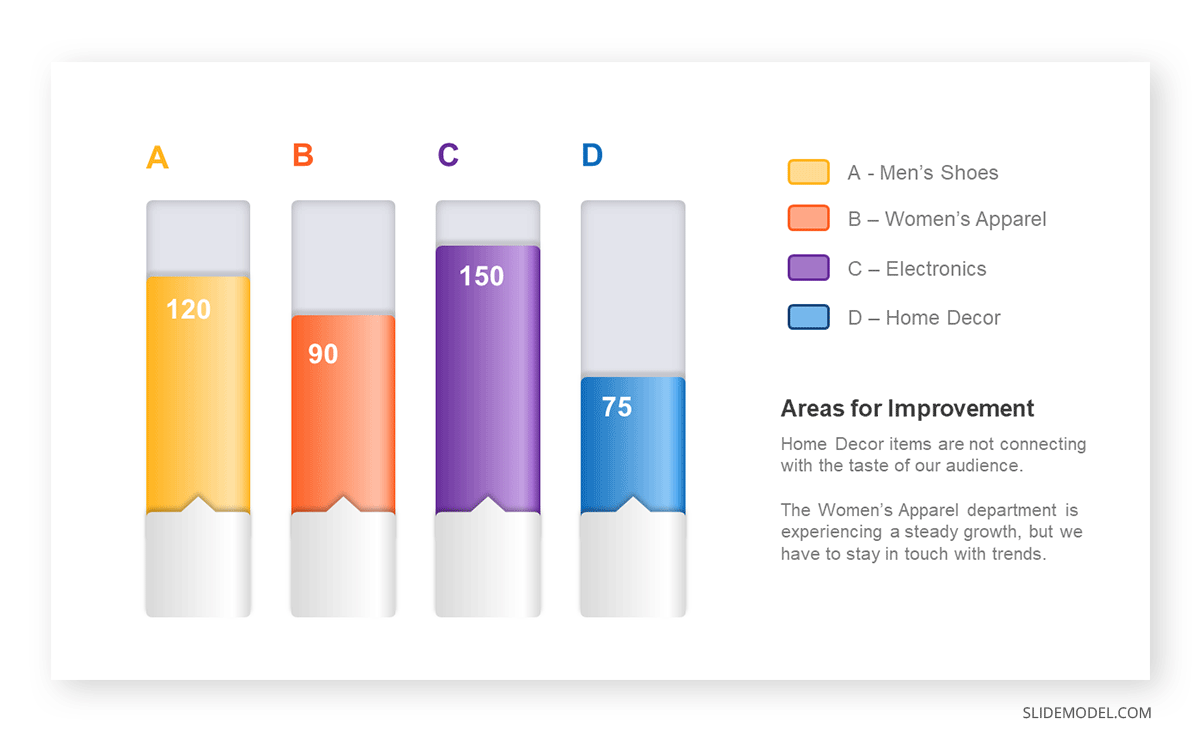
Bar charts are straightforward and easily understandable for presenting data. They are versatile when comparing products or any categorical data [2] . Bar charts adapt seamlessly to retail scenarios. Despite that, bar charts have a few shortcomings. They cannot illustrate data trends over time. Besides, overloading the chart with numerous products can lead to visual clutter, diminishing its effectiveness.
For more information, check our collection of bar chart templates for PowerPoint .
Line graphs help illustrate data trends, progressions, or fluctuations by connecting a series of data points called ‘markers’ with straight line segments. This provides a straightforward representation of how values change [5] . Their versatility makes them invaluable for scenarios requiring a visual understanding of continuous data. In addition, line graphs are also useful for comparing multiple datasets over the same timeline. Using multiple line graphs allows us to compare more than one data set. They simplify complex information so the audience can quickly grasp the ups and downs of values. From tracking stock prices to analyzing experimental results, you can use line graphs to show how data changes over a continuous timeline. They show trends with simplicity and clarity.
Real-life Application of Line Graphs
To understand line graphs thoroughly, we will use a real case. Imagine you’re a financial analyst presenting a tech company’s monthly sales for a licensed product over the past year. Investors want insights into sales behavior by month, how market trends may have influenced sales performance and reception to the new pricing strategy. To present data via a line graph, you will complete these steps.
First, you need to gather the data. In this case, your data will be the sales numbers. For example:
- January: $45,000
- February: $55,000
- March: $45,000
- April: $60,000
- May: $ 70,000
- June: $65,000
- July: $62,000
- August: $68,000
- September: $81,000
- October: $76,000
- November: $87,000
- December: $91,000
After choosing the data, the next step is to select the orientation. Like bar charts, you can use vertical or horizontal line graphs. However, we want to keep this simple, so we will keep the timeline (x-axis) horizontal while the sales numbers (y-axis) vertical.
Step 3: Connecting Trends
After adding the data to your preferred software, you will plot a line graph. In the graph, each month’s sales are represented by data points connected by a line.
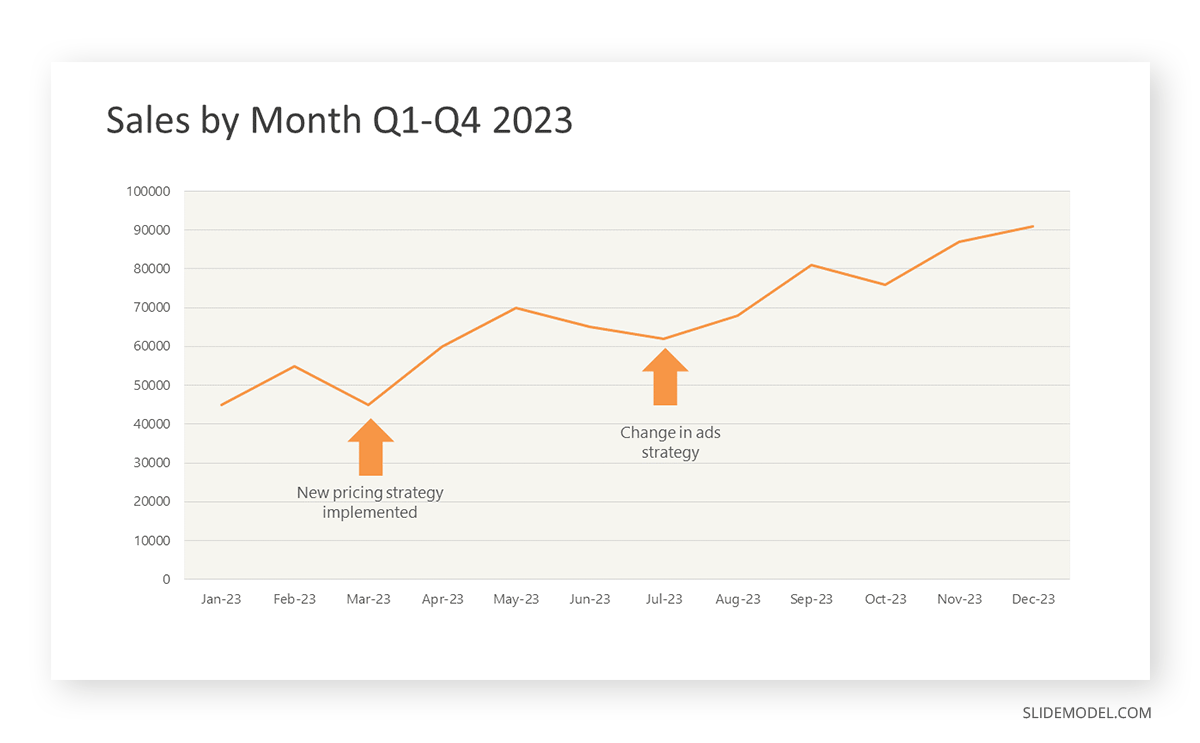
Step 4: Adding Clarity with Color
If there are multiple lines, you can also add colors to highlight each one, making it easier to follow.
Line graphs excel at visually presenting trends over time. These presentation aids identify patterns, like upward or downward trends. However, too many data points can clutter the graph, making it harder to interpret. Line graphs work best with continuous data but are not suitable for categories.
For more information, check our collection of line chart templates for PowerPoint and our article about how to make a presentation graph .
A data dashboard is a visual tool for analyzing information. Different graphs, charts, and tables are consolidated in a layout to showcase the information required to achieve one or more objectives. Dashboards help quickly see Key Performance Indicators (KPIs). You don’t make new visuals in the dashboard; instead, you use it to display visuals you’ve already made in worksheets [3] .
Keeping the number of visuals on a dashboard to three or four is recommended. Adding too many can make it hard to see the main points [4]. Dashboards can be used for business analytics to analyze sales, revenue, and marketing metrics at a time. They are also used in the manufacturing industry, as they allow users to grasp the entire production scenario at the moment while tracking the core KPIs for each line.
Real-Life Application of a Dashboard
Consider a project manager presenting a software development project’s progress to a tech company’s leadership team. He follows the following steps.
Step 1: Defining Key Metrics
To effectively communicate the project’s status, identify key metrics such as completion status, budget, and bug resolution rates. Then, choose measurable metrics aligned with project objectives.
Step 2: Choosing Visualization Widgets
After finalizing the data, presentation aids that align with each metric are selected. For this project, the project manager chooses a progress bar for the completion status and uses bar charts for budget allocation. Likewise, he implements line charts for bug resolution rates.
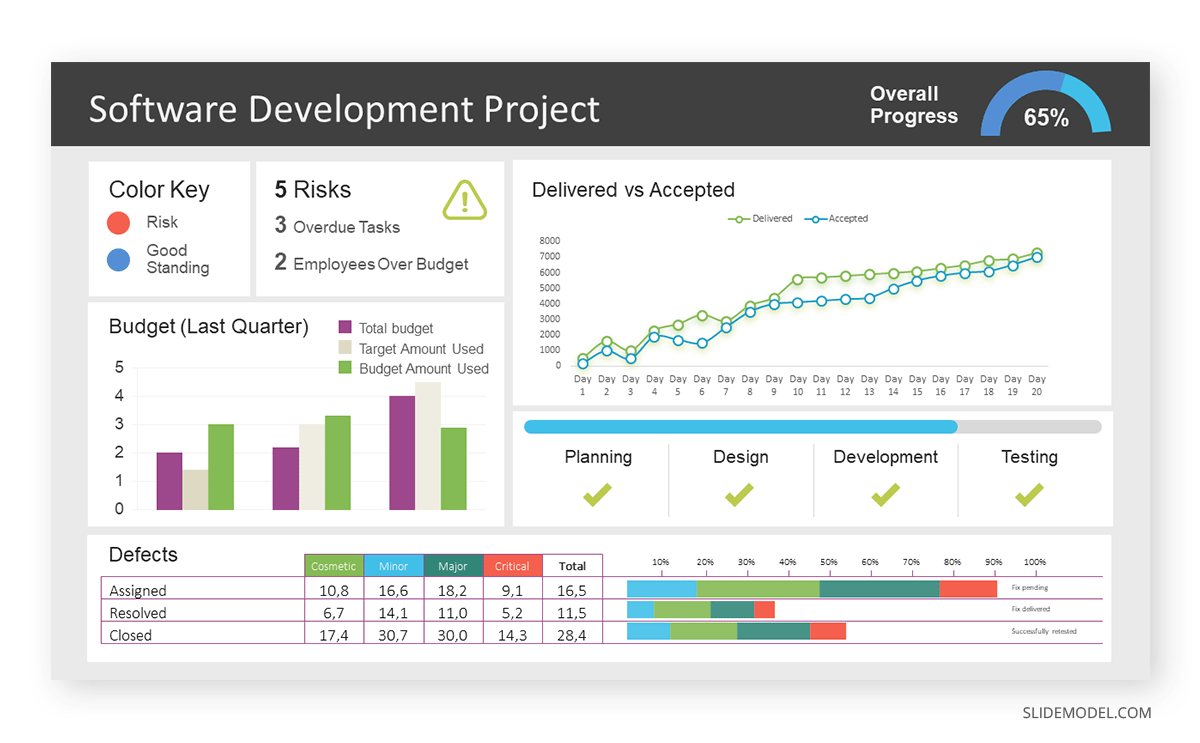
Step 3: Dashboard Layout
Key metrics are prominently placed in the dashboard for easy visibility, and the manager ensures that it appears clean and organized.
Dashboards provide a comprehensive view of key project metrics. Users can interact with data, customize views, and drill down for detailed analysis. However, creating an effective dashboard requires careful planning to avoid clutter. Besides, dashboards rely on the availability and accuracy of underlying data sources.
For more information, check our article on how to design a dashboard presentation , and discover our collection of dashboard PowerPoint templates .
Treemap charts represent hierarchical data structured in a series of nested rectangles [6] . As each branch of the ‘tree’ is given a rectangle, smaller tiles can be seen representing sub-branches, meaning elements on a lower hierarchical level than the parent rectangle. Each one of those rectangular nodes is built by representing an area proportional to the specified data dimension.
Treemaps are useful for visualizing large datasets in compact space. It is easy to identify patterns, such as which categories are dominant. Common applications of the treemap chart are seen in the IT industry, such as resource allocation, disk space management, website analytics, etc. Also, they can be used in multiple industries like healthcare data analysis, market share across different product categories, or even in finance to visualize portfolios.
Real-Life Application of a Treemap Chart
Let’s consider a financial scenario where a financial team wants to represent the budget allocation of a company. There is a hierarchy in the process, so it is helpful to use a treemap chart. In the chart, the top-level rectangle could represent the total budget, and it would be subdivided into smaller rectangles, each denoting a specific department. Further subdivisions within these smaller rectangles might represent individual projects or cost categories.
Step 1: Define Your Data Hierarchy
While presenting data on the budget allocation, start by outlining the hierarchical structure. The sequence will be like the overall budget at the top, followed by departments, projects within each department, and finally, individual cost categories for each project.
- Top-level rectangle: Total Budget
- Second-level rectangles: Departments (Engineering, Marketing, Sales)
- Third-level rectangles: Projects within each department
- Fourth-level rectangles: Cost categories for each project (Personnel, Marketing Expenses, Equipment)
Step 2: Choose a Suitable Tool
It’s time to select a data visualization tool supporting Treemaps. Popular choices include Tableau, Microsoft Power BI, PowerPoint, or even coding with libraries like D3.js. It is vital to ensure that the chosen tool provides customization options for colors, labels, and hierarchical structures.
Here, the team uses PowerPoint for this guide because of its user-friendly interface and robust Treemap capabilities.
Step 3: Make a Treemap Chart with PowerPoint
After opening the PowerPoint presentation, they chose “SmartArt” to form the chart. The SmartArt Graphic window has a “Hierarchy” category on the left. Here, you will see multiple options. You can choose any layout that resembles a Treemap. The “Table Hierarchy” or “Organization Chart” options can be adapted. The team selects the Table Hierarchy as it looks close to a Treemap.
Step 5: Input Your Data
After that, a new window will open with a basic structure. They add the data one by one by clicking on the text boxes. They start with the top-level rectangle, representing the total budget.
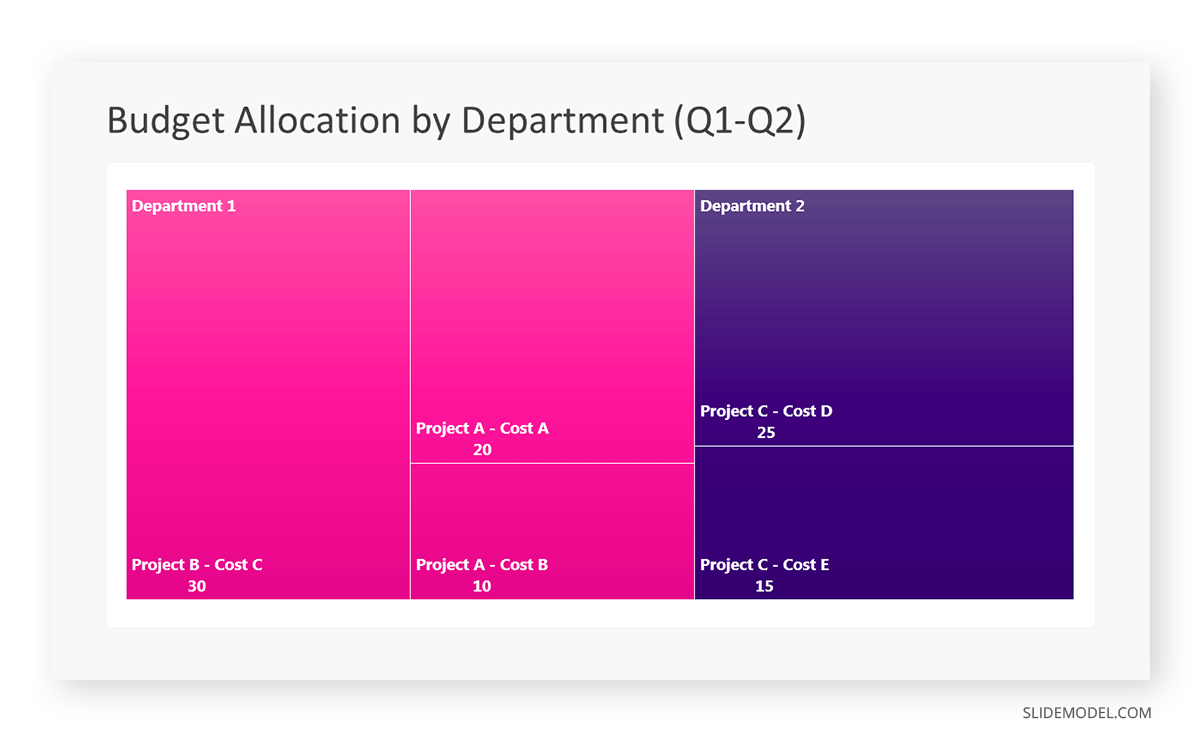
Step 6: Customize the Treemap
By clicking on each shape, they customize its color, size, and label. At the same time, they can adjust the font size, style, and color of labels by using the options in the “Format” tab in PowerPoint. Using different colors for each level enhances the visual difference.
Treemaps excel at illustrating hierarchical structures. These charts make it easy to understand relationships and dependencies. They efficiently use space, compactly displaying a large amount of data, reducing the need for excessive scrolling or navigation. Additionally, using colors enhances the understanding of data by representing different variables or categories.
In some cases, treemaps might become complex, especially with deep hierarchies. It becomes challenging for some users to interpret the chart. At the same time, displaying detailed information within each rectangle might be constrained by space. It potentially limits the amount of data that can be shown clearly. Without proper labeling and color coding, there’s a risk of misinterpretation.
A heatmap is a data visualization tool that uses color coding to represent values across a two-dimensional surface. In these, colors replace numbers to indicate the magnitude of each cell. This color-shaded matrix display is valuable for summarizing and understanding data sets with a glance [7] . The intensity of the color corresponds to the value it represents, making it easy to identify patterns, trends, and variations in the data.
As a tool, heatmaps help businesses analyze website interactions, revealing user behavior patterns and preferences to enhance overall user experience. In addition, companies use heatmaps to assess content engagement, identifying popular sections and areas of improvement for more effective communication. They excel at highlighting patterns and trends in large datasets, making it easy to identify areas of interest.
We can implement heatmaps to express multiple data types, such as numerical values, percentages, or even categorical data. Heatmaps help us easily spot areas with lots of activity, making them helpful in figuring out clusters [8] . When making these maps, it is important to pick colors carefully. The colors need to show the differences between groups or levels of something. And it is good to use colors that people with colorblindness can easily see.
Check our detailed guide on how to create a heatmap here. Also discover our collection of heatmap PowerPoint templates .
Pie charts are circular statistical graphics divided into slices to illustrate numerical proportions. Each slice represents a proportionate part of the whole, making it easy to visualize the contribution of each component to the total.
The size of the pie charts is influenced by the value of data points within each pie. The total of all data points in a pie determines its size. The pie with the highest data points appears as the largest, whereas the others are proportionally smaller. However, you can present all pies of the same size if proportional representation is not required [9] . Sometimes, pie charts are difficult to read, or additional information is required. A variation of this tool can be used instead, known as the donut chart , which has the same structure but a blank center, creating a ring shape. Presenters can add extra information, and the ring shape helps to declutter the graph.
Pie charts are used in business to show percentage distribution, compare relative sizes of categories, or present straightforward data sets where visualizing ratios is essential.
Real-Life Application of Pie Charts
Consider a scenario where you want to represent the distribution of the data. Each slice of the pie chart would represent a different category, and the size of each slice would indicate the percentage of the total portion allocated to that category.
Step 1: Define Your Data Structure
Imagine you are presenting the distribution of a project budget among different expense categories.
- Column A: Expense Categories (Personnel, Equipment, Marketing, Miscellaneous)
- Column B: Budget Amounts ($40,000, $30,000, $20,000, $10,000) Column B represents the values of your categories in Column A.
Step 2: Insert a Pie Chart
Using any of the accessible tools, you can create a pie chart. The most convenient tools for forming a pie chart in a presentation are presentation tools such as PowerPoint or Google Slides. You will notice that the pie chart assigns each expense category a percentage of the total budget by dividing it by the total budget.
For instance:
- Personnel: $40,000 / ($40,000 + $30,000 + $20,000 + $10,000) = 40%
- Equipment: $30,000 / ($40,000 + $30,000 + $20,000 + $10,000) = 30%
- Marketing: $20,000 / ($40,000 + $30,000 + $20,000 + $10,000) = 20%
- Miscellaneous: $10,000 / ($40,000 + $30,000 + $20,000 + $10,000) = 10%
You can make a chart out of this or just pull out the pie chart from the data.
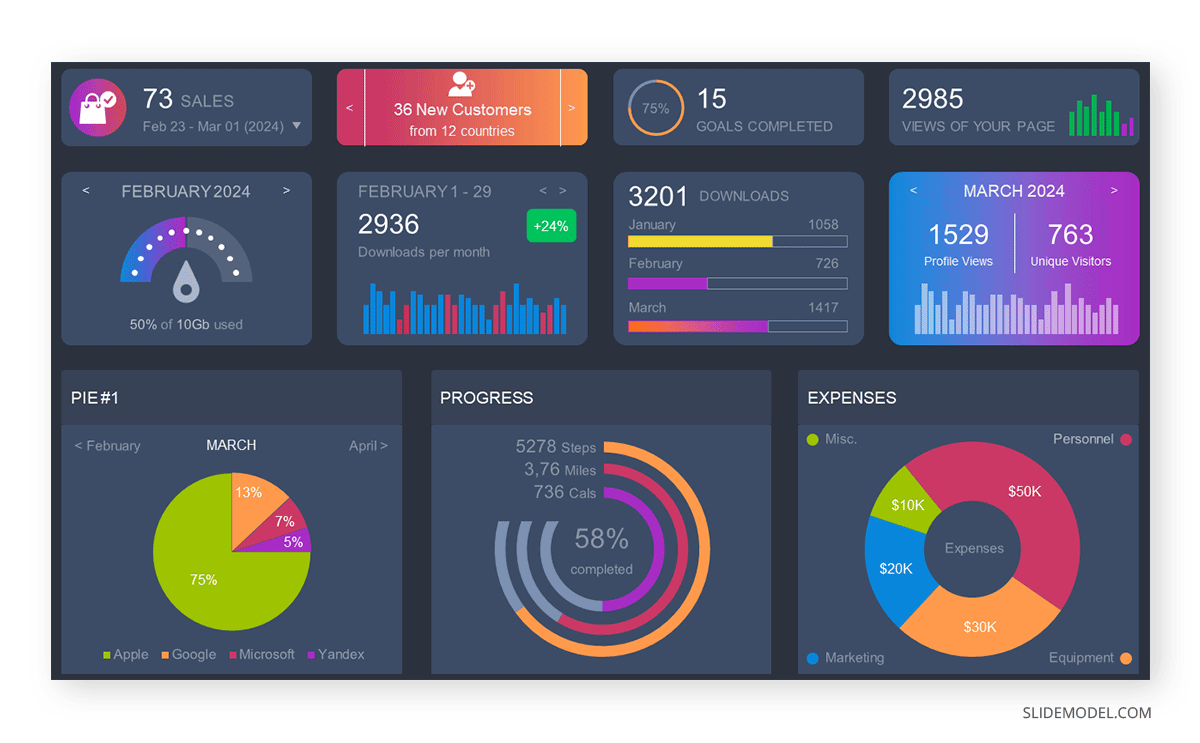
3D pie charts and 3D donut charts are quite popular among the audience. They stand out as visual elements in any presentation slide, so let’s take a look at how our pie chart example would look in 3D pie chart format.
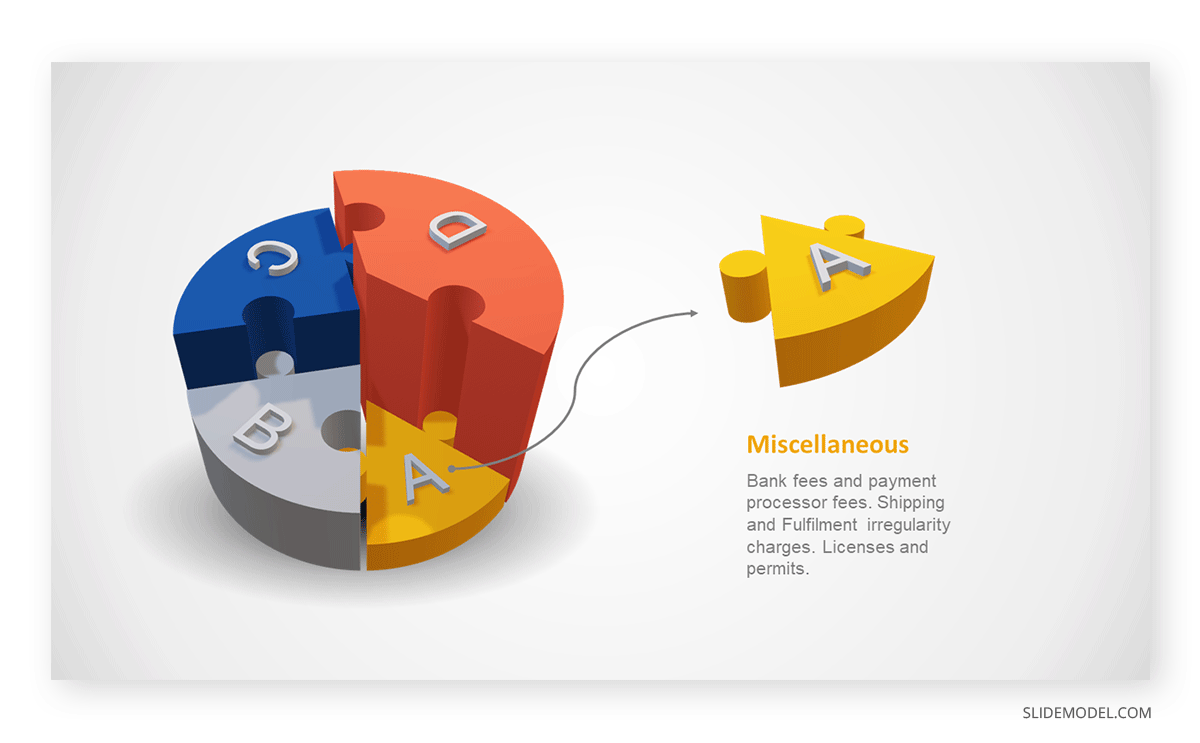
Step 03: Results Interpretation
The pie chart visually illustrates the distribution of the project budget among different expense categories. Personnel constitutes the largest portion at 40%, followed by equipment at 30%, marketing at 20%, and miscellaneous at 10%. This breakdown provides a clear overview of where the project funds are allocated, which helps in informed decision-making and resource management. It is evident that personnel are a significant investment, emphasizing their importance in the overall project budget.
Pie charts provide a straightforward way to represent proportions and percentages. They are easy to understand, even for individuals with limited data analysis experience. These charts work well for small datasets with a limited number of categories.
However, a pie chart can become cluttered and less effective in situations with many categories. Accurate interpretation may be challenging, especially when dealing with slight differences in slice sizes. In addition, these charts are static and do not effectively convey trends over time.
For more information, check our collection of pie chart templates for PowerPoint .
Histograms present the distribution of numerical variables. Unlike a bar chart that records each unique response separately, histograms organize numeric responses into bins and show the frequency of reactions within each bin [10] . The x-axis of a histogram shows the range of values for a numeric variable. At the same time, the y-axis indicates the relative frequencies (percentage of the total counts) for that range of values.
Whenever you want to understand the distribution of your data, check which values are more common, or identify outliers, histograms are your go-to. Think of them as a spotlight on the story your data is telling. A histogram can provide a quick and insightful overview if you’re curious about exam scores, sales figures, or any numerical data distribution.
Real-Life Application of a Histogram
In the histogram data analysis presentation example, imagine an instructor analyzing a class’s grades to identify the most common score range. A histogram could effectively display the distribution. It will show whether most students scored in the average range or if there are significant outliers.
Step 1: Gather Data
He begins by gathering the data. The scores of each student in class are gathered to analyze exam scores.
Names | Score |
---|---|
Alice | 78 |
Bob | 85 |
Clara | 92 |
David | 65 |
Emma | 72 |
Frank | 88 |
Grace | 76 |
Henry | 95 |
Isabel | 81 |
Jack | 70 |
Kate | 60 |
Liam | 89 |
Mia | 75 |
Noah | 84 |
Olivia | 92 |
After arranging the scores in ascending order, bin ranges are set.
Step 2: Define Bins
Bins are like categories that group similar values. Think of them as buckets that organize your data. The presenter decides how wide each bin should be based on the range of the values. For instance, the instructor sets the bin ranges based on score intervals: 60-69, 70-79, 80-89, and 90-100.
Step 3: Count Frequency
Now, he counts how many data points fall into each bin. This step is crucial because it tells you how often specific ranges of values occur. The result is the frequency distribution, showing the occurrences of each group.
Here, the instructor counts the number of students in each category.
- 60-69: 1 student (Kate)
- 70-79: 4 students (David, Emma, Grace, Jack)
- 80-89: 7 students (Alice, Bob, Frank, Isabel, Liam, Mia, Noah)
- 90-100: 3 students (Clara, Henry, Olivia)
Step 4: Create the Histogram
It’s time to turn the data into a visual representation. Draw a bar for each bin on a graph. The width of the bar should correspond to the range of the bin, and the height should correspond to the frequency. To make your histogram understandable, label the X and Y axes.
In this case, the X-axis should represent the bins (e.g., test score ranges), and the Y-axis represents the frequency.
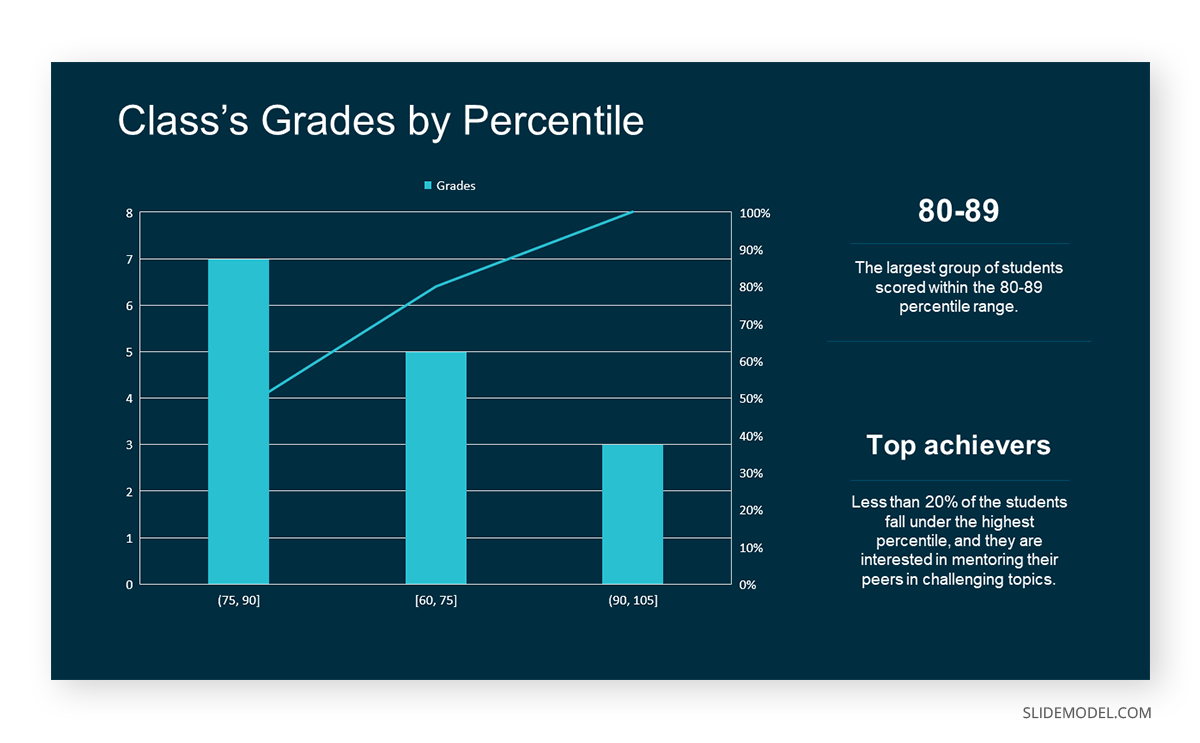
The histogram of the class grades reveals insightful patterns in the distribution. Most students, with seven students, fall within the 80-89 score range. The histogram provides a clear visualization of the class’s performance. It showcases a concentration of grades in the upper-middle range with few outliers at both ends. This analysis helps in understanding the overall academic standing of the class. It also identifies the areas for potential improvement or recognition.
Thus, histograms provide a clear visual representation of data distribution. They are easy to interpret, even for those without a statistical background. They apply to various types of data, including continuous and discrete variables. One weak point is that histograms do not capture detailed patterns in students’ data, with seven compared to other visualization methods.
A scatter plot is a graphical representation of the relationship between two variables. It consists of individual data points on a two-dimensional plane. This plane plots one variable on the x-axis and the other on the y-axis. Each point represents a unique observation. It visualizes patterns, trends, or correlations between the two variables.
Scatter plots are also effective in revealing the strength and direction of relationships. They identify outliers and assess the overall distribution of data points. The points’ dispersion and clustering reflect the relationship’s nature, whether it is positive, negative, or lacks a discernible pattern. In business, scatter plots assess relationships between variables such as marketing cost and sales revenue. They help present data correlations and decision-making.
Real-Life Application of Scatter Plot
A group of scientists is conducting a study on the relationship between daily hours of screen time and sleep quality. After reviewing the data, they managed to create this table to help them build a scatter plot graph:
Participant ID | Daily Hours of Screen Time | Sleep Quality Rating |
---|---|---|
1 | 9 | 3 |
2 | 2 | 8 |
3 | 1 | 9 |
4 | 0 | 10 |
5 | 1 | 9 |
6 | 3 | 7 |
7 | 4 | 7 |
8 | 5 | 6 |
9 | 5 | 6 |
10 | 7 | 3 |
11 | 10 | 1 |
12 | 6 | 5 |
13 | 7 | 3 |
14 | 8 | 2 |
15 | 9 | 2 |
16 | 4 | 7 |
17 | 5 | 6 |
18 | 4 | 7 |
19 | 9 | 2 |
20 | 6 | 4 |
21 | 3 | 7 |
22 | 10 | 1 |
23 | 2 | 8 |
24 | 5 | 6 |
25 | 3 | 7 |
26 | 1 | 9 |
27 | 8 | 2 |
28 | 4 | 6 |
29 | 7 | 3 |
30 | 2 | 8 |
31 | 7 | 4 |
32 | 9 | 2 |
33 | 10 | 1 |
34 | 10 | 1 |
35 | 10 | 1 |
In the provided example, the x-axis represents Daily Hours of Screen Time, and the y-axis represents the Sleep Quality Rating.
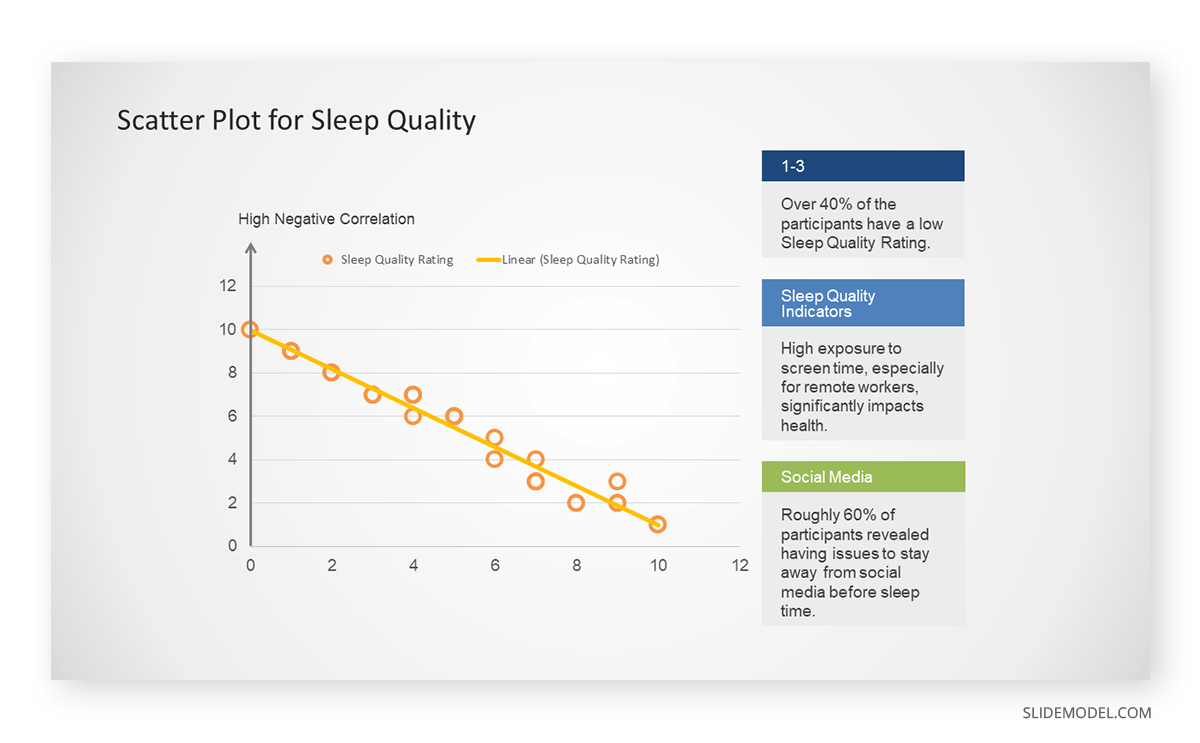
The scientists observe a negative correlation between the amount of screen time and the quality of sleep. This is consistent with their hypothesis that blue light, especially before bedtime, has a significant impact on sleep quality and metabolic processes.
There are a few things to remember when using a scatter plot. Even when a scatter diagram indicates a relationship, it doesn’t mean one variable affects the other. A third factor can influence both variables. The more the plot resembles a straight line, the stronger the relationship is perceived [11] . If it suggests no ties, the observed pattern might be due to random fluctuations in data. When the scatter diagram depicts no correlation, whether the data might be stratified is worth considering.
Choosing the appropriate data presentation type is crucial when making a presentation . Understanding the nature of your data and the message you intend to convey will guide this selection process. For instance, when showcasing quantitative relationships, scatter plots become instrumental in revealing correlations between variables. If the focus is on emphasizing parts of a whole, pie charts offer a concise display of proportions. Histograms, on the other hand, prove valuable for illustrating distributions and frequency patterns.
Bar charts provide a clear visual comparison of different categories. Likewise, line charts excel in showcasing trends over time, while tables are ideal for detailed data examination. Starting a presentation on data presentation types involves evaluating the specific information you want to communicate and selecting the format that aligns with your message. This ensures clarity and resonance with your audience from the beginning of your presentation.
1. Fact Sheet Dashboard for Data Presentation
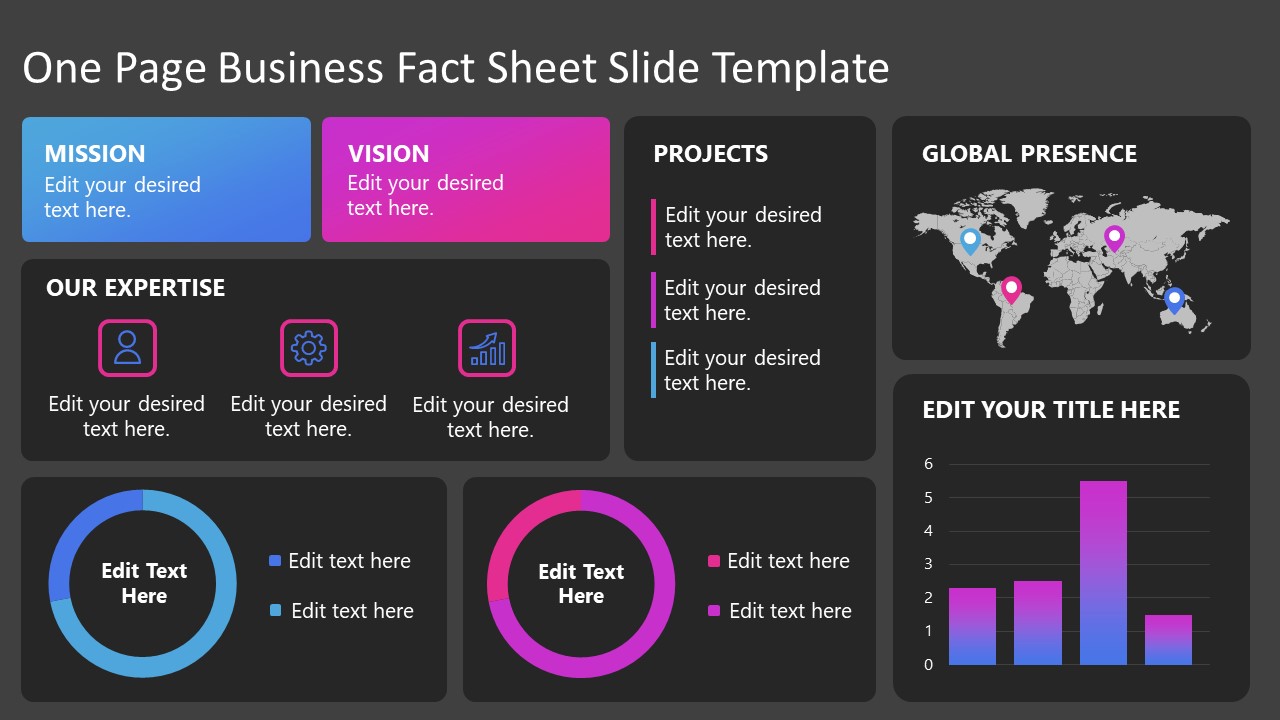
Convey all the data you need to present in this one-pager format, an ideal solution tailored for users looking for presentation aids. Global maps, donut chats, column graphs, and text neatly arranged in a clean layout presented in light and dark themes.
Use This Template
2. 3D Column Chart Infographic PPT Template
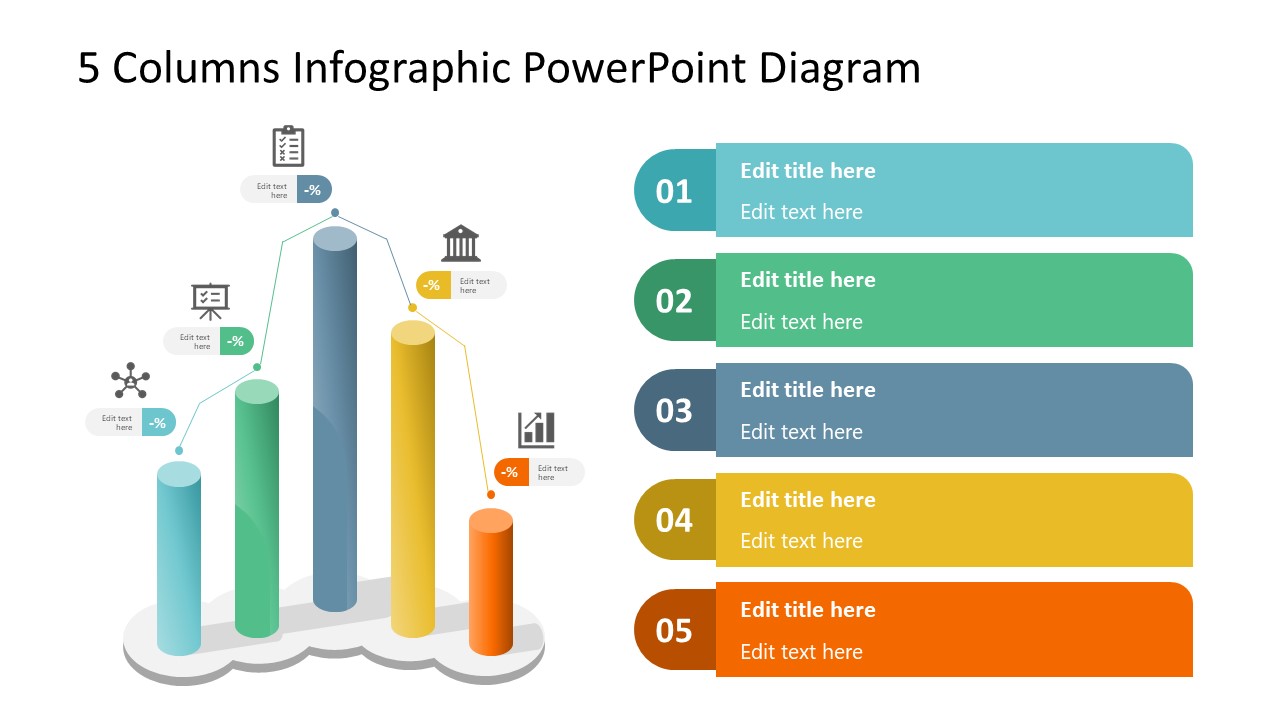
Represent column charts in a highly visual 3D format with this PPT template. A creative way to present data, this template is entirely editable, and we can craft either a one-page infographic or a series of slides explaining what we intend to disclose point by point.
3. Data Circles Infographic PowerPoint Template
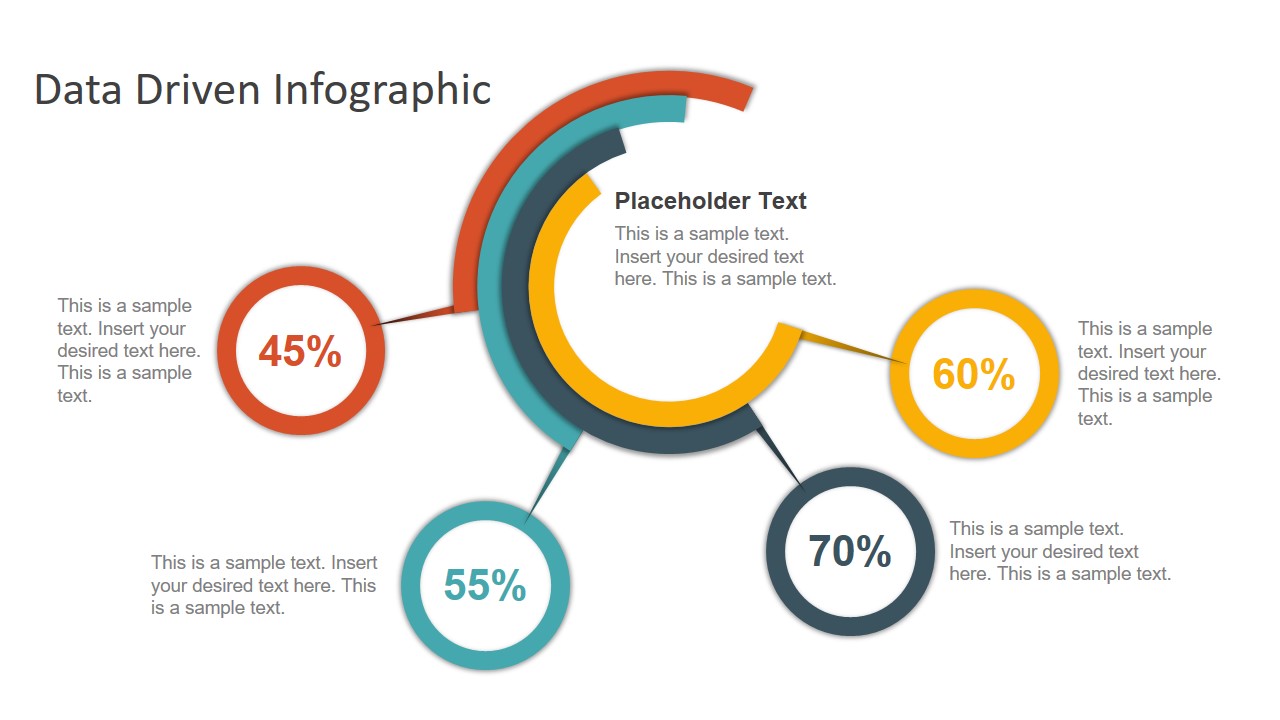
An alternative to the pie chart and donut chart diagrams, this template features a series of curved shapes with bubble callouts as ways of presenting data. Expand the information for each arch in the text placeholder areas.
4. Colorful Metrics Dashboard for Data Presentation
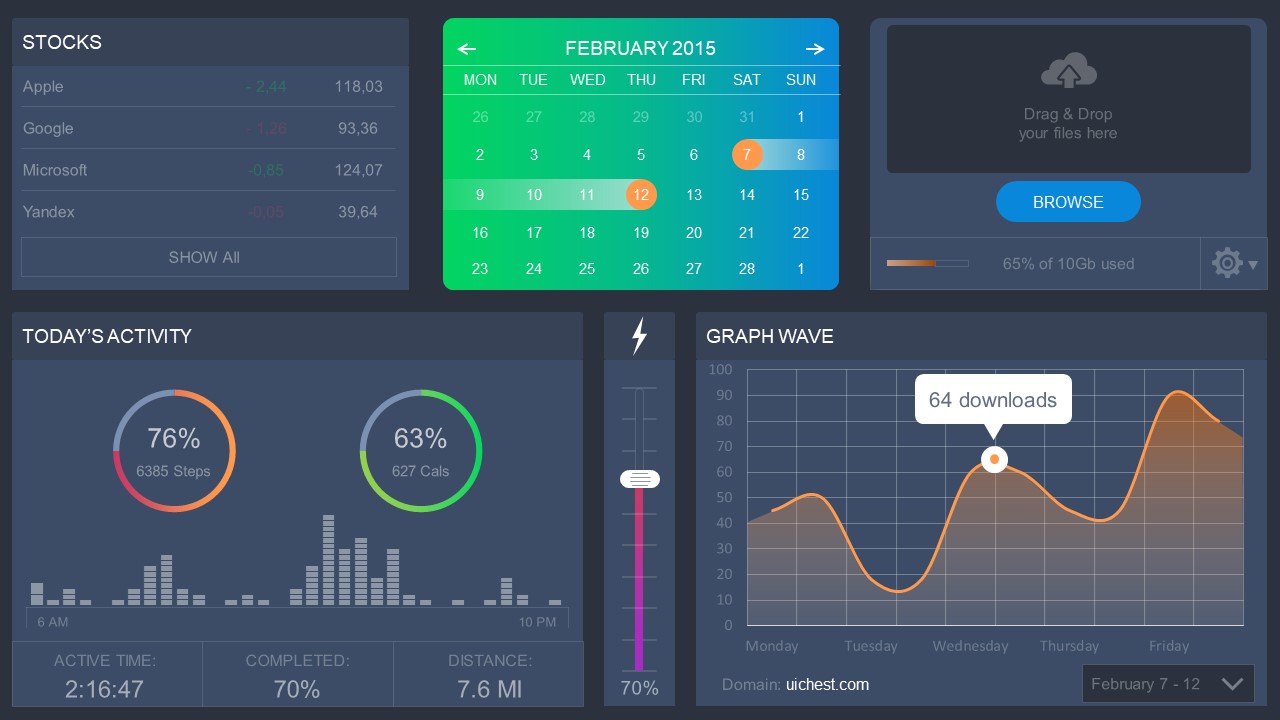
This versatile dashboard template helps us in the presentation of the data by offering several graphs and methods to convert numbers into graphics. Implement it for e-commerce projects, financial projections, project development, and more.
5. Animated Data Presentation Tools for PowerPoint & Google Slides
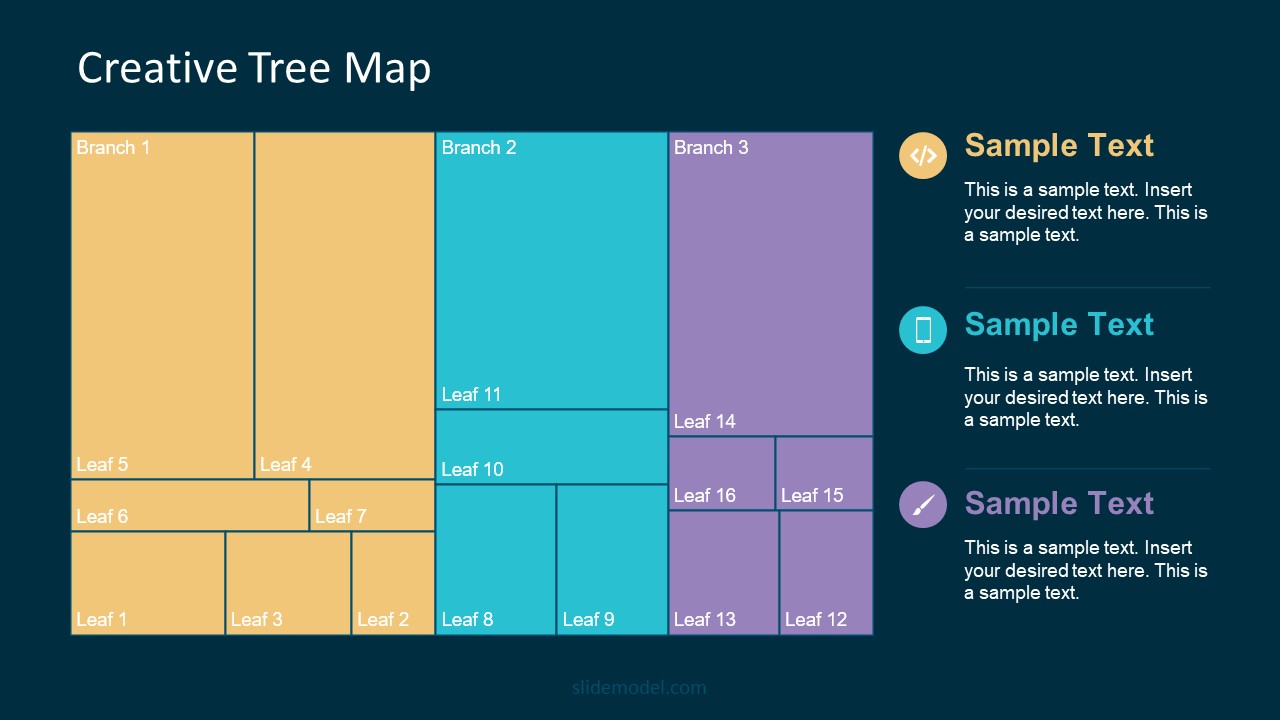
A slide deck filled with most of the tools mentioned in this article, from bar charts, column charts, treemap graphs, pie charts, histogram, etc. Animated effects make each slide look dynamic when sharing data with stakeholders.
6. Statistics Waffle Charts PPT Template for Data Presentations
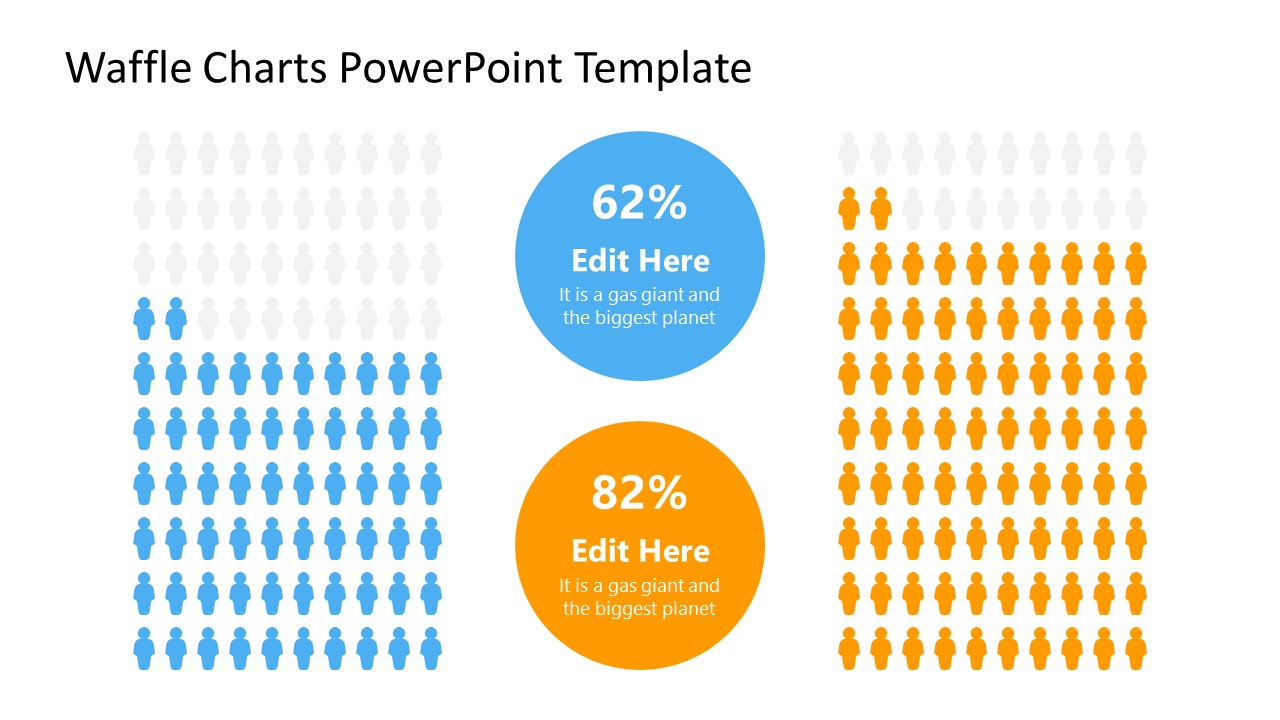
This PPT template helps us how to present data beyond the typical pie chart representation. It is widely used for demographics, so it’s a great fit for marketing teams, data science professionals, HR personnel, and more.
7. Data Presentation Dashboard Template for Google Slides
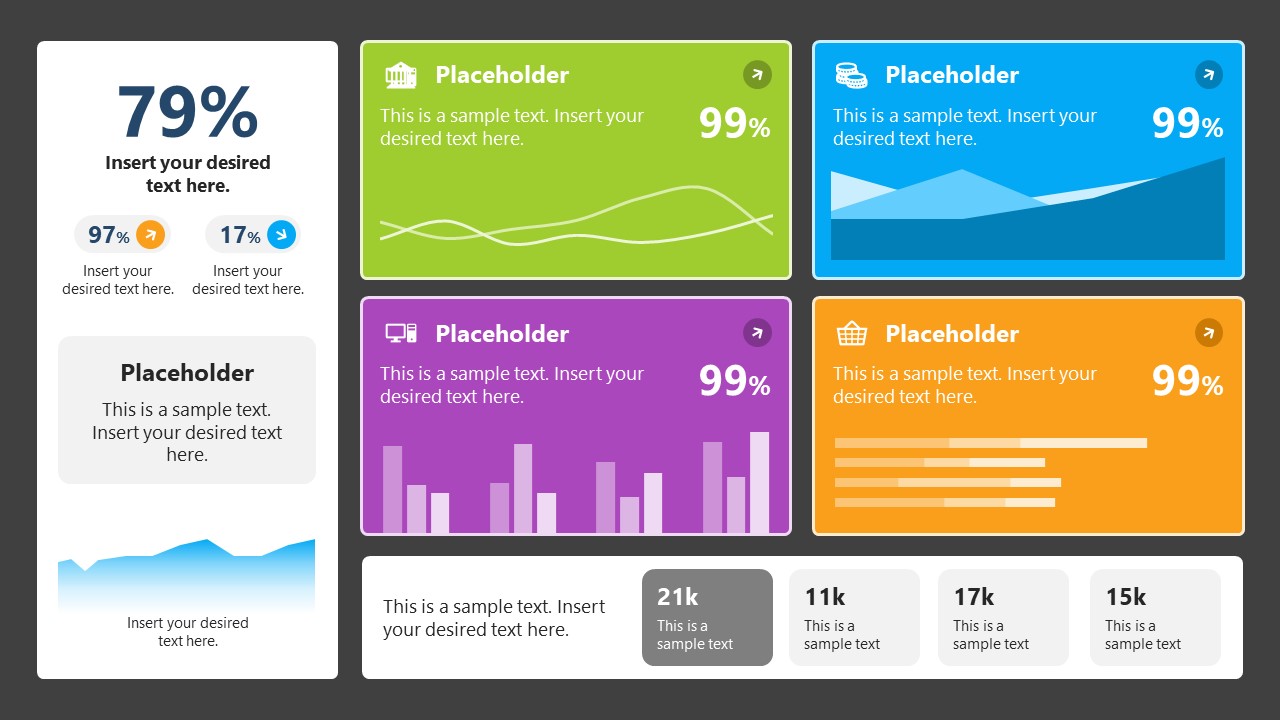
A compendium of tools in dashboard format featuring line graphs, bar charts, column charts, and neatly arranged placeholder text areas.
8. Weather Dashboard for Data Presentation
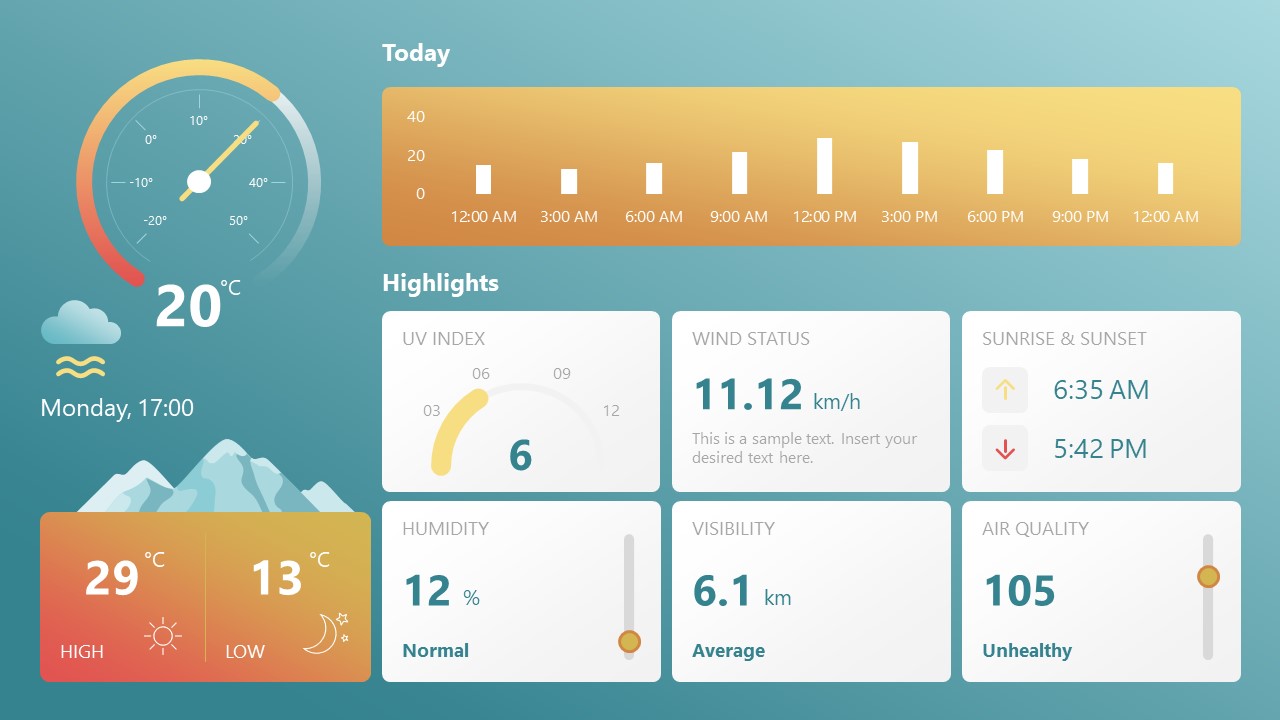
Share weather data for agricultural presentation topics, environmental studies, or any kind of presentation that requires a highly visual layout for weather forecasting on a single day. Two color themes are available.
9. Social Media Marketing Dashboard Data Presentation Template
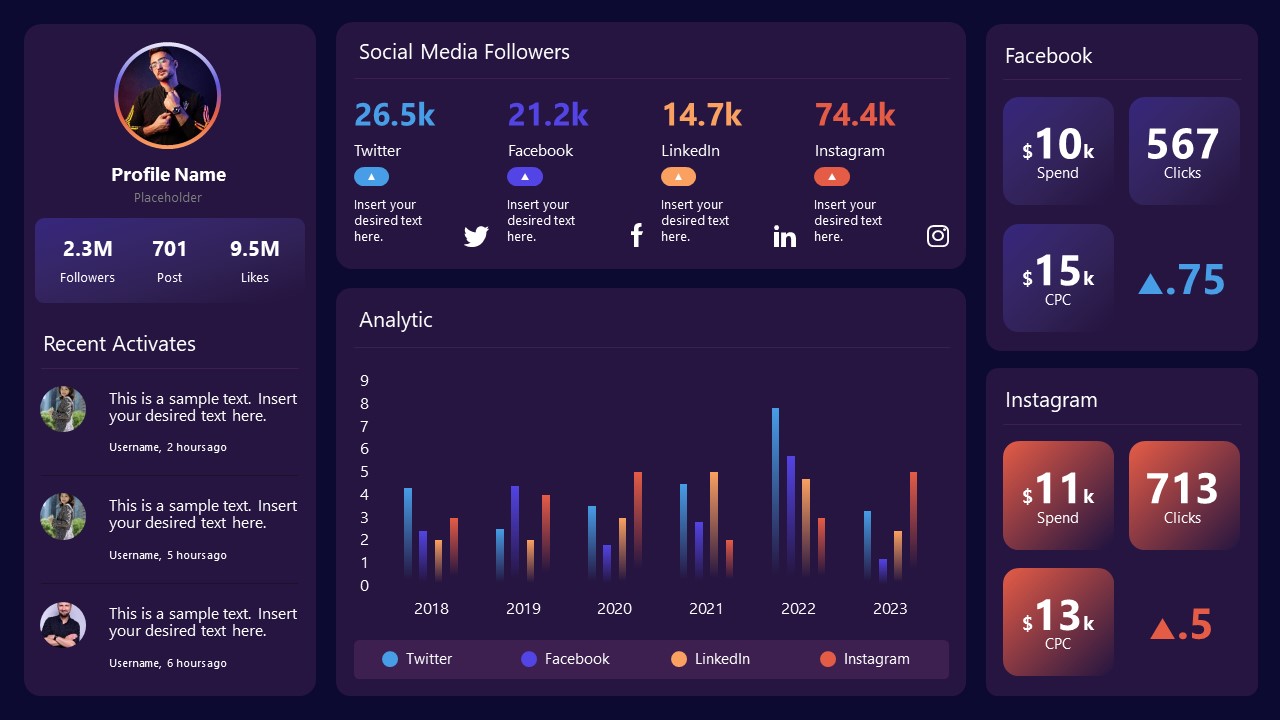
Intended for marketing professionals, this dashboard template for data presentation is a tool for presenting data analytics from social media channels. Two slide layouts featuring line graphs and column charts.
10. Project Management Summary Dashboard Template
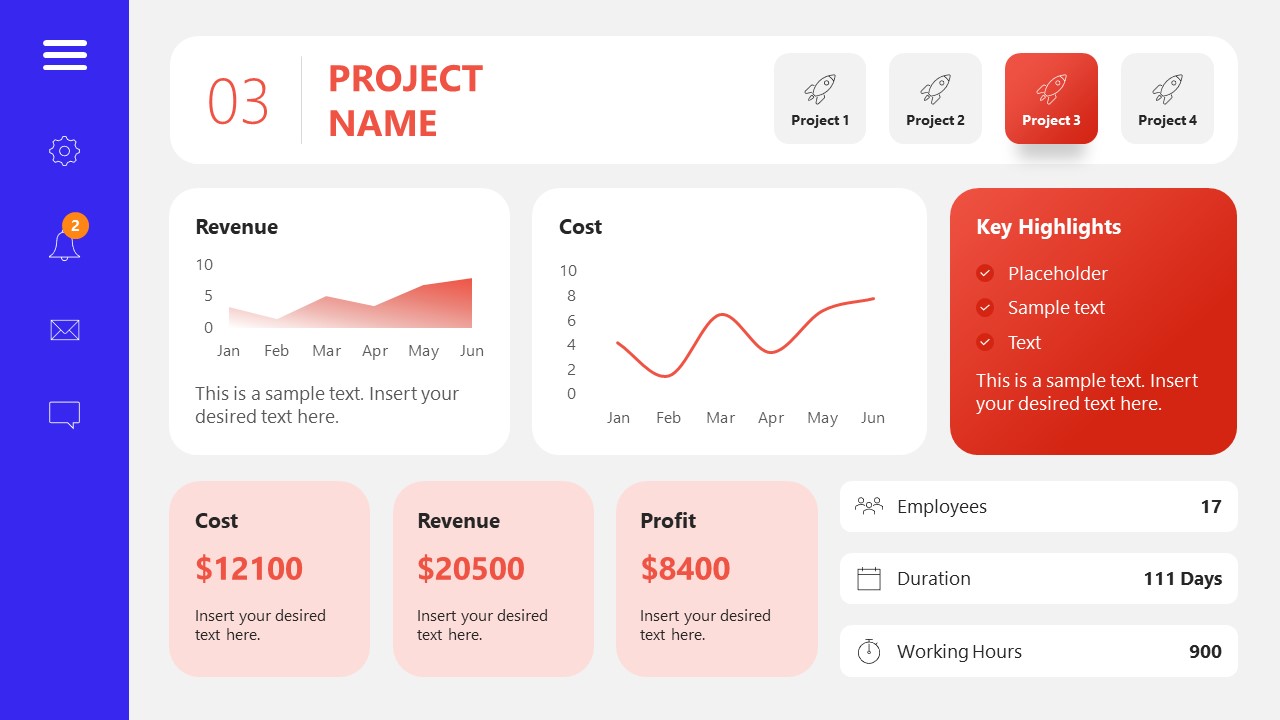
A tool crafted for project managers to deliver highly visual reports on a project’s completion, the profits it delivered for the company, and expenses/time required to execute it. 4 different color layouts are available.
11. Profit & Loss Dashboard for PowerPoint and Google Slides
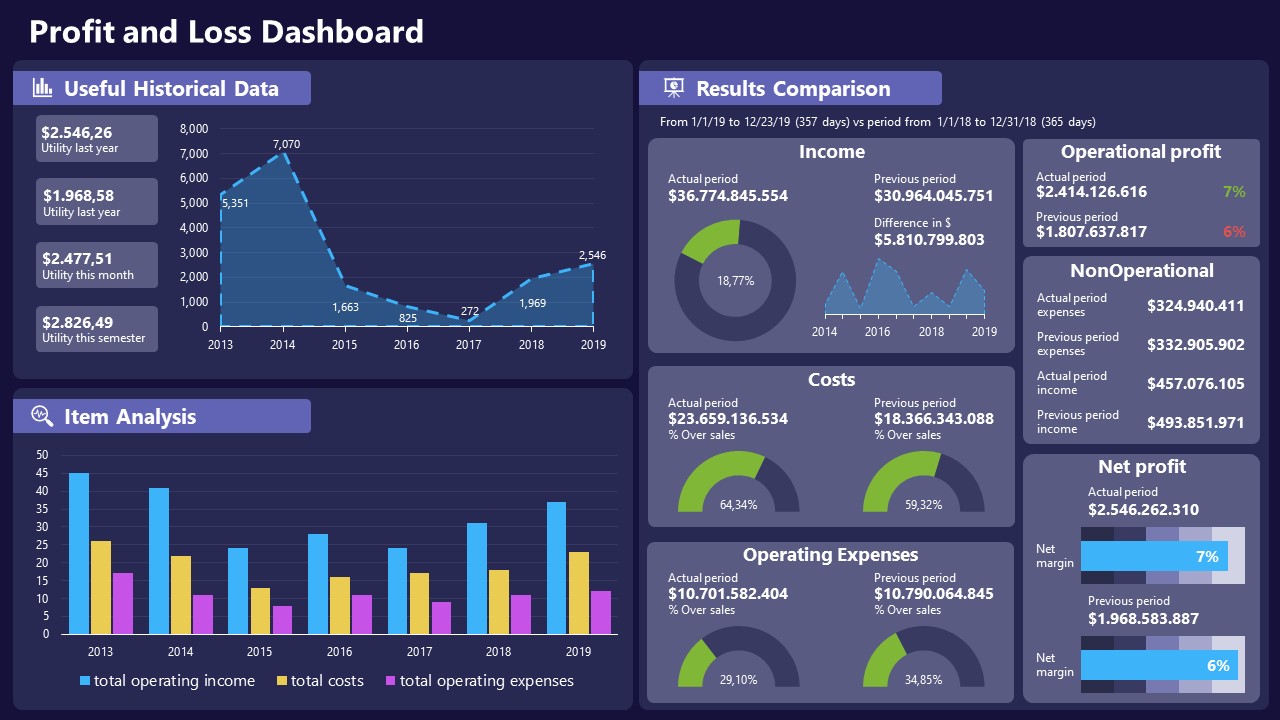
A must-have for finance professionals. This typical profit & loss dashboard includes progress bars, donut charts, column charts, line graphs, and everything that’s required to deliver a comprehensive report about a company’s financial situation.
Overwhelming visuals
One of the mistakes related to using data-presenting methods is including too much data or using overly complex visualizations. They can confuse the audience and dilute the key message.
Inappropriate chart types
Choosing the wrong type of chart for the data at hand can lead to misinterpretation. For example, using a pie chart for data that doesn’t represent parts of a whole is not right.
Lack of context
Failing to provide context or sufficient labeling can make it challenging for the audience to understand the significance of the presented data.
Inconsistency in design
Using inconsistent design elements and color schemes across different visualizations can create confusion and visual disarray.
Failure to provide details
Simply presenting raw data without offering clear insights or takeaways can leave the audience without a meaningful conclusion.
Lack of focus
Not having a clear focus on the key message or main takeaway can result in a presentation that lacks a central theme.
Visual accessibility issues
Overlooking the visual accessibility of charts and graphs can exclude certain audience members who may have difficulty interpreting visual information.
In order to avoid these mistakes in data presentation, presenters can benefit from using presentation templates . These templates provide a structured framework. They ensure consistency, clarity, and an aesthetically pleasing design, enhancing data communication’s overall impact.
Understanding and choosing data presentation types are pivotal in effective communication. Each method serves a unique purpose, so selecting the appropriate one depends on the nature of the data and the message to be conveyed. The diverse array of presentation types offers versatility in visually representing information, from bar charts showing values to pie charts illustrating proportions.
Using the proper method enhances clarity, engages the audience, and ensures that data sets are not just presented but comprehensively understood. By appreciating the strengths and limitations of different presentation types, communicators can tailor their approach to convey information accurately, developing a deeper connection between data and audience understanding.
If you need a quick method to create a data presentation, check out our AI presentation maker . A tool in which you add the topic, curate the outline, select a design, and let AI do the work for you.
[1] Government of Canada, S.C. (2021) 5 Data Visualization 5.2 Bar Chart , 5.2 Bar chart . https://www150.statcan.gc.ca/n1/edu/power-pouvoir/ch9/bargraph-diagrammeabarres/5214818-eng.htm
[2] Kosslyn, S.M., 1989. Understanding charts and graphs. Applied cognitive psychology, 3(3), pp.185-225. https://apps.dtic.mil/sti/pdfs/ADA183409.pdf
[3] Creating a Dashboard . https://it.tufts.edu/book/export/html/1870
[4] https://www.goldenwestcollege.edu/research/data-and-more/data-dashboards/index.html
[5] https://www.mit.edu/course/21/21.guide/grf-line.htm
[6] Jadeja, M. and Shah, K., 2015, January. Tree-Map: A Visualization Tool for Large Data. In GSB@ SIGIR (pp. 9-13). https://ceur-ws.org/Vol-1393/gsb15proceedings.pdf#page=15
[7] Heat Maps and Quilt Plots. https://www.publichealth.columbia.edu/research/population-health-methods/heat-maps-and-quilt-plots
[8] EIU QGIS WORKSHOP. https://www.eiu.edu/qgisworkshop/heatmaps.php
[9] About Pie Charts. https://www.mit.edu/~mbarker/formula1/f1help/11-ch-c8.htm
[10] Histograms. https://sites.utexas.edu/sos/guided/descriptive/numericaldd/descriptiven2/histogram/ [11] https://asq.org/quality-resources/scatter-diagram
Like this article? Please share
Data Analysis, Data Science, Data Visualization Filed under Design
Related Articles
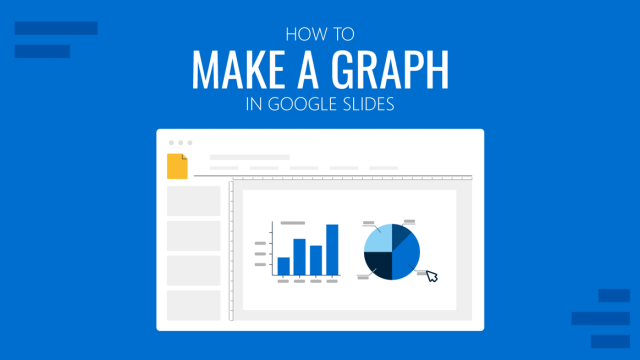
Filed under Google Slides Tutorials • June 3rd, 2024
How To Make a Graph on Google Slides
Creating quality graphics is an essential aspect of designing data presentations. Learn how to make a graph in Google Slides with this guide.
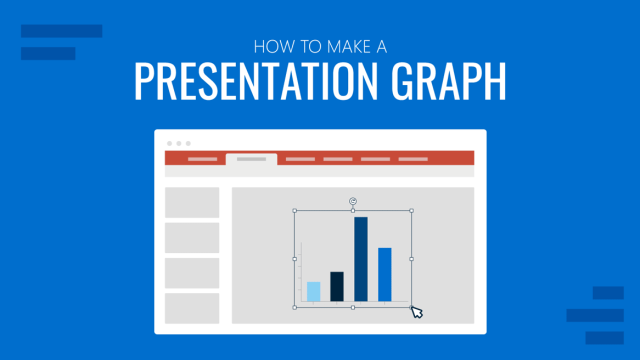
Filed under Design • March 27th, 2024
How to Make a Presentation Graph
Detailed step-by-step instructions to master the art of how to make a presentation graph in PowerPoint and Google Slides. Check it out!
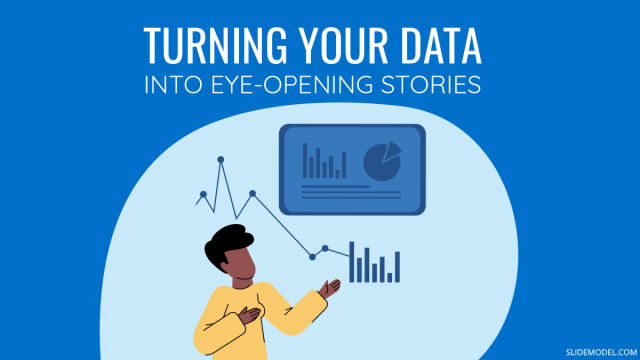
Filed under Presentation Ideas • February 12th, 2024
Turning Your Data into Eye-opening Stories
What is Data Storytelling is a question that people are constantly asking now. If you seek to understand how to create a data storytelling ppt that will complete the information for your audience, you should read this blog post.
Leave a Reply
What is Data Analysis? An Expert Guide With Examples
What is data analysis.
Data analysis is a comprehensive method of inspecting, cleansing, transforming, and modeling data to discover useful information, draw conclusions, and support decision-making. It is a multifaceted process involving various techniques and methodologies to interpret data from various sources in different formats, both structured and unstructured.
Data analysis is not just a mere process; it's a tool that empowers organizations to make informed decisions, predict trends, and improve operational efficiency. It's the backbone of strategic planning in businesses, governments, and other organizations.
Consider the example of a leading e-commerce company. Through data analysis, they can understand their customers' buying behavior, preferences, and patterns. They can then use this information to personalize customer experiences, forecast sales, and optimize marketing strategies, ultimately driving business growth and customer satisfaction.
Learn more about how to become a data analyst in our separate article, which covers everything you need to know about launching your career in this field and the skills you’ll need to master.
AI Upskilling for Beginners
The importance of data analysis in today's digital world.
In the era of digital transformation, data analysis has become more critical than ever. The explosion of data generated by digital technologies has led to the advent of what we now call 'big data.' This vast amount of data, if analyzed correctly, can provide invaluable insights that can revolutionize businesses.
Data analysis is the key to unlocking the potential of big data. It helps organizations to make sense of this data, turning it into actionable insights. These insights can be used to improve products and services, enhance experiences, streamline operations, and increase profitability.
A good example is the healthcare industry . Through data analysis, healthcare providers can predict disease outbreaks, improve patient care, and make informed decisions about treatment strategies. Similarly, in the finance sector, data analysis can help in risk assessment, fraud detection, and investment decision-making.
The Data Analysis Process: A Step-by-Step Guide
The process of data analysis is a systematic approach that involves several stages, each crucial to ensuring the accuracy and usefulness of the results. Here, we'll walk you through each step, from defining objectives to data storytelling. You can learn more about how businesses analyze data in a separate guide.

