AI research landscape at U of T
- GenAI at U of T Home
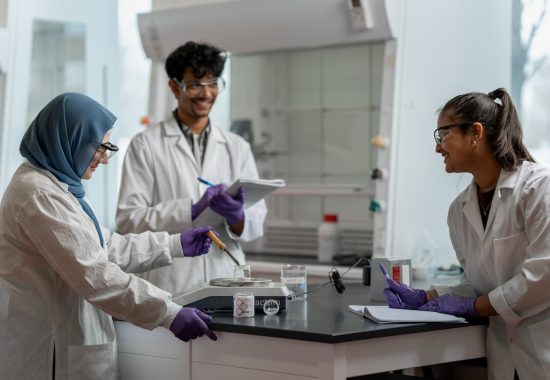

Research centres & units
Centres focusing on ai research.
- Acceleration Consortium for AI in Materials Research
- Artificial Intelligence for Justice Lab
- Data Sciences Institute: Emerging Data Science Program on a Fair & Inclusive Future of Work with GenAI
- Digital Humanities Network: Critical Digital Humanities Initiative, AI and Humanities Lab
- Faculty of Applied Science & Engineering: Centre for Analytics & Artificial Intelligence Engineering
- Faculty of Arts & Science: Department of Computer Science, AI Research Areas
- Rotman School of Management: Creative Destruction Lab, AI Stream
- Schwartz Reisman Institute for Technology and Society
- Temerty Centre for Artificial Intelligence Research and Education in Medicine
- University of Toronto Joint Centre for Bioethics: AI and Health Systems
- Vector Institute for AI Research (U of T-partnered organization)
Using AI as a research tool
Resources for using ai in research.
- University of Toronto Libraries: GenAI and Copyright Considerations
- University of Toronto Libraries: Citing GenAI Tools
- University of Toronto Libraries: AI for Image Research in Art and Architecture
- School of Graduate Studies: Guidance on the Appropriate Use of GenAI in Graduate Theses
- Information Security Council: Use AI Intelligently
- Information Technology Services: AI Chatbot Microsoft Copilot Guidelines
School of Graduate Studies
Guidance on the appropriate use of generative artificial intelligence in graduate theses.
In response to the rapidly evolving landscape of generative artificial intelligence (AI) [1] use in academic and educational settings, this preliminary guidance has been produced to address frequently asked questions ( FAQ ) in the context of graduate thesis work at the University of Toronto. More detailed guidance on this topic, as well as new or updated policies may be issued in future, in which case this preliminary guidance will also be updated. The FAQ s below outline important considerations for graduate students, supervisors, supervisory committees, and graduate units on the use of generative AI tools (such as ChatGPT) in graduate student research and thesis writing, while upholding the core principles of academic quality, research integrity, and transparency. The FAQ s cover requirements both for approval and for documentation of the use of generative AI tools in graduate thesis research and writing, as well as risks and other considerations in using generative AI in graduate thesis research and writing.
Innovative and creative uses of generative AI may support scholarly activities and help facilitate high quality research, particularly in certain disciplines. Graduate students and faculty supervisors are expected to strive for the highest standards of academic quality and research integrity in all scholarly activities, and therefore the use of generative AI tools in the process of graduate thesis research and writing must always take place with full transparency. This includes transparency between students and their supervisors, who must agree in advance how any generative AI tools will be used; as well as transparency between graduate students and the audiences of their work, who must be provided a clear and complete description and citation of any use of generative AI tools in creating the scholarly work.
Students who plan to use generative AI tools in researching or writing their graduate thesis must always seek and document in writing unambiguous approval for the planned uses in advance from their supervisor(s) and supervisory committee. Unauthorized use of generative AI tools for scholarly work at the University of Toronto may be considered an offence under the Code of Behaviour on Academic Matters , and research misconduct as defined in the Policy on Ethical Conduct in Research and the Framework to Address Allegations of Research Misconduct . Furthermore, careful attention must be paid in the thesis to appropriate citation and describing any use of generative AI tools that took place in the research or writing process, in line with disciplinary norms. This includes, for example, using generative AI tools in searching, designing, outlining, drafting, writing, or editing the thesis, or in producing audio or visual content for the thesis, and may include other uses of generative AI. Even when engaging in authorized generative AI use, faculty and graduate students must be aware of the risks in using such tools, some of which are discussed below.
Faculties and graduate units may have specific requirements or restrictions regarding the use of generative AI in some or all phases of the graduate research lifecycle. Individual graduate units may therefore issue additional guidance outlining field-specific appropriate uses of generative AI tools in researching and writing a doctoral thesis. This could include, for example, guidance on use in writing text, conducting analytical work, reporting results (e.g., tables or figures) or writing computer code. Graduate units issuing additional guidance should take into account the issues discussed in the FAQ below. Additional relevant guidance and further reading can be found in the FAQs and guidance on syllabi and assignments (PDF) issued by the Office of the Vice-Provost, Innovations in Undergraduate Education, and in the guidance on generative AI in the classroom from the Centre for Teaching Support & Innovation.
[1] In referring to generative AI in this document, we include tools that use predictive technology to produce new text, charts, images, audio, or video. For example uses and more detail, please see the F AQs and guidance on syllabi and assignments issued by the Office of the Vice-Provost, Innovations in Undergraduate Education, and the guidance on generative AI in the classroom from the Centre for Teaching Support & Innovation.
Frequently Asked Questions ( FAQ )
Last Updated: May 2, 2024
The School of Graduate Studies ( SGS ) Doctoral Thesis Guidelines state that students must produce a thesis that demonstrates academic rigour and makes a distinct contribution to the knowledge in the student’s field. The University expects that a thesis submitted to satisfy degree requirements at the doctoral level is the work of the student, carried out under the guidance of the supervisor and committee. The SGS Guidelines specify Key Criteria of the Doctoral Thesis that students must meet, in addition to the Ontario Council of Academic Vice-Presidents’ Doctoral Degree Expectations for Doctoral Students in Ontario . The Key Criteria of the Doctoral Thesis include presenting the results and analysis of original research, and demonstrating that the thesis makes an original contribution to advancing knowledge. These originality requirements may not be met by work produced using generative AI tools, which rely on existing sources to generate content-based probabilistic or other predictive functions that may not result in sufficiently original content to meet the criteria.
If a student plans to use generative AI tools in any aspect of researching or writing of their thesis, this must be done with the prior approval of the supervisor(s) and supervisory committee. This is consistent with how other decisions about the thesis, including structure and format, are decided, as detailed in the SGS guidance . (See also the Guideline for Graduate Student Supervision & Mentorship for more detail on the supervisor’s and committee’s roles in guiding students to produce research of high quality and integrity). Careful attention must be paid in the thesis to appropriately citing and describing any use of generative AI tools in the research process. It must be clear to the reader which generative AI tools were used, as well as how and why they were used. In the same way that analytical tools and specific analytical approaches are identified and described in the thesis, generative AI tools and interactions with them must be equivalently described.
When supervisors and committees approve student use of generative AI in any aspect of producing the doctoral thesis, it must be clear how the student’s versus the AI tool’s contributions will be identified, and it must be possible for the student to provide sufficient evidence that they themselves have met the Key Criteria of the Doctoral Thesis and demonstrated the doctoral level degree expectations. It must be clear to the student what evidence they need to provide to clarify their own contributions and how they made use of any AI tools, and how their work will be assessed by the supervisor and committee at each supervisory committee meeting. (Consult the Guidelines for Departmental Monitoring of the Progress of Doctoral Students and the Guideline for Graduate Student Supervision & Mentorship for more detail on responsibilities in student evaluation and monitoring of doctoral student progress.) Students are responsible for any content generated by AI that they include in their thesis. Note also that at the University of Toronto, the outcome of the final oral examination is based not only on the submitted written thesis, but also the student’s performance in the oral examination. Students must be able to describe and defend any use of generative AI, as well as the contents of the thesis during their final oral examination.
Learning the practices of disciplinary scholarly writing is a key aspect of graduate education. The use of generative AI could hamper the development of graduate writing skills because writing capacity is highly dependent on practice. Novice scholarly writers need to write frequently, with in-depth feedback from members of their disciplinary community. Using AI to lessen the burdens of writing could undermine the development of these invaluable skills. This diminished capacity in writing could have serious consequence for graduate students, who need to be able to use writing as a vital element of their overall research process. The act of drafting and revising scholarly work often entails an essential deepening of the engagement with their research. Most writers learn about their own thinking through the iterative act of writing; if AI is doing some part of that writing, writers may be missing a crucial opportunity to cement their own scholarly expertise.
Last Updated: July 4, 2023
The same principles that apply to the use of generative AI tools to produce or edit text also apply to the use of these tools to produce or edit figures, images, graphs, sound files, videos, or other audio or visual content. It should be noted, however, that some publication policies permitting the use of AI-generated text in certain contexts apply more stringent criteria to image content, in some cases completely prohibiting such content, for example, see the editorial policy on the use of AI-generated images at Nature .
SGS does not regulate master’s-level theses, Major Research Papers, or qualifying / comprehensive exams. However, SGS recommends that graduate units make use of the principles outlined for doctoral-level work in articulating requirements for any master’s-level research-based works. The Code of Behaviour on Academic Matters , Policy on Ethical Conduct in Research and Framework to Address Allegations of Research Misconduct also apply to master’s research, theses, Major Research Papers, and other research-based works, including qualifying and comprehensive exams. Faculties and graduate units may issue specific guidance on the use of generative AI in such works. The F AQs and guidance on syllabi and assignments issued by the Office of the Vice-Provost, Innovations in Undergraduate Education may be more relevant in the context of some papers or projects, and also apply to the undergraduate context.
Different disciplinary norms are likely to emerge around the appropriate use of generative AI in research, even in fields in which the focus of the research is not specifically the development and implementation of AI. If use of generative AI is permitted by a graduate unit in the research process, it must be clear to faculty and students which methods (if any) are acceptable and which (if any) are not. Supervisors should seek clarification from their graduate unit if uncertain about a particular use of generative AI in doctoral research and thesis writing.