The data analysis process in a nutshell
Step 1: Defining objectives and questions
The first step in the data analysis process is to define the objectives and formulate clear, specific questions that your analysis aims to answer. This step is crucial as it sets the direction for the entire process. It involves understanding the problem or situation at hand, identifying the data needed to address it, and defining the metrics or indicators to measure the outcomes.
Step 2: Data collection
Once the objectives and questions are defined, the next step is to collect the relevant data. This can be done through various methods such as surveys, interviews, observations, or extracting from existing databases. The data collected can be quantitative (numerical) or qualitative (non-numerical), depending on the nature of the problem and the questions being asked.
Step 3: Data cleaning
Data cleaning, also known as data cleansing, is a critical step in the data analysis process. It involves checking the data for errors and inconsistencies, and correcting or removing them. This step ensures the quality and reliability of the data, which is crucial for obtaining accurate and meaningful results from the analysis.
Step 4: Data analysis
Once the data is cleaned, it's time for the actual analysis. This involves applying statistical or mathematical techniques to the data to discover patterns, relationships, or trends. There are various tools and software available for this purpose, such as Python, R, Excel, and specialized software like SPSS and SAS.
Step 5: Data interpretation and visualization
After the data is analyzed, the next step is to interpret the results and visualize them in a way that is easy to understand. This could involve creating charts, graphs, or other visual representations of the data. Data visualization helps to make complex data more understandable and provides a clear picture of the findings.
Step 6: Data storytelling
The final step in the data analysis process is data storytelling. This involves presenting the findings of the analysis in a narrative form that is engaging and easy to understand. Data storytelling is crucial for communicating the results to non-technical audiences and for making data-driven decisions.
The Types of Data Analysis
Data analysis can be categorized into four main types, each serving a unique purpose and providing different insights. These are descriptive, diagnostic, predictive, and prescriptive analyses.
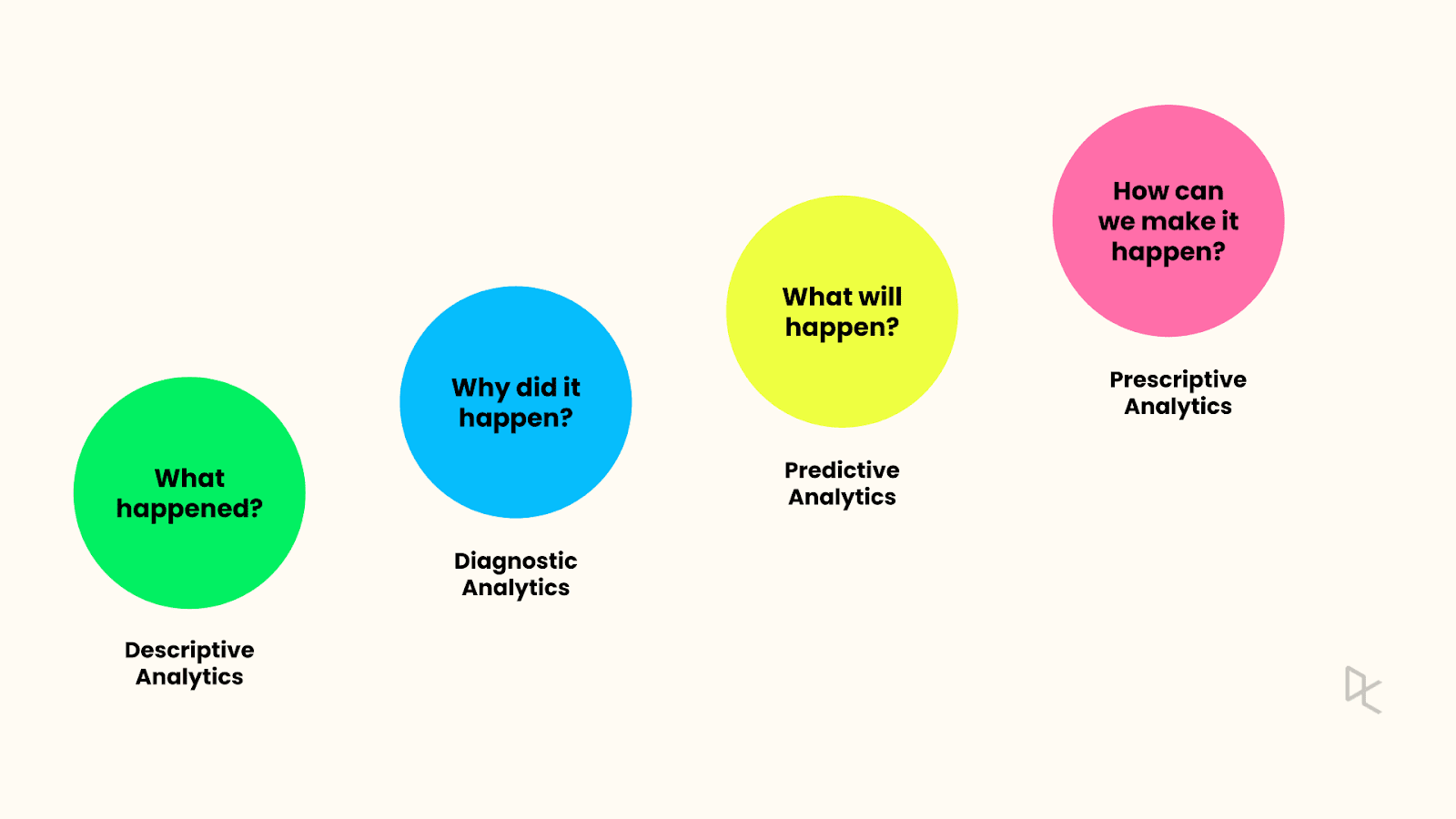
The four types of analytics
Descriptive analysis
Descriptive analysis , as the name suggests, describes or summarizes raw data and makes it interpretable. It involves analyzing historical data to understand what has happened in the past.
This type of analysis is used to identify patterns and trends over time.
For example, a business might use descriptive analysis to understand the average monthly sales for the past year.
Diagnostic analysis
Diagnostic analysis goes a step further than descriptive analysis by determining why something happened. It involves more detailed data exploration and comparing different data sets to understand the cause of a particular outcome.
For instance, if a company's sales dropped in a particular month, diagnostic analysis could be used to find out why.
Predictive analysis
Predictive analysis uses statistical models and forecasting techniques to understand the future. It involves using data from the past to predict what could happen in the future. This type of analysis is often used in risk assessment, marketing, and sales forecasting.
For example, a company might use predictive analysis to forecast the next quarter's sales based on historical data.
Prescriptive analysis
Prescriptive analysis is the most advanced type of data analysis. It not only predicts future outcomes but also suggests actions to benefit from these predictions. It uses sophisticated tools and technologies like machine learning and artificial intelligence to recommend decisions.
For example, a prescriptive analysis might suggest the best marketing strategies to increase future sales.
Data Analysis Techniques
There are numerous techniques used in data analysis, each with its unique purpose and application. Here, we will discuss some of the most commonly used techniques, including exploratory analysis, regression analysis, Monte Carlo simulation, factor analysis, cohort analysis, cluster analysis, time series analysis, and sentiment analysis.
Exploratory analysis
Exploratory analysis is used to understand the main characteristics of a data set. It is often used at the beginning of a data analysis process to summarize the main aspects of the data, check for missing data, and test assumptions. This technique involves visual methods such as scatter plots, histograms, and box plots.
You can learn more about exploratory data analysis with our course, covering how to explore, visualize, and extract insights from data using Python.
Regression analysis
Regression analysis is a statistical method used to understand the relationship between a dependent variable and one or more independent variables. It is commonly used for forecasting, time series modeling, and finding the causal effect relationships between variables.
We have a tutorial exploring the essentials of linear regression , which is one of the most widely used regression algorithms in areas like machine learning.
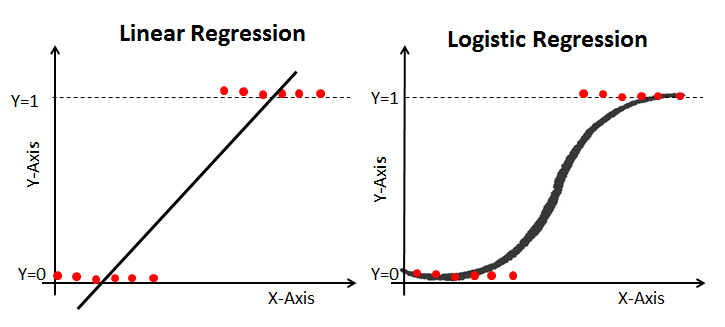
Linear and logistic regression
Factor analysis
Factor analysis is a technique used to reduce a large number of variables into fewer factors. The factors are constructed in such a way that they capture the maximum possible information from the original variables. This technique is often used in market research, customer segmentation, and image recognition.
Learn more about factor analysis in R with our course, which explores latent variables, such as personality, using exploratory and confirmatory factor analyses.
Monte Carlo simulation
Monte Carlo simulation is a technique that uses probability distributions and random sampling to estimate numerical results. It is often used in risk analysis and decision-making where there is significant uncertainty.
We have a tutorial that explores Monte Carlo methods in R , as well as a course on Monte Carlo simulations in Python , which can estimate a range of outcomes for uncertain events.
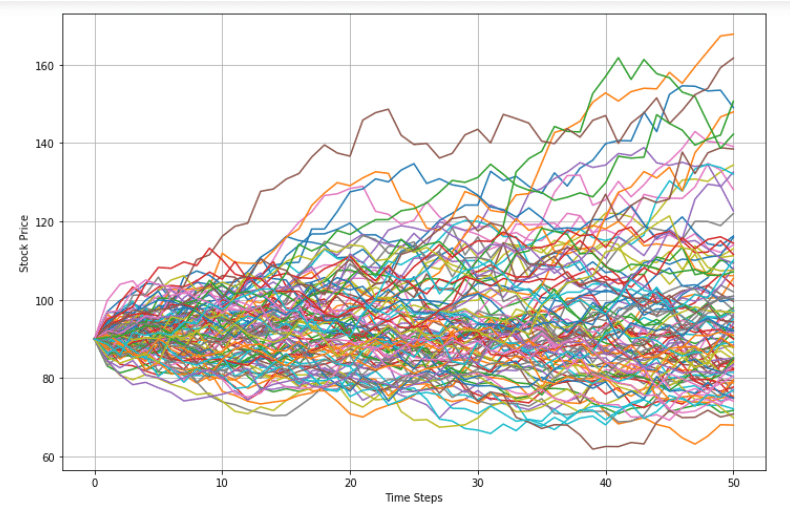
Example of a Monte Carlo simulation
Cluster analysis
Cluster analysis is a technique used to group a set of objects in such a way that objects in the same group (called a cluster) are more similar to each other than to those in other groups. It is often used in market segmentation, image segmentation, and recommendation systems.
You can explore a range of clustering techniques, including hierarchical clustering and k-means clustering, in our Cluster Analysis in R course.
Cohort analysis
Cohort analysis is a subset of behavioral analytics that takes data from a given dataset and groups it into related groups for analysis. These related groups, or cohorts, usually share common characteristics within a defined time span. This technique is often used in marketing, user engagement, and customer lifecycle analysis.
Our course, Customer Segmentation in Python , explores a range of techniques for segmenting and analyzing customer data, including cohort analysis.
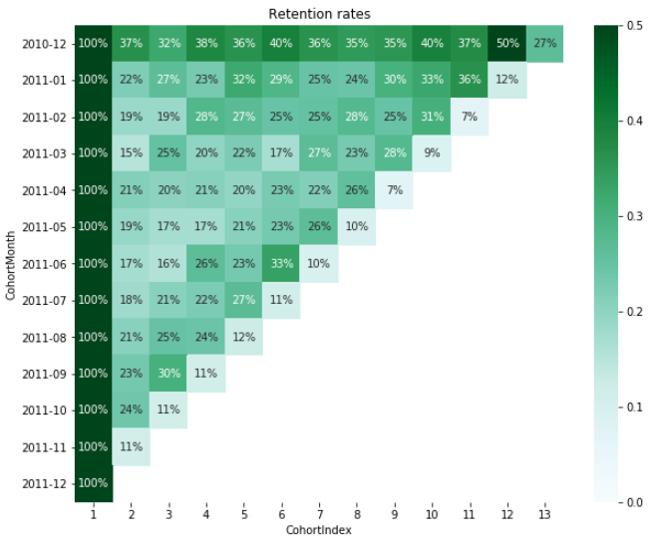
Graph showing an example of cohort analysis
Time series analysis
Time series analysis is a statistical technique that deals with time series data, or trend analysis. It is used to analyze the sequence of data points to extract meaningful statistics and other characteristics of the data. This technique is often used in sales forecasting, economic forecasting, and weather forecasting.
Our Time Series with Python skill track takes you through how to manipulate and analyze time series data, working with a variety of Python libraries.
Sentiment analysis
Sentiment analysis, also known as opinion mining, uses natural language processing, text analysis, and computational linguistics to identify and extract subjective information from source materials. It is often used in social media monitoring, brand monitoring, and understanding customer feedback.
To get familiar with sentiment analysis in Python , you can take our online course, which will teach you how to perform an end-to-end sentiment analysis.
Data Analysis Tools
In the realm of data analysis, various tools are available that cater to different needs, complexities, and levels of expertise. These tools range from programming languages like Python and R to visualization software like Power BI and Tableau. Let's delve into some of these tools.
Python is a high-level, general-purpose programming language that has become a favorite among data analysts and data scientists. Its simplicity and readability, coupled with a wide range of libraries like pandas , NumPy , and Matplotlib , make it an excellent tool for data analysis and data visualization.
" dir="ltr">Resources to get you started
- You can start learning Python today with our Python Fundamentals skill track, which covers all the foundational skills you need to understand the language.
- You can also take out Data Analyst with Python career track to start your journey to becoming a data analyst.
- Check out our Python for beginners cheat sheet as a handy reference guide.
R is a programming language and free software environment specifically designed for statistical computing and graphics. It is widely used among statisticians and data miners for developing statistical software and data analysis. R provides a wide variety of statistical and graphical techniques, including linear and nonlinear modeling, classical statistical tests, time-series analysis, and more.
- Our R Programming skill track will introduce you to R and help you develop the skills you’ll need to start coding in R.
- With the Data Analyst with R career track, you’ll gain the skills you need to start your journey to becoming a data analyst.
- Our Getting Started with R cheat sheet helps give an overview of how to start learning R Programming.
SQL (Structured Query Language) is a standard language for managing and manipulating databases. It is used to retrieve and manipulate data stored in relational databases. SQL is essential for tasks that involve data management or manipulation within databases.
- To get familiar with SQL, consider taking our SQL Fundamentals skill track, where you’ll learn how to interact with and query your data.
- SQL for Business Analysts will boost your business SQL skills.
- Our SQL Basics cheat sheet covers a list of functions for querying data, filtering data, aggregation, and more.
Power BI is a business analytics tool developed by Microsoft. It provides interactive visualizations with self-service business intelligence capabilities. Power BI is used to transform raw data into meaningful insights through easy-to-understand dashboards and reports.
- Explore the power of Power BI with our Power BI Fundamentals skill track, where you’ll learn to get the most from the business intelligence tool.
- With Exploratory Data Analysis in Power BI you’ll learn how to enhance your reports with EDA.
- We have a Power BI cheat sheet which covers many of the basics you’ll need to get started.
Tableau is a powerful data visualization tool used in the Business Intelligence industry. It allows you to create interactive and shareable dashboards, which depict trends, variations, and density of the data in the form of charts and graphs.
- The Tableau Fundamentals skill track will introduce you to the business intelligence tool and how you can use it to clear, analyze, and visualize data.
- Analyzing Data in Tableau will give you some of the advanced skills needed to improve your analytics and visualizations.
- Check out our Tableau cheat sheet , which runs you through the essentials of how to get started using the tool.
Microsoft Excel is one of the most widely used tools for data analysis. It offers a range of features for data manipulation, statistical analysis, and visualization. Excel's simplicity and versatility make it a great tool for both simple and complex data analysis tasks.
- Check out our Data Analysis in Excel course to build functional skills in Excel.
- For spreadsheet skills in general, check out Marketing Analytics in Spreadsheets .
- The Excel Basics cheat sheet covers many of the basic formulas and operations you’ll need to make a start.
Understanding the Impact of Data Analysis
Data analysis, whether on a small or large scale, can have a profound impact on business performance. It can drive significant changes, leading to improved efficiency, increased profitability, and a deeper understanding of market trends and customer behavior.
Informed decision-making
Data analysis allows businesses to make informed decisions based on facts, figures, and trends, rather than relying on guesswork or intuition. It provides a solid foundation for strategic planning and policy-making, ensuring that resources are allocated effectively and that efforts are directed towards areas that will yield the most benefit.
Impact on small businesses
For small businesses, even simple data analysis can lead to significant improvements. For example, analyzing sales data can help identify which products are performing well and which are not. This information can then be used to adjust marketing strategies, pricing, and inventory management, leading to increased sales and profitability.
Impact on large businesses
For larger businesses, the impact of data analysis can be even more profound. Big data analysis can uncover complex patterns and trends that would be impossible to detect otherwise. This can lead to breakthrough insights, driving innovation and giving the business a competitive edge.
For example, a large retailer might use data analysis to optimize its supply chain, reducing costs and improving efficiency. Or a tech company might use data analysis to understand user behavior, leading to improved product design and better user engagement.
The critical role of data analysis
In today's data-driven world, the ability to analyze and interpret data is a critical skill. Businesses that can harness the power of data analysis are better positioned to adapt to changing market conditions, meet customer needs, and drive growth and profitability.
Get started with DataCamp for Business
Build a data-driven workforce with DataCamp for business
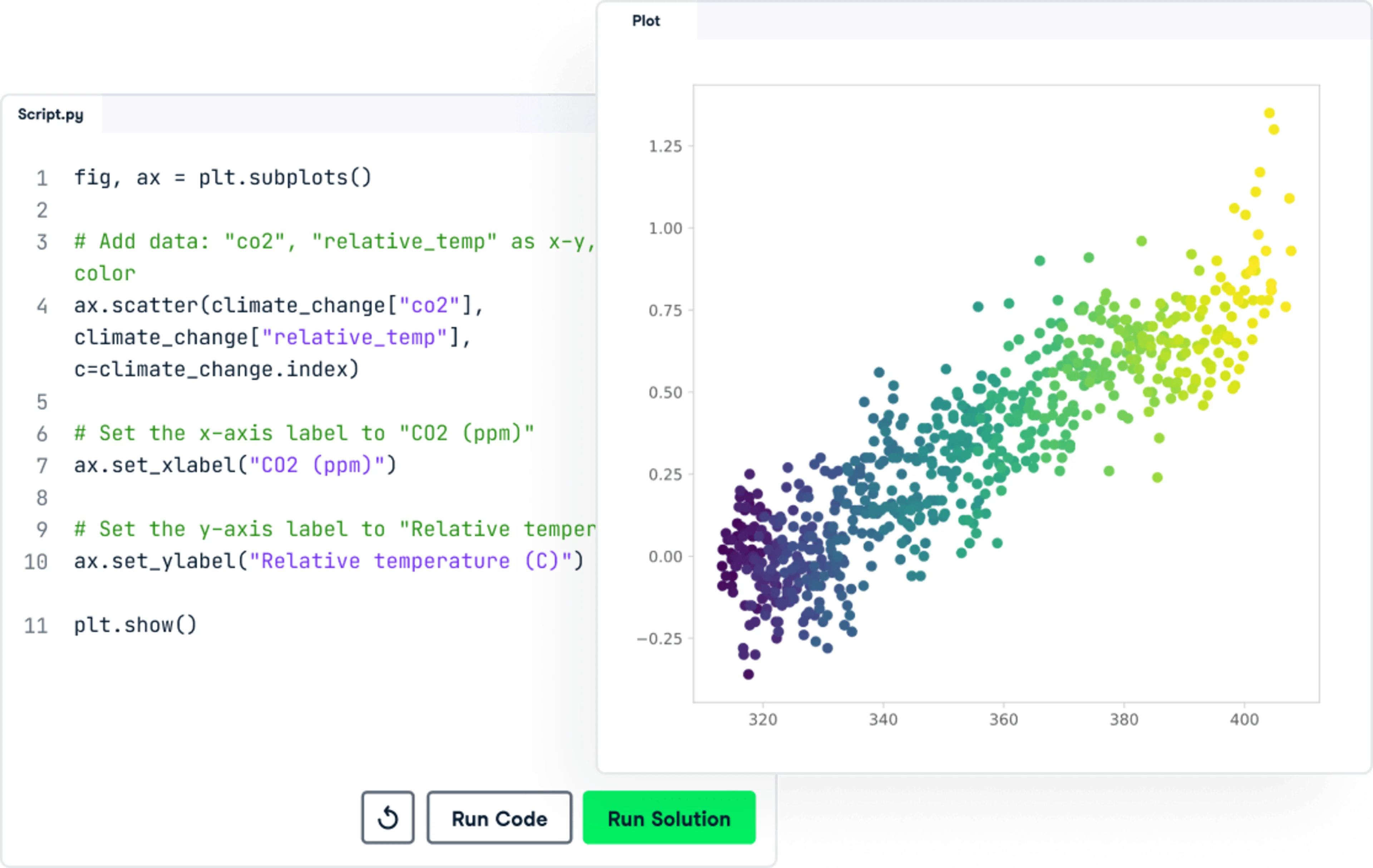
Top Careers in Data Analysis in 2023
In the era of Big Data, careers in data analysis are flourishing. With the increasing demand for data-driven insights, these professions offer promising prospects. Here, we will discuss some of the top careers in data analysis in 2023, referring to our full guide on the top ten analytics careers .
1. Data scientist
Data scientists are the detectives of the data world, uncovering patterns, insights, and trends from vast amounts of information. They use a combination of programming, statistical skills, and machine learning to make sense of complex data sets. Data scientists not only analyze data but also use their insights to influence strategic decisions within their organization.
We’ve got a complete guide on how to become a data scientist , which outlines everything you need to know about starting your career in the industry.
Key skills :
- Proficiency in programming languages like Python or R
- Strong knowledge of statistics and probability
- Familiarity with machine learning algorithms
- Data wrangling and data cleaning skills
- Ability to communicate complex data insights in a clear and understandable manner
Essential tools :
- Jupyter Notebook
- Machine learning libraries like Scikit-learn, TensorFlow
- Data visualization libraries like Matplotlib, Seaborn
2. Business intelligence analyst
Business intelligence analysts are responsible for providing a clear picture of a business's performance by analyzing data related to market trends, business processes, and industry competition. They use tools and software to convert complex data into digestible reports and dashboards, helping decision-makers to understand the business's position and make informed decisions.
- Strong analytical skills
- Proficiency in SQL and other database technologies
- Understanding of data warehousing and ETL processes
- Ability to create clear visualizations and reports
- Business acumen
- Power BI, Tableau
3. Data engineer
Data engineers are the builders and maintainers of the data pipeline. They design, construct, install, test, and maintain highly scalable data management systems. They also ensure that data is clean, reliable, and preprocessed for data scientists to perform analysis.
Read more about what a data engineer does and how you can become a data engineer in our separate guide.
- Proficiency in SQL and NoSQL databases
- Knowledge of distributed systems and data architecture
- Familiarity with ETL tools and processes
- Programming skills, particularly in Python and Java
- Understanding of machine learning algorithms
- Hadoop, Spark
- Python, Java
4. Business analyst
Business analysts are the bridge between IT and business stakeholders. They use data to assess processes, determine requirements, and deliver data-driven recommendations and reports to executives and stakeholders. They are involved in strategic planning, business model analysis, process design, and system analysis.
- Understanding of business processes and strategies
- Proficiency in SQL
- Ability to communicate effectively with both IT and business stakeholders
- Project management skills
|
|
|
| Proficiency in programming, strong statistical knowledge, familiarity with machine learning, data wrangling skills, and effective communication. | Python, R, SQL, Scikit-learn, TensorFlow, Matplotlib, Seaborn |
| Strong analytical skills, proficiency in SQL, understanding of data warehousing and ETL, ability to create visualizations and reports, and business acumen. | SQL, Power BI, Tableau, Excel, Python |
| Proficiency in SQL and NoSQL, knowledge of distributed systems and data architecture, familiarity with ETL, programming skills, and understanding of machine learning. | SQL, NoSQL, Hadoop, Spark, Python, Java, ETL tools |
| Strong analytical skills, understanding of business processes, proficiency in SQL, effective communication, and project management skills. | SQL, Excel,Power BI, Tableau, Python |
A table outlining different data analysis careers
How to Get Started with Data Analysis
Embarking on your journey into data analysis might seem daunting at first, but with the right resources and guidance, you can develop the necessary skills and knowledge. Here are some steps to help you get started, focusing on the resources available at DataCamp.
.css-138yw8m{-webkit-align-self:start;-ms-flex-item-align:start;align-self:start;-webkit-flex-shrink:0;-ms-flex-negative:0;flex-shrink:0;width:-webkit-max-content;width:-moz-max-content;width:max-content;} .css-b8zm5p{box-sizing:border-box;margin:0;min-width:0;-webkit-align-self:start;-ms-flex-item-align:start;align-self:start;-webkit-flex-shrink:0;-ms-flex-negative:0;flex-shrink:0;width:-webkit-max-content;width:-moz-max-content;width:max-content;} .css-i98n7q{-webkit-flex-shrink:0;-ms-flex-negative:0;flex-shrink:0;margin-top:5px;}.css-i98n7q .quote-new_svg__quote{fill:#7933ff;} .css-xfsibo{-webkit-align-self:center;-ms-flex-item-align:center;align-self:center;color:#000820;-webkit-flex-direction:column;-ms-flex-direction:column;flex-direction:column;-webkit-box-flex:1;-webkit-flex-grow:1;-ms-flex-positive:1;flex-grow:1;-webkit-box-pack:space-evenly;-ms-flex-pack:space-evenly;-webkit-justify-content:space-evenly;justify-content:space-evenly;margin-left:16px;} .css-gt3aw7{display:-webkit-box;display:-webkit-flex;display:-ms-flexbox;display:flex;-webkit-align-self:center;-ms-flex-item-align:center;align-self:center;color:#000820;-webkit-flex-direction:column;-ms-flex-direction:column;flex-direction:column;-webkit-box-flex:1;-webkit-flex-grow:1;-ms-flex-positive:1;flex-grow:1;-webkit-box-pack:space-evenly;-ms-flex-pack:space-evenly;-webkit-justify-content:space-evenly;justify-content:space-evenly;margin-left:16px;} .css-n2j7xo{box-sizing:border-box;margin:0;min-width:0;display:-webkit-box;display:-webkit-flex;display:-ms-flexbox;display:flex;-webkit-align-self:center;-ms-flex-item-align:center;align-self:center;color:#000820;-webkit-flex-direction:column;-ms-flex-direction:column;flex-direction:column;-webkit-box-flex:1;-webkit-flex-grow:1;-ms-flex-positive:1;flex-grow:1;-webkit-box-pack:space-evenly;-ms-flex-pack:space-evenly;-webkit-justify-content:space-evenly;justify-content:space-evenly;margin-left:16px;} .css-1yf55a1{margin-bottom:8px;}.css-1yf55a1 a{color:#05192d;font-weight:700;line-height:1.5;-webkit-text-decoration:none;text-decoration:none;}.css-1yf55a1 a:active,.css-1yf55a1 a:focus,.css-1yf55a1 a:hover{-webkit-text-decoration:underline;text-decoration:underline;}.css-1yf55a1 p{font-size:16px;font-weight:800;line-height:24px;} .css-xjjmwi{box-sizing:border-box;margin:0;min-width:0;font-size:1.5rem;letter-spacing:-0.5px;line-height:1.2;margin-top:0;margin-bottom:8px;}.css-xjjmwi a{color:#05192d;font-weight:700;line-height:1.5;-webkit-text-decoration:none;text-decoration:none;}.css-xjjmwi a:active,.css-xjjmwi a:focus,.css-xjjmwi a:hover{-webkit-text-decoration:underline;text-decoration:underline;}.css-xjjmwi p{font-size:16px;font-weight:800;line-height:24px;} To thrive in data analysis, you must build a strong foundation of knowledge, sharpen practical skills, and accumulate valuable experience. Start with statistics, mathematics, and programming and tackle real-world projects. Then, gain domain expertise, and connect with professionals in the field. Combine expertise, skills, and experience for a successful data analysis career. .css-16mqoqa{color:#626D79;font-weight:400;} .css-1k1umiz{box-sizing:border-box;margin:0;min-width:0;font-size:0.875rem;line-height:1.5;margin-top:0;color:#626D79;font-weight:400;} Richie Cotton , Data Evangelist at DataCamp
Understand the basics
Before diving into data analysis, it's important to understand the basics. This includes familiarizing yourself with statistical concepts, data types, and data structures. DataCamp's Introduction to Data Science in Python or Introduction to Data Science in R courses are great starting points.
Learn a programming language
Data analysis requires proficiency in at least one programming language. Python and R are among the most popular choices due to their versatility and the vast array of libraries they offer for data analysis. We offer comprehensive learning paths for both Python and R .
Master data manipulation and visualization
Data manipulation and visualization are key components of data analysis. They allow you to clean, transform, and visualize your data, making it easier to understand and analyze. Courses like Data Manipulation with pandas or Data Visualization with ggplot2 can help you develop these skills.
Dive into Specific Data Analysis Techniques
Once you've mastered the basics, you can delve into specific data analysis techniques like regression analysis , time series analysis , or machine learning . We offer a wide range of courses across many topics, allowing you to specialize based on your interests and career goals.
Practice, Practice, Practice
The key to mastering data analysis is practice. DataCamp's practice mode and projects provide hands-on experience with real-world data, helping you consolidate your learning and apply your skills. You can find a list of 20 data analytics projects for all levels to give you some inspiration.
Remember, learning data analysis is a journey. It's okay to start small and gradually build up your skills over time. With patience, persistence, and the right resources, you'll be well on your way to becoming a proficient data analyst.
Become a ML Scientist
Final thoughts.
In the era of digital transformation, data analysis has emerged as a crucial skill, regardless of your field or industry. The ability to make sense of data, to extract insights, and to use those insights to make informed decisions can give you a significant advantage in today's data-driven world.
Whether you're a marketer looking to understand customer behavior, a healthcare professional aiming to improve patient outcomes, or a business leader seeking to drive growth and profitability, data analysis can provide the insights you need to succeed.
Remember, data analysis is not just about numbers and statistics. It's about asking the right questions, being curious about patterns and trends, and having the courage to make data-driven decisions. It's about telling a story with data, a story that can influence strategies, change perspectives, and drive innovation.
So, we encourage you to apply your understanding of data analysis in your respective fields. Harness the power of data to uncover insights, make informed decisions, and drive success. The world of data is at your fingertips, waiting to be explored.
Data Analyst with Python
.css-1531qan{-webkit-text-decoration:none;text-decoration:none;color:inherit;} data analyst, what is data analysis .css-18x2vi3{-webkit-flex-shrink:0;-ms-flex-negative:0;flex-shrink:0;height:18px;padding-top:6px;-webkit-transform:rotate(0.5turn) translate(21%, -10%);-moz-transform:rotate(0.5turn) translate(21%, -10%);-ms-transform:rotate(0.5turn) translate(21%, -10%);transform:rotate(0.5turn) translate(21%, -10%);-webkit-transition:-webkit-transform 0.3s cubic-bezier(0.85, 0, 0.15, 1);transition:transform 0.3s cubic-bezier(0.85, 0, 0.15, 1);width:18px;}.
Data analysis is a comprehensive method that involves inspecting, cleansing, transforming, and modeling data to discover useful information, make conclusions, and support decision-making. It's a process that empowers organizations to make informed decisions, predict trends, and improve operational efficiency.
What are the steps in the data analysis process? .css-167dpqb{-webkit-flex-shrink:0;-ms-flex-negative:0;flex-shrink:0;height:18px;padding-top:6px;-webkit-transform:none;-moz-transform:none;-ms-transform:none;transform:none;-webkit-transition:-webkit-transform 0.3s cubic-bezier(0.85, 0, 0.15, 1);transition:transform 0.3s cubic-bezier(0.85, 0, 0.15, 1);width:18px;}
The data analysis process involves several steps, including defining objectives and questions, data collection, data cleaning, data analysis, data interpretation and visualization, and data storytelling. Each step is crucial to ensuring the accuracy and usefulness of the results.
What are the different types of data analysis?
Data analysis can be categorized into four types: descriptive, diagnostic, predictive, and prescriptive analysis. Descriptive analysis summarizes raw data, diagnostic analysis determines why something happened, predictive analysis uses past data to predict the future, and prescriptive analysis suggests actions based on predictions.
What are some commonly used data analysis techniques?
There are various data analysis techniques, including exploratory analysis, regression analysis, Monte Carlo simulation, factor analysis, cohort analysis, cluster analysis, time series analysis, and sentiment analysis. Each has its unique purpose and application in interpreting data.
What are some of the tools used in data analysis?
Data analysis typically utilizes tools such as Python, R, SQL for programming, and Power BI, Tableau, and Excel for visualization and data management.
How can I start learning data analysis?
You can start learning data analysis by understanding the basics of statistical concepts, data types, and structures. Then learn a programming language like Python or R, master data manipulation and visualization, and delve into specific data analysis techniques.
How can I become a data analyst?
Becoming a Data Analyst requires a strong understanding of statistical techniques and data analysis tools. Mastery of software such as Python, R, Excel, and specialized software like SPSS and SAS is typically necessary. Read our full guide on how to become a Data Analyst and consider our Data Analyst Certification to get noticed by recruiters.
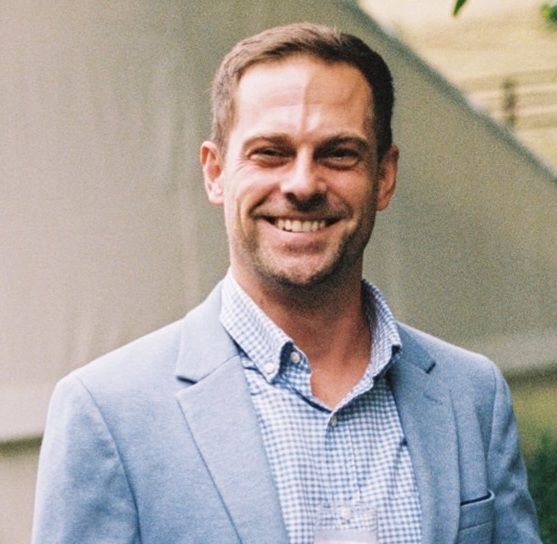
A writer and content editor in the edtech space. Committed to exploring data trends and enthusiastic about learning data science.
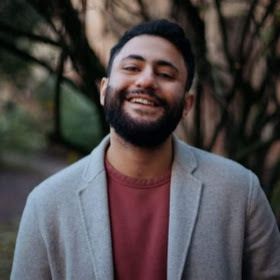
Adel is a Data Science educator, speaker, and Evangelist at DataCamp where he has released various courses and live training on data analysis, machine learning, and data engineering. He is passionate about spreading data skills and data literacy throughout organizations and the intersection of technology and society. He has an MSc in Data Science and Business Analytics. In his free time, you can find him hanging out with his cat Louis.
What is Business Analytics? Everything You Need to Know