Privacy concerns have been raised in relation to the data processing undertaken to train generative AI tools, as well as the (mis)information that such tools provide about individuals or groups. Investigations have been initiated in Canada and in the EU regarding the privacy implications of ChatGPT, for example. For graduate student researchers working with certain kinds of data, using third-party generative AI tools to process the data may come with additional privacy and security risks. For example, students working with data from human research participants must not submit any personal or identifying participant information, nor any information that could be used to reidentify an individual or group of participants to third-party generative AI tools, as these data may then become available to others, constituting a major breach of research participant privacy. Similarly, students working with other types of confidential information, such as information disclosed as part of an industry partnership, must not submit these data to third-party generative AI tools, as this could breach non-disclosure terms in an agreement. Students wishing to use generative AI tools for processing such data must have documented appropriate permissions to do so, for example, explicit approval from a Research Ethics Board or industry partner.
Researchers are advised to seek help assessing the risk prior to engaging in any data or information processing with third-party AI tools. Information Security and Enterprise Architecture has additional guidance on information risk management, including a risk assessment questionnaire. Your Divisional IT team or Library may be able to provide help assessing the risk attached to a particular use case. The Centre for Teaching Support & Innovation also has a checklist designed for teaching tools that may be a helpful starting point in assessing particular tools for use in academic contexts.
If a graduate unit permits the use of generative AI in research, the graduate unit should ensure discipline-specific norms regarding description of the method of use and appropriate references are clear. For example, is it adequate to include the prompts provided to a tool along with excerpts of responses? Should students save or include the full text of their interactions with AI tools in an appendix? Different citation style guides are starting to include specific information on how to cite generative AI tools, for example, see the American Psychological Association Style Blog . Links to major style guides can be found on the University of Toronto Library citation webpages .
Graduate units and individual supervisors who embrace the use of generative AI tools in research methods may still wish to restrict the use of such tools in other aspects of writing or editing papers or theses. There must be clear guidance for graduate students on what degree of engagement with generative AI in writing is acceptable (if any). If there are specific tools that are (un)authorized, these should be explained. Graduate students must seek and document approval from their supervisors and committees for the use of generative AI in writing even if they already have approval to use generative AI tools in their research.
Most major journals and scholarly publishers now have policies regarding the use of generative AI in publication. These policies vary widely, and researchers must ensure they are adhering to the specific policies of the pre-print server, journal, or publisher to which they are submitting. For example, some publishers allow use of generative AI in the research process, with appropriate description, references, and supplementary material to show the interaction with the AI tool, but do not allow the inclusion of AI-generated text. Others allow the inclusion of AI-generated text, but not images.
Emerging consensus is that generative AI tools do not meet the criteria for authorship of scholarly works, because these tools cannot take responsibility or be held accountable for submitted work. These issues are discussed in more detail in the statements on authorship from the Committee on Publication Ethics , and the World Association of Medical Editors , for example.
Graduate units, supervisors, and students must be familiar with and adhere to the requirements in their field regarding authorship and use of AI in works submitted to pre-print servers or for publication.
Generative AI may produce content that is wholly inaccurate or biased. AI tools can reproduce biases that already exist in the content they are trained on, include outdated information, and can present untrue statements as facts. Students remain responsible for the content of their thesis, no matter what sources are used (see also Who is responsible for AI-generated content used in research or other scholarly work? ) Generative AI tools have also been shown to reference scholarly works that do not exist, and to generate offensive content. Therefore, AI-generated content may not meet the academic or research integrity standards expected at the University of Toronto. Generative AI tools are also predictive, and may not generate the type of novel content expected of graduate students, nor arrange existing knowledge in such a way as to reveal the need for the novel contribution made by the research underlying a graduate student thesis. (See also the information on originality in Can students use generative AI tools to research or write a doctoral thesis? )
Last Updated: October 12, 2023
The legal landscape with respect to intellectual property and copyright in the context of generative AI is uneven across jurisdictions and rapidly evolving, and the full implications are not yet clear. Researchers, including graduate students, must exercise caution in using generative AI tools, because some uses may infringe on copyright or other intellectual property protections. Similarly, providing data to an AI tool may complicate future attempts to enforce intellectual property protections. Generative AI may also produce content that plagiarizes others’ work, failing to cite sources or make appropriate attribution. Graduate students including AI-generated content in their own academic writing risk including plagiarized material or someone else’s intellectual property. Since students are responsible for the content of their academic work, including AI-generated content may result in a violation of the Code of Behaviour on Academic Matters or other University of Toronto policies.
For more information, please see:
- Generative AI Tools and Copyright Considerations (University Toronto Libraries) for more detail on copyright and generative AI
- Who is responsible for AI-generated content used in research or other scholarly work? ( SGS )
Graduate students who make use of AI tools and include the output in their research and written work are ultimately responsible for the content. This applies to work submitted as part of degree requirements, as well as in scholarly publishing or the use of pre-print servers. Graduate students and their co-authors must understand the terms and conditions of any submission of their work and for any tools they use, as these often hold the user responsible for the content. This means graduate students may find themselves in a position where they face allegations of perpetuating false or misleading information, infringement of intellectual property rights, violating the conditions of research ethics approval, other research misconduct, infringement of privacy rights, or other issues that carry academic, civil, or criminal penalties.
Relevant Policies and Further Reading
- Artificial Intelligence at U of T
- Generative AI Tools and Copyright Considerations
- Code of Behaviour on Academic Matters
- Policy on Ethical Conduct in Research
- Framework to Address Allegations of Research Misconduct
- Research Misconduct Framework Addendum
- Statement on Research Integrity
- SGS Doctoral Thesis Guidelines
- ChatGPT and Generative AI in the Classroom
- Generative Artificial Intelligence in the Classroom
- Graduate Centre for Academic Communication
Vice Dean, Research & Program Innovation: [email protected] Graduate Program Completion Office Doctoral: Coordinator, Graduate Program Completion, [email protected] Master’s: Program Completion Officer, Master’s, [email protected]
Machine Learning at the University of Toronto
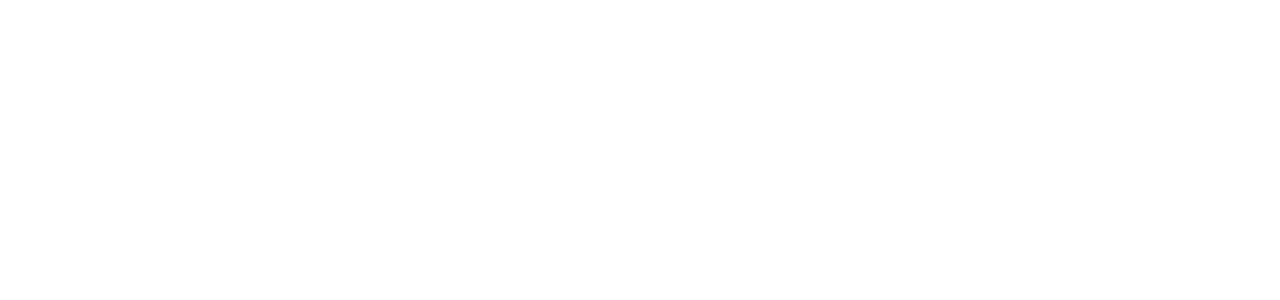
Artificial Intelligence in healthcare
From machine learning to computational medicine and biology, Artificial Intelligence (AI) is the future of healthcare.
By establishing the Temerty Centre for Artificial Intelligence Research and Education in Medicine (T-CAIREM), we are integrating AI, such as machine learning, and analytics into our research and education.
Far from replacing humans at the forefront of healthcare and science, AI enables our clinicians to enhance patient diagnosis and care, and our researchers to mine data to reach new boundaries in research.
Areas include:
- Machine learning and medical imaging for diagnostics and research
- 'Omics' dataset analysis
- Natural language processing to predict outcomes from clinical notes
- Advancing cardiovascular and other programs
- Computational pathology
- Point-of-Care Testing (POCT) monitoring
Find out more about Pathology and AI (and how to access a free online module) in our story: Understanding Artificial Intelligence from a Cytopathologist’s perspective
The Emergence of General AI for Medicine
Latest news on Artificial Intelligence in healthcare research
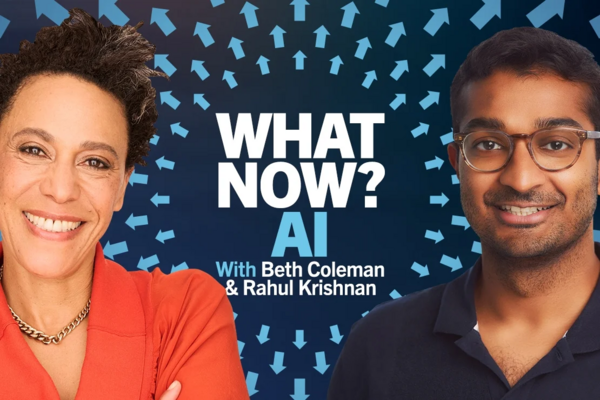
How will AI change our world? Rahul Krishnan launches podcast to explore technology’s impact on society
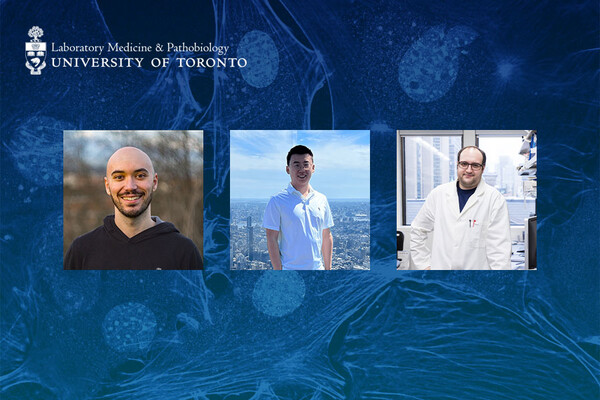
"Cornerstones of biomedical research labs”: celebrating our postdocs
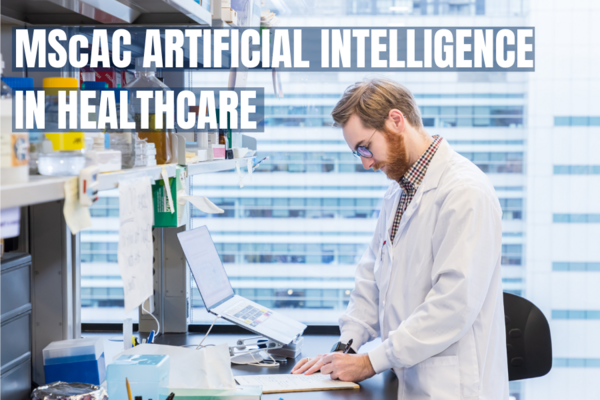
New AI in Healthcare masters program starts this Fall
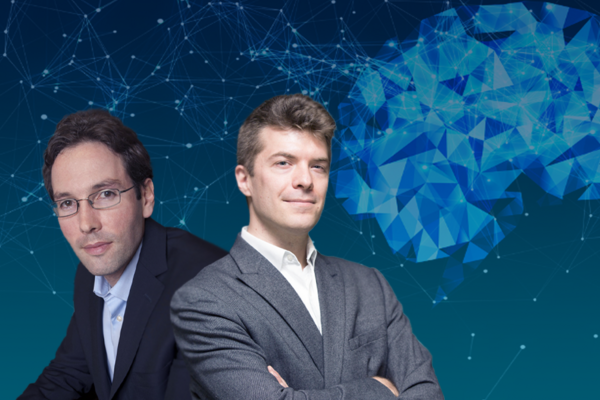
Using voice to diagnose disease: collaboration uses machine learning to aid diagnostics
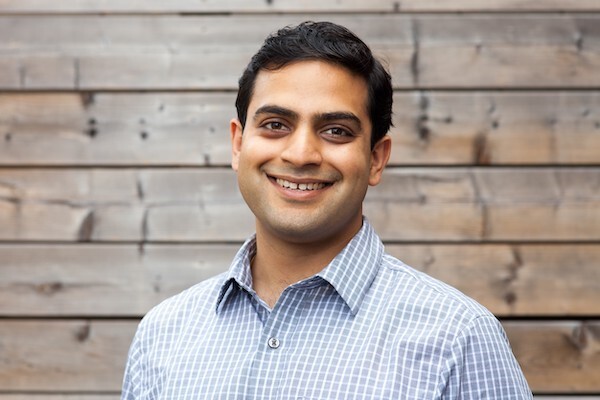
Dr. Amol Verma announced as 2023 Temerty Professorship in AI Research and Education in Medicine
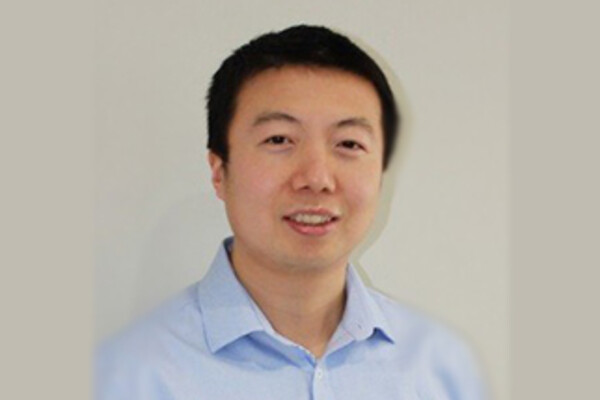
Opening up a new world of machine learning for biomedical students
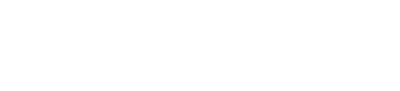
Deep Learning & Artificial Intelligence
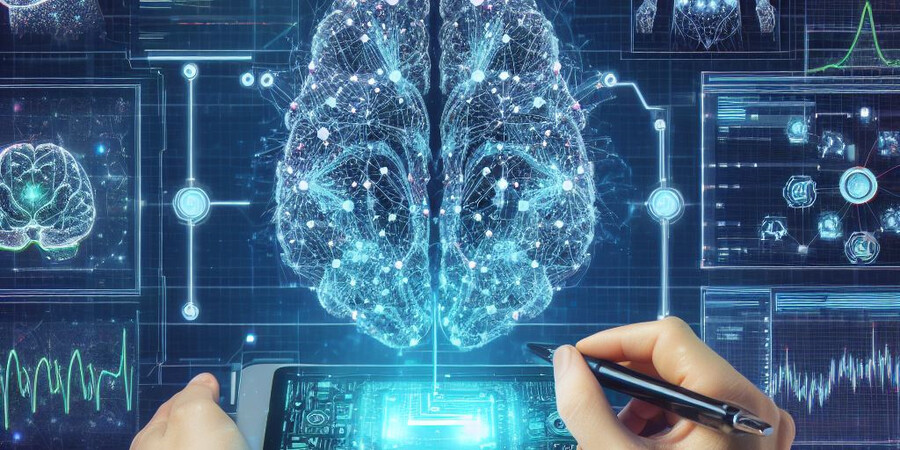
Deep Learning is changing the way we understand diseases, make diagnosis, and treat patients. Armed with vast data sets scientists in the field of Deep Learning and Artificial Intelligence in healthcare leverage techniques rooted in mathematics, statistics, computer science, and machine learning to develop novel algorithms and models that positively impact healthcare. This data encompasses a wide spectrum including medical imaging, omics data, digital pathology, wearables, clinical notes, and more. A significant portion of contemporary research in this domain focuses on developing and applying deep learning algorithms to harness these vast datasets to develop algorithms and models for improving our diagnosis, treatment, and understanding of diseases.
Examples of specific research topics include:
- Disease Diagnosis: Developing AI-driven models to enhance the accuracy and timeliness of disease diagnosis in cancer, cardiovascular, neurological, and psychiatric diseases and more.
- Disease and Outcome Prediction: Developing AI models to predict disease progression, patient risk, and patient outcomes or response to therapy, as well as perform patient stratification for clinical trials.
- Medical Imaging Analysis: Utilizing deep learning for the interpretation of medical images, including radiology, pathology, microscopy, and dermatology, to develop biomarkers, understand disease models, and assist in more precise diagnostics.
- Electronic Health Record (EHR) Mining: Extracting valuable insights with natural language processing from electronic health records to improve patient care, treatment strategies, and healthcare management.
- Telemedicine and Remote Monitoring: Developing AI solutions for remote patient monitoring and telemedicine to enhance accessibility and the quality of care.
- Computational Imaging: Using AI and computational models to facilitate the acquisition and reconstruction of medical images, including modalities such as microscopy, magnetic resonance imaging, ultrasound and computed tomography.
- Ethical and Regulatory Considerations: Investigating the ethical and regulatory challenges associated with the integration of AI in healthcare, including data privacy, bias mitigation, and compliance with healthcare standards.
- Explainable AI (XAI) in Healthcare: Advancing methods to make AI models interpretable and transparent to clinicians and patients to ensure trust and accountability.
View Medical Biophysics faculty working in this area.
View recent research posters from MBP labs working in this area.

- View your wishlist
- Share on Facebook
- Share on LinkedIn
Certificate Overview
Artificial Intelligence
About the certificate, what you'll learn, required courses.
{{ course.d_course_code }}
{{ course.d_course_name }}
Elective Courses
Sign up with us to receive the latest news about our courses and programs, speaker series, course bundles and more.
- U of T Home
- Current Instructors
- Policies and Guidelines
- Help and Information
- Blueprint Career Services
- Organizational and Corporate Training
- PSE Preparedness
- Knowledge Hub
- Financial Aid
- U of T Alumni Benefit
- Biomanufacturing
- Micro Courses and Micro-Credentials
- Professional Edge Program
- SCS XR Courses
- Passing the CFA® Exams
- Passing the Canadian Securities Course®
- SCS Boot Camps
- Skill Builder Courses
- Health, Environment, and Science
- Life and Leisure
- Philosophy and Law
- University Lecture Series
- Visual Art and Architecture
- Business Analysis
- Entrepreneurship
- Human Resources
- Occupational Health and Safety
- Process Improvement
- Project Management
- Test Preparation
- Career Development
- Workplace Communications
- Creative Non-Fiction
- Escritura Creativa en Español
- Literary Fiction
- Multi-genre
- Poetry and Songwriting
- Popular Fiction
- Stage and Screenwriting
- U of T Summer Writing School
- Writing for Children
- Public Health
- Human Services and Social Work
- Medical Sciences
- Mindfulness
- Continuous Professional Development
- International Pharmacy Graduate Program
- Building Science and Architecture
- Engineering and Applied Science
- Environment and Sustainability
- Information Management
- Information Technology (IT)
- Property & Facilities Management
- Arabic Translation
- Spanish Translation
- Portuguese Translation
- Japanese Translation
- French Translation
- Chinese Translation
- Korean Translation
- Business English for International Professionals
- Learning Design
- Multimedia Journalism
- Communications
- Public Relations
- Partnerships with Associations and Certifying Bodies
- U of T Partnerships
- English Language Program
- Educational Credential Assessment
- Leadership Team
- Academic Leadership
- Teach with us
- Instructor Awards and Recognition
- Instructor Biographies
- Equity, Diversity, and Inclusion Commitments
- Our History
- Media Inquiries
- Curious U Blog
Centre for Ethics, University of Toronto
Where conversations about ethics happen..
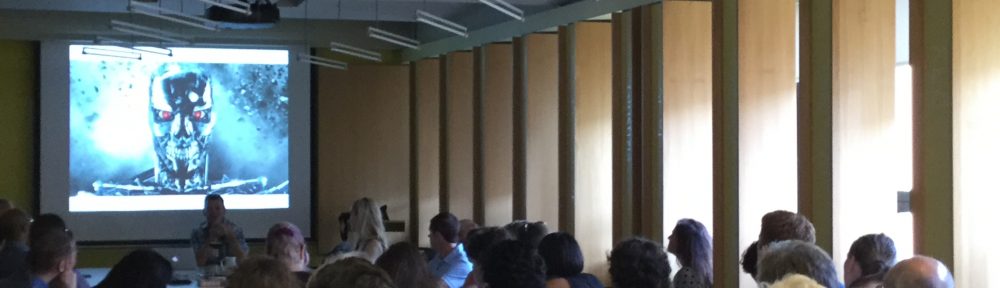
Ethics of AI Lab
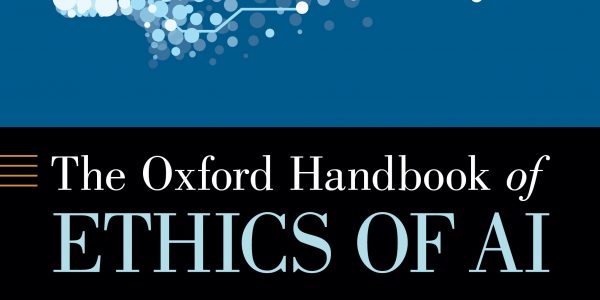
OUR APPROACH
Since 2017, the Ethics of AI Lab at the University of Toronto’s Centre for Ethics has fostered academic and public dialogue about Ethics of AI in Context —the normative dimensions of artificial intelligence and related phenomena in all aspects of private, public, and political life. It is interdisciplinary from the ground up, by pursuing critical analysis from any disciplinary perspective, including STEM, health sciences, social sciences, and—crucially—the humanities, that can shed light on the complex nature of the challenge at hand and on the sustained effort required to address it. The Lab’s Ethics of AI in Context approach to this critical challenge finds expression in the preface to the Oxford Handbook of Ethics of AI (2020; paperback 2021 ):
- it locates ethical analysis of artificial intelligence in the context of other modes of normative analysis, including legal, regulatory, philosophical, and policy approaches,
- it interrogates artificial intelligence within the context of related modes of technological innovation, including machine learning, Big Data, and robotics,
- it is interdisciplinary from the ground up, broadening the conversation about the ethics of artificial intelligence beyond computer science and related fields to include other fields of scholarly endeavor, including the social sciences, humanities, and the professions (law, medicine, engineering, etc.), and
- it invites critical analysis of all aspects of—and participants in—the wide and continuously expanding artificial intelligence complex, from production to commercialization to consumption, from technical experts to venture capitalists to self-regulating professionals to government officials to the general public.
The Ethics of AI Lab also publishes the Handbook’s Online Supplement , which includes the Annotated Bibliograph y of Ethics of AI , with 900+ sources, organized by chapter topic. The Handbook’s online version can be accessed through Oxford Handbooks Online .
- Ethics of AI in Context | Ethics in the City (incl. Sidewalk Toronto) | Ethics of AI in Context: Emerging Scholars
- Ethics of AI Film Series
- Ethics of Artificial Intelligence in Context ( graduate / undergraduate )
- The Future of Work in the Age of AI (undergraduate)
- Bias in Medicine: From Evidence Based Medicine to Artificial Intelligence (undergraduate)
- Visiting Faculty Fellowships | Postdoctoral Fellow in Ethics of Artificial Intelligence | Ethics of AI Graduate Research Fellowships
- Trust and the Ethics of AI (June 2022)
- special issue Critical Analysis of Law: Afrofuturism and the Law (April 2022)
- special issue Critical Analysis of Law: An International & Interdisciplinary Law Review (April 2021)
- video | symposium issue
- program | symposium
- videos | podcasts | journal
- editors’ preface
- online supplement & annotated bibliography
- Ethics of AI Lab Affiliates
- Postdoctoral Fellow in Ethics of Artificial Intelligence
- Ethics of AI Graduate Research Fellows
HOW TO STAY IN TOUCH
- sign up for C4E newsletter
- follow Ethics of AI Lab on twitter
- contact us
- register for events
- donate online
Principal Investigator
- [email protected]
- ashton1anderson
- ashtonanderson
Graduate Students
- [email protected]
- [email protected]
- [email protected]
- [email protected]
- [email protected]
- [email protected]
Undergraduate Students and Interns
- https://github.com/prokopchukdim
- https://github.com/dhkim2810
- [email protected]
- [email protected]
- isaacwaller
- lanaelsanyoura
- [email protected]
let’s connect
Our concentrations, artificial intelligence in healthcare, what is artificial intelligence in healthcare.
This subdiscipline is at the forefront of a new chapter of medicine we are entering and provides students with the opportunity and ability to improve patient care and quality of life. Medical professionals are recognizing the value of acquiring AI skills as their work aligns with colleagues in computer science and engineering to develop and deploy new and exciting tools.
Computer Science and Engineering majors undertaking this field need to be well versed in working with medical data and medical experts. These specialists must be aware of the biases AI tools are likely to introduce to clinical practice and prevent these and other undesirable effects from happening. At the same time medical professionals are eager to acquire the skills that will enable them to be the drivers of the development and deployment of AI into clinical practice. They must also understand what is involved in the creation of these tools so they can understand the limitations.
The proposed AI and Healthcare concentration aims to provide a training background for students who desire to enter the field as either medical experts or computer scientists/engineers. There is currently no program in Canada that is truly joint between the Departments of Computer Science and Medicine that would achieve the rigour required for safe and secure development and deployment of AI in the field of healthcare broadly, making this concentration one of a kind.
Endless Career Opportunities
Discover the endless possibilities to accelerate your career as a world-class innovator.
Career Opportunities in Artificial Intelligence in Healthcare
Data Analytics Lead
Director, Product Development
Junior Data Specialist
Machine Learning Specialist
Machine Learning Team Lead
PhD Student
Principal Research Associate, Data Science and AI
Senior Software Engineer
Senior Software Engineer – Machine Learning
Staff Software Developer
Program Requirements
- 0.5 FCE of coursework in the area of Data Science from an approved list
- 0.5 FCE of coursework in approved AI courses
- 0.5 FCE in approved Group 3 courses (visualization/systems/software engineering) Course groupings can be found on the Computer Science website
- 0.5 FCE of LMP/MHI coursework from an approved list
- 1.0 FCEs required professional courses Communication for Computer Scientists ( CSC 2701H ) and Technical Entrepreneurship ( CSC 2702H )
- An eight-month industrial internship , CSC 2703H (3.5 FCEs). The internship is coordinated by the department and evaluated on a pass/fail basis. ‘Pass’ grades are awarded based on evaluations received from the industry/academic supervisors of the internship project and submission of an appropriately written final report, documenting the applied research internship.
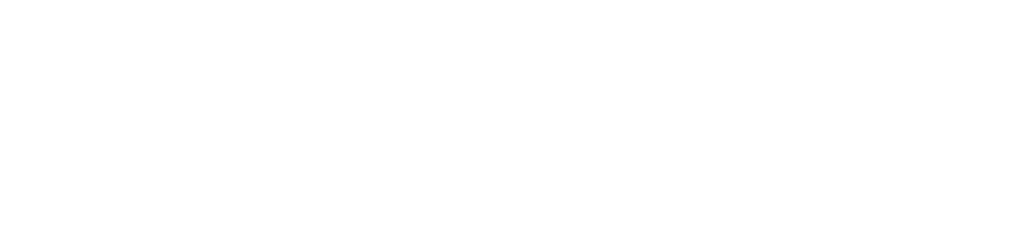
Copyright © 2024 Master of Science in Applied Computing (MScAC) Program, Department of Computer Science, University of Toronto. All rights reserved.
Office of the Vice-Provost, Innovations in Undergraduate Education
Generative artificial intelligence in the classroom: faq’s.
The latest generation of Artificial Intelligence (AI) systems is impacting teaching and learning in many ways, presenting both opportunities and challenges for the ways our course instructors and students engage in learning. At the University of Toronto, we remain committed to providing students with transformative learning experiences and to supporting instructors as they adapt their pedagogy in response to this emerging technology.
Many generative AI systems have become available, including Microsoft Copilot , ChatGPT, Gemini, and others. These AI tools use predictive technology to create or revise written products of all kinds, including essays, computer code, lesson plans, poems, reports, and letters. They also summarize text, respond to questions, and so on. The products that the tools create are generally of good quality, although they can have inaccuracies. We encourage you to try these systems to test their capabilities and limitations.
May 2024: A new institutional website on artificial intelligence was launched. This site provides a space for U of T community members and the public to find academic and research opportunities at the University, information on technologies currently in use, institutional guidelines and policies, and updates on new artificial intelligence activities across the University. Visit https://ai.utoronto.ca/ .
Sample Syllabus Statements
Revised April 2024: The University has created sample statements for instructors to include in course syllabi and course assignments to help shape the message to students about what AI technology is, or is not, allowed. These statements may be used for both graduate and undergraduate level courses.
You may also want to include a statement to the effect that students may be asked to explain their work at a meeting with the instructor. While you can call a student in for such a discussion whether you include a statement to this effect on your syllabus or not, a reminder of this on the syllabus may help remind students that they are responsible for the work they submit for credit.
Microsoft Copilot
In December 2023, Microsoft Copilot (formerly Bing AI) became available to all U of T faculty, librarians, and staff. This protected version is now also available to U of T students. Copilot is an enterprise version of an AI-powered chatbot and search engine which better protects the privacy and security of end users (when users are signed into their U of T account). Copilot, like other generative AI tools, may provide information that is not correct (“hallucinations”), and it is up to each individual user to determine if the results are acceptable. For information and instructions on accessing the enterprise edition, please read and adhere to the Microsoft Copilot guidelines for use .
If you are an instructor who is interested in using generative AI with students or to develop course materials, review the FAQ below for considerations.
Frequently Asked Questions
About generative ai.
Updated: April 10, 2024
Large Language Models are trained to predict the next word in a sentence, given the text that has already been written. Early attempts at addressing this task (such as the next-word prediction on a smartphone keyboard) are only coherent within a few words, but as the sentence continues, these earlier systems quickly digress. A major innovation of models such as GPT is their ability to pay attention to words and phrases which were written much earlier in the text, allowing them to maintain context for much longer and in a sense remember the topic of conversation. This capacity is combined with a training phase that involves looking at billions of pages of text. As a result, models like ChatGPT, Gemini, and their underlying foundational models are good at predicting what words are most likely to come next in a sentence, which results in generally coherent text.
One area where generative AI tools sometimes struggle is in stating facts or quotations accurately. This means that these tools sometimes generate claims that sound real, but to an expert are clearly wrong.
The best way to become familiar with the capabilities and limitations of the tools is to try them. Their capabilities continue to grow, so we recommend continuing to engage with the tools to keep your knowledge of their abilities current.
Instructors are welcome and encouraged to test Microsoft Copilot, ChatGPT, Gemini, Perplexity and other tools, use of which are currently free. You can also test other AI tools to assess their capability, for instance to see how they respond to the assignments used in your courses, the way in which they improve the readability and grammar of a paragraph, or the way they provide answers to typical questions students may have about course concepts. Experimentation is also useful to assess the limits of the tool. However, confidential information should never be entered into unprotected AI tools. Content entered into ChatGPT, Gemini, or other public, unprotected tools may become part of the tool’s dataset. Note that information entered into the protected version of Microsoft Copilot is not used for training.
Updated: April 4, 2023
This is a threshold question that instructors may want to consider. Mainstream media has been covering this issue extensively, and alternate viewpoints are widely available.
Given that generative AI systems are trained on materials that are available online, it is possible that they will repeat biases present online. OpenAI has invested substantial effort into addressing this problem, but it remains a danger with these types of systems. You may also want to familiarize yourself regarding questions about the way the technology was developed and trained (e.g., who were the people who trained it?), the way we use the responses it provides, and the long-term impacts of these technologies on the world.