Joleen Bothma
How to Analyze Data For Your Business in 5 Steps
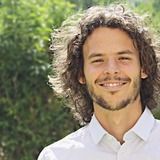
Javier Canales Luna
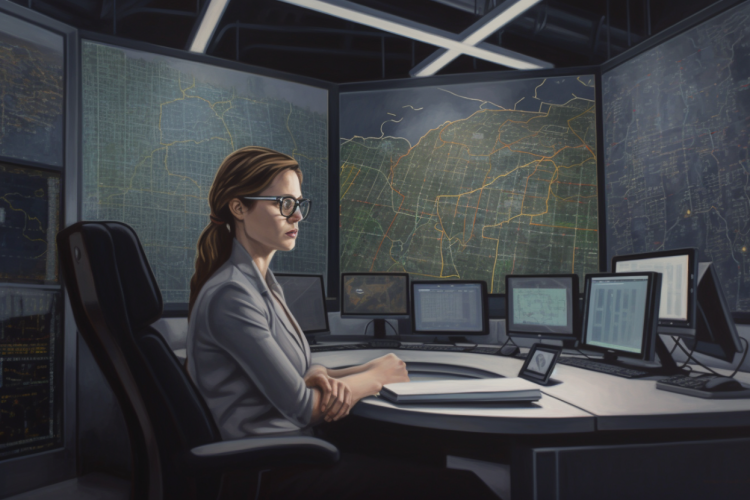
What is Data Science? Definition, Examples, Tools & More
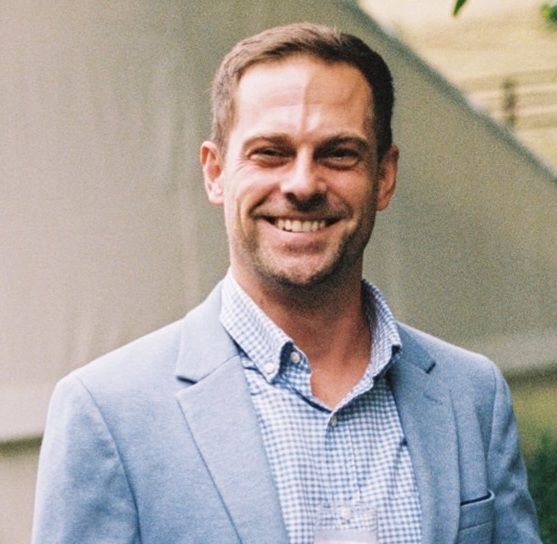
Matt Crabtree
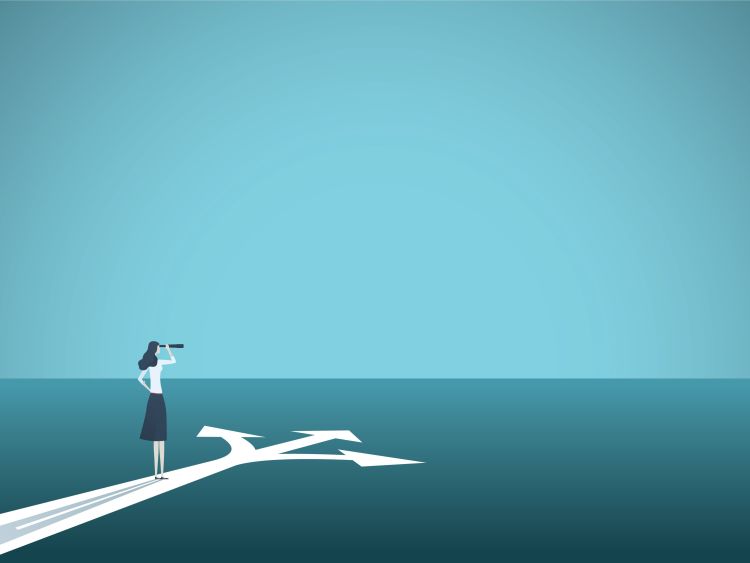
Data Analyst vs. Data Scientist: A Comparative Guide For 2024
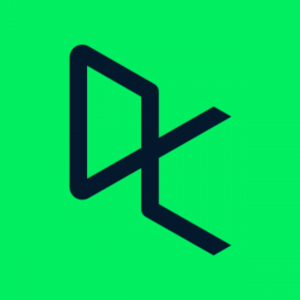
DataCamp Team
A Beginner's Guide to Predictive Analytics
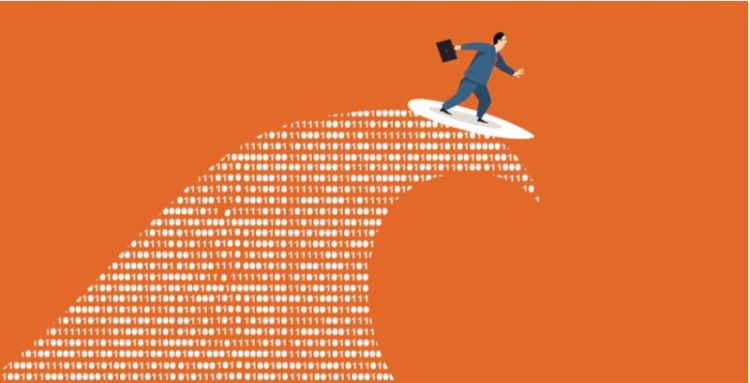
9 Essential Data Analyst Skills: A Comprehensive Career Guide
8 Types of Data Analysis
The different types of data analysis include descriptive, diagnostic, exploratory, inferential, predictive, causal, mechanistic and prescriptive. Here’s what you need to know about each one.

Data analysis is an aspect of data science and data analytics that is all about analyzing data for different kinds of purposes. The data analysis process involves inspecting, cleaning, transforming and modeling data to draw useful insights from it.
Types of Data Analysis
- Descriptive analysis
- Diagnostic analysis
- Exploratory analysis
- Inferential analysis
- Predictive analysis
- Causal analysis
- Mechanistic analysis
- Prescriptive analysis
With its multiple facets, methodologies and techniques, data analysis is used in a variety of fields, including energy, healthcare and marketing, among others. As businesses thrive under the influence of technological advancements in data analytics, data analysis plays a huge role in decision-making , providing a better, faster and more effective system that minimizes risks and reduces human biases .
That said, there are different kinds of data analysis with different goals. We’ll examine each one below.
Two Camps of Data Analysis
Data analysis can be divided into two camps, according to the book R for Data Science :
- Hypothesis Generation: This involves looking deeply at the data and combining your domain knowledge to generate hypotheses about why the data behaves the way it does.
- Hypothesis Confirmation: This involves using a precise mathematical model to generate falsifiable predictions with statistical sophistication to confirm your prior hypotheses.
More on Data Analysis: Data Analyst vs. Data Scientist: Similarities and Differences Explained
Data analysis can be separated and organized into types, arranged in an increasing order of complexity.
1. Descriptive Analysis
The goal of descriptive analysis is to describe or summarize a set of data . Here’s what you need to know:
- Descriptive analysis is the very first analysis performed in the data analysis process.
- It generates simple summaries of samples and measurements.
- It involves common, descriptive statistics like measures of central tendency, variability, frequency and position.
Descriptive Analysis Example
Take the Covid-19 statistics page on Google, for example. The line graph is a pure summary of the cases/deaths, a presentation and description of the population of a particular country infected by the virus.
Descriptive analysis is the first step in analysis where you summarize and describe the data you have using descriptive statistics, and the result is a simple presentation of your data.
2. Diagnostic Analysis
Diagnostic analysis seeks to answer the question “Why did this happen?” by taking a more in-depth look at data to uncover subtle patterns. Here’s what you need to know:
- Diagnostic analysis typically comes after descriptive analysis, taking initial findings and investigating why certain patterns in data happen.
- Diagnostic analysis may involve analyzing other related data sources, including past data, to reveal more insights into current data trends.
- Diagnostic analysis is ideal for further exploring patterns in data to explain anomalies .
Diagnostic Analysis Example
A footwear store wants to review its website traffic levels over the previous 12 months. Upon compiling and assessing the data, the company’s marketing team finds that June experienced above-average levels of traffic while July and August witnessed slightly lower levels of traffic.
To find out why this difference occurred, the marketing team takes a deeper look. Team members break down the data to focus on specific categories of footwear. In the month of June, they discovered that pages featuring sandals and other beach-related footwear received a high number of views while these numbers dropped in July and August.
Marketers may also review other factors like seasonal changes and company sales events to see if other variables could have contributed to this trend.
3. Exploratory Analysis (EDA)
Exploratory analysis involves examining or exploring data and finding relationships between variables that were previously unknown. Here’s what you need to know:
- EDA helps you discover relationships between measures in your data, which are not evidence for the existence of the correlation, as denoted by the phrase, “ Correlation doesn’t imply causation .”
- It’s useful for discovering new connections and forming hypotheses. It drives design planning and data collection .
Exploratory Analysis Example
Climate change is an increasingly important topic as the global temperature has gradually risen over the years. One example of an exploratory data analysis on climate change involves taking the rise in temperature over the years from 1950 to 2020 and the increase of human activities and industrialization to find relationships from the data. For example, you may increase the number of factories, cars on the road and airplane flights to see how that correlates with the rise in temperature.
Exploratory analysis explores data to find relationships between measures without identifying the cause. It’s most useful when formulating hypotheses.
4. Inferential Analysis
Inferential analysis involves using a small sample of data to infer information about a larger population of data.
The goal of statistical modeling itself is all about using a small amount of information to extrapolate and generalize information to a larger group. Here’s what you need to know:
- Inferential analysis involves using estimated data that is representative of a population and gives a measure of uncertainty or standard deviation to your estimation.
- The accuracy of inference depends heavily on your sampling scheme. If the sample isn’t representative of the population, the generalization will be inaccurate. This is known as the central limit theorem .
Inferential Analysis Example
A psychological study on the benefits of sleep might have a total of 500 people involved. When they followed up with the candidates, the candidates reported to have better overall attention spans and well-being with seven to nine hours of sleep, while those with less sleep and more sleep than the given range suffered from reduced attention spans and energy. This study drawn from 500 people was just a tiny portion of the 7 billion people in the world, and is thus an inference of the larger population.
Inferential analysis extrapolates and generalizes the information of the larger group with a smaller sample to generate analysis and predictions.
5. Predictive Analysis
Predictive analysis involves using historical or current data to find patterns and make predictions about the future. Here’s what you need to know:
- The accuracy of the predictions depends on the input variables.
- Accuracy also depends on the types of models. A linear model might work well in some cases, and in other cases it might not.
- Using a variable to predict another one doesn’t denote a causal relationship.
Predictive Analysis Example
The 2020 United States election is a popular topic and many prediction models are built to predict the winning candidate. FiveThirtyEight did this to forecast the 2016 and 2020 elections. Prediction analysis for an election would require input variables such as historical polling data, trends and current polling data in order to return a good prediction. Something as large as an election wouldn’t just be using a linear model, but a complex model with certain tunings to best serve its purpose.
6. Causal Analysis
Causal analysis looks at the cause and effect of relationships between variables and is focused on finding the cause of a correlation. This way, researchers can examine how a change in one variable affects another. Here’s what you need to know:
- To find the cause, you have to question whether the observed correlations driving your conclusion are valid. Just looking at the surface data won’t help you discover the hidden mechanisms underlying the correlations.
- Causal analysis is applied in randomized studies focused on identifying causation.
- Causal analysis is the gold standard in data analysis and scientific studies where the cause of a phenomenon is to be extracted and singled out, like separating wheat from chaff.
- Good data is hard to find and requires expensive research and studies. These studies are analyzed in aggregate (multiple groups), and the observed relationships are just average effects (mean) of the whole population. This means the results might not apply to everyone.
Causal Analysis Example
Say you want to test out whether a new drug improves human strength and focus. To do that, you perform randomized control trials for the drug to test its effect. You compare the sample of candidates for your new drug against the candidates receiving a mock control drug through a few tests focused on strength and overall focus and attention. This will allow you to observe how the drug affects the outcome.
7. Mechanistic Analysis
Mechanistic analysis is used to understand exact changes in variables that lead to other changes in other variables . In some ways, it is a predictive analysis, but it’s modified to tackle studies that require high precision and meticulous methodologies for physical or engineering science. Here’s what you need to know:
- It’s applied in physical or engineering sciences, situations that require high precision and little room for error, only noise in data is measurement error.
- It’s designed to understand a biological or behavioral process, the pathophysiology of a disease or the mechanism of action of an intervention.
Mechanistic Analysis Example
Say an experiment is done to simulate safe and effective nuclear fusion to power the world. A mechanistic analysis of the study would entail a precise balance of controlling and manipulating variables with highly accurate measures of both variables and the desired outcomes. It’s this intricate and meticulous modus operandi toward these big topics that allows for scientific breakthroughs and advancement of society.
8. Prescriptive Analysis
Prescriptive analysis compiles insights from other previous data analyses and determines actions that teams or companies can take to prepare for predicted trends. Here’s what you need to know:
- Prescriptive analysis may come right after predictive analysis, but it may involve combining many different data analyses.
- Companies need advanced technology and plenty of resources to conduct prescriptive analysis. Artificial intelligence systems that process data and adjust automated tasks are an example of the technology required to perform prescriptive analysis.
Prescriptive Analysis Example
Prescriptive analysis is pervasive in everyday life, driving the curated content users consume on social media. On platforms like TikTok and Instagram, algorithms can apply prescriptive analysis to review past content a user has engaged with and the kinds of behaviors they exhibited with specific posts. Based on these factors, an algorithm seeks out similar content that is likely to elicit the same response and recommends it on a user’s personal feed.
More on Data Explaining the Empirical Rule for Normal Distribution
When to Use the Different Types of Data Analysis
- Descriptive analysis summarizes the data at hand and presents your data in a comprehensible way.
- Diagnostic analysis takes a more detailed look at data to reveal why certain patterns occur, making it a good method for explaining anomalies.
- Exploratory data analysis helps you discover correlations and relationships between variables in your data.
- Inferential analysis is for generalizing the larger population with a smaller sample size of data.
- Predictive analysis helps you make predictions about the future with data.
- Causal analysis emphasizes finding the cause of a correlation between variables.
- Mechanistic analysis is for measuring the exact changes in variables that lead to other changes in other variables.
- Prescriptive analysis combines insights from different data analyses to develop a course of action teams and companies can take to capitalize on predicted outcomes.
A few important tips to remember about data analysis include:
- Correlation doesn’t imply causation.
- EDA helps discover new connections and form hypotheses.
- Accuracy of inference depends on the sampling scheme.
- A good prediction depends on the right input variables.
- A simple linear model with enough data usually does the trick.
- Using a variable to predict another doesn’t denote causal relationships.
- Good data is hard to find, and to produce it requires expensive research.
- Results from studies are done in aggregate and are average effects and might not apply to everyone.
Frequently Asked Questions
What is an example of data analysis.
A marketing team reviews a company’s web traffic over the past 12 months. To understand why sales rise and fall during certain months, the team breaks down the data to look at shoe type, seasonal patterns and sales events. Based on this in-depth analysis, the team can determine variables that influenced web traffic and make adjustments as needed.