The Provost is consulting with faculty and staff experts on these larger questions involving generative AI systems, and welcomes debate and discussion on these issues.
There remains significant legal uncertainty concerning the use of generative AI tools in regard to copyright. This is an evolving area, and our understanding will develop as new policies, regulations, and case law become settled. Some of the concerns surrounding generative AI and copyright include:
- Input : The legality of the content used to train AI models is unknown in some cases. There are a number of lawsuits originating from the US that allege Generative AI tools infringe on copyright and it remains unclear if and how the fair use doctrine can be applied. In Canada, there also remains uncertainty regarding the extent to which existing exceptions in the copyright framework, such as fair dealing, apply to this activity.
- Output : Authorship and ownership of works created by AI is unclear. Traditionally, Canadian law has indicated that an author must be a natural person (human) who exercises skill and judgement in the creation of a work. As there are likely to be varying degrees of human input in generated content, it is unclear in Canada how it will be determined who the appropriate author and owner of works are. More recently, the US Copyright Office has published the following guide addressing these issues: Copyright Registration Guidance for Works Containing AI-Generated Materials .
If you have further questions about copyright, please view the U of T Libraries webpage, Generative AI tools and Copyright Considerations for the latest information.
Student Use of Generative AI
Yes. Instructors may wish to use the technology to demonstrate how it can be used productively, or what its limitations are. The U of T Teaching Centres are continuing to develop more information and advice about how you might use generative AI as part of your learning experience design .
You can ask your students to use the protected version of Microsoft Copilot. However, keep in mind that asking or requiring your students to access other tools is complicated by the fact that they have not been vetted by the University for privacy or security. The University generally discourages the use of such systems for instruction until we are assured that the system is protecting any personal data (e.g., the email address used to register on the system). These tools should be considered with the same cautions as other third-party applications that ingest personal data.
If you decide to ask or encourage students to use this an AI system in your courses, there are a few issues to consider before you do so:
- Never input confidential information or student work into an unprotected/unvetted AI tool. All content entered may become part of the tool’s dataset and may inadvertently resurface in response to other prompts.
- Note that if you ask ChatGPT or other tools whether they wrote something, like a paragraph or other work, they will not give you an accurate answer.
- There may be some students who are opposed to using AI tools. Instructors should consider offering alternative forms of assessment for those students who might object to using the tools, assuming that AI is not a core part of the course.
- Instructors should consider indicating on their syllabus that AI tools may be used in the course and, as relevant, identify restrictions to this usage in relation to learning outcomes and assessments.
- Be aware that not all text that generative AI technology produces is factually correct. You may wish to experiment with ChatGPT and other tools to see what kinds of errors it generates; citations are often fabricated, and inaccurate prompts are sometimes taken as fact.
- Different tools will create different responses to the same prompt, with varying quality. You may want to try several different systems to see how they respond.
- There is a risk that Large Language Models may perpetuate biases inherent in the material on which they were trained.
- OpenAI and other companies may change their terms of use without notice. If you plan on using a system in the classroom, consider having a back-up plan. Because of the University’s relationship with Microsoft, use of Microsoft Copilot may help you avoid unexpected and disruptive changes in terms of use.
The University expects students to complete assignments on their own, without any outside assistance, unless otherwise specified. However, for the purposes of transparency and clarity for students, instructors are strongly encouraged to go further and to specify what tools may be used, if any, in completing assessments in their courses. Written assignment instructions should indicate what types of tools are permitted; vague references to not using ‘the internet’ will generally not suffice today.
If you are permitting, or even encouraging, students to use generative AI tools for developing their assignments, be explicit about this on the syllabus. Consider what tools and what use is acceptable. Can students use it for critiquing their work? For editing? For creating an outline? For summarizing sources? For searching the literature (e.g., using Semantic Scholar)? You may also want to ask students to reflect on how they used the tools to improve their writing/learning process.
If adding a prohibition on AI tools to assignment instructions, it is best to suggest that the ‘use of generative AI tools’ is prohibited, as opposed to the use of one particular tool, such as ChatGPT. There are many generative AI tools available today.
The University has created sample language that instructors may include in their course syllabi to clarify for students if the use of generative AI tools for completing course work is acceptable, or not, and why.
We also encourage instructors to include information on assignment instructions to explicitly indicate whether the use of generative AI is acceptable or not.
If an instructor indicates that use of AI tools is not permitted on an assessment, and a student is later found to have used such a tool on the assessment, the instructor should consider meeting with the student as the first step of a process under the Code of Behaviour on Academic Matters .
Some students may ask if they can create their assignment outline or draft using generative AI, and then simply edit the generated first draft; consider before discussing the assignment with your students what your response to this question might be, and perhaps address this question in advance.
You may wish to consider some of the tips for assessment design available on the Centre for Teaching Support & Innovation’s webpage, Generative AI in the Classroom . You might also consider meeting with, or attending a workshop at, your local Teaching Centre to get more information about assignment design. Consider what your learning goals are for the assignment, and how you can best achieve those considering this new technology.
If an instructor specified that no outside assistance was permitted on an assignment, the University would typically consider a student’s use of generative AI to be use of an “unauthorized aid” under the Code of Behaviour on Academic Matters , or as “any other form of cheating”. We are in an interim period where students are receiving conflicting instructions in their various courses as to whether they can use AI or not. We therefore encourage all instructors to be very transparent and clear as to whether use of AI is permitted on any given assessment.
Updated: June 7, 2023
The University does not support the use of AI-detection software programs on student work. None of these software programs have been found to be sufficiently reliable, and they are known to incorrectly flag instances of AI use in human-written content. Some of the AI-detection software programs assess if a piece of writing was generated by AI simply on its level of sophistication.
Sharing your students’ work with these software programs without their permission also raises a range of privacy and ethical concerns.
However, instructors are encouraged to continue to use their traditional methods for detection of potential academic misconduct, including meeting with a student to discuss their assignment in person.
Yes. If you use multiple-choice quizzes/tests, assume that generative AI systems will be able to answer the questions unless they pertain to the specifics of a classroom discussion, the content of which cannot be found on the internet. Some instructors may wish to test the capability of generative AI systems by using their multiple-choice/short answer assessments as prompts, and reviewing responses from a variety of tools (e.g., ChatGPT, Microsoft Copilot, Gemini, Perplexity, Poe Assistant, etc.).
Talking to students about generative AI tools and their limitations will let students know that you are well aware of the technology, and will generate interesting discussion and help to set guidelines for students. Let students know clearly, both verbally and in assignment instructions, what tools may or may not be used to complete the assignment. Advise students of the limitations of the technology, and its propensity to generate erroneous content.
Please note that detection of student use, especially if these tools are used to their best effect, is not possible. Like use of the internet, generative AI use will become ubiquitous.
Visit the Centre for Teaching Support & Innovation’s webpage, Generative AI in the Classroom , for course and assessment design considerations.
Updated: September 29, 2023
Students and faculty can refer to the U of T Libraries Citation Guide for Artificial Intelligence Generative Tools , which provides guidance on how to cite generative AI use in MLA, APA and Chicago Style.
Updated: July 17, 2023
The School of Graduate Studies (SGS) has posted Guidance on the Appropriate Use of Generative Artificial Intelligence in Graduate Theses which will be of interest to graduate students, supervisors, supervisory committee members, Graduate Chairs and Graduate Units.
No. Large Language Model (LLM) technology is at the heart of a variety of generative AI products that are currently available, including writing assistant programs (e.g., Microsoft Copilot, Gemini, and a huge number of others), image creation programs (e.g., DALL-E 3, Midjourney, etc.) and programs to assist people who are creating computer code (e.g., GitHub Copilot). It is also possible for you to build a system which utilizes this underlying technology (GPT-4 or another model) if you are interested in doing so.
It is also worth noting that there are a variety of products (online and mobile apps) that have popped up which use GPT-4, Gemini or other AI models and require paid subscriptions. Some add additional features such as editing tools and templates. However, there are others that do nothing more than the free version but are meant to fool people into paying for a service that is currently free.
Instructor Use of Generative AI
Currently, Microsoft Copilot is the recommended generative AI tool to use at U of T. When a user signs in using University credentials, Microsoft Copilot conforms to U of T’s privacy and security standards (i.e., does not share any data with Microsoft or any other company). It is also free to use. Microsoft Copilot uses OpenAI’s GPT-4 model and performs comparably to ChatGPT. For more information about Copilot, refer to CTSI’s Copilot Tool Guide
The question of copyright ownership remains one of the biggest unknowns when using generative AI tools. The ownership of outputs produced by generative AI is unsettled in law at the current time. If, as an instructor, you would like to use generative AI tools for content generation in your course, consider the following before doing so:
- Have an understanding that while you can use these tools to create content, you may not own or hold copyright in the works generated.
- Be mindful of what you input into tools: never input confidential information or intellectual property you do not have the rights or permissions to (e.g., do not submit student work or questions without their permission). All content entered may become part of the tool’s dataset and may inadvertently resurface in response to other prompts (tools like the protected version of Microsoft Copilot are an exception to this).
- Review the terms of service of each tool, which will establish terms of use and ownership of inputs and outputs (for example, view the Terms of Use for OpenAI ). Note that terms of use are subject to change without notice.
- Be explicit in how you have used these tools in the creation of your work.
View the U of T Libraries, Generative AI tools and Copyright Considerations for more information.
Updated: January 27, 2023
Please note that the instructor is ultimately responsible for ensuring the grade accurately reflects the quality of the student’s work, regardless of the tool used. The University asks that you not submit student work to any third-party software system for grading, or any other purpose, unless the software is approved by the University. A completed assignment, or any student work, is the student’s intellectual property (IP), and should be treated with care.
The University currently has several licensed software tools available for facilitating grading, such as SpeedGrader and Crowdmark. These systems safeguard the student’s IP while also supporting the grading process. In the future these types of systems may include AI-powered grading assistance.
A Provostial Advisory Group on Generative AI in Teaching and Learning was struck in spring 2023 to identify areas in teaching and learning that require an institutional response or guidance. One such example is providing instructors with sample language to include in their course syllabi to clarify for students if the use of generative AI tools for completing course work is acceptable, or not, and why. A Generative AI in Teaching and Learning Working Group, chaired by the Centre for Teaching Support & Innovation, coordinates and plans for instructor resources needed to support generative AI in the classroom. There are also groups around the university (e.g., the libraries ) that are tracking the technology and identifying opportunities and issues that we will need to confront.
Decisions regarding the use of generative AI tools in courses will remain with instructors based on the type of course and assessments within them. Regardless of your stance on this technology, it is important that you discuss it with your students, so they understand the course expectations.
Have feedback or want more information?
If you have any suggestions for teaching and learning resources that would be helpful to you as a course instructor, or if you have any other questions about generative AI at U of T that are not addressed through this FAQ, contact us now:

David Acuna
I am a Senior Research Scientist at NVIDIA Research in the Toronto AI Lab . I earned my PhD in Machine Learning and Computer Vision from the University of Toronto under the supervision of Prof. Sanja Fidler . During this time, I was also affiliated with the Vector Institute for AI . In 2018, I completed my Master’s Degree in Applied Computing at the same institution.
My current work lies at the intersection of Generative AI and Neural Simulation with a focus on Data Generation. I am also particularly interested in finding optimal ways to “adapt” large foundation models to produce enterprise value. More broadly, my research interests span representation learning, model adaptation, controllable generation, synthetic data, optimization and generative modelling. I also have interest in scene understanding and low-level vision.
I am honored to have received the 2020 Microsoft Ada Lovelace Fellowship .
Graduate students interested in internships at NVIDIA are welcome to contact me with a CV and summary of research interests.
--> Date --> News --> Jun, 2023: Papers accepted to ICCV 2023 and TMLR . Dec, 2022: Papers accepted to ECCV 2022 and to CVPR 2022 . Apr, 2022: Papers accepted to ICLR 2022 and to AISTATS 2022 . Nov, 2021: 2 papers accepted to NeurIPS 2021 . May, 2021: f-DAL paper accepted to ICML 2021 . Dec, 2020: 1 paper accepted to NeurIPS 2020 . Mar, 2020: 1 paper accepted to CVPR 2020 (Oral Presentation) . Jan, 2020: Received the 2020 Microsoft Ada Lovelace Fellowship . July, 2019: 3 papers accepted to ICCV 2019 . 2 orals, 1 poster . Jun, 2019: STEAL (CVPR2019) is featured in the media: VentureBeat , Nvidia Developer Center , Edgy and, ... . Jun, 2019: Gave a talk at CVPR2019, Devil is in the Edges (STEAL) . May, 2019: Released Inference Code for STEAL . Mar, 2019: 2 papers accepted to CVPR 2019 . 1 oral , 1 poster. Feb, 2019: 1 paper accepted to ICRA 2019 . Jan, 2019: Polygon-RNN++ and Training DeepNets with Synth Data featured as Breakthrough Developments of 2017-2018 by MIT DeepLearning. . Jun, 2018: Interviewed by UToronto News MScAC Program . --> Jun, 2018: Polygon-RNN++ and Training DeepNets with Synth Data featured as "the 10 coolest papers from CVPR2018" by TowardsDataScience .
Selected Publications
Dreamteacher: pretraining image backbones with deep generative models.