How do you know which data analysis method to use?
Selecting a data analysis method depends on the goals of the analysis and the complexity of the task, among other factors. It’s best to assess the circumstances and consider the pros and cons of each type of data analysis before moving forward with a particular method.
Recent Data Science Articles
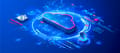
We use essential cookies to make Venngage work. By clicking “Accept All Cookies”, you agree to the storing of cookies on your device to enhance site navigation, analyze site usage, and assist in our marketing efforts.
Manage Cookies
Cookies and similar technologies collect certain information about how you’re using our website. Some of them are essential, and without them you wouldn’t be able to use Venngage. But others are optional, and you get to choose whether we use them or not.
Strictly Necessary Cookies
These cookies are always on, as they’re essential for making Venngage work, and making it safe. Without these cookies, services you’ve asked for can’t be provided.
Show cookie providers
- Google Login
Functionality Cookies
These cookies help us provide enhanced functionality and personalisation, and remember your settings. They may be set by us or by third party providers.
Performance Cookies
These cookies help us analyze how many people are using Venngage, where they come from and how they're using it. If you opt out of these cookies, we can’t get feedback to make Venngage better for you and all our users.
- Google Analytics
Targeting Cookies
These cookies are set by our advertising partners to track your activity and show you relevant Venngage ads on other sites as you browse the internet.
- Google Tag Manager
- Infographics
- Daily Infographics
- Popular Templates
- Accessibility
- Graphic Design
- Graphs and Charts
- Data Visualization
- Human Resources
- Beginner Guides
Blog Data Visualization 10 Data Presentation Examples For Strategic Communication
10 Data Presentation Examples For Strategic Communication
Written by: Krystle Wong Sep 28, 2023
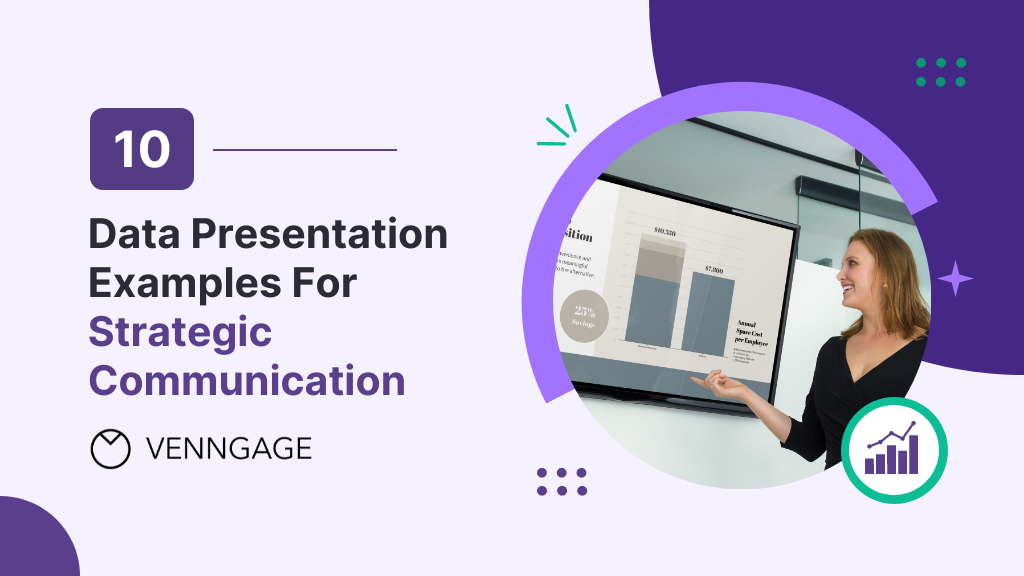
Knowing how to present data is like having a superpower.
Data presentation today is no longer just about numbers on a screen; it’s storytelling with a purpose. It’s about captivating your audience, making complex stuff look simple and inspiring action.
To help turn your data into stories that stick, influence decisions and make an impact, check out Venngage’s free chart maker or follow me on a tour into the world of data storytelling along with data presentation templates that work across different fields, from business boardrooms to the classroom and beyond. Keep scrolling to learn more!
Click to jump ahead:
10 Essential data presentation examples + methods you should know
What should be included in a data presentation, what are some common mistakes to avoid when presenting data, faqs on data presentation examples, transform your message with impactful data storytelling.
Data presentation is a vital skill in today’s information-driven world. Whether you’re in business, academia, or simply want to convey information effectively, knowing the different ways of presenting data is crucial. For impactful data storytelling, consider these essential data presentation methods:
1. Bar graph
Ideal for comparing data across categories or showing trends over time.
Bar graphs, also known as bar charts are workhorses of data presentation. They’re like the Swiss Army knives of visualization methods because they can be used to compare data in different categories or display data changes over time.
In a bar chart, categories are displayed on the x-axis and the corresponding values are represented by the height of the bars on the y-axis.
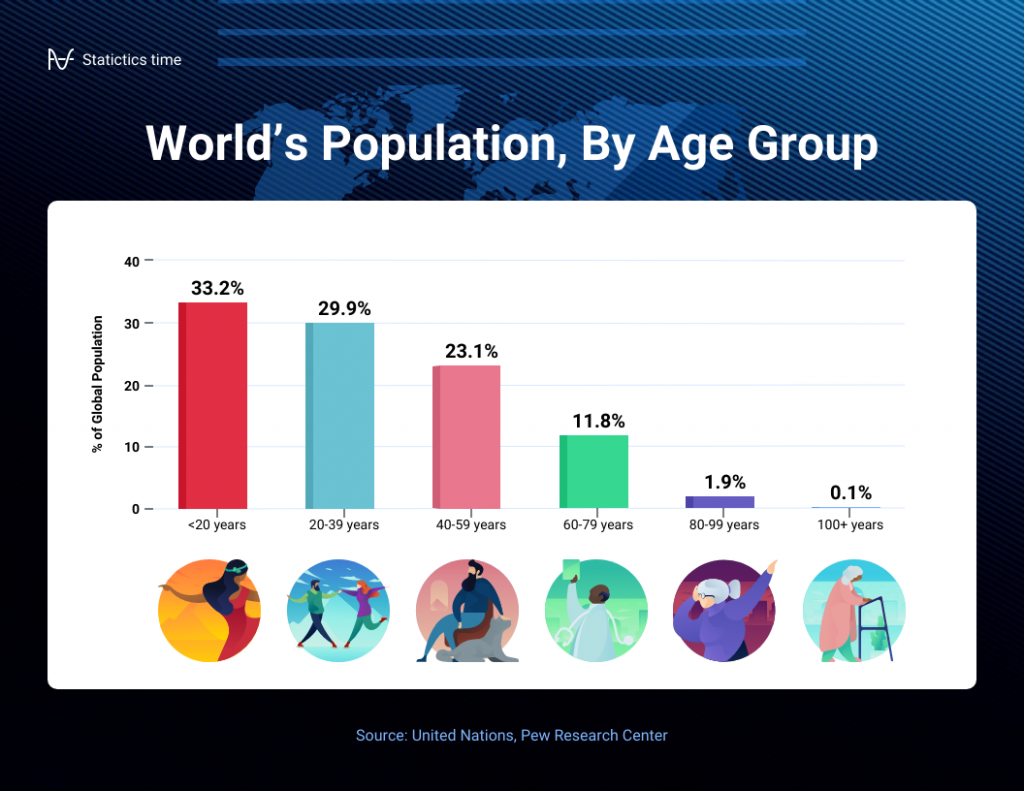
It’s a straightforward and effective way to showcase raw data, making it a staple in business reports, academic presentations and beyond.
Make sure your bar charts are concise with easy-to-read labels. Whether your bars go up or sideways, keep it simple by not overloading with too many categories.
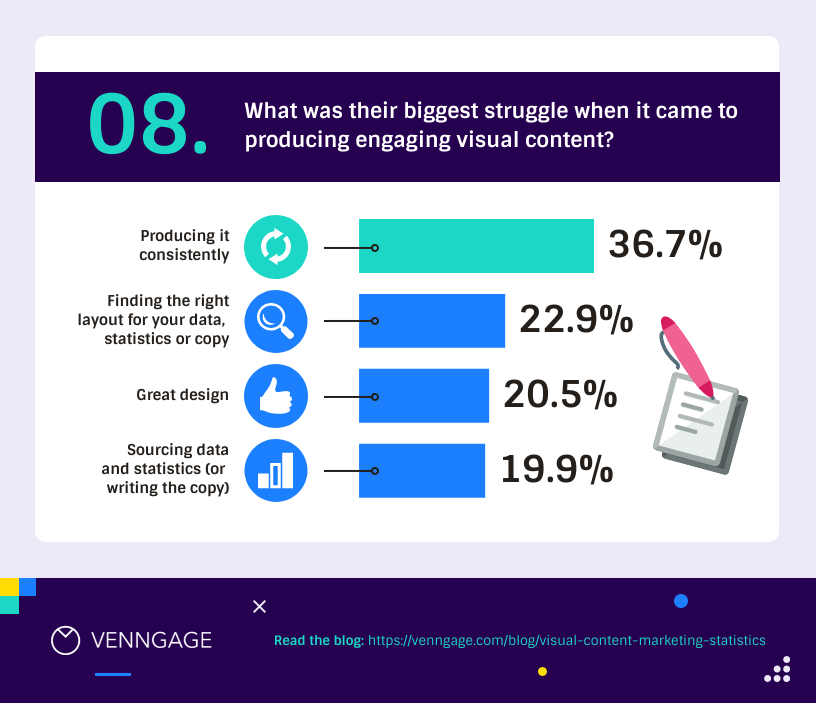
2. Line graph
Great for displaying trends and variations in data points over time or continuous variables.
Line charts or line graphs are your go-to when you want to visualize trends and variations in data sets over time.
One of the best quantitative data presentation examples, they work exceptionally well for showing continuous data, such as sales projections over the last couple of years or supply and demand fluctuations.
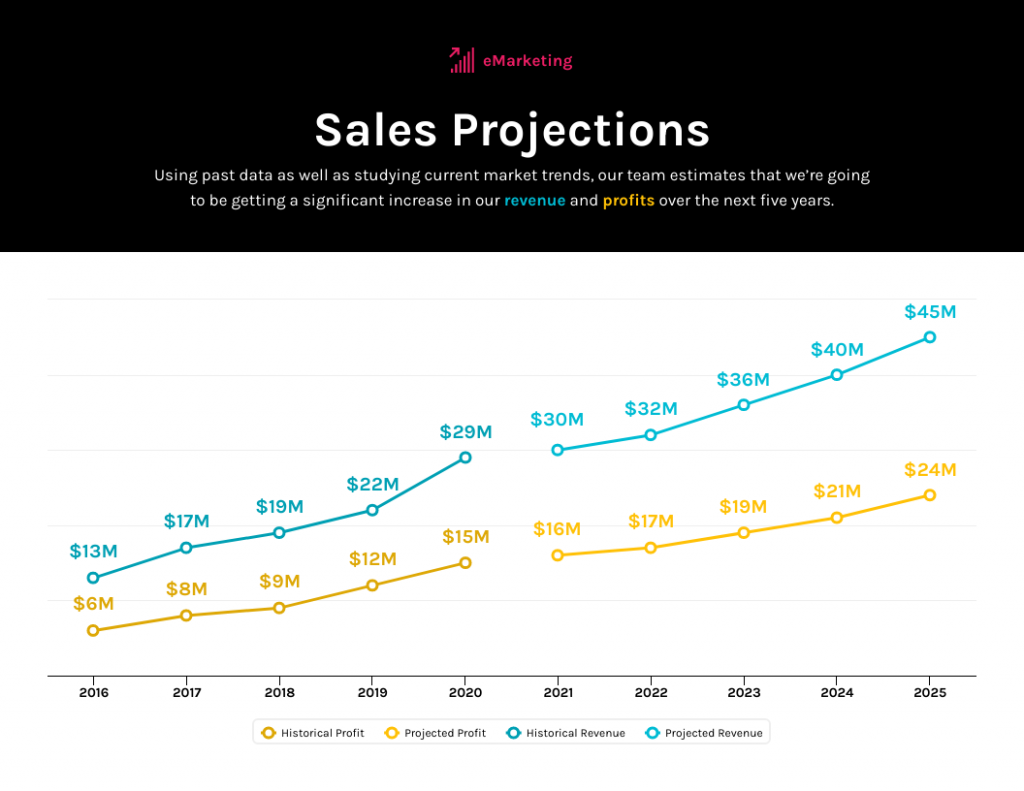
The x-axis represents time or a continuous variable and the y-axis represents the data values. By connecting the data points with lines, you can easily spot trends and fluctuations.
A tip when presenting data with line charts is to minimize the lines and not make it too crowded. Highlight the big changes, put on some labels and give it a catchy title.
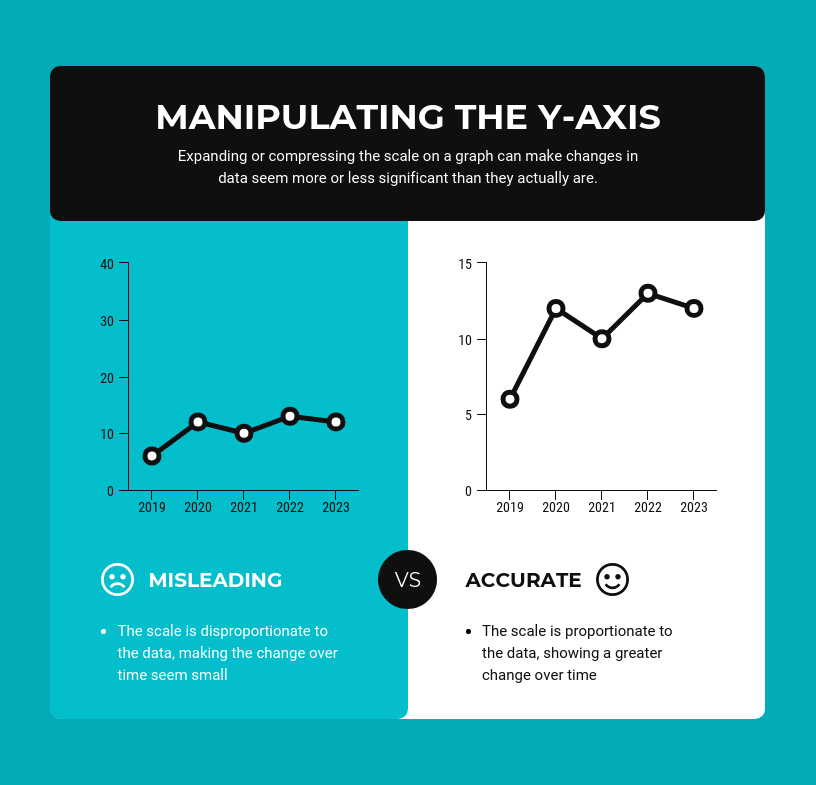
3. Pie chart
Useful for illustrating parts of a whole, such as percentages or proportions.
Pie charts are perfect for showing how a whole is divided into parts. They’re commonly used to represent percentages or proportions and are great for presenting survey results that involve demographic data.
Each “slice” of the pie represents a portion of the whole and the size of each slice corresponds to its share of the total.
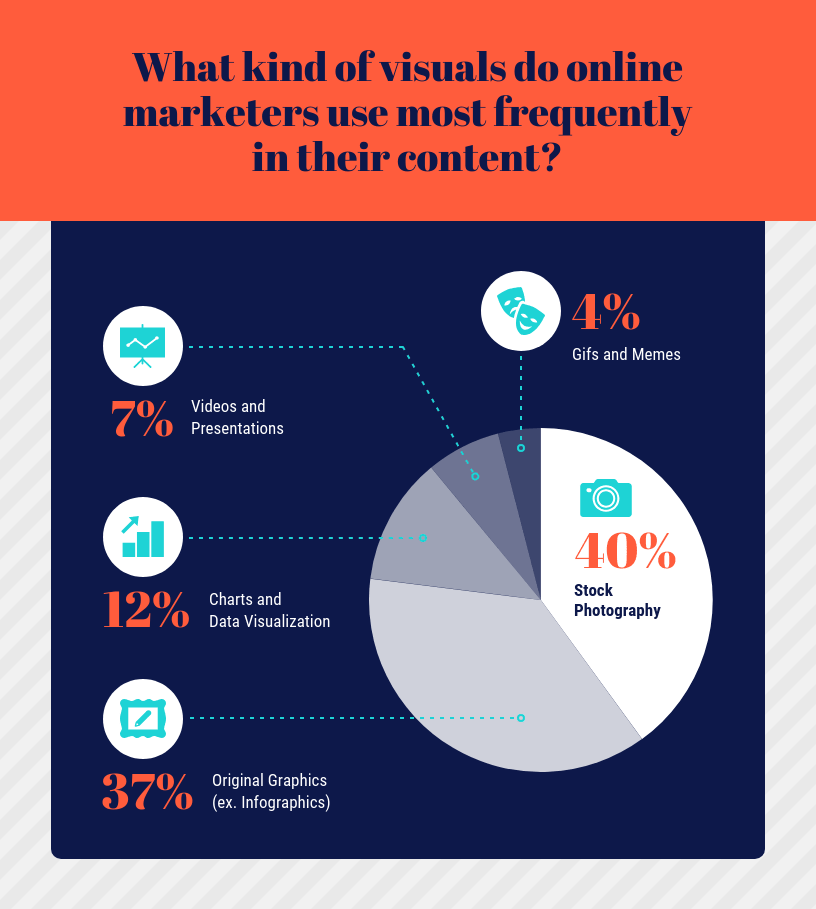
While pie charts are handy for illustrating simple distributions, they can become confusing when dealing with too many categories or when the differences in proportions are subtle.
Don’t get too carried away with slices — label those slices with percentages or values so people know what’s what and consider using a legend for more categories.
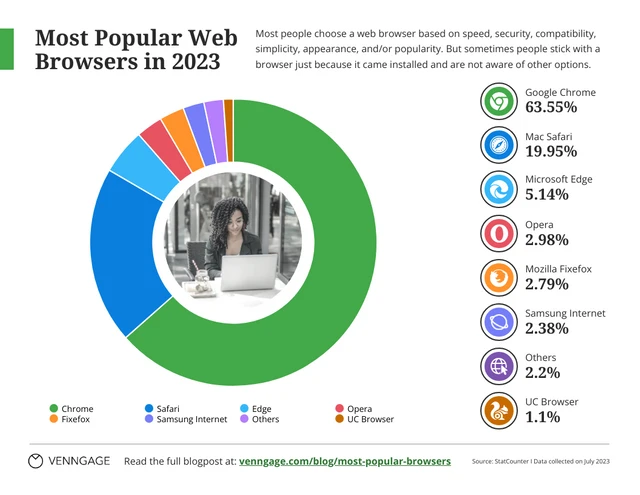
4. Scatter plot
Effective for showing the relationship between two variables and identifying correlations.
Scatter plots are all about exploring relationships between two variables. They’re great for uncovering correlations, trends or patterns in data.
In a scatter plot, every data point appears as a dot on the chart, with one variable marked on the horizontal x-axis and the other on the vertical y-axis.
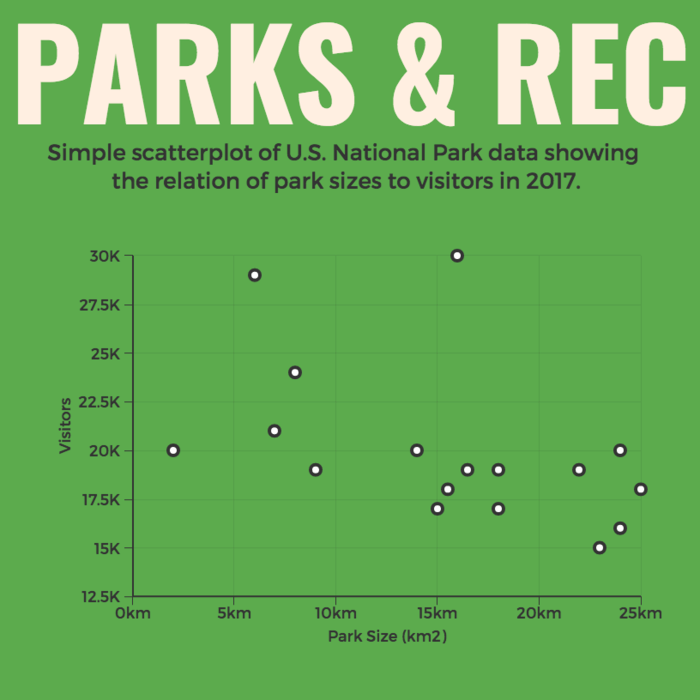
By examining the scatter of points, you can discern the nature of the relationship between the variables, whether it’s positive, negative or no correlation at all.
If you’re using scatter plots to reveal relationships between two variables, be sure to add trendlines or regression analysis when appropriate to clarify patterns. Label data points selectively or provide tooltips for detailed information.
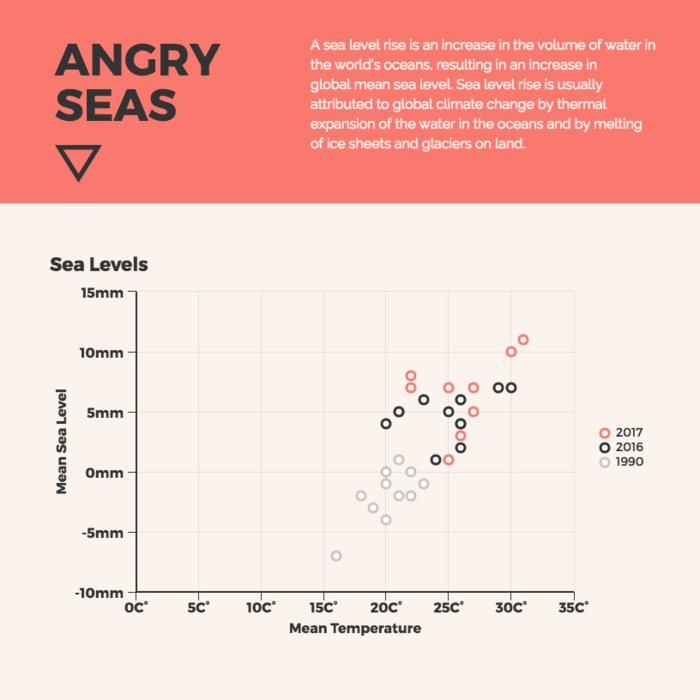
5. Histogram
Best for visualizing the distribution and frequency of a single variable.
Histograms are your choice when you want to understand the distribution and frequency of a single variable.
They divide the data into “bins” or intervals and the height of each bar represents the frequency or count of data points falling into that interval.
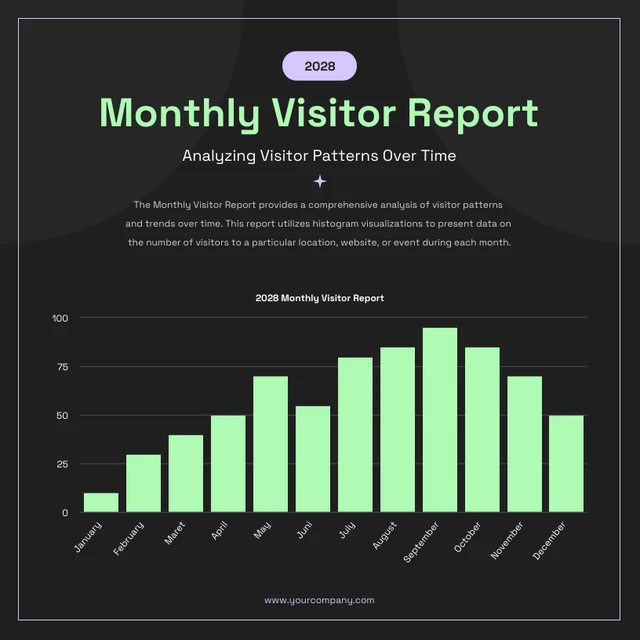
Histograms are excellent for helping to identify trends in data distributions, such as peaks, gaps or skewness.
Here’s something to take note of — ensure that your histogram bins are appropriately sized to capture meaningful data patterns. Using clear axis labels and titles can also help explain the distribution of the data effectively.
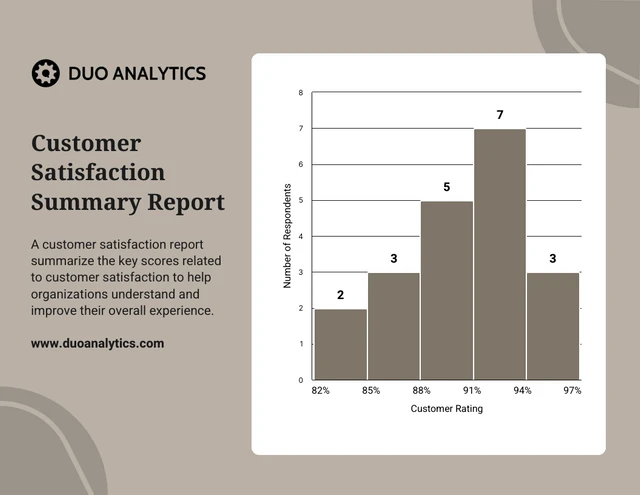
6. Stacked bar chart
Useful for showing how different components contribute to a whole over multiple categories.
Stacked bar charts are a handy choice when you want to illustrate how different components contribute to a whole across multiple categories.
Each bar represents a category and the bars are divided into segments to show the contribution of various components within each category.
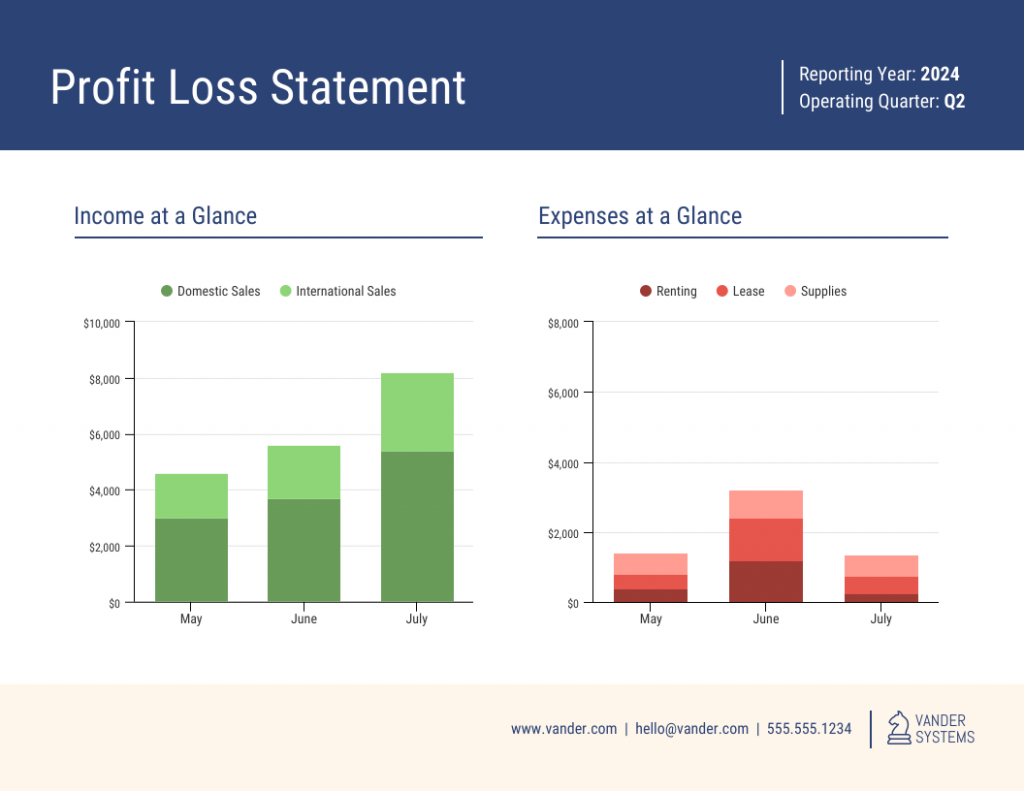
This method is ideal for highlighting both the individual and collective significance of each component, making it a valuable tool for comparative analysis.
Stacked bar charts are like data sandwiches—label each layer so people know what’s what. Keep the order logical and don’t forget the paintbrush for snazzy colors. Here’s a data analysis presentation example on writers’ productivity using stacked bar charts:
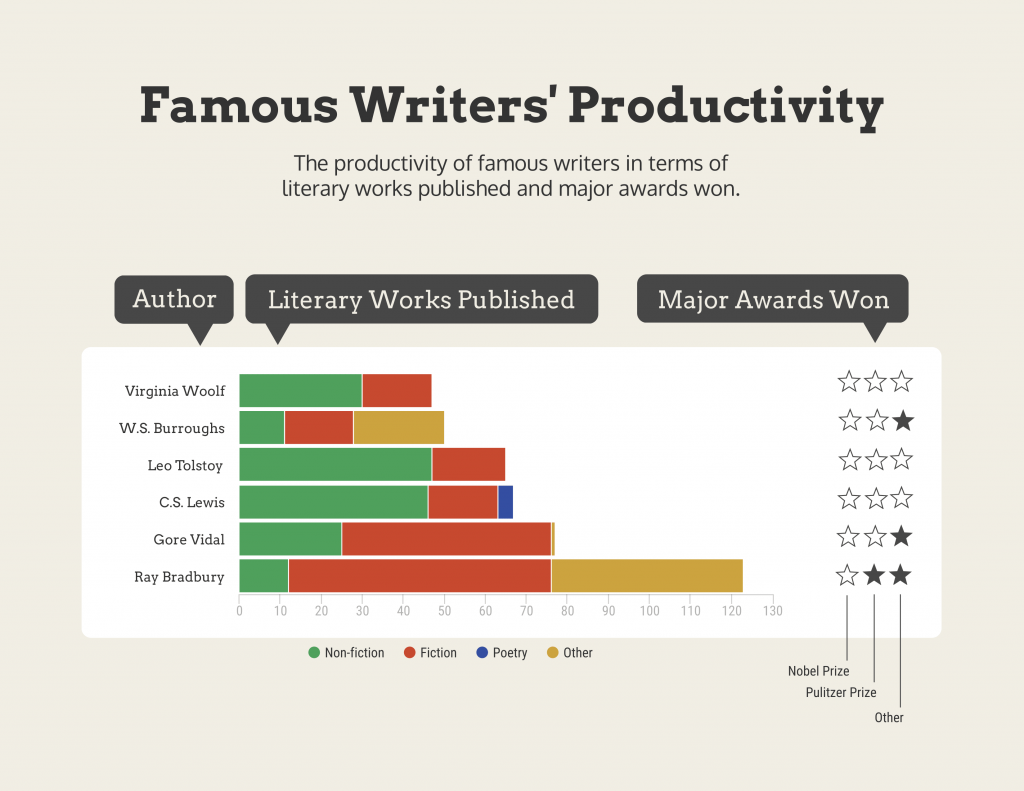
7. Area chart
Similar to line charts but with the area below the lines filled, making them suitable for showing cumulative data.
Area charts are close cousins of line charts but come with a twist.
Imagine plotting the sales of a product over several months. In an area chart, the space between the line and the x-axis is filled, providing a visual representation of the cumulative total.
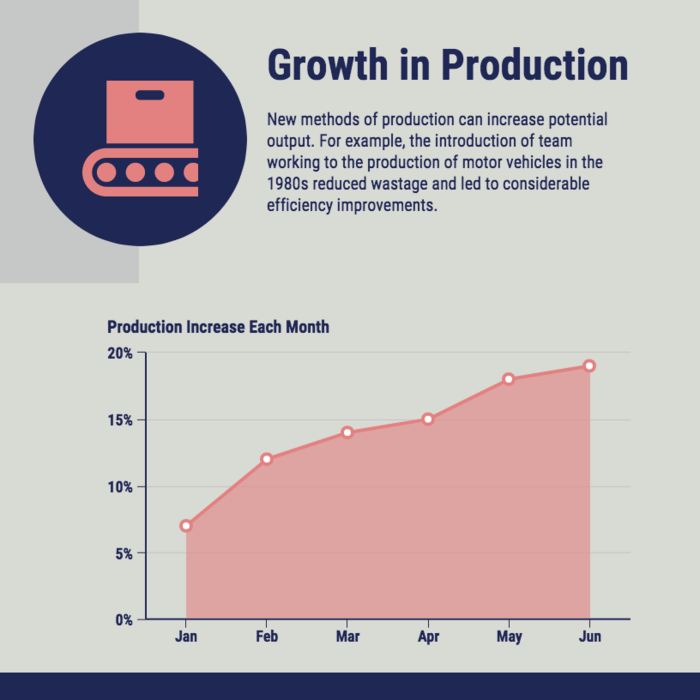
This makes it easy to see how values stack up over time, making area charts a valuable tool for tracking trends in data.
For area charts, use them to visualize cumulative data and trends, but avoid overcrowding the chart. Add labels, especially at significant points and make sure the area under the lines is filled with a visually appealing color gradient.
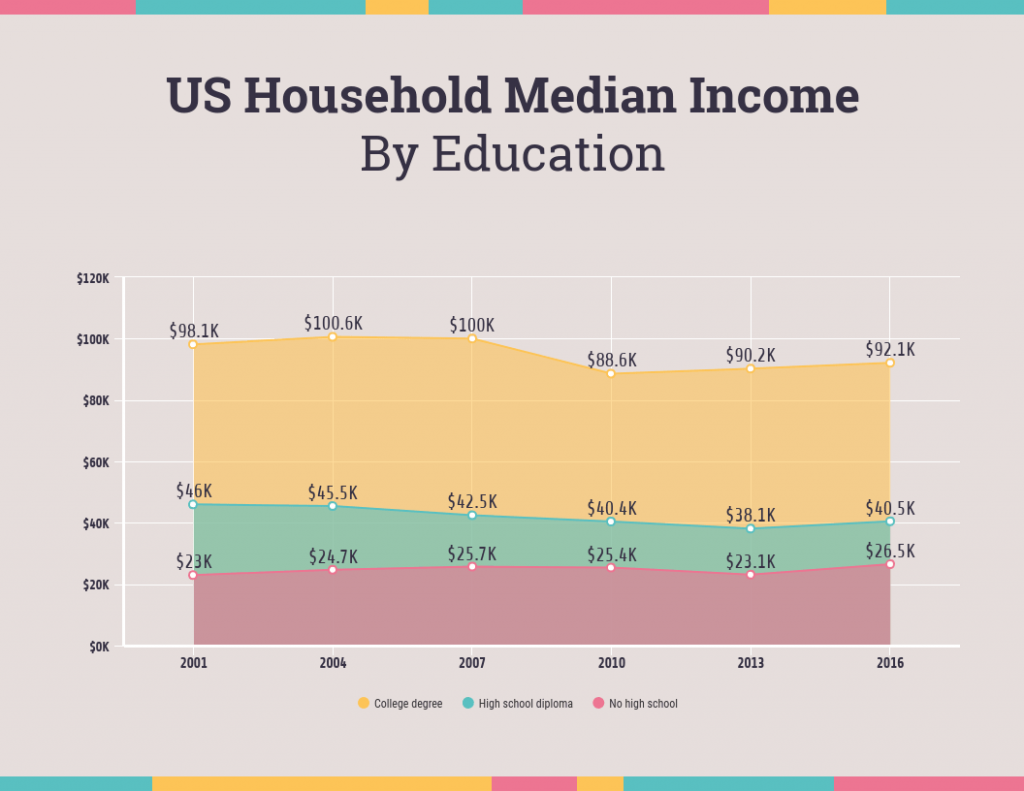
8. Tabular presentation
Presenting data in rows and columns, often used for precise data values and comparisons.
Tabular data presentation is all about clarity and precision. Think of it as presenting numerical data in a structured grid, with rows and columns clearly displaying individual data points.
A table is invaluable for showcasing detailed data, facilitating comparisons and presenting numerical information that needs to be exact. They’re commonly used in reports, spreadsheets and academic papers.
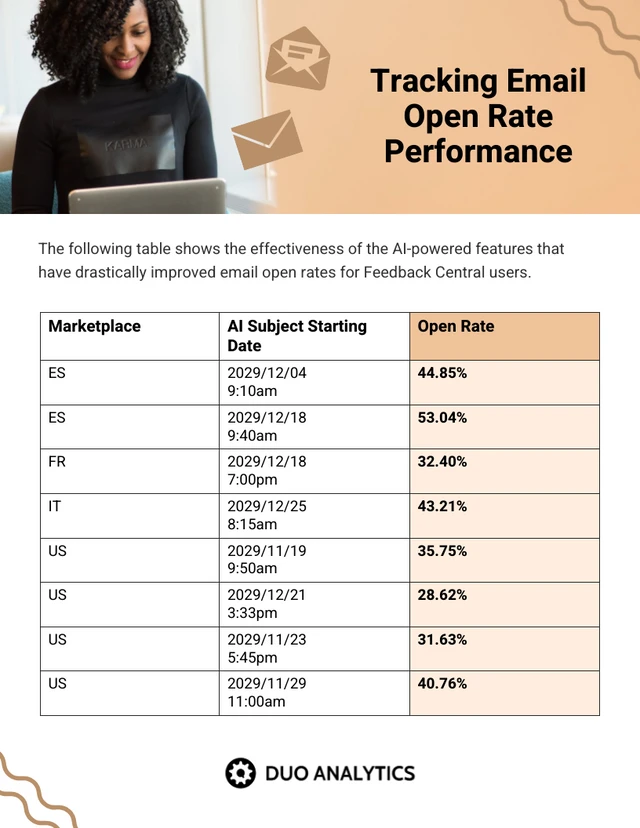
When presenting tabular data, organize it neatly with clear headers and appropriate column widths. Highlight important data points or patterns using shading or font formatting for better readability.
9. Textual data
Utilizing written or descriptive content to explain or complement data, such as annotations or explanatory text.
Textual data presentation may not involve charts or graphs, but it’s one of the most used qualitative data presentation examples.
It involves using written content to provide context, explanations or annotations alongside data visuals. Think of it as the narrative that guides your audience through the data.
Well-crafted textual data can make complex information more accessible and help your audience understand the significance of the numbers and visuals.
Textual data is your chance to tell a story. Break down complex information into bullet points or short paragraphs and use headings to guide the reader’s attention.
10. Pictogram
Using simple icons or images to represent data is especially useful for conveying information in a visually intuitive manner.
Pictograms are all about harnessing the power of images to convey data in an easy-to-understand way.
Instead of using numbers or complex graphs, you use simple icons or images to represent data points.
For instance, you could use a thumbs up emoji to illustrate customer satisfaction levels, where each face represents a different level of satisfaction.
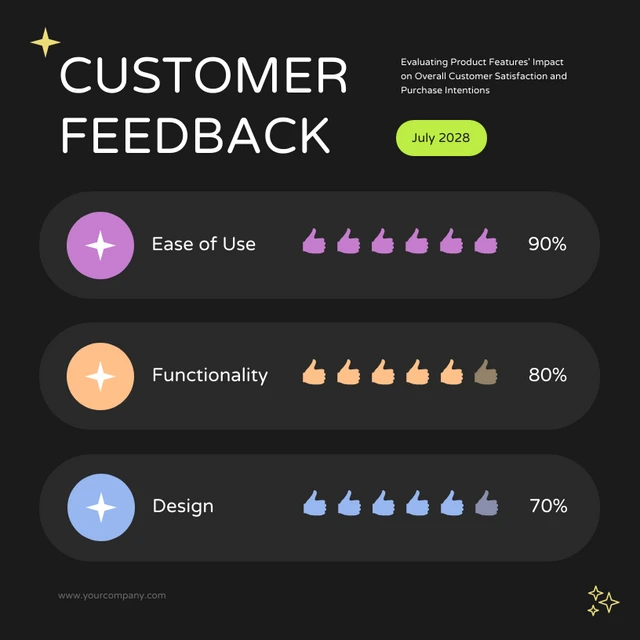
Pictograms are great for conveying data visually, so choose symbols that are easy to interpret and relevant to the data. Use consistent scaling and a legend to explain the symbols’ meanings, ensuring clarity in your presentation.
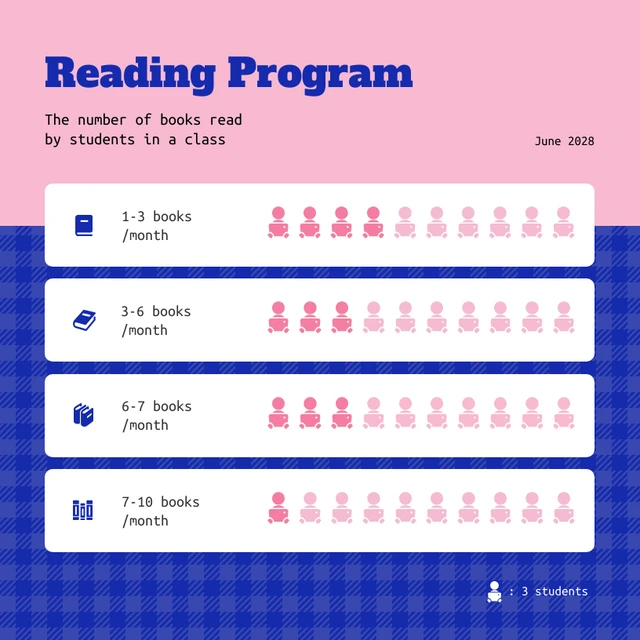
Looking for more data presentation ideas? Use the Venngage graph maker or browse through our gallery of chart templates to pick a template and get started!
A comprehensive data presentation should include several key elements to effectively convey information and insights to your audience. Here’s a list of what should be included in a data presentation:
1. Title and objective
- Begin with a clear and informative title that sets the context for your presentation.
- State the primary objective or purpose of the presentation to provide a clear focus.
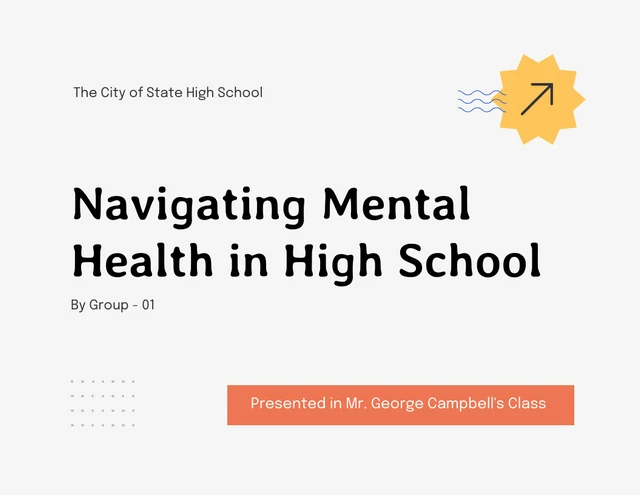
2. Key data points
- Present the most essential data points or findings that align with your objective.
- Use charts, graphical presentations or visuals to illustrate these key points for better comprehension.
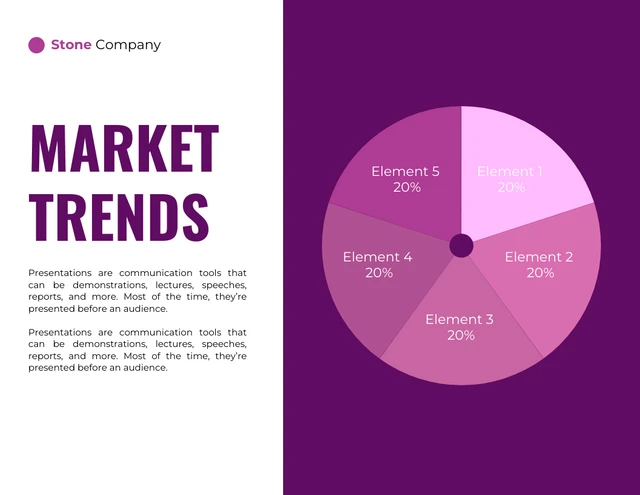
3. Context and significance
- Provide a brief overview of the context in which the data was collected and why it’s significant.
- Explain how the data relates to the larger picture or the problem you’re addressing.
4. Key takeaways
- Summarize the main insights or conclusions that can be drawn from the data.
- Highlight the key takeaways that the audience should remember.
5. Visuals and charts
- Use clear and appropriate visual aids to complement the data.
- Ensure that visuals are easy to understand and support your narrative.
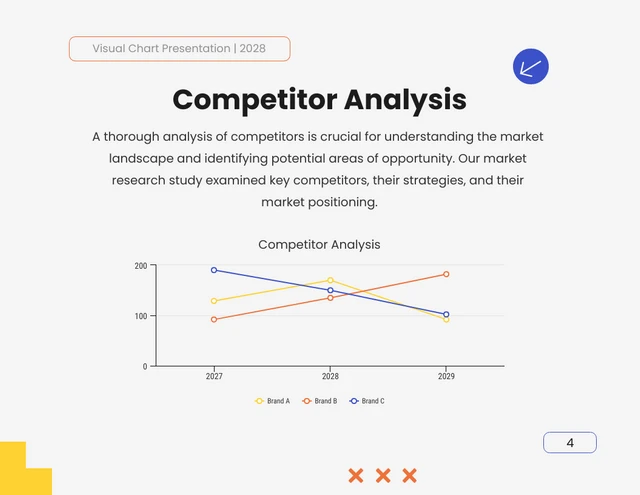
6. Implications or actions
- Discuss the practical implications of the data or any recommended actions.
- If applicable, outline next steps or decisions that should be taken based on the data.
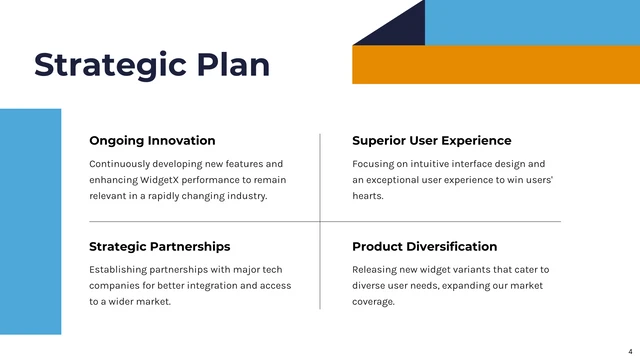
7. Q&A and discussion
- Allocate time for questions and open discussion to engage the audience.
- Address queries and provide additional insights or context as needed.
Presenting data is a crucial skill in various professional fields, from business to academia and beyond. To ensure your data presentations hit the mark, here are some common mistakes that you should steer clear of:
Overloading with data
Presenting too much data at once can overwhelm your audience. Focus on the key points and relevant information to keep the presentation concise and focused. Here are some free data visualization tools you can use to convey data in an engaging and impactful way.
Assuming everyone’s on the same page
It’s easy to assume that your audience understands as much about the topic as you do. But this can lead to either dumbing things down too much or diving into a bunch of jargon that leaves folks scratching their heads. Take a beat to figure out where your audience is coming from and tailor your presentation accordingly.
Misleading visuals
Using misleading visuals, such as distorted scales or inappropriate chart types can distort the data’s meaning. Pick the right data infographics and understandable charts to ensure that your visual representations accurately reflect the data.
Not providing context
Data without context is like a puzzle piece with no picture on it. Without proper context, data may be meaningless or misinterpreted. Explain the background, methodology and significance of the data.
Not citing sources properly
Neglecting to cite sources and provide citations for your data can erode its credibility. Always attribute data to its source and utilize reliable sources for your presentation.
Not telling a story
Avoid simply presenting numbers. If your presentation lacks a clear, engaging story that takes your audience on a journey from the beginning (setting the scene) through the middle (data analysis) to the end (the big insights and recommendations), you’re likely to lose their interest.
Infographics are great for storytelling because they mix cool visuals with short and sweet text to explain complicated stuff in a fun and easy way. Create one with Venngage’s free infographic maker to create a memorable story that your audience will remember.
Ignoring data quality
Presenting data without first checking its quality and accuracy can lead to misinformation. Validate and clean your data before presenting it.
Simplify your visuals
Fancy charts might look cool, but if they confuse people, what’s the point? Go for the simplest visual that gets your message across. Having a dilemma between presenting data with infographics v.s data design? This article on the difference between data design and infographics might help you out.
Missing the emotional connection
Data isn’t just about numbers; it’s about people and real-life situations. Don’t forget to sprinkle in some human touch, whether it’s through relatable stories, examples or showing how the data impacts real lives.
Skipping the actionable insights
At the end of the day, your audience wants to know what they should do with all the data. If you don’t wrap up with clear, actionable insights or recommendations, you’re leaving them hanging. Always finish up with practical takeaways and the next steps.
Can you provide some data presentation examples for business reports?
Business reports often benefit from data presentation through bar charts showing sales trends over time, pie charts displaying market share,or tables presenting financial performance metrics like revenue and profit margins.
What are some creative data presentation examples for academic presentations?
Creative data presentation ideas for academic presentations include using statistical infographics to illustrate research findings and statistical data, incorporating storytelling techniques to engage the audience or utilizing heat maps to visualize data patterns.
What are the key considerations when choosing the right data presentation format?
When choosing a chart format , consider factors like data complexity, audience expertise and the message you want to convey. Options include charts (e.g., bar, line, pie), tables, heat maps, data visualization infographics and interactive dashboards.
Knowing the type of data visualization that best serves your data is just half the battle. Here are some best practices for data visualization to make sure that the final output is optimized.
How can I choose the right data presentation method for my data?
To select the right data presentation method, start by defining your presentation’s purpose and audience. Then, match your data type (e.g., quantitative, qualitative) with suitable visualization techniques (e.g., histograms, word clouds) and choose an appropriate presentation format (e.g., slide deck, report, live demo).
For more presentation ideas , check out this guide on how to make a good presentation or use a presentation software to simplify the process.
How can I make my data presentations more engaging and informative?
To enhance data presentations, use compelling narratives, relatable examples and fun data infographics that simplify complex data. Encourage audience interaction, offer actionable insights and incorporate storytelling elements to engage and inform effectively.
The opening of your presentation holds immense power in setting the stage for your audience. To design a presentation and convey your data in an engaging and informative, try out Venngage’s free presentation maker to pick the right presentation design for your audience and topic.
What is the difference between data visualization and data presentation?
Data presentation typically involves conveying data reports and insights to an audience, often using visuals like charts and graphs. Data visualization , on the other hand, focuses on creating those visual representations of data to facilitate understanding and analysis.
Now that you’ve learned a thing or two about how to use these methods of data presentation to tell a compelling data story , it’s time to take these strategies and make them your own.
But here’s the deal: these aren’t just one-size-fits-all solutions. Remember that each example we’ve uncovered here is not a rigid template but a source of inspiration. It’s all about making your audience go, “Wow, I get it now!”
Think of your data presentations as your canvas – it’s where you paint your story, convey meaningful insights and make real change happen.
So, go forth, present your data with confidence and purpose and watch as your strategic influence grows, one compelling presentation at a time.
Discover popular designs
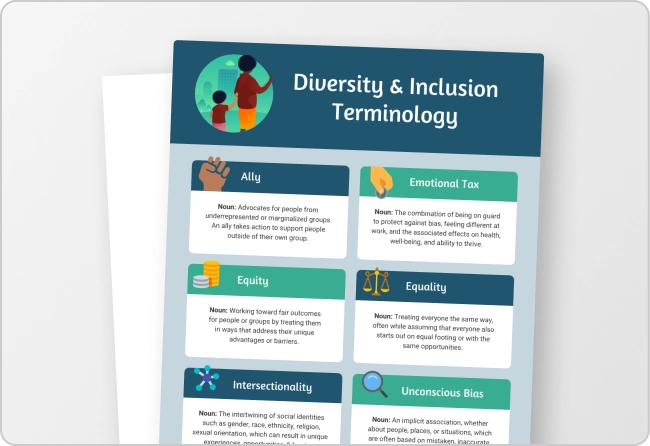
Infographic maker
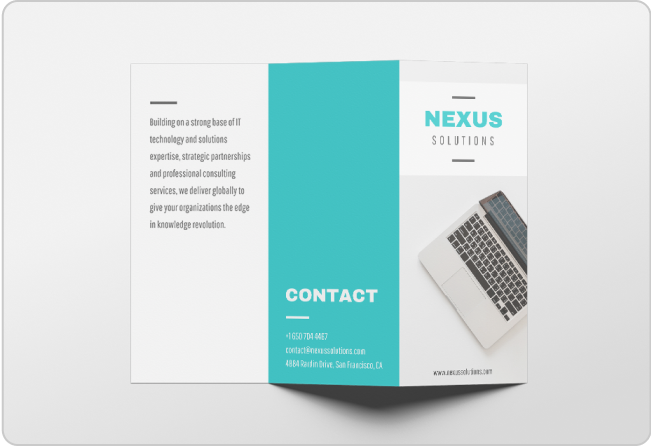
Brochure maker
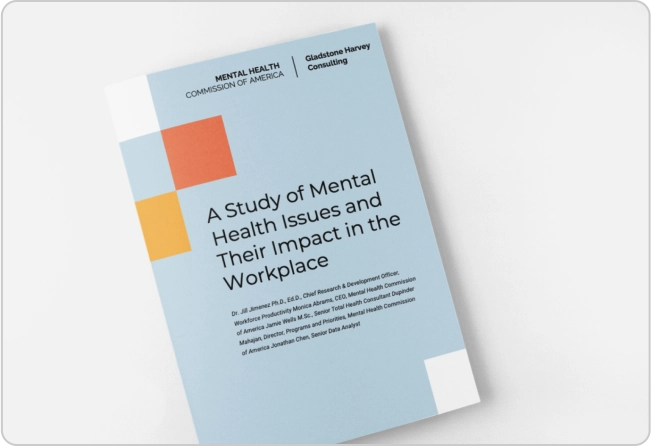
White paper online
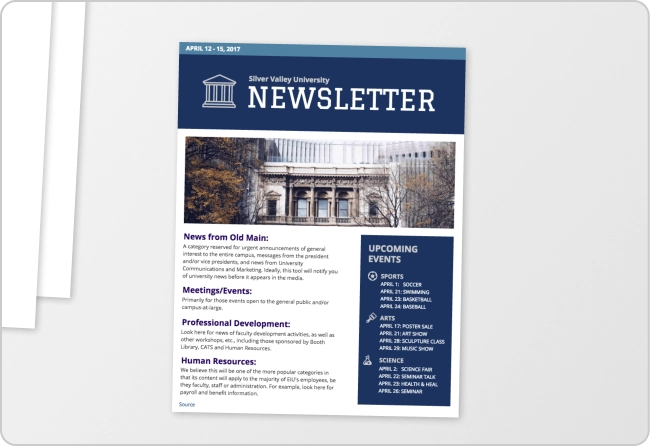
Newsletter creator
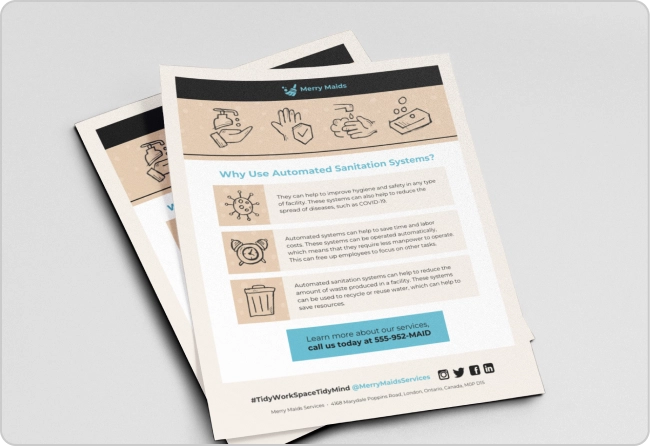
Flyer maker
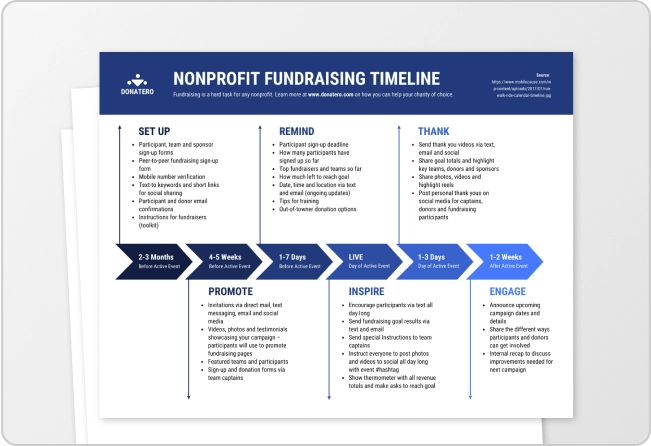
Timeline maker
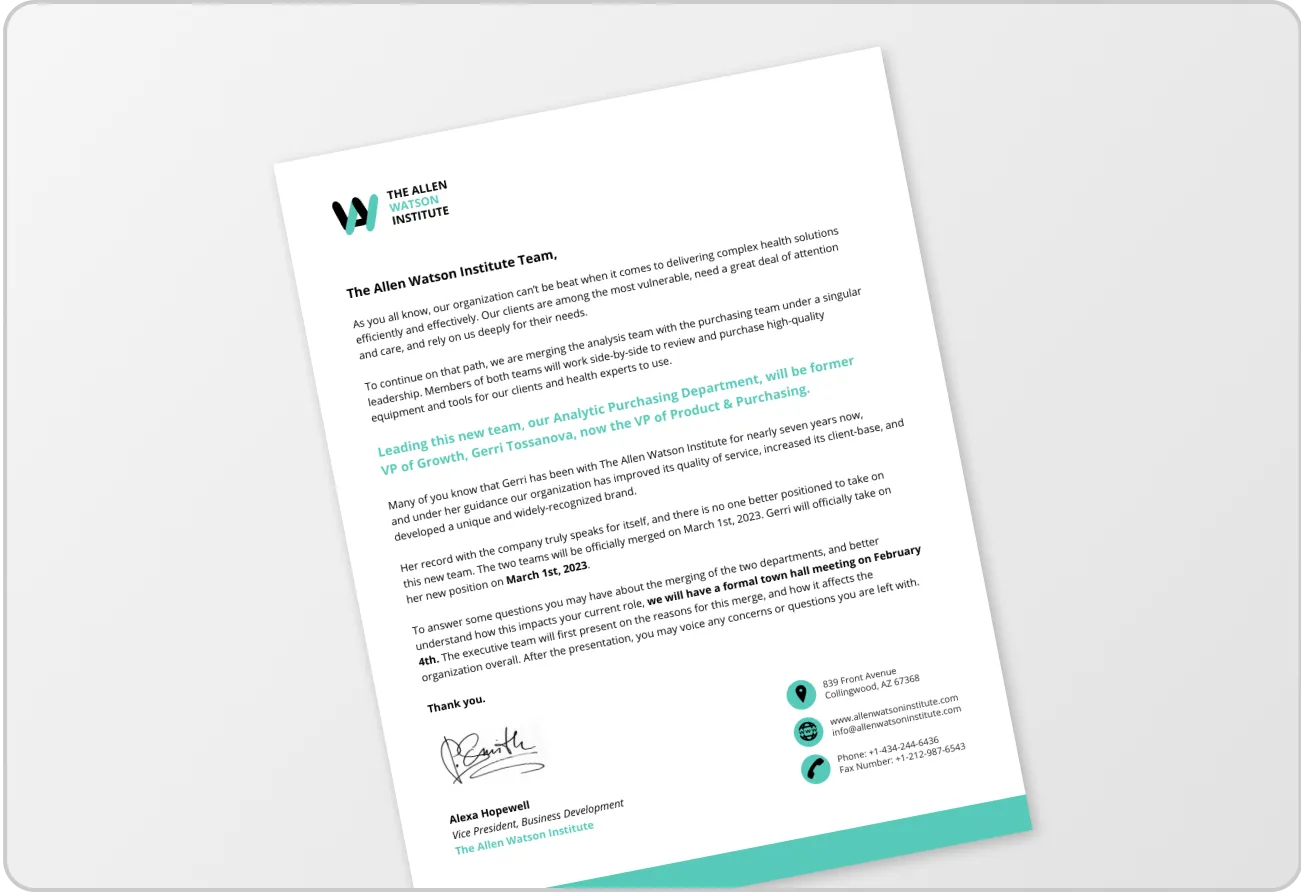
Letterhead maker
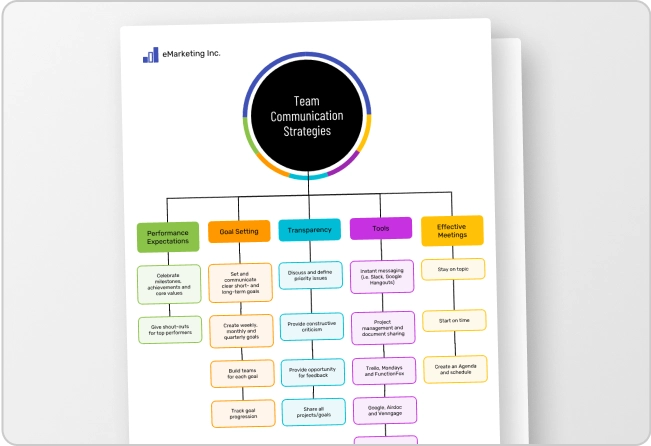
Mind map maker
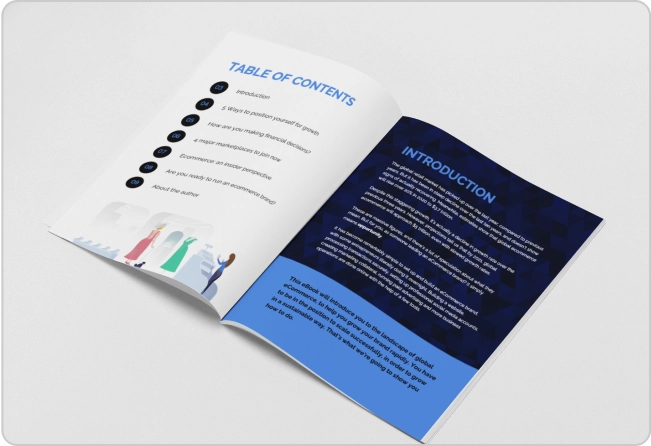
Ebook maker
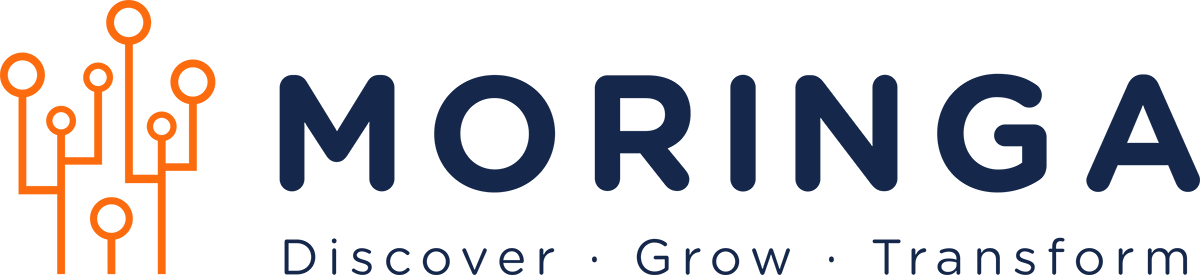
- All Courses
- Corporate Courses
- Software Engineering Online
- Software Engineering Hybrid
- Mobile App Development
- Part-time Software Engineering
- Data Science Full-time Online
- Data Science Full-time Hybrid
- Data Science Part-time Online
- Data Visualization with Python
- Data Analytics with PowerBI & Excel
- DevOps Engineering
- Introduction to Cybersecurity
- CompTIA Security+ Bootcamp
- CompTIA Cybersecurity Analyst Bootcamp (CYSA+)
- UI/UX Product Design Part-time
- UI/UX Product Design Full-time
- High School Holiday Tech Bootcamp
- Financial Aid
- Soma Student Loan
- Corporate Solutions
- Corporate Training
- Hire Our Graduates
Data Science vs. Data Analysis vs. Data Visualization: What’s the Difference?
In today’s data-driven world, terms like data science, data analysis, and data visualization are often used interchangeably, but they represent distinct fields with unique roles, required skills, and contributions to the data ecosystem. Understanding these differences is crucial for anyone looking to build a career in data or leverage data for decision-making. This blog provides a detailed comparison of these fields, their roles, required skills, and how they interconnect in the data ecosystem.
What Is Data Science?
Data science is a multidisciplinary field that uses scientific methods, algorithms, and systems to extract knowledge and insights from structured and unstructured data. It encompasses various domains, including statistics, machine learning, data mining, and big data technologies.
Career Pathways for Learning Data Science:-
- Data Scientist: Develops complex models and algorithms to analyze large datasets, identifies patterns, and makes predictions. They work on projects involving machine learning, artificial intelligence, and predictive analytics.
- Machine Learning Engineer: Designs and implements machine learning algorithms and models that can learn from and make predictions on data.
- Big Data Engineer: Manages and optimizes large-scale data processing systems, ensuring efficient storage, retrieval, and processing of big data.
Required Skills To Become A Data Scientist
- Programming Languages: Proficiency in Python, R, and SQL.
- Statistical Analysis: Strong understanding of statistical methods and tools.
- Machine Learning: Knowledge of machine learning algorithms, libraries, and frameworks (e.g., TensorFlow, Scikit-Learn).
- Data Wrangling: Ability to clean, transform, and process large datasets.
- Domain Expertise: Understanding of the specific industry or domain to apply data science effectively.
What is Data Analysis?
Data analysis involves inspecting, cleaning, transforming, and modeling data to discover useful information, draw conclusions, and support decision-making. It focuses on interpreting data to provide actionable insights.
Different roles/jobs you can go for with Data Analyst certification
- Data Analyst: Collects, processes, and performs statistical analysis on data. They generate reports, dashboards, and visualizations to communicate findings to stakeholders.
- Business Analyst: Uses data analysis to inform business decisions and strategy. They bridge the gap between data and business operations.
- Financial Analyst: Analyzes financial data to identify trends, forecast performance, and provide investment recommendations.
Skills to master to become a Data Analyst
- Statistical Tools: Proficiency in Excel, SAS, and other statistical software.
- Data Visualization: Ability to create charts, graphs, and dashboards using tools like Tableau, Power BI, and Matplotlib.
- SQL: Skills to query and manipulate databases.
- Critical Thinking: Strong analytical skills to interpret data and draw meaningful conclusions.
- Communication: Ability to present data findings clearly to non-technical stakeholders.
About Data Visualization
Data visualization is the graphical representation of data and information using visual elements like charts, graphs, and maps. It helps to make complex data more accessible, understandable, and usable by highlighting trends, outliers, and patterns.
The different Careers To Pursue with a Data Visualization background
- Data Visualization Specialist: Designs and creates visual representations of data to communicate insights effectively. They focus on the aesthetic and functional aspects of data presentation.
- BI Developer: Develops business intelligence solutions, including dashboards and reports, to visualize key metrics and support decision-making.
- UI/UX Designer: Works on the user interface and experience design of data visualization tools to ensure they are intuitive and user-friendly.
What are the Required Skills ?
- Visualization Tools: Expertise in tools like Tableau, Power BI, D3.js, and ggplot2.
- Design Principles: Understanding of visual design principles and best practices for creating effective visualizations.
- Data Interpretation: Ability to interpret and represent data accurately.
- Programming: Basic programming skills in languages like JavaScript (for D3.js) and Python (for libraries like Matplotlib and Seaborn).
- Storytelling: Skills to craft compelling narratives around data to engage and inform the audience.
How Do The 3 Specializations Interconnect?
- The Data Ecosystem
- Data Collection and Storage: Data engineers and IT professionals gather and store data from various sources in databases or data lakes.
- Data Cleaning and Preparation: Data analysts and data scientists clean and pre-process the data to ensure its quality and usability.
- Data Analysis and Modeling: Data scientists and analysts analyze the data to extract insights, build models, and make predictions.
- Data Visualization and Communication: Data visualization specialists and analysts create visual representations of the data to communicate findings effectively to stakeholders.
2. Collaboration and Synergy
- Interdisciplinary Teams: Successful data projects often involve interdisciplinary teams where data scientists, analysts, and visualization specialists collaborate to achieve common goals.
- Feedback Loops: Continuous feedback between data analysts and data scientists can refine models and improve the accuracy of predictions.
- Unified Insights: Combining robust data analysis with compelling visualizations ensures that insights are not only accurate but also easily understood and actionable by decision-makers.
While data science, data analysis, and data visualization are distinct fields, they are deeply interconnected and complementary. Data scientists focus on developing models and algorithms, data analysts interpret data to generate insights, and data visualization specialists make those insights accessible and understandable. Together, they form a comprehensive approach to leveraging data for informed decision-making and strategic advantage. By understanding the differences and synergies between these roles, individuals and organizations can better navigate the data landscape and harness the full potential of their data assets.
Moringa School offers all 3 courses explore and pursue a short course before the year ends!
Data Science Bootcamp
Blog written by Elisha Kibet a Moringa Brand Ambassador and avid technical writer passionate about tech
Recent Posts
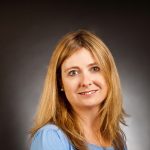
Post Categories
- → Career Readiness
- → Community Stories
- → Cybersecurity
- → Data Science
- → Devops Engineering
- → Mini Apps
- → Opinion Pieces
- → Press Releases
- → Product Design
- → Professional Development
- → Software Development
- → Uncategorized
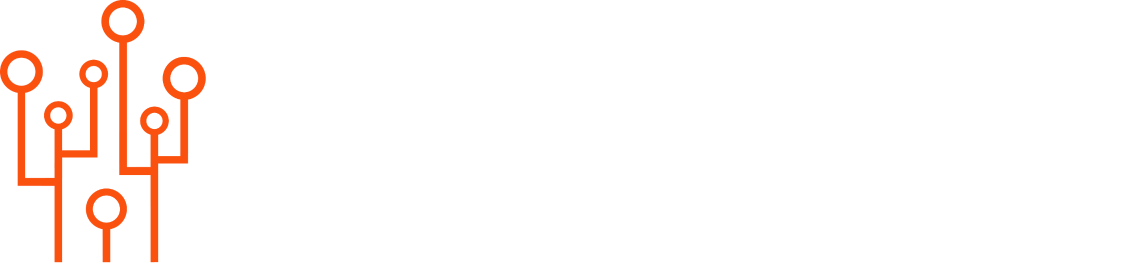