Bridging the Sim2Real gap with CARE: Supervised Detection Adaptation with Conditional Alignment and Reweighting

Visual Learning using Synthetic Data

Neural Light Field Estimation for Street Scenes with Differentiable Virtual Object Insertion

How much more data do I need? Estimating requirements for downstream tasks

Domain Adversarial Training: A Game Perspective

Complex Momentum for Optimization in Games

Federated Learning with Heterogeneous Architectures using Graph HyperNetworks

Towards Optimal Strategies for Training Self-Driving Perception Models in Simulation

Scalable Neural Data Server: A Data Recommender for Transfer Learning

f-Domain-Adversarial Learning: Theory and Algorithms

Variational Amodal Object Completion

Neural Data Server: A Large-Scale Search Engine for Transfer Learning Data

Gated-SCNN: Gated Shape CNNs for Semantic Segmentation
Neural turtle graphics for modeling city road layouts.

Meta-Sim: Learning to Generate Synthetic Datasets

Devil is in the Edges: Learning Semantic Boundaries from Noisy Annotations

Object Instance Annotation with Deep Extreme Level Set Evolution

Structured Domain Randomization: Bridging the Reality Gap by Context-Aware Synthetic Data

Efficient Interactive Annotation of Segmentation Dataset with Polygon-RNN ++

Training Deep Networks with Synthetic Data: Bridging the Reality Gap by Domain Randomization
Direct optimization of the latent representation for fast conditional generation.

Generating Class-conditional Images with Gradient-based Inference

- Engineering
Institute of Biomedical Engineering (BME)
Doctor of Philosophy (PhD)
The PhD program in Biomedical Engineering at the University of Toronto is a research-intensive program that immerses students in the application of biomedical sciences and engineering principles to advance solutions for challenges in human health. Students can be admitted to the PhD program through direct entry after completion of a bachelor’s degree or, alternatively, after the completion of a master’s degree. PhD students receive a guaranteed minimum stipend for four years.
Criteria for success
The PhD program is designed to train students in becoming experts and leaders in research in any setting, such as (but not limited to) academic institutions, industry, non-governmental organizations, and government agencies. The core focus of a doctorate is the development and honing of five essential skills: 1) the acquisition of broad knowledge of the field and hands-on methodology; 2) the ability to create, design, and execute original, innovative and high-quality work; 3) the capacity for critical thinking and synthesis of new and complex ideas; 4) the effective communication of scientific results in all written, verbal and visual formats; and 5) adherence to the highest standards of ethics and integrity. The end-goal of the PhD training is to push the limits of current scientific knowledge, whether through solving previously unresolved questions or creating new solutions for yet-to-be-identified problems. Ideally, the research should be framed carefully within the context of the broader field, showing a deep and integrated understanding of the big picture and where the doctoral research fits. In keeping with the expectations of most PhD programs in STEM in Canada and the United States, PhD candidates in Biomedical Engineering must meet the following requirements for successful completion of the program:
- Completion of compulsory coursework, training activities (e.g., regular supervisory meetings), and exams.
- A written dissertation that demonstrates strong scientific motivation and substantial, cohesive aims to support a rational scientific enquiry.
- An oral defense that demonstrates thorough knowledge of the field, methods employed, contributions to the field, and significance of the work.
- Three first-authored original peer-reviewed research articles published in the leading journals of the field. In many instances, these three articles correspond to the three scientific aims that comprise the main chapters of a cohesive dissertation.
Length of study
Four years (defined as the period for an academically well-prepared student to complete all program requirements while registered full-time).
Admission requirements
- Entry into PhD program after completion of a bachelor’s degree (i.e., direct entry) : A four-year bachelor’s degree in engineering, medicine, dentistry, physical sciences, or biological sciences, or its equivalent , with an average of at least 3.7 on a 4.0 grade point average scale (i.e., A minus) in the final two years of study from a recognized university ; or
- Entry into PhD program after completion of a master’s degree : A master’s degree in engineering, medicine, dentistry, physical sciences, or biological sciences, or its equivalent , with a cumulative average of at least 3.3 on a 4.0 grade point average scale (i.e., B plus) from a recognized university .
- Proof of English-language proficiency is required for all applicants educated outside of Canada whose native language is not English. View the BME English-language requirement policy to determine whether you are required to take a language test and for a list of accepted testing agencies and their minimum scores required for admission.
- Applicants must find a BME faculty supervisor. ( NB : You do not need a supervisor at the time of application. However, admission is competitive and only candidates who have found and secured a research supervisor will be admitted to begin graduate studies.)
- MD/PhD candidates must apply through the MD program
- Possession of the minimum requirements for entry does not guarantee admission
- GRE score is not required
Application procedures
- Complete the online application (see requirements ) and pay the application fee
- Arrange for your English test score to be reported electronically to the University of Toronto by the testing agency if applicable. The institution code for U of T is 0982-00 (there is no need to specify a department)
- Contact the BME Graduate Office to identify your BME faculty supervisor
Rolling admission; multiple rounds with different enrollment capacity in each cycle
Tuition fees
Status | Option | Program Fee |
---|---|---|
Domestic | Full-time: Fall - Winter | |
International | Full-time: Fall - Winter |
Last updated: January, 2022
Program / Topic | Service / Contact |
---|---|
Graduate Admissions | |
Graduate Awards | |
Financial Aid – OSAP, UTAPS | |
Financial Aid – U.S. Citizens | |
Financial Aid – Provinces outside Ontario | |
Tuition & Fees | |
Study Permits & Immigration |
More information
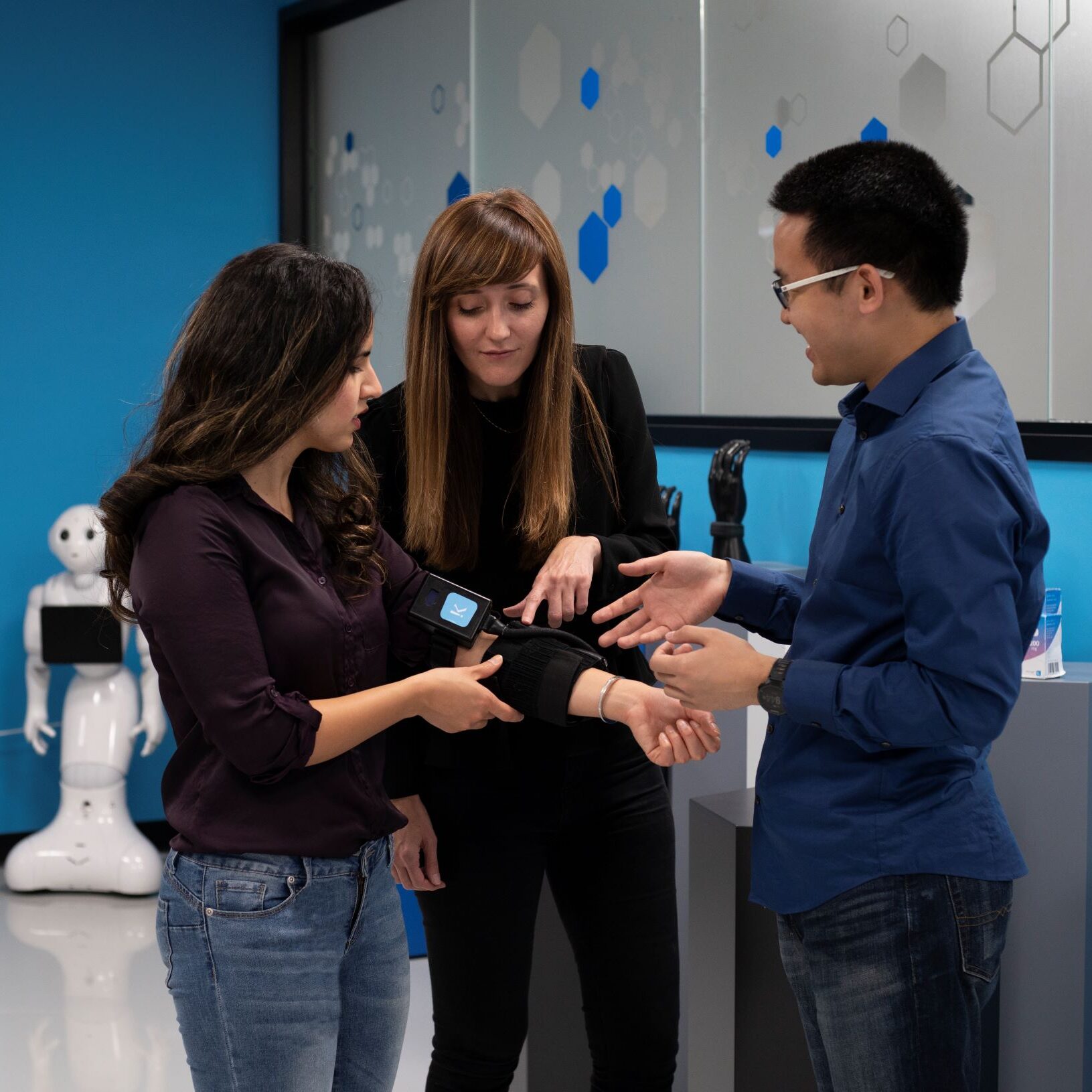
What can I do with my degree? Read our alumni stories
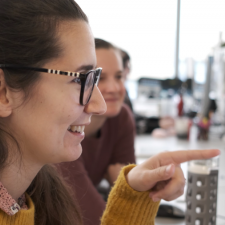
Life at BME, from BME students
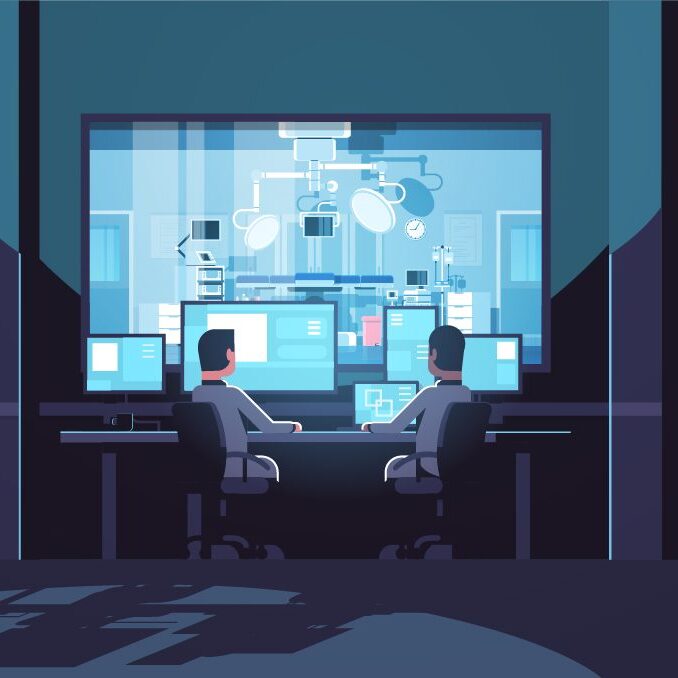
Learn about different research labs
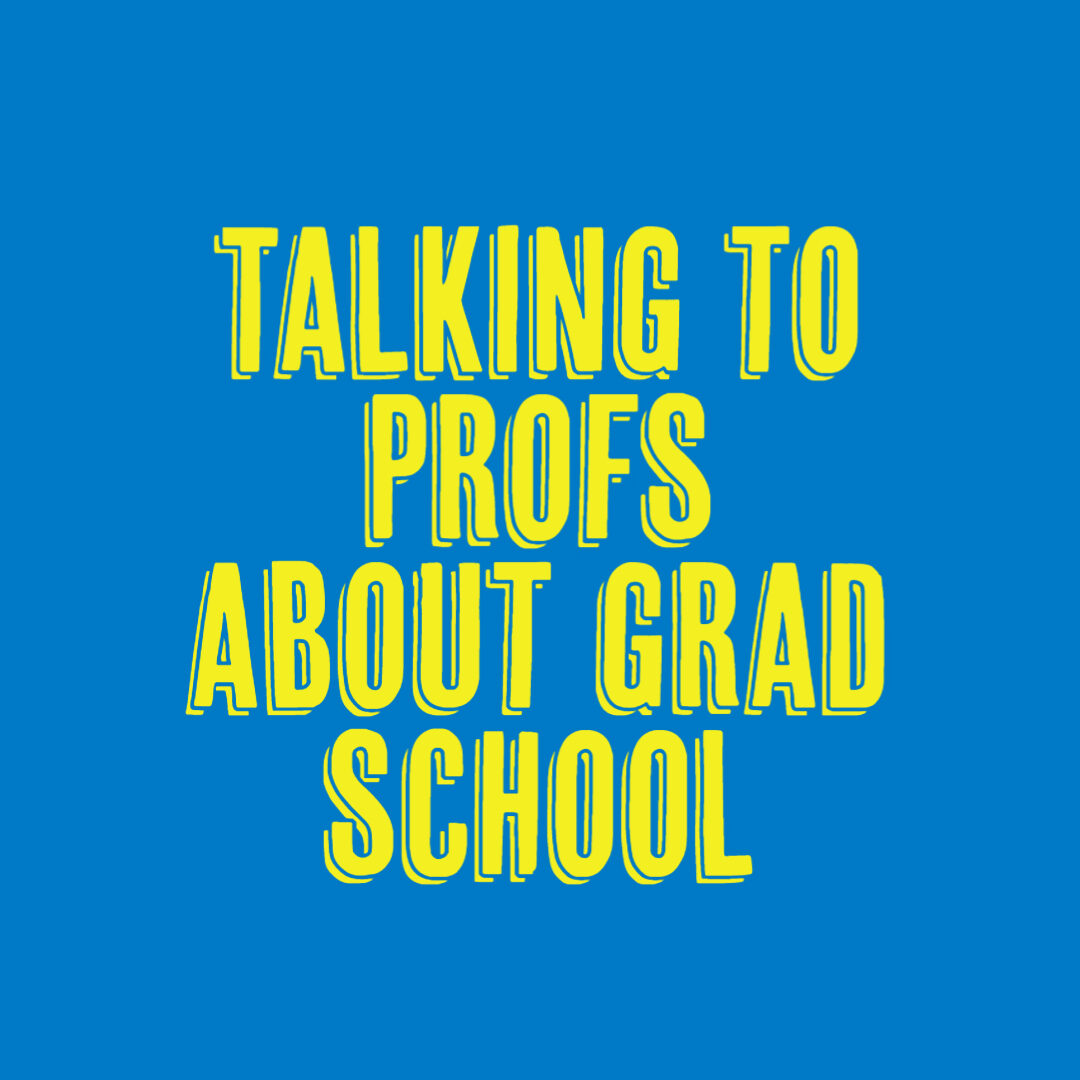
Don't know how to approach a faculty? Listen to our podcasts
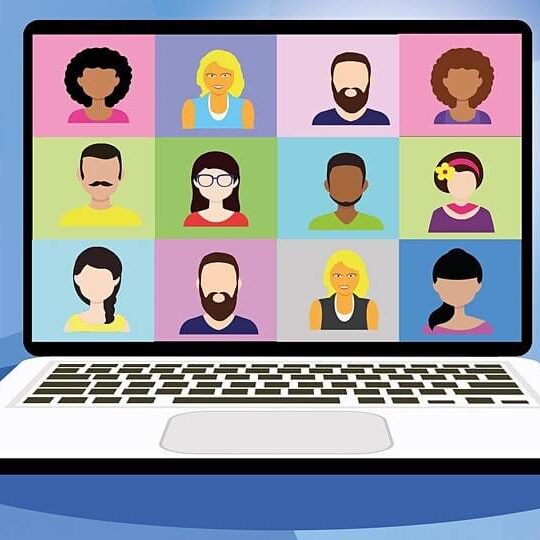
Sign up for an information webinar
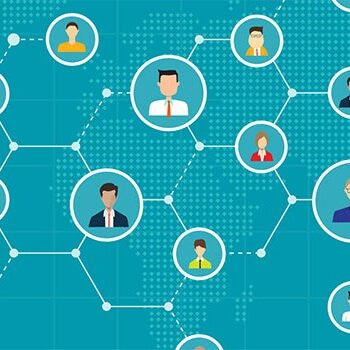
Network with faculty
© 2024 Faculty of Applied Science and Engineering
- U of T Home
- Accessibility
- Student Data Practices
- Website Feedback
Thank you for visiting nature.com. You are using a browser version with limited support for CSS. To obtain the best experience, we recommend you use a more up to date browser (or turn off compatibility mode in Internet Explorer). In the meantime, to ensure continued support, we are displaying the site without styles and JavaScript.
- View all journals
- Explore content
- About the journal
- Publish with us
- Sign up for alerts
- Open access
- Published: 06 August 2024
AI and ethics: Investigating the first policy responses of higher education institutions to the challenge of generative AI
- Attila Dabis ORCID: orcid.org/0000-0003-4924-7664 1 &
- Csaba Csáki ORCID: orcid.org/0000-0002-8245-1002 1
Humanities and Social Sciences Communications volume 11 , Article number: 1006 ( 2024 ) Cite this article
122 Accesses
1 Altmetric
Metrics details
- Science, technology and society
This article addresses the ethical challenges posed by generative artificial intelligence (AI) tools in higher education and explores the first responses of universities to these challenges globally. Drawing on five key international documents from the UN, EU, and OECD, the study used content analysis to identify key ethical dimensions related to the use of generative AI in academia, such as accountability, human oversight, transparency, or inclusiveness. Empirical evidence was compiled from 30 leading universities ranked among the top 500 in the Shanghai Ranking list from May to July 2023, covering those institutions that already had publicly available responses to these dimensions in the form of policy documents or guidelines. The paper identifies the central ethical imperative that student assignments must reflect individual knowledge acquired during their education, with human individuals retaining moral and legal responsibility for AI-related wrongdoings. This top-down requirement aligns with a bottom-up approach, allowing instructors flexibility in determining how they utilize generative AI especially large language models in their own courses. Regarding human oversight, the typical response identified by the study involves a blend of preventive measures (e.g., course assessment modifications) and soft, dialogue-based sanctioning procedures. The challenge of transparency induced the good practice of clear communication of AI use in course syllabi in the first university responses examined by this study.
Similar content being viewed by others
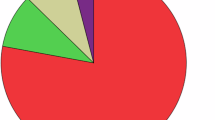
Exploring the impact of artificial intelligence on higher education: The dynamics of ethical, social, and educational implications
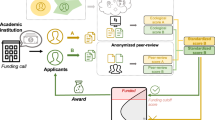