An official website of the United States government
The .gov means it’s official. Federal government websites often end in .gov or .mil. Before sharing sensitive information, make sure you’re on a federal government site.
The site is secure. The https:// ensures that you are connecting to the official website and that any information you provide is encrypted and transmitted securely.
- Publications
- Account settings
Preview improvements coming to the PMC website in October 2024. Learn More or Try it out now .
- Advanced Search
- Journal List
- Korean J Anesthesiol
- v.70(3); 2017 Jun
Statistical data presentation
1 Department of Anesthesiology and Pain Medicine, Dongguk University Ilsan Hospital, Goyang, Korea.
Sangseok Lee
2 Department of Anesthesiology and Pain Medicine, Sanggye Paik Hospital, Inje University College of Medicine, Seoul, Korea.
Data are usually collected in a raw format and thus the inherent information is difficult to understand. Therefore, raw data need to be summarized, processed, and analyzed. However, no matter how well manipulated, the information derived from the raw data should be presented in an effective format, otherwise, it would be a great loss for both authors and readers. In this article, the techniques of data and information presentation in textual, tabular, and graphical forms are introduced. Text is the principal method for explaining findings, outlining trends, and providing contextual information. A table is best suited for representing individual information and represents both quantitative and qualitative information. A graph is a very effective visual tool as it displays data at a glance, facilitates comparison, and can reveal trends and relationships within the data such as changes over time, frequency distribution, and correlation or relative share of a whole. Text, tables, and graphs for data and information presentation are very powerful communication tools. They can make an article easy to understand, attract and sustain the interest of readers, and efficiently present large amounts of complex information. Moreover, as journal editors and reviewers glance at these presentations before reading the whole article, their importance cannot be ignored.
Introduction
Data are a set of facts, and provide a partial picture of reality. Whether data are being collected with a certain purpose or collected data are being utilized, questions regarding what information the data are conveying, how the data can be used, and what must be done to include more useful information must constantly be kept in mind.
Since most data are available to researchers in a raw format, they must be summarized, organized, and analyzed to usefully derive information from them. Furthermore, each data set needs to be presented in a certain way depending on what it is used for. Planning how the data will be presented is essential before appropriately processing raw data.
First, a question for which an answer is desired must be clearly defined. The more detailed the question is, the more detailed and clearer the results are. A broad question results in vague answers and results that are hard to interpret. In other words, a well-defined question is crucial for the data to be well-understood later. Once a detailed question is ready, the raw data must be prepared before processing. These days, data are often summarized, organized, and analyzed with statistical packages or graphics software. Data must be prepared in such a way they are properly recognized by the program being used. The present study does not discuss this data preparation process, which involves creating a data frame, creating/changing rows and columns, changing the level of a factor, categorical variable, coding, dummy variables, variable transformation, data transformation, missing value, outlier treatment, and noise removal.
We describe the roles and appropriate use of text, tables, and graphs (graphs, plots, or charts), all of which are commonly used in reports, articles, posters, and presentations. Furthermore, we discuss the issues that must be addressed when presenting various kinds of information, and effective methods of presenting data, which are the end products of research, and of emphasizing specific information.
Data Presentation
Data can be presented in one of the three ways:
–as text;
–in tabular form; or
–in graphical form.
Methods of presentation must be determined according to the data format, the method of analysis to be used, and the information to be emphasized. Inappropriately presented data fail to clearly convey information to readers and reviewers. Even when the same information is being conveyed, different methods of presentation must be employed depending on what specific information is going to be emphasized. A method of presentation must be chosen after carefully weighing the advantages and disadvantages of different methods of presentation. For easy comparison of different methods of presentation, let us look at a table ( Table 1 ) and a line graph ( Fig. 1 ) that present the same information [ 1 ]. If one wishes to compare or introduce two values at a certain time point, it is appropriate to use text or the written language. However, a table is the most appropriate when all information requires equal attention, and it allows readers to selectively look at information of their own interest. Graphs allow readers to understand the overall trend in data, and intuitively understand the comparison results between two groups. One thing to always bear in mind regardless of what method is used, however, is the simplicity of presentation.

Variable | Group | Baseline | After drug | 1 min | 3 min | 5 min |
---|---|---|---|---|---|---|
SBP | C | 135.1 ± 13.4 | 139.2 ± 17.1 | 186.0 ± 26.6 | 160.1 ± 23.2 | 140.7 ± 18.3 |
D | 135.4 ± 23.8 | 131.9 ± 13.5 | 165.2 ± 16.2 | 127.9 ± 17.5 | 108.4 ± 12.6 | |
DBP | C | 79.7 ± 9.8 | 79.4 ± 15.8 | 104.8 ± 14.9 | 87.9 ± 15.5 | 78.9 ± 11.6 |
D | 76.7 ± 8.3 | 78.4 ± 6.3 | 97.0 ± 14.5 | 74.1 ± 8.3 | 66.5 ± 7.2 | |
MBP | C | 100.3 ± 11.9 | 103.5 ± 16.8 | 137.2 ± 18.3 | 116.9 ± 16.2 | 103.9 ± 13.3 |
D | 97.7 ± 14.9 | 98.1 ± 8.7 | 123.4 ± 13.8 | 95.4 ± 11.7 | 83.4 ± 8.4 |
Values are expressed as mean ± SD. Group C: normal saline, Group D: dexmedetomidine. SBP: systolic blood pressure, DBP: diastolic blood pressure, MBP: mean blood pressure, HR: heart rate. * P < 0.05 indicates a significant increase in each group, compared with the baseline values. † P < 0.05 indicates a significant decrease noted in Group D, compared with the baseline values. ‡ P < 0.05 indicates a significant difference between the groups.
Text presentation
Text is the main method of conveying information as it is used to explain results and trends, and provide contextual information. Data are fundamentally presented in paragraphs or sentences. Text can be used to provide interpretation or emphasize certain data. If quantitative information to be conveyed consists of one or two numbers, it is more appropriate to use written language than tables or graphs. For instance, information about the incidence rates of delirium following anesthesia in 2016–2017 can be presented with the use of a few numbers: “The incidence rate of delirium following anesthesia was 11% in 2016 and 15% in 2017; no significant difference of incidence rates was found between the two years.” If this information were to be presented in a graph or a table, it would occupy an unnecessarily large space on the page, without enhancing the readers' understanding of the data. If more data are to be presented, or other information such as that regarding data trends are to be conveyed, a table or a graph would be more appropriate. By nature, data take longer to read when presented as texts and when the main text includes a long list of information, readers and reviewers may have difficulties in understanding the information.
Table presentation
Tables, which convey information that has been converted into words or numbers in rows and columns, have been used for nearly 2,000 years. Anyone with a sufficient level of literacy can easily understand the information presented in a table. Tables are the most appropriate for presenting individual information, and can present both quantitative and qualitative information. Examples of qualitative information are the level of sedation [ 2 ], statistical methods/functions [ 3 , 4 ], and intubation conditions [ 5 ].
The strength of tables is that they can accurately present information that cannot be presented with a graph. A number such as “132.145852” can be accurately expressed in a table. Another strength is that information with different units can be presented together. For instance, blood pressure, heart rate, number of drugs administered, and anesthesia time can be presented together in one table. Finally, tables are useful for summarizing and comparing quantitative information of different variables. However, the interpretation of information takes longer in tables than in graphs, and tables are not appropriate for studying data trends. Furthermore, since all data are of equal importance in a table, it is not easy to identify and selectively choose the information required.
For a general guideline for creating tables, refer to the journal submission requirements 1) .
Heat maps for better visualization of information than tables
Heat maps help to further visualize the information presented in a table by applying colors to the background of cells. By adjusting the colors or color saturation, information is conveyed in a more visible manner, and readers can quickly identify the information of interest ( Table 2 ). Software such as Excel (in Microsoft Office, Microsoft, WA, USA) have features that enable easy creation of heat maps through the options available on the “conditional formatting” menu.
Example of a regular table | Example of a heat map | ||||||
---|---|---|---|---|---|---|---|
SBP | DBP | MBP | HR | SBP | DBP | MBP | HR |
128 | 66 | 87 | 87 | 128 | 66 | 87 | 87 |
125 | 43 | 70 | 85 | 125 | 43 | 70 | 85 |
114 | 52 | 68 | 103 | 114 | 52 | 68 | 103 |
111 | 44 | 66 | 79 | 111 | 44 | 66 | 79 |
139 | 61 | 81 | 90 | 139 | 61 | 81 | 90 |
103 | 44 | 61 | 96 | 103 | 44 | 61 | 96 |
94 | 47 | 61 | 83 | 94 | 47 | 61 | 83 |
All numbers were created by the author. SBP: systolic blood pressure, DBP: diastolic blood pressure, MBP: mean blood pressure, HR: heart rate.
Graph presentation
Whereas tables can be used for presenting all the information, graphs simplify complex information by using images and emphasizing data patterns or trends, and are useful for summarizing, explaining, or exploring quantitative data. While graphs are effective for presenting large amounts of data, they can be used in place of tables to present small sets of data. A graph format that best presents information must be chosen so that readers and reviewers can easily understand the information. In the following, we describe frequently used graph formats and the types of data that are appropriately presented with each format with examples.
Scatter plot
Scatter plots present data on the x - and y -axes and are used to investigate an association between two variables. A point represents each individual or object, and an association between two variables can be studied by analyzing patterns across multiple points. A regression line is added to a graph to determine whether the association between two variables can be explained or not. Fig. 2 illustrates correlations between pain scoring systems that are currently used (PSQ, Pain Sensitivity Questionnaire; PASS, Pain Anxiety Symptoms Scale; PCS, Pain Catastrophizing Scale) and Geop-Pain Questionnaire (GPQ) with the correlation coefficient, R, and regression line indicated on the scatter plot [ 6 ]. If multiple points exist at an identical location as in this example ( Fig. 2 ), the correlation level may not be clear. In this case, a correlation coefficient or regression line can be added to further elucidate the correlation.

Bar graph and histogram
A bar graph is used to indicate and compare values in a discrete category or group, and the frequency or other measurement parameters (i.e. mean). Depending on the number of categories, and the size or complexity of each category, bars may be created vertically or horizontally. The height (or length) of a bar represents the amount of information in a category. Bar graphs are flexible, and can be used in a grouped or subdivided bar format in cases of two or more data sets in each category. Fig. 3 is a representative example of a vertical bar graph, with the x -axis representing the length of recovery room stay and drug-treated group, and the y -axis representing the visual analog scale (VAS) score. The mean and standard deviation of the VAS scores are expressed as whiskers on the bars ( Fig. 3 ) [ 7 ].

By comparing the endpoints of bars, one can identify the largest and the smallest categories, and understand gradual differences between each category. It is advised to start the x - and y -axes from 0. Illustration of comparison results in the x - and y -axes that do not start from 0 can deceive readers' eyes and lead to overrepresentation of the results.
One form of vertical bar graph is the stacked vertical bar graph. A stack vertical bar graph is used to compare the sum of each category, and analyze parts of a category. While stacked vertical bar graphs are excellent from the aspect of visualization, they do not have a reference line, making comparison of parts of various categories challenging ( Fig. 4 ) [ 8 ].

A pie chart, which is used to represent nominal data (in other words, data classified in different categories), visually represents a distribution of categories. It is generally the most appropriate format for representing information grouped into a small number of categories. It is also used for data that have no other way of being represented aside from a table (i.e. frequency table). Fig. 5 illustrates the distribution of regular waste from operation rooms by their weight [ 8 ]. A pie chart is also commonly used to illustrate the number of votes each candidate won in an election.

Line plot with whiskers
A line plot is useful for representing time-series data such as monthly precipitation and yearly unemployment rates; in other words, it is used to study variables that are observed over time. Line graphs are especially useful for studying patterns and trends across data that include climatic influence, large changes or turning points, and are also appropriate for representing not only time-series data, but also data measured over the progression of a continuous variable such as distance. As can be seen in Fig. 1 , mean and standard deviation of systolic blood pressure are indicated for each time point, which enables readers to easily understand changes of systolic pressure over time [ 1 ]. If data are collected at a regular interval, values in between the measurements can be estimated. In a line graph, the x-axis represents the continuous variable, while the y-axis represents the scale and measurement values. It is also useful to represent multiple data sets on a single line graph to compare and analyze patterns across different data sets.
Box and whisker chart
A box and whisker chart does not make any assumptions about the underlying statistical distribution, and represents variations in samples of a population; therefore, it is appropriate for representing nonparametric data. AA box and whisker chart consists of boxes that represent interquartile range (one to three), the median and the mean of the data, and whiskers presented as lines outside of the boxes. Whiskers can be used to present the largest and smallest values in a set of data or only a part of the data (i.e. 95% of all the data). Data that are excluded from the data set are presented as individual points and are called outliers. The spacing at both ends of the box indicates dispersion in the data. The relative location of the median demonstrated within the box indicates skewness ( Fig. 6 ). The box and whisker chart provided as an example represents calculated volumes of an anesthetic, desflurane, consumed over the course of the observation period ( Fig. 7 ) [ 9 ].

Three-dimensional effects
Most of the recently introduced statistical packages and graphics software have the three-dimensional (3D) effect feature. The 3D effects can add depth and perspective to a graph. However, since they may make reading and interpreting data more difficult, they must only be used after careful consideration. The application of 3D effects on a pie chart makes distinguishing the size of each slice difficult. Even if slices are of similar sizes, slices farther from the front of the pie chart may appear smaller than the slices closer to the front ( Fig. 8 ).

Drawing a graph: example
Finally, we explain how to create a graph by using a line graph as an example ( Fig. 9 ). In Fig. 9 , the mean values of arterial pressure were randomly produced and assumed to have been measured on an hourly basis. In many graphs, the x- and y-axes meet at the zero point ( Fig. 9A ). In this case, information regarding the mean and standard deviation of mean arterial pressure measurements corresponding to t = 0 cannot be conveyed as the values overlap with the y-axis. The data can be clearly exposed by separating the zero point ( Fig. 9B ). In Fig. 9B , the mean and standard deviation of different groups overlap and cannot be clearly distinguished from each other. Separating the data sets and presenting standard deviations in a single direction prevents overlapping and, therefore, reduces the visual inconvenience. Doing so also reduces the excessive number of ticks on the y-axis, increasing the legibility of the graph ( Fig. 9C ). In the last graph, different shapes were used for the lines connecting different time points to further allow the data to be distinguished, and the y-axis was shortened to get rid of the unnecessary empty space present in the previous graphs ( Fig. 9D ). A graph can be made easier to interpret by assigning each group to a different color, changing the shape of a point, or including graphs of different formats [ 10 ]. The use of random settings for the scale in a graph may lead to inappropriate presentation or presentation of data that can deceive readers' eyes ( Fig. 10 ).

Owing to the lack of space, we could not discuss all types of graphs, but have focused on describing graphs that are frequently used in scholarly articles. We have summarized the commonly used types of graphs according to the method of data analysis in Table 3 . For general guidelines on graph designs, please refer to the journal submission requirements 2) .
Analysis | Subgroup | Number of variables | Type |
---|---|---|---|
Comparison | Among items | Two per items | Variable width column chart |
One per item | Bar/column chart | ||
Over time | Many periods | Circular area/line chart | |
Few periods | Column/line chart | ||
Relationship | Two | Scatter chart | |
Three | Bubble chart | ||
Distribution | Single | Column/line histogram | |
Two | Scatter chart | ||
Three | Three-dimensional area chart | ||
Comparison | Changing over time | Only relative differences matter | Stacked 100% column chart |
Relative and absolute differences matter | Stacked column chart | ||
Static | Simple share of total | Pie chart | |
Accumulation | Waterfall chart | ||
Components of components | Stacked 100% column chart with subcomponents |
Conclusions
Text, tables, and graphs are effective communication media that present and convey data and information. They aid readers in understanding the content of research, sustain their interest, and effectively present large quantities of complex information. As journal editors and reviewers will scan through these presentations before reading the entire text, their importance cannot be disregarded. For this reason, authors must pay as close attention to selecting appropriate methods of data presentation as when they were collecting data of good quality and analyzing them. In addition, having a well-established understanding of different methods of data presentation and their appropriate use will enable one to develop the ability to recognize and interpret inappropriately presented data or data presented in such a way that it deceives readers' eyes [ 11 ].
<Appendix>
Output for presentation.
Discovery and communication are the two objectives of data visualization. In the discovery phase, various types of graphs must be tried to understand the rough and overall information the data are conveying. The communication phase is focused on presenting the discovered information in a summarized form. During this phase, it is necessary to polish images including graphs, pictures, and videos, and consider the fact that the images may look different when printed than how appear on a computer screen. In this appendix, we discuss important concepts that one must be familiar with to print graphs appropriately.
The KJA asks that pictures and images meet the following requirement before submission 3)
“Figures and photographs should be submitted as ‘TIFF’ files. Submit files of figures and photographs separately from the text of the paper. Width of figure should be 84 mm (one column). Contrast of photos or graphs should be at least 600 dpi. Contrast of line drawings should be at least 1,200 dpi. The Powerpoint file (ppt, pptx) is also acceptable.”
Unfortunately, without sufficient knowledge of computer graphics, it is not easy to understand the submission requirement above. Therefore, it is necessary to develop an understanding of image resolution, image format (bitmap and vector images), and the corresponding file specifications.
Resolution is often mentioned to describe the quality of images containing graphs or CT/MRI scans, and video files. The higher the resolution, the clearer and closer to reality the image is, while the opposite is true for low resolutions. The most representative unit used to describe a resolution is “dpi” (dots per inch): this literally translates to the number of dots required to constitute 1 inch. The greater the number of dots, the higher the resolution. The KJA submission requirements recommend 600 dpi for images, and 1,200 dpi 4) for graphs. In other words, resolutions in which 600 or 1,200 dots constitute one inch are required for submission.
There are requirements for the horizontal length of an image in addition to the resolution requirements. While there are no requirements for the vertical length of an image, it must not exceed the vertical length of a page. The width of a column on one side of a printed page is 84 mm, or 3.3 inches (84/25.4 mm ≒ 3.3 inches). Therefore, a graph must have a resolution in which 1,200 dots constitute 1 inch, and have a width of 3.3 inches.
Bitmap and Vector
Methods of image construction are important. Bitmap images can be considered as images drawn on section paper. Enlarging the image will enlarge the picture along with the grid, resulting in a lower resolution; in other words, aliasing occurs. On the other hand, reducing the size of the image will reduce the size of the picture, while increasing the resolution. In other words, resolution and the size of an image are inversely proportionate to one another in bitmap images, and it is a drawback of bitmap images that resolution must be considered when adjusting the size of an image. To enlarge an image while maintaining the same resolution, the size and resolution of the image must be determined before saving the image. An image that has already been created cannot avoid changes to its resolution according to changes in size. Enlarging an image while maintaining the same resolution will increase the number of horizontal and vertical dots, ultimately increasing the number of pixels 5) of the image, and the file size. In other words, the file size of a bitmap image is affected by the size and resolution of the image (file extensions include JPG [JPEG] 6) , PNG 7) , GIF 8) , and TIF [TIFF] 9) . To avoid this complexity, the width of an image can be set to 4 inches and its resolution to 900 dpi to satisfy the submission requirements of most journals [ 12 ].
Vector images overcome the shortcomings of bitmap images. Vector images are created based on mathematical operations of line segments and areas between different points, and are not affected by aliasing or pixelation. Furthermore, they result in a smaller file size that is not affected by the size of the image. They are commonly used for drawings and illustrations (file extensions include EPS 10) , CGM 11) , and SVG 12) ).
Finally, the PDF 13) is a file format developed by Adobe Systems (Adobe Systems, CA, USA) for electronic documents, and can contain general documents, text, drawings, images, and fonts. They can also contain bitmap and vector images. While vector images are used by researchers when working in Powerpoint, they are saved as 960 × 720 dots when saved in TIFF format in Powerpoint. This results in a resolution that is inappropriate for printing on a paper medium. To save high-resolution bitmap images, the image must be saved as a PDF file instead of a TIFF, and the saved PDF file must be imported into an imaging processing program such as Photoshop™(Adobe Systems, CA, USA) to be saved in TIFF format [ 12 ].
1) Instructions to authors in KJA; section 5-(9) Table; https://ekja.org/index.php?body=instruction
2) Instructions to Authors in KJA; section 6-1)-(10) Figures and illustrations in Manuscript preparation; https://ekja.org/index.php?body=instruction
3) Instructions to Authors in KJA; section 6-1)-(10) Figures and illustrations in Manuscript preparation; https://ekja.org/index.php?body=instruction
4) Resolution; in KJA, it is represented by “contrast.”
5) Pixel is a minimum unit of an image and contains information of a dot and color. It is derived by multiplying the number of vertical and horizontal dots regardless of image size. For example, Full High Definition (FHD) monitor has 1920 × 1080 dots ≒ 2.07 million pixel.
6) Joint Photographic Experts Group.
7) Portable Network Graphics.
8) Graphics Interchange Format
9) Tagged Image File Format; TIFF
10) Encapsulated PostScript.
11) Computer Graphics Metafile.
12) Scalable Vector Graphics.
13) Portable Document Format.
- For Individuals
- For Businesses
- For Universities
- For Governments
- Online Degrees
- Find your New Career
- Join for Free
Data Science vs Data Analytics: Which Should You Choose?
Data scientists primarily use data science in their careers, while data analysts use data analytics. We will explore how these roles differ regarding skill sets, responsibilities, and career outlook.
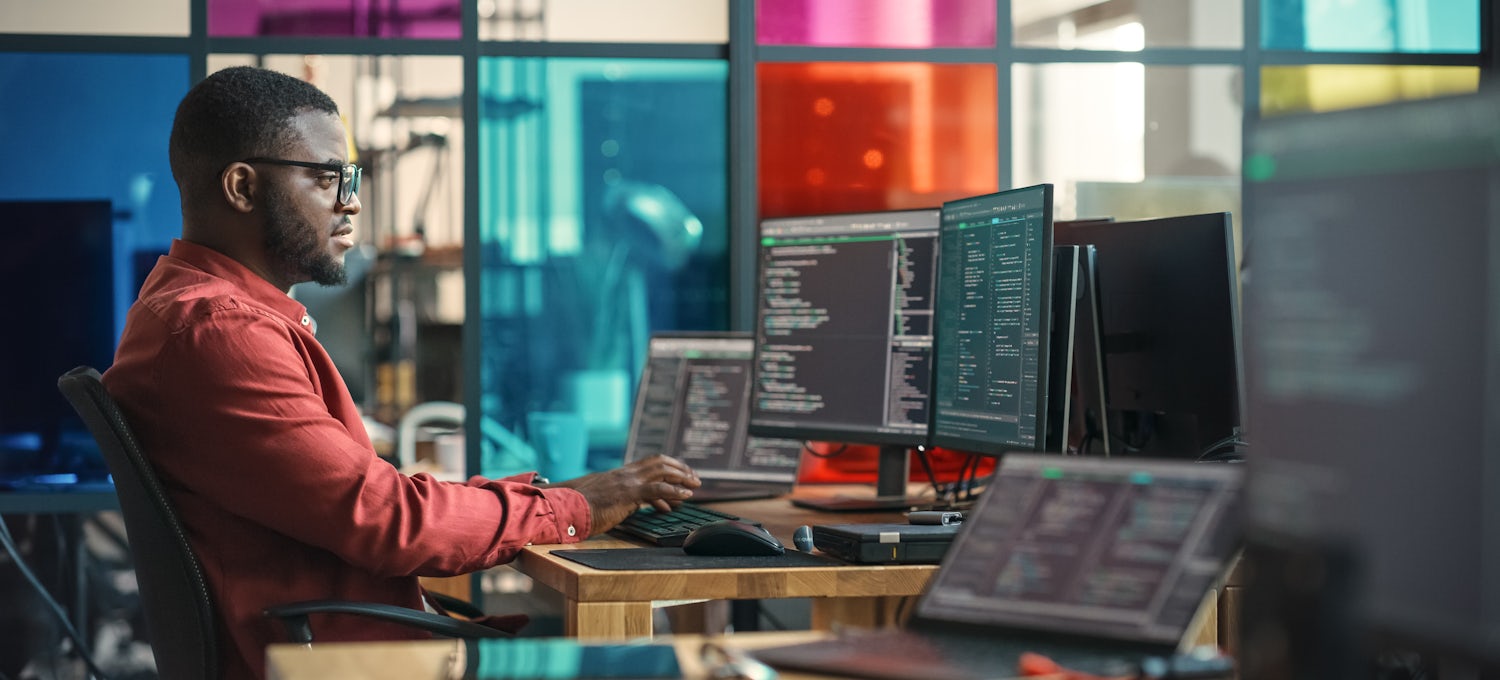
Data science and data analytics are two closely related fields, but there are key differences that set them apart. Data scientists primarily use data science in their careers, while data analysts use data analytics. To illustrate the differences and similarities between data science and data analytics, we will explore how these roles differ regarding skill sets, responsibilities, and career outlook.
A quick guide to data science and data analytics roles
You will find similarities and discrepancies when exploring job opportunities related to data science and data analytics. While there is some overlap between the two positions, ultimately, they serve different purposes for their organisations:
Data scientist: Data scientists rely on machine learning algorithms to automate processes, such as data modelling, to predict future outcomes and deliver insights for their organisations.
Data analyst: Data analysts sort and analyse data to spot trends and solve specific problems, allowing companies to optimise their processes based on valuable insights.
Data scientist | Data analyst |
---|---|
Machine learning, predictive modelling, data visualisation | Data visualisation, data analytics |
Knowledge of common programming languages such as SQL, R, and Python, as well as big data platforms and cloud tools | Knowledge of common programming languages such as SQL, R, and data analytics tools |
Advanced knowledge of maths and statistics | Strong knowledge of maths and statistics |
Bachelor’s or master's degree in computer science, mathematics, data science, or similar field | Bachelor's degree in statistics, data analytics, business analytics, or similar field |
Average annual salary ₹23,04,872 | Average annual salary ₹18,69,606 |
What does a data scientist do?
As a data scientist, it would be your job to organise large raw data sets and design machine learning algorithms to process data and build predictive models. Using data visualisation tools, you would create graphs, charts, maps, and other visuals to illustrate trends and patterns in more easily understood forms for non-technical audiences. In an increasingly technical world, data scientists are precious to organisations by allowing them to make data-driven decisions.
Data science skills
Data scientists have a very diverse and advanced skill set. With a foundation in computer science, statistics, and business practices, data scientists are highly skilled in many technical areas. Here are some of the primary skills needed to succeed as a data scientist:
Machine learning
Data visualisation and reporting
Computer programming
Artificial intelligence
Predictive modelling
What does a data analyst do?
As a data analyst, you would gather, clean, sort, and interpret data to solve complex problems. Data analysts use many of the same programming languages as data scientists, such as SQL, Python, and R. You would use these tools to spot trends and use insights to make better business decisions. Data analysts are highly skilled in working with databases and creating visualisations to demonstrate their findings.
Data analyst skills
Like their data science counterparts, data analysts are highly skilled and bring a depth of knowledge to their company. As such, you need to communicate your findings in this role effectively. While an analyst's knowledge isn’t quite as advanced as a data scientist's in computer programming and mathematics, you would still have an impressively diverse and valuable skill set, including:
SQL (Structured Query Language)
Data visualisation
Microsoft Excel
Software as a service (SAS)
Problem-solving and communication skills
Educational requirements
There are varying educational requirements for careers in data science and data analytics. Although post-secondary education is expected from an employer, hiring managers in both these fields are most concerned about whether or not you have the necessary skills, regardless of what your studies were concentrated on. Because of this, obtaining a degree explicitly focused on data science or analytics is an option as courses that teach you other related technical concepts.
Data science education
Because data science is a relatively new field, many data scientists don’t have a degree in data science. Instead, computer science, mathematics, and statistics are common degree courses. To progress in your career as a data scientist, getting an advanced degree is valuable as it’s common for data scientists to obtain a master’s degree or even a PhD. However, more institutions are adding data science degrees and courses to their curriculum with the rise in popularity.
Data analytics education
Like their data science counterparts, data analysts have various options for what they may choose to study. The exact computer science, mathematics, and statistics majors are all popular choices. Some institutions offer specified degree courses, such as business analytics, but any related degree will generally suffice if you have the necessary skills mentioned earlier.
According to information from Salary Expert, 47 percent of data analysts in India hold a bachelor's degree, while 40 percent have earned their master’s. Six percent have completed a PhD, and 5 percent have only completed secondary school [ 2 ]. Regardless of your educational background, you typically have opportunities if you are skilled in data analytics.
Salary and job outlook
A career in data science or analytics will create opportunities to earn an above-average income and see significant job growth in the coming years.
The average data scientist salary in India is ₹23,04,872 [ 1 ]. Senior-level positions, which can typically be reached within eight years, come with an increase in salary with average earnings of ₹29,04,781. Based on Salary Expert's data, it anticipates a 30 percent increase in salary over the next five years, which would bring the average estimated salary up to ₹29,89,603 by 2029.
While the earning potential isn’t as high for data analysts as data scientists, analysts still have a powerful outlook and can expect similar growth. According to Salary Expert, the average salary for a data analyst is ₹18,69,606, with senior-level analysts earning ₹23,54,293 [ 2 ]. The average salary is estimated to grow to reach ₹24,25,028 by 2029.
Things to consider
Other factors are worth considering beyond education and salary when pursuing a career in data science or analytics.
Domain knowledge can be a great asset to have. If there’s a specific field you know well, combining your domain-specific knowledge with your ability to work with data can set you apart from other candidates.
To have long-term success in data science or data analytics, it’s important for you to constantly stay up to date on the latest tools and programs available. Since this space constantly evolves, committing to lifelong learning and keeping up with the latest advancements will help you progress in your career.
Many Coursera courses can help start your data science or data analytics career. Coursera offers various courses covering all the skills needed in data science and analytics.
If you’re new to data science, consider enrolling in IBM’s Introduction to Data Science Specialisation . In this course, you’ll have the opportunity to gain familiarity with many popular data science tools and get an introduction to machine learning concepts. The University of Michigan’s Applied Data Science with Python Specialisation is an intermediate-level course that can help you further develop your skills in the popular programming language Python.
For aspiring data analysts, bolster your resume with a Google Data Analytics Professional Certificate . Courses in this program will help you learn SQL, data visualisation, data analysis, and many other valuable skills to prepare you for a career in data analytics.
Article sources
Salary Expert India. “ Data Scientist Salary and Education , https://www.salaryexpert.com/salary/job/data-scientist/india.” Accessed February 13, 2024.
Salary Expert India. “ Data Analyst Salary and Education, https://www.salaryexpert.com/salary/job/data-analyst/india.” Accessed February 13, 2024.
Keep reading
Coursera staff.
Editorial Team
Coursera’s editorial team is comprised of highly experienced professional editors, writers, and fact...
This content has been made available for informational purposes only. Learners are advised to conduct additional research to ensure that courses and other credentials pursued meet their personal, professional, and financial goals.
The Community
Modern analyst blog, community blog.
- Member Profiles
Networking Opportunities
Community spotlight, business analysis glossary, articles listing, business analyst humor, self assessment.
- Training Courses
- Organizations
- Resume Writing Tips
- Interview Questions
Let Us Help Your Business
Advertise with us, rss feeds & syndication, privacy policy.

The Community Blog for Business Analysts
6 Differences Between Data Exploration and Data Presentation
There are big differences between data exploration versus data presentation. And you need to be aware of these differences as you're creating data stories and data presentations. Let’s start by defining our terms:
Data exploration means the deep-dive analysis of data in search of new insights.
Data presentation means the delivery of data insights to an audience in a form that makes clear the implications.
Your toolbox for data exploration tools is flush with technology solutions such as Tableau, PowerBI, Looker, and Qlik. "Visual analytics" tools give analysts a super-powered version of Excel for dicing data to facilitate the search for valuable insights. Flexibility and breadth of features is critical; the user needs to handle lots of data sources and doesn’t know in which direction she will go with the analysis.
Data presentation is a different class of problem with distinct use cases, goals, and audience needs. Think about the incredible data stories delivered by the The Upshot , Fivethirtyeight , and Bloomberg . These data journalists often demonstrate data presentation at its finest, complete with guided storytelling, compelling visuals, and thoughtful text descriptions. When compared to these examples, it becomes obvious that the best efforts by a data exploration tool cannot deliver high-quality data presentation.

You need a specialized solution if you really want to communicate data in ways that engage your audience. To understand the differences between data exploration and data presentation tools, let me offer six key ways that the activities are fundamentally different.
1. Audience — Who is the data for?
For data exploration , the primary audience is the data analyst herself. She is the person who is both manipulating the data and seeing the results. She needs to work with tight feedback cycles of defining hypotheses, analyzing data, and visualizing results.
For data presentation , the audience is a separate group of end-users, not the author of the analysis. These end-users are often non-analytical, they are on the front-lines of business decision-making, and may difficulty connecting the dots between an analysis and the implications for their job.

2. Message — What do you want to say?
Data exploration is about the journey to find a message in your data. The analyst is trying to put together the pieces of a puzzle.
Data presentation is about sharing the solved puzzle with people who can take action on the insights. Authors of data presentations need to guide an audience through the content with a purpose and point of view.

3. Explanation — What does the data mean?
For the analysts using data exploration tools, the meaning of their analysis can be self-evident. A 1% jump in your conversion metric may represent a big change that changes your marketing tactics. The important challenge for the analysts is to answer why is this happening.
Data presentations carry a heavier burden in explaining the results of analysis. When the audience isn’t as familiar with the data, the data presentation author needs to start with more basic descriptions and context. How do we measure the conversion metric? Is a 1% change a big deal or not? What is the business impact of this change?

4. Visualizations — How do I show the data?
The visualizations for data exploration need to be easy to create and may often show multiple dimensions to unearth complex patterns.
For data presentation , it is important that visualizations be simple and intuitive. The audience doesn’t have the patience to decipher the meaning of a chart. I used to love presenting data in treemaps but found that as a visualization it could seldom stand-alone without a two-minute tutorial to teach new users how to read the content.

5. Goal — What should I do about the insights?
The goal of data exploration is often to ask a better question. The process of finding better questions gets to new insights and a better understanding of how your business works.
Data presentations are about guiding decision-makers to make smarter choices. Much of the learning (through data exploration) should be done, leaving the equally difficult task of communicating the insights and the actions that should result.
In all these ways, data exploration and data presentation are different beasts. This is why we’ve chosen to focus on building the best possible data presentation tool, Juicebox.
6. Interactions — How are data insights created and shared?
Data exploration can be a lonely endeavor: Analysts work on their own to gather data, connect data across silos, and dig into the data to find insights. Data exploration is often a solitary activity that only connects with other people when insights are found and need to be shared. That is, when…
Data presentation is a collaborative, social activity. The value emerges when insights found in data are shared with people who understand the context of the business. The dialogue that emerges is the point, not a failure of the analysis.
Finding the Middle Ground: Data Storytelling
There is something between the extreme ends of data exploration and data presentation. We believe data storytelling lies in this intersection. Data stories aren’t entirely about “telling”, nor are they in the wilderness of “finding”. It is the opportunity to explain the data in a guided, narrative way where message meets exploration.

While there are tools for exploration (e.g. Tableau) and tools for presentation (e.g. PowerPoint), it is only recently that you’ve had the change to bring both together in one solution.
Zach Gemignani ( [email protected] )
CEO, co-founder, author at Juice Analytics
www.juiceanalytics.com
Related Articles
Modern analyst blog latests.
Three Audacious Goals for Business Analysts in 2013
Hey IIBA, What’s Up With That?
BA ABCs: “C” is for Class Diagram
Blog information.
» What is the Community Blog and what are the Benefits of Contributing ?
» Review our Blog Posting Guidelines .
» I am looking for the original Modern Analyst blog posts .
Blog Entry Categories
Roles and Titles
- Business Analyst
- Business Process Analyst
- IT Business Analyst
- Requirements Engineer
- Business Systems Analyst
- Systems Analyst
- Data Analyst
Career Resources
- Interview Tips
- Salary Information
- Directory of Links
Community Resources
- Project Members
Advertising Opportunities | Contact Us | Privacy Policy
INTERACTION DESIGN: beyond human-computer interaction, 3rd Edition by
Get full access to INTERACTION DESIGN: beyond human-computer interaction, 3rd Edition and 60K+ other titles, with a free 10-day trial of O'Reilly.
There are also live events, courses curated by job role, and more.
DATA ANALYSIS, INTERPRETATION, AND PRESENTATION
8.1 introduction.
- 8.2 Qualitative and Quantitative
- 8.3 Simple Quantitative Analysis
- 8.4 Simple Qualitative Analysis
- 8.5 Tools to Support Data Analysis
- 8.6 Using Theoretical Frameworks
- 8.7 Presenting the Findings
The main aims of this chapter are to:
- Discuss the difference between qualitative and quantitative data and analysis.
- Enable you to analyze data gathered from questionnaires.
- Enable you to analyze data gathered from interviews.
- Enable you to analyze data gathered from observation studies.
- Make you aware of software packages that are available to help your analysis.
- Identify some of the common pitfalls in data analysis, interpretation, and presentation.
- Enable you to be able to interpret and present your findings in a meaningful and appropriate manner.
The kind of analysis that can be performed on a set of data will be influenced by the goals identified at the outset, and the data actually gathered. Broadly speaking, you may take a qualitative analysis approach or a quantitative analysis approach, or a combination of qualitative and quantitative. The last of these is very common as it provides a more comprehensive account of the behavior being observed or performance being measured.
Most analysis, whether it is quantitative or qualitative, begins with initial reactions or observations from the data. This might involve identifying patterns or calculating simple numerical values such ...
Get INTERACTION DESIGN: beyond human-computer interaction, 3rd Edition now with the O’Reilly learning platform.
O’Reilly members experience books, live events, courses curated by job role, and more from O’Reilly and nearly 200 top publishers.
Don’t leave empty-handed
Get Mark Richards’s Software Architecture Patterns ebook to better understand how to design components—and how they should interact.
It’s yours, free.
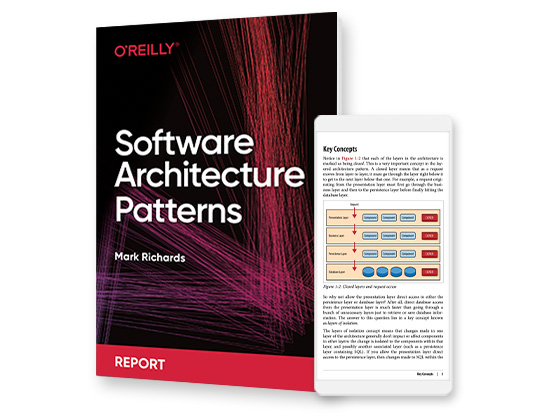
Check it out now on O’Reilly
Dive in for free with a 10-day trial of the O’Reilly learning platform—then explore all the other resources our members count on to build skills and solve problems every day.
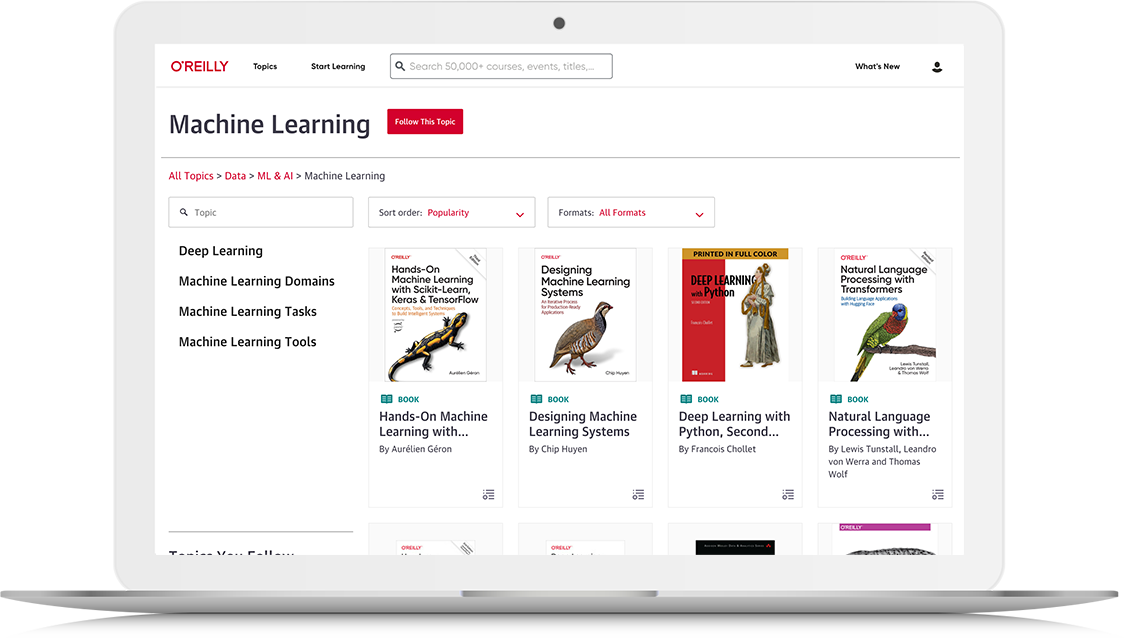
- Business Essentials
- Leadership & Management
- Credential of Leadership, Impact, and Management in Business (CLIMB)
- Entrepreneurship & Innovation
- Digital Transformation
- Finance & Accounting
- Business in Society
- For Organizations
- Support Portal
- Media Coverage
- Founding Donors
- Leadership Team
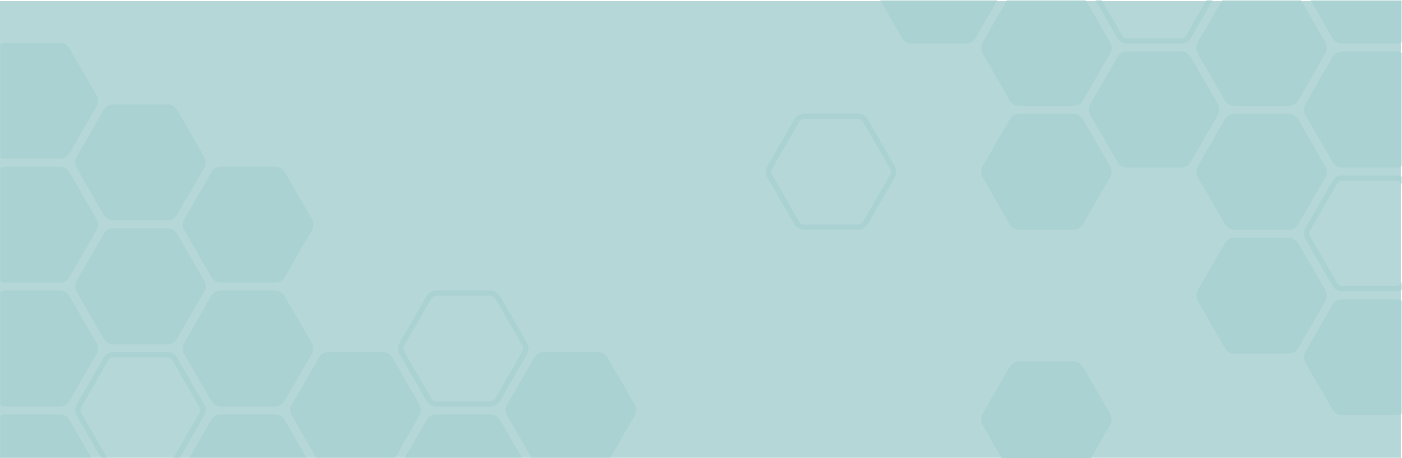
- Harvard Business School →
- HBS Online →
- Business Insights →
Business Insights
Harvard Business School Online's Business Insights Blog provides the career insights you need to achieve your goals and gain confidence in your business skills.
- Career Development
- Communication
- Decision-Making
- Earning Your MBA
- Negotiation
- News & Events
- Productivity
- Staff Spotlight
- Student Profiles
- Work-Life Balance
- AI Essentials for Business
- Alternative Investments
- Business Analytics
- Business Strategy
- Business and Climate Change
- Creating Brand Value
- Design Thinking and Innovation
- Digital Marketing Strategy
- Disruptive Strategy
- Economics for Managers
- Entrepreneurship Essentials
- Financial Accounting
- Global Business
- Launching Tech Ventures
- Leadership Principles
- Leadership, Ethics, and Corporate Accountability
- Leading Change and Organizational Renewal
- Leading with Finance
- Management Essentials
- Negotiation Mastery
- Organizational Leadership
- Power and Influence for Positive Impact
- Strategy Execution
- Sustainable Business Strategy
- Sustainable Investing
- Winning with Digital Platforms
What's the Difference Between Data Analytics & Data Science?
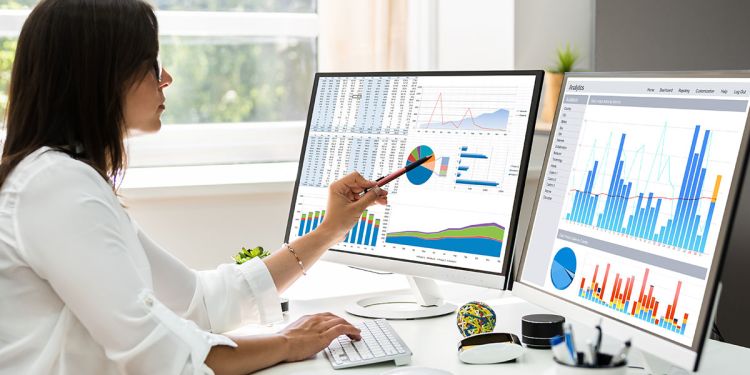
- 05 Jan 2021
If you were to gather the world’s foremost business leaders and ask them to identify the biggest difference between doing business in the 20th century versus the 21st, a large percentage would likely say one word: data.
Since the turn of the century, data’s proliferation in business has grown with the emergence of social media, smartphones, the internet of things (IoT), and other technological advances. By some estimates , if you were to take all of the data generated by humanity in 2020 and divide it among the world’s population, you’d find that each person created 1.7 megabytes of data every second . In fact, it’s estimated that more than 90 percent of the total data created by humans has been generated in just the last two years.
This exponential growth has led organizations of all sizes to wonder how they can leverage information to realize business benefits. Meanwhile, individuals are increasingly seeking to develop their data skills to make their resumes stand out , advance their careers, and gain job security.
“In this world of big data, basic data literacy—the ability to analyze, interpret, and even question data—is an increasingly valuable skill,” says Harvard Business School Professor Jan Hammond in the online course Business Analytics .
If you’re new to the world of data and want to bolster your skills , two terms you’re likely to encounter are “data analytics” and “ data science .” While these terms are related, they refer to different things. Below is an overview of what each word means and how it applies in business.
Access your free e-book today.
Data Analytics vs. Data Science
What is data analytics.
Data analytics refers to the process and practice of analyzing data to answer questions, extract insights, and identify trends. This is done using an array of tools, techniques, and frameworks that vary depending on the type of analysis being conducted.
The four major types of analytics include:
- Descriptive analytics , which looks at data to examine, understand, and describe something that’s already happened.
- Diagnostic analytics , which goes deeper than descriptive analytics by seeking to understand the why behind what happened.
- Predictive analytics , which relies on historical data, past trends, and assumptions to answer questions about what will happen in the future.
- Prescriptive analytics , which aims to identify specific actions that an individual or organization should take to reach future targets or goals.
Applying data analytics tools and methodologies in a business setting is typically referred to as business analytics . The main goal of business analytics is to extract meaningful insights from data that an organization can use to inform its strategy and, ultimately, reach its objectives.
Business analytics can be leveraged in a variety of ways. Here are a few examples to consider:
- Budgeting and forecasting : By assessing a company’s historical revenue, sales, and costs data alongside its goals for future growth, an analyst can identify the budget and investments required to make those goals a reality.
- Risk management : By understanding the likelihood of certain business risks occurring—and their associated costs—an analyst can make cost-effective recommendations to help mitigate them.
- Marketing and sales : By understanding key metrics, such as lead-to-customer conversion rate, a marketing analyst can identify the number of leads their efforts must generate to fill the sales pipeline.
- Product development (or research and development) : By understanding how customers have reacted to product features in the past, an analyst can help guide product development, design, and user experience in the future.
What Is Data Science?
Whereas data analytics is primarily focused on understanding datasets and gleaning insights that can be turned into actions, data science is centered on building, cleaning, and organizing datasets. Data scientists create and leverage algorithms, statistical models, and their own custom analyses to collect and shape raw data into something that can be more easily understood.
“From the first steps of determining the quality of a data source to determining the success of an algorithm, critical thinking is at the heart of every decision data scientists—and those who work with them—make,” says Professor Dustin Tingley in the Harvard Online course Data Science Principles . “Data science is a discipline that’s built on a foundation of critical thinking.”
Data scientists lay the groundwork for all of the analyses an organization performs. They do this by performing key functions, including:
- Data wrangling : The process of cleaning and organizing data to be more readily used.
- Statistical modeling : The process of running data through different models—such as regression, classification, and clustering models, among others—to identify relationships between variables and gain insight from the numbers.
- Programming : The process of writing computer programs and algorithms in a variety of languages—such as R, Python, and SQL—that can be used to analyze large datasets more efficiently than through manual analysis.
While it’s unlikely you’ll need to perform any of these duties in your job unless you’re specifically hired as a data scientist, data science still holds value for business professionals. Familiarizing yourself with the concepts, terminology, and techniques used by data scientists on your team can empower you to better communicate with these important professionals and give you a firmer understanding of what insights are and aren’t possible to glean from the data.
Additionally, a proficiency in key data science skills can enable you to assess and draw insights from your organization’s data—increasing the value you bring to your organization while reducing your reliance on others. Developing your data science skills can allow you to:
- Identify and avoid mistakes that commonly arise while interpreting datasets, metrics, and visualizations
- Embrace data-driven decision-making and ensure your business decisions are backed by numbers
- Form hypotheses, run experiments, and gather evidence that empowers you to recognize business challenges and solutions
- Understand market size, buyer trends, competition, and opportunities and risks your business faces
The examples above are just a selection of potential applications for data science in business. Many others exist, depending on the specific data being leveraged.

Not an Either/Or Decision
There are key differences between data science and data analytics. The good news is that, unless you plan on going into one of those fields—for example, as a data scientist or data analyst—the differences are relatively small.
For business professionals seeking to increase their understanding of data and how it can be leveraged in their organizations, it’s more important to understand the key concepts, frameworks, and techniques that underlie both fields.
Are you ready to accelerate your career by developing a data mindset that can help inform your business decisions? Download our Beginner’s Guide to Data & Analytics to learn how you can leverage the power of data for professional and organizational success.

About the Author

Data Collection vs. Data Analysis: What’s The Difference?

Data Journal
In the world of data, there are two big steps: data collection and data analysis. They’re super important because they help me understand stuff better. First, I gather data from different sources. Then, I analyze it to make sense of what I’ve collected. It’s like solving a mystery by putting together clues. Mastering both steps helps me make better decisions and understand the world.
What is Data Collection?
Data collection is the process of gathering data on specific things using a good system. It helps answer important questions and see what happens.
For instance, if I’m studying coffee drinkers’ preferences, I might conduct surveys asking questions about their favorite brews or visit coffee shops to observe customer behavior firsthand. This raw data forms the foundation of my analysis.
It’s important to ensure the data I gather is correct, useful, and covers everything. Good data is like a strong base for a house — it keeps everything steady. This helps me trust my analysis later.
I am not going into structured vs. unstructured data collection, it’ll be a separate article.
What is Data Analytics?
Data analytics means examining shaped data to better understand it, discover important information, and help solve research problems.
After collecting raw data, I start making sense of it with data analysis. It’s like building on a foundation. I use different methods and tools to explore, clean, and understand the data.
First, I look at the data for patterns, trends, and connections. This could mean organizing numbers or making graphs. For example, I might use a graph to compare coffee brands people like.
Next, I clean the data to fix any mistakes, copies, or mix-ups. It’s like tidying up before starting work to make sure everything’s right.
Finally, I interpret the data to find insights and make conclusions. This is where I put everything together. For example, I might find that younger people prefer special coffee while older people like classic kinds.
The Difference Between Collection and Analysis
Data collection and data analysis go hand in hand. When I collect data, I keep my analysis goals in mind. This means asking the right questions and gathering the right information. For example, if I want to know about coffee preferences, I won’t collect data about tea.
Similarly, my analysis helps me collect better data. If I find something unexpected, I might change how I collect data to learn more. For instance, if I see a sudden rise in cold brew coffee popularity, I might focus on asking more questions to cold brew drinkers.
Key Differences:
Different sources for collecting data include:
- Gathering fresh data from the internet and other places.
- Using data collected and stored previously.
- Reusing data collected by others.
- Buying data.
The methods for collecting data depend on:
- The problem being studied in the research.
- The design of the research.
- The details collected about the variable.
Different types of data management depend on how the data was collected:
Quantitative Information: Numerical data from surveys or experiments, including details like dates, locations, units, and methods used.
Qualitative Information: Non-numerical data like videos or audio recordings can later be turned into written transcripts.
Working with Your Data: Researchers often have a lot of data that needs summarizing before drawing conclusions. This could include numerical spreadsheets, interview transcripts, or descriptions.
Presenting Your Data: When writing a thesis or report, it’s important to present your data clearly using tables and figures to support your points.
Challenges and Opportunities
Data collection and analysis have problems. When collecting data, I might face low response rates, biased samples, or incomplete data, which can make it hard to understand. But with good planning and attention, I can overcome these challenges.
Similarly, analyzing data isn’t always easy. I might deal with messy data, unclear results, or hard math. But I can handle even tough data with patience, not giving up, and using the right tools.
Even with challenges, both stages let me be creative. I can create interesting survey questions, try new ways of analyzing data, or find cool things in the data. Working with data is like being an artist and a scientist at the same time.
Data collection and analysis are like two parts of a puzzle. One gets the information ready, and the other helps understand it better. Knowing about both, I can use data to help me make choices, fix things, and make progress.
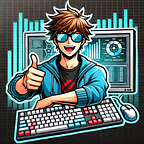
Written by Data Journal
Exploring the secrets of web data through scraping, collection, and proxies. Dive into the art of online data collection for growth and insight.
Text to speech
- Submit a Manuscript
- Advanced search

American Journal of Neuroradiology
Advanced Search
Risk of Hemorrhagic Transformation after Mechanical Thrombectomy without versus with IV Thrombolysis for Acute Ischemic Stroke: A Systematic Review and Meta-analysis of Randomized Clinical Trials
- Find this author on Google Scholar
- Find this author on PubMed
- Search for this author on this site
- ORCID record for Seyed Behnam Jazayeri
- ORCID record for Sherief Ghozy
- ORCID record for Cem Bilgin
- ORCID record for Mohamed Elfil
- ORCID record for Ramanathan Kadirvel
- ORCID record for David F. Kallmes
- Figures & Data
- Info & Metrics
This article requires a subscription to view the full text. If you have a subscription you may use the login form below to view the article. Access to this article can also be purchased.
BACKGROUND: When treating acute ischemic stroke due to large-vessel occlusion, both mechanical thrombectomy and intravenous (IV) thrombolysis carry the risk of intracerebral hemorrhage.
PURPOSE: This study aimed to delve deeper into the risk of intracerebral hemorrhage and its subtypes associated with mechanical thrombectomy with or without IV thrombolysis to contribute to better decision-making in the treatment of acute ischemic stroke due to large-vessel occlusion.
DATA SOURCES: PubMed, EMBASE, and Scopus databases were searched for relevant studies from inception to September 6, 2023.
STUDY SELECTION: The eligibility criteria included randomized clinical trials or post hoc analysis of randomized controlled trials that focused on patients with acute ischemic stroke in the anterior circulation. After screening 4870 retrieved records, we included 9 studies (6 randomized controlled trials and 3 post hoc analyses of randomized controlled trials) with 3241 patients.
DATA ANALYSIS: The interventions compared were mechanical thrombectomy + IV thrombolysis versus mechanical thrombectomy alone, with the outcome of interest being any form of intracerebral hemorrhage and symptomatic intracerebral hemorrhage after intervention. A common definition for symptomatic intracerebral hemorrhage was pooled from various classification systems, and subgroup analyses were performed on the basis of different definitions and anatomic descriptions of hemorrhage. The quality of the studies was assessed using the revised version of Cochrane Risk of Bias 2 assessment tool. Meta-analysis was performed using the random effects model.
DATA SYNTHESIS: Eight studies had some concerns, and 1 study was considered high risk. Overall, the risk of symptomatic intracerebral hemorrhage was comparable between mechanical thrombectomy + IV thrombolysis and mechanical thrombectomy alone (risk ratio, 1.24 [95% CI, 0.89–1.72]; P = .20), with no heterogeneity across studies. Subgroup analysis of symptomatic intracerebral hemorrhage showed a non-significant difference between 2 groups based on the National Institute of Neurological Disorders and Stroke ( P = .3), the Heidelberg Bleeding Classification ( P = .5), the Safe Implementation of Thrombolysis in Stroke-Monitoring Study ( P = .4), and the European Cooperative Acute Stroke Study III ( P = .7) criteria. Subgroup analysis of different anatomic descriptions of intracerebral hemorrhage showed no difference between the 2 groups. Also, we found no difference in the risk of any intracerebral hemorrhage between two groups (risk ratio, 1.10 [95% CI, 1.00–1.21]; P = .052) with no heterogeneity across studies.
LIMITATIONS: There was a potential for performance bias in most studies.
CONCLUSIONS: In this systematic review and meta-analysis, the risk of any intracerebral hemorrhage and symptomatic intracerebral hemorrhage, including its various classifications and anatomic descriptions, was comparable between mechanical thrombectomy + IV thrombolysis and mechanical thrombectomy alone.
- ABBREVIATIONS:
- © 2024 by American Journal of Neuroradiology
Log in using your username and password
In this issue.

- Table of Contents
- Index by author
- Complete Issue (PDF)
Thank you for your interest in spreading the word on American Journal of Neuroradiology.
NOTE: We only request your email address so that the person you are recommending the page to knows that you wanted them to see it, and that it is not junk mail. We do not capture any email address.
Citation Manager Formats
- EndNote (tagged)
- EndNote 8 (xml)
- RefWorks Tagged
- Ref Manager

- Tweet Widget
- Facebook Like
- Google Plus One
Jump to section
- MATERIALS AND METHODS
- CONCLUSIONS
Related Articles
- No related articles found.
- Google Scholar
Cited By...
- No citing articles found.
This article has not yet been cited by articles in journals that are participating in Crossref Cited-by Linking.
More in this TOC Section
- Stent Retriever AssIsted Lysis Technique with Tirofiban: A Potential Bailout Alternative to Angioplasty and Stenting
- ACCESS: ACtive Catheterization for EndovaScular TreatmentS—A First-in-Human, Single-Center, Nonrandomized, Open Clinical Study of the G60 Active Device for Endovascular Neurointerventions
- Double Stent Retriever Technique for Mechanical Thrombectomy: A Systematic Review and Meta-Analysis
Similar Articles
Hearing preservation and quality of life in small to medium sized vestibular schwannomas after a wait and scan approach or stereotactic radiosurgery: a systematic review and meta-analysis
- Published: 09 September 2024
- Volume 166 , article number 361 , ( 2024 )
Cite this article
- Anderson Brito 1 ,
- Jackson Daniel Sousa Silva 2 ,
- Fernando Terry 3 ,
- Anuraag Punukollu 4 ,
- Adam S. Levy 5 ,
- Anna Lydia Machado Silva 6 ,
- Herwin Speckter 1 ,
- Alexis A. Morell 7 ,
- Alejandro Enriquez-Marulanda 3 ,
- Ziev B. Moses 3 ,
- Ricardo J. Komotar 5 &
- Rafael A. Vega 3
The management of vestibular schwannomas (VS) encompasses a choice between conservative “wait-and-scan” (WAS) approach, stereotactic radiosurgery (SRS) or open microsurgical resection. Currently, there is no consensus on the optimal management approach for small to medium sized VS. This study aims to compared outcomes related to hearing in patients with small and medium sized VS who underwent initial treatment with WAS versus SRS.
A systematic review of the available literature was conducted using PubMed/MEDLINE, Embase, and Cochrane up December 08, 2023. Meta-analysis was performed using a random-effect model to calculate mean difference (MD) and relative risk (RR). A leave-one-out analysis was conducted. The risk of bias was assessed via the Risk of Bias in Non-randomized Studies-Interventions (ROBINS-I) and Cochrane Risk of Bias assessment tool (RoB-2). Ultimately, the certainty of evidence was evaluated using the GRADE assessment. The primary outcomes were serviceable hearing, and pure-tone average (PTA). The secondary outcome was the Penn Acoustic Neuroma Quality of Life Scale (PANQOL) total score.
Nine studies were eligible for inclusion, comprising a total of 1,275 patients. Among these, 674 (52.86%) underwent WAS, while 601 patients (47.14%) received SRS. Follow-up duration ranged from two to eight years. The meta-analysis indicated that WAS had a better outcome for serviceable hearing (0.47; 95% CI: 0.32 – 0.68; p < 0.001), as well as for postoperative functional measures including PTA score (MD 13.48; 95% CI 3.83 – 23.13; p < 0.01), and PANQOL total score (MD 3.83; 95% CI 0.42 – 7.25; p = 0.03). The overall certainty of evidence ranged from “very low” to “moderate”.
Conclusions
Treating small to medium sized VS with WAS increases the likelihood of preserving serviceable hearing and optimized PANQOL overall postoperative score compared to SRS. Nevertheless, the limited availability of literature and the methodological weakness observed in existing studies outline the need for higher-quality studies.
This is a preview of subscription content, log in via an institution to check access.
Access this article
Subscribe and save.
- Get 10 units per month
- Download Article/Chapter or eBook
- 1 Unit = 1 Article or 1 Chapter
- Cancel anytime
Price includes VAT (Russian Federation)
Instant access to the full article PDF.
Rent this article via DeepDyve
Institutional subscriptions
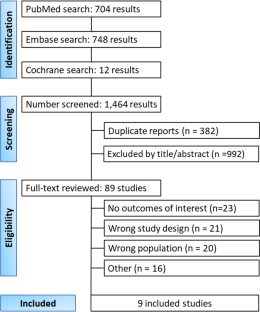
Data availability
Data extraction spreadsheet is provided within the manuscript.
Balduzzi S, Rücker G, Schwarzer G (2019) How to perform a meta-analysis with R: a practical tutorial. Evid Based Ment Health 22(4):153–160
Article PubMed PubMed Central Google Scholar
Balossier A, Delsanti C, Troude L, Thomassin J-M, Roche P-H, Régis J (2023) Assessing tumor volume for sporadic vestibular schwannomas: a comparison of methods of volumetry. Stereotact Funct Neurosurg 101(4):265–276
Article PubMed Google Scholar
Barnes JH, Patel NS, Lohse CM, Tombers NM, Link MJ, Carlson ML (2021) Impact of treatment on vestibular schwannoma-associated symptoms: a prospective study comparing treatment modalities. Otolaryngology-Head and Neck Surgery 165(3):458–464
Carlson ML, Barnes JH, Nassiri A, Patel NS, Tombers NM, Lohse CM, Van Gompel JJ, Neff BA, Driscoll CLW, Link MJ (2021) prospective study of disease-specific quality-of-life in sporadic vestibular schwannoma comparing observation, radiosurgery, and microsurgery. Otol Neurotol 42(2):e199–e208
Carlson ML, Dowling EM, Lohse CM, O’Connell BP, Driscoll CLW, Haynes DS, Link MJ, Hunter JB (2019) Rate of initial hearing loss during early observation predicts time to non-serviceable hearing in patients with conservatively managed sporadic vestibular schwannoma. Otol Neurotol 40(10):e1012–e1017
Carlson ML, Jacob JT, Pollock BE, Neff BA, Tombers NM, Driscoll CLW, Link MJ (2013) Long-term hearing outcomes following stereotactic radiosurgery for vestibular schwannoma: patterns of hearing loss and variables influencing audiometric decline: Clinical article. J Neurosurg 118(3):579–587
Carlson ML, Tveiten OV, Driscoll CL et al (2015) Long-term quality of life in patients with vestibular schwannoma: an international multicenter cross-sectional study comparing microsurgery, stereotactic radiosurgery, observation, and nontumor controls. J Neurosurg 122(4):833–842
Committee on Hearing and Equilibrium (1995) Committee on hearing and equilibrium guidelines for the evaluation of hearing preservation in acoustic neuroma (vestibular schwannoma)∗. Otolaryngology-Head and Neck Surgery 113(3):179–180
Article Google Scholar
Dalton JE, Bolen SD, Mascha EJ (2016) Publication Bias: The Elephant in the Review. Anesth Analg 123(4):812–813
Deberge S, Meyer A, Le Pabic E, Peigne L, Morandi X, Godey B (2018) Quality of life in the management of small vestibular schwannomas: Observation, radiotherapy and microsurgery. Clin Otolaryngol 43(6):1478–1486
DerSimonian R, Laird N (1986) Meta-analysis in clinical trials. Control Clin Trials 7(3):177–188
Article CAS PubMed Google Scholar
Dhayalan D, Tveiten ØV, Finnkirk M, Storstein A, Hufthammer KO, Goplen FK, Lund-Johansen M (2023) Upfront radiosurgery vs a wait-and-scan approach for small- or medium-sized vestibular schwannoma: the v-rex randomized clinical trial. JAMA 330(5):421–431
Gallogly JA, Jumaily M, Faraji F, Mikulec AA (2018) Stereotactic radiotherapy in three weekly fractions for the management of vestibular schwannomas. Am J Otolaryngol 39(5):561–566
Goldbrunner R, Weller M, Regis J et al (2020) EANO guideline on the diagnosis and treatment of vestibular schwannoma. Neuro Oncol 22(1):31–45
GRADEpro GDT: GRADEpro Guideline Development Tool [Software]. https://www.gradepro.org/ . Accessed 15 Apr 2024
Guadix SW, Tao AJ, An A, Demetres M, Tosi U, Chidambaram S, Knisely JPS, Ramakrishna R, Pannullo SC (2021) Assessing the long-term safety and efficacy of gamma knife and linear accelerator radiosurgery for vestibular schwannoma: A systematic review and meta-analysis. Neurooncol Pract 8(6):639–651
PubMed PubMed Central Google Scholar
Higgins J, Thomas J, Chandler J, Cumpston M, Li T, Page M, Welch V Cochrane Handbook for Systematic Reviews of Interventions. https://training.cochrane.org/handbook/archive/v6.3 . Accessed 15 Apr 2024
Ismail O, Sobhy O, Assal S, Sanghera P, Begg P, Irving R (2022) Comparing hearing outcomes in irradiated and conservatively managed vestibular schwannoma. Otol Neurotol 43(3):e374
Leon J, Lehrer EJ, Peterson J et al (2019) Observation or stereotactic radiosurgery for newly diagnosed vestibular schwannomas: A systematic review and meta-analysis. J Radiosurg SBRT 6(2):91–100
Luo D Optimally estimating the sample mean from the sample size, median, mid-range, and/or mid-quartile range - Dehui Luo, Xiang Wan, Jiming Liu, Tiejun Tong, 2018. https://journals.sagepub.com/doi/10.1177/0962280216669183?url_ver=Z39.88-2003&rfr_id=ori:rid:crossref.org&rfr_dat=cr_pub%20%200pubmed . Accessed 15 Apr 2024
Marinelli JP, Schnurman Z, Killeen DE et al (2022) Long-term natural history and patterns of sporadic vestibular schwannoma growth: A multi-institutional volumetric analysis of 952 patients. Neuro Oncol 24(8):1298–1306
McLaughlin EJ, Bigelow DC, Lee JYK, Ruckenstein MJ (2015) Quality of life in acoustic neuroma patients. Otol Neurotol 36(4):653–656
Miller LE, Brant JA, Chen J, Kaufman AC, Ruckenstein MJ (2019) Hearing and quality of life over time in vestibular schwannoma patients: observation compared to stereotactic radiosurgery. Otol Neurotol 40(8):1094–1100
Mousavi SH, Niranjan A, Akpinar B, Huang M, Kano H, Tonetti D, Flickinger JC, Lunsford LD (2016) Hearing subclassification may predict long-term auditory outcomes after radiosurgery for vestibular schwannoma patients with good hearing. J Neurosurg 125(4):845–852
Munn Z, Barker TH, Moola S, Tufanaru C, Stern C, McArthur A, Stephenson M, Aromataris E (2020) Methodological quality of case series studies: an introduction to the JBI critical appraisal tool. JBI Evidence Synthesis 18(10):2127
PubMed Google Scholar
Ouzzani M, Hammady H, Fedorowicz Z, Elmagarmid A (2016) Rayyan—a web and mobile app for systematic reviews. Syst Rev 5(1):210
Page MJ, McKenzie JE, Bossuyt PM et al (2021) The PRISMA 2020 statement: an updated guideline for reporting systematic reviews. BMJ 372:n71
Pruijn IMJ, Kievit W, Hentschel MA, Mulder JJS, Kunst HPM (2021) What determines quality of life in patients with vestibular schwannoma? Clin Otolaryngol 46(2):412–420
Reznitsky M, Petersen MMBS, West N, Stangerup S-E, Cayé-Thomasen P (2021) The natural history of vestibular schwannoma growth-prospective 40-year data from an unselected national cohort. Neuro Oncol 23(5):827–836
Robinett ZN, Walz PC, Miles-Markley B, Moberly AC, Welling DB (2014) Comparison of long-term quality-of-life outcomes in vestibular schwannoma patients. Otolaryngol Head Neck Surg 150(6):1024–1032
Schnurman Z, Gurewitz J, Smouha E, McMenomey SO, Roland JTJ, Golfinos JG, Kondziolka D (2022) Matched comparison of hearing outcomes in patients with vestibular schwannoma treated with stereotactic radiosurgery or observation. Neurosurgery 91(4):641
Schünemann H, Brożek, Guyatt G, Oxman A GRADE handbook for grading quality of evidence and strength of recommendations. https://gdt.gradepro.org/app/handbook/handbook.html . Accessed 15 Apr 2024
Shi J Optimally estimating the sample standard deviation from the five‐number summary - Shi - 2020 - Research Synthesis Methods - Wiley Online Library. https://onlinelibrary.wiley.com/doi.org/10.1002/jrsm.1429 . Accessed 15 Apr 2024
Soulier G, van Leeuwen BM, Putter H, Jansen JC, Malessy MJA, van Benthem PPG, van der Mey AGL, Stiggelbout AM (2017) Quality of Life in 807 Patients with Vestibular Schwannoma: Comparing Treatment Modalities. Otolaryngology-Head and Neck Surgery 157(1):92–98
Sterkers J-M Preservation of facial, cochlear, and other nerve functions in acoustic neuroma treatment - Sterkers - 1994 - Otolaryngology–Head and Neck Surgery - Wiley Online Library. https://aao-hnsfjournals.onlinelibrary.wiley.com/doi/10.1177/019459989411000202 . Accessed 8 Jul 2024
Sterne JA, Hernán MA, Reeves BC et al (2016) ROBINS-I: a tool for assessing risk of bias in non-randomised studies of interventions. BMJ 355:i4919
Sterne JAC, Savović J, Page MJ et al (2019) RoB 2: a revised tool for assessing risk of bias in randomised trials. BMJ 366:l4898
Tamura M, Carron R, Yomo S, Arkha Y, Muraciolle X, Porcheron D, Thomassin JM, Roche PH, Régis J (2009) Hearing preservation after gamma knife radiosurgery for vestibualr schwannomas presenting with high-level hearing. Neurosurgery 64(2):289
Tveiten OV, Carlson ML, Goplen F, Vassbotn F, Link MJ, Lund-Johansen M (2015) Long-term auditory symptoms in patients with sporadic vestibular schwannoma: an international cross-sectional study. Neurosurgery 77(2):218
Willis BH, Riley RD (2017) Measuring the statistical validity of summary meta-analysis and meta-regression results for use in clinical practice. Stat Med 36(21):3283–3301
Wu H, Zhang L, Han D, Mao Y, Yang J, Wang Z, Jia W, Zhong P, Jia H (2016) Summary and consensus in 7th International Conference on acoustic neuroma: An update for the management of sporadic acoustic neuromas. World J Otorhinolaryngol Head Neck Surg 2(4):234–239
Download references
No funding was received for this research.
Author information
Authors and affiliations.
Dominican Gamma Knife Center, CEDIMAT, Caribbean, Santo Domingo, Dominican Republic
Anderson Brito & Herwin Speckter
Federal University of Piaui, Teresina, Piaui, Brazil
Jackson Daniel Sousa Silva
Division of Neurosurgery, Beth Israel Deaconess Medical Center, Harvard Medical School, Boston, MA, USA
Fernando Terry, Alejandro Enriquez-Marulanda, Ziev B. Moses & Rafael A. Vega
Andhra Medical College, Visakhapatnam, India
Anuraag Punukollu
Department of Neurosurgery, Miller School of Medicine, University of Miami, Coral Gables, FL, USA
Adam S. Levy & Ricardo J. Komotar
University City of São Paulo (UNICID), São Paulo, Brazil
Anna Lydia Machado Silva
Jackson Memorial Hospital, Miami, FL, USA
Alexis A. Morell
You can also search for this author in PubMed Google Scholar
Contributions
A.B. wrote the main manuscript text, did the data analysis and prepared Figs. 1 - 5 . A.B., F.T. and A.P., did the data extraction. A.B. and F.T. prepared Table 1 and Table 2 . A.S. data acquisition. J.D.S.S. analyzed the extracted data before proceeding. All authors reviewed the manuscript.
Corresponding author
Correspondence to Rafael A. Vega .
Ethics declarations
Ethical approval.
This article does not contain any studies with human or animal participants performed by any of the authors. Additionally, this article is a systematic review and meta-analysis. Therefore, we did not require any prior consent of the IRB.
Competing interests
The authors declare no competing interests.
Additional information
Publisher's note.
Springer Nature remains neutral with regard to jurisdictional claims in published maps and institutional affiliations.
Supplementary Information
Below is the link to the electronic supplementary material.
Supplementary file1 (DOCX 587 KB)
Supplementary file2 (docx 26 kb), rights and permissions.
Springer Nature or its licensor (e.g. a society or other partner) holds exclusive rights to this article under a publishing agreement with the author(s) or other rightsholder(s); author self-archiving of the accepted manuscript version of this article is solely governed by the terms of such publishing agreement and applicable law.
Reprints and permissions
About this article
Brito, A., Silva, J.D.S., Terry, F. et al. Hearing preservation and quality of life in small to medium sized vestibular schwannomas after a wait and scan approach or stereotactic radiosurgery: a systematic review and meta-analysis. Acta Neurochir 166 , 361 (2024). https://doi.org/10.1007/s00701-024-06249-x
Download citation
Received : 24 July 2024
Accepted : 23 August 2024
Published : 09 September 2024
DOI : https://doi.org/10.1007/s00701-024-06249-x
Share this article
Anyone you share the following link with will be able to read this content:
Sorry, a shareable link is not currently available for this article.
Provided by the Springer Nature SharedIt content-sharing initiative
- Acoustic neuroma
- Vestibular schwannoma
- Quality of life
- Radiosurgery
- Stereotactic radiosurgery
- Find a journal
- Publish with us
- Track your research

IMAGES
VIDEO
COMMENTS
The purpose of analysing data is to obtain usable and useful information. The analysis, irrespective of whether the data is qualitative or quantitative, may: • describe and summarise the data • identify relationships between variables • compare variables • identify the difference between variables • forecast outcomes
Data Presentation - Types & Its Importance
Understanding Data Presentations (Guide Examples)
6 Differences Between Data Exploration and Data Presentation. Let's start by defining our terms: Data exploration means the deep-dive analysis of data in search of new insights. Data presentation means the delivery of data insights to an audience in a form that makes clear the implications. Your toolbox for data exploration tools is flush ...
It is important to know whether you are in the unruly "herding cats" stage of your analysis or whether you are ready to present a clear message with data. This lesson helps draw the distinctions so you know whether you are ready to tell a data story. ... The Difference Between Data Analysis and Data Presentation. Zach Gemignani. December 24 ...
What is Data Analysis? An Expert Guide With Examples
Abstract. This chapter covers the topics of data collection, data presentation and data analysis. It gives attention to data collection for studies based on experiments, on data derived from existing published or unpublished data sets, on observation, on simulation and digital twins, on surveys, on interviews and on focus group discussions.
What Is Data Analysis? (With Examples)
Types of Data Analysis: A Guide
Data Analysis vs. Data Analytics: 5 Key Differences
Chapter 2 Data and Data Presentation. hapter 2 Data and Data PresentationPlanning is a process that designs a plan of action or evaluates the impact proposed. ction to achieve a desirable future. During this process planners obtain the necessary data from different sources, analyze them efficiently comprehensively, and present the results in ...
10 Data Presentation Examples For Strategic Communication
While data science, data analysis, and data visualization are distinct fields, they are deeply interconnected and complementary. Together, they form a comprehensive approach to leveraging data for informed decision-making and strategic advantage. By understanding the differences and synergies between these roles, individuals and organizations can better navigate the data landscape and harness ...
The Difference Between Data Analysis and Data Interpretation. Data analysis involves processing data to uncover patterns and insights, while data interpretation involves making sense of these patterns and understanding their implications in the context of your research questions and hypotheses. ... Link your data presentation directly to your ...
Data Presentation. Data can be presented in one of the three ways: -as text; -in tabular form; or -in graphical form. Methods of presentation must be determined according to the data format, the method of analysis to be used, and the information to be emphasized.
Written by Coursera Staff • Updated on Mar 4, 2024. Data scientists primarily use data science in their careers, while data analysts use data analytics. We will explore how these roles differ regarding skill sets, responsibilities, and career outlook. Data science and data analytics are two closely related fields, but there are key ...
Let's start by defining our terms: Data exploration means the deep-dive analysis of data in search of new insights. Data presentation means the delivery of data insights to an audience in a form that makes clear the implications. Your toolbox for data exploration tools is flush with technology solutions such as Tableau, PowerBI, Looker, and Qlik.
Data is the basis of information, reasoning, or calcul ation, it is analysed to obtain. information. Data analysis is a process of inspecting, cleansing, transforming, and data. modeling with the ...
Discuss the difference between qualitative and quantitative data and analysis. Enable you to analyze data gathered from questionnaires. Enable you to analyze data gathered from interviews. Enable you to analyze data gathered from observation studies. Make you aware of software packages that are available to help your analysis. Identify some of ...
What's the Difference Between Data Analytics & Data Science?
CHAPTER FOUR DATA PRESENTATION, ANALYSIS AND ...
The dividing line between the analysis of data and interpretation is difficult to draw as the two processes are symbolic and merge imperceptibly. Interpretation is inextricably interwoven with analysis. The analysis is a critical examination of the assembled data. Analysis of data leads to generalization. Interpretation refers to the analysis ...
Data Collection vs. Data Analysis: What's The Difference?
DATA SYNTHESIS: Eight studies had some concerns, and 1 study was considered high risk. Overall, the risk of symptomatic intracerebral hemorrhage was comparable between mechanical thrombectomy + IV thrombolysis and mechanical thrombectomy alone (risk ratio, 1.24 [95% CI, 0.89-1.72]; P = .20), with no heterogeneity across studies. Subgroup analysis of symptomatic intracerebral hemorrhage ...
This study aimed to examine longitudinal relationships among perceived teacher support, intrinsic motivation for math, and math learning outcomes. Focusing on gender differences among high-achieving adolescents, we explored whether there were significant differences in the levels of research variables and the associations among them. Data from 639 high-achieving students (boys 57.2%, girls 42. ...
Purpose The immune profiles of elder patients with non-small cell lung cancer (NSCLC) differ significantly from those of younger patients. The tumor microenvironment (TME) is a crucial factor in cancer progression and therapeutic responses. The present study aims to decipher the difference in TME between younger and elderly patients with lung cancers. Methods We downloaded single-cell RNA data ...
Background The management of vestibular schwannomas (VS) encompasses a choice between conservative "wait-and-scan" (WAS) approach, stereotactic radiosurgery (SRS) or open microsurgical resection. Currently, there is no consensus on the optimal management approach for small to medium sized VS. This study aims to compared outcomes related to hearing in patients with small and medium sized VS ...