Intersectionality of social and philosophical frameworks with technology: could ethical AI restore equality of opportunities in academia?
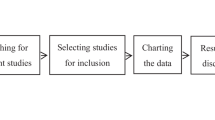
Research on flipped classrooms in foreign language teaching in Chinese higher education
Introduction.
The competition in generative artificial intelligence (AI) ignited by the arrival of ChatGPT, the conversational platform based on a large language model (LLM) in late November 2022 (OpenAI, 2022 ) had a shocking effect even on those who are not involved in the industry (Rudolph et al. 2023 ). Within four months, on 22 March 2023, an open letter was signed by several hundred IT professionals, corporate stakeholders, and academics calling on all AI labs to immediately pause the training of AI systems more powerful than GPT-4 (i.e., those that may trick a human being into believing it is conversing with a peer rather than a machine) for at least six months (Future of Life Institute, 2023 ).
Despite these concerns, competition in generative AI and LLMs does not seem to lose momentum, forcing various social systems to overcome the existential distress they might feel about the changes and the uncertainty of what the future may bring (Roose, 2023 ). Organisations and individuals from different sectors of the economy and various industries are looking for adaptive strategies to accommodate the emerging new normal. This includes lawmakers, international organisations, employers, and employees, as well as academic and higher education institutions (Ray, 2023 ; Wach et al. 2023 ). This fierce competition generates gaps in real-time in everyday and academic life, the latter of which is also trying to make sense of the rapid technological advancement and its effects on university-level education (Perkins, 2023 ). Naturally, these gaps can only be filled, and relevant questions answered much slower by academia, making AI-related research topics timely.
This article aims to reduce the magnitude of these gaps and is intended to help leaders, administrators, teachers, and students better understand the ramifications of AI tools on higher education institutions. It will do so by providing a non-exhaustive snapshot of how various universities around the world responded to generative AI-induced ethical challenges in their everyday academic lives within six-eights months after the arrival of ChatGPT. Thus, the research had asked what expectations and guidelines the first policies introduced into existing academic structures to ensure the informed, transparent, responsible and ethical use of the new tools of generative AI (henceforth GAI) by students and teachers. Through reviewing and evaluating first responses and related difficulties the paper helps institutional decision-makers to create better policies to address AI issues specific to academia. The research reported here thus addressed actual answers to the question of what happened at the institutional (policy) level as opposed to what should happen with the use of AI in classrooms. Based on such a descriptive overview, one may contemplate normative recommendations and their realistic implementability.
Given the global nature of the study’s subject matter, the paper presents examples from various continents. Even though it was not yet a widespread practice to adopt separate, AI-related guidelines, the research focused on universities that had already done so quite early. Furthermore, as best practices most often accrue from the highest-ranking universities, the analysis only considered higher education institutions that were represented among the top 500 universities in the Shanghai Ranking list (containing 3041 Universities at the time), a commonly used source to rank academic excellence. Footnote 1 The main sources of this content analysis are internal documents (such as Codes of Ethics, Academic Regulations, Codes of Practice and Procedure, Guidelines for Students and Teachers or similar policy documents) from those institutions whose response to the GAI challenge was publicly accessible.
The investigation is organised around AI-related ethical dilemmas as concluded from relevant international documents, such as the instruments published by the UN, the EU, and the OECD (often considered soft law material). Through these sources, the study inductively identifies the primary aspects that these AI guidelines mention and can be connected to higher education. Thus it only contains concise references to the main ethical implications of the manifold pedagogical practices in which AI tools can be utilised in the classroom. The paper starts with a review of the challenges posed by AI technology to higher education with special focus on ethical dilemmas. Section 3 covers the research objective and the methodology followed. Section 4 presents the analysis of the selected international documents and establishes a list of key ethical principles relevant in HE contexts and in parallel presents the analysis of the examples distilled from the institutional policy documents and guidelines along that dimension. The paper closes with drawing key conclusions as well as listing limitations and ideas for future research.
Generative AI and higher education: Developments in the literature
General ai-related challenges in the classroom from a historical perspective.
Jacque Ellul fatalistically wrote already in 1954 that the “infusion of some more or less vague sentiment of human welfare” cannot fundamentally alter technology’s “rigorous autonomy”, bringing him to the conclusion that “technology never observes the distinction between moral and immoral use” (Ellul, 1964 , p. 97). Footnote 2 Jumping ahead nearly six decades, the above quote comes to the fore, among others, when evaluating the moral and ethical aspects of the services offered by specific software programs, like ChatGPT. While they might be trained to give ethical answers, these moral barriers can be circumvented by prompt injection (Blalock, 2022 ), or manipulated with tricks (Alberti, 2022 ), so generative AI platforms can hardly be held accountable for the inaccuracy of their responses Footnote 3 or how the physical user who inserted a prompt will make use of the output. Indeed, the AI chatbot is now considered to be a potentially disruptive technology in higher education practices (Farazouli et al. 2024 ).
Educators and educational institution leaders have from the beginning sought solutions on how “to use a variety of the strategies and technologies of the day to help their institutions adapt to dramatically changing social needs” (Miller, 2023 , p. 3). Education in the past had always had high hopes for applying the latest technological advances (Reiser, 2001 ; Howard and Mozejko, 2015 ), including the promise of providing personalised learning or using the latest tools to create and manage courses (Crompton and Burke, 2023 ).
The most basic (and original) educational settings include three components: the blackboard with chalk, the instructor, and textbooks as elementary “educational technologies” at any level (Reiser, 2001 ). Beyond these, one may talk about “educational media” which, once digital technology had entered the picture, have progressed from Computer Based Learning to Learning Management Systems to the use of the Internet, and lately to online shared learning environments with various stages in between including intelligent tutoring system, Dialogue-based Tutoring System, and Exploratory Learning Environment and Artificial Intelligence (Paek and Kim, 2021 ). And now the latest craze is about the generative form of AI often called conversational chatbot (Rudolph et al. 2023 ).
The above-mentioned promises appear to be no different in the case of using generative AI tools in education (Baskara, 2023a ; Mhlanga, 2023 ; Yan et al. 2023 ). The general claim is that GAI chatbots have transformative potential in HE (Mollick and Mollick, 2022 ; Ilieva et al. 2023 ). It is further alleged, that feedback mechanisms supposedly provided by GAI can be used to provide personalised guidance to students (Baskara, 2023b ). Some argue, that “AI education should be expanded and improved, especially by presenting realistic use cases and the real limitations of the technology, so that students are able to use AI confidently and responsibly in their professional future” (Almaraz-López et al. 2023 , p. 1). It is still debated whether the hype is justified, yet the question still remains, how to address the issues arising in the wake of the educational application of GAI tools (Ivanov, 2023 ; Memarian and Doleck, 2023 ).
Generative AI tools, such as their most-known representative, ChatGPT impact several areas of learning and teaching. From the point of view of students, chatbots may help with so-called Self-Regulated or Self-Determined Learning (Nicol and Macfarlane‐Dick, 2006 ; Baskara, 2023b ), where students either dialogue with chatbots or AI help with reviewing student work, even correcting it and giving feedback (Uchiyama et al. 2023 ). There are innovative ideas on how to use AI to support peer feedback (Bauer et al. 2023 ). Some consider that GAI can provide adaptive and personalised environments (Qadir, 2023 ) and may offer personalised tutoring (see, for example, Limo et al. ( 2023 ) on ChatGPT as a virtual tutor for personalized learning experiences). Furthermore, Yan et al. ( 2023 ) lists nine different categories of educational tasks that prior studies have attempted to automate using LLMs: Profiling and labelling (various educational or related content), Detection, Assessment and grading, Teaching support (in various educational and communication activities), Prediction, Knowledge representation, Feedback, Content generation (outline, questions, cases, etc.), Recommendation.
From the lecturers’ point of view, one of the most argued impacts is that assessment practices need to be revisited (Chaudhry et al. 2023 ; Gamage et al. 2023 ; Lim et al. 2023 ). For example, ChatGPT-written responses to exam questions may not be distinguished from student-written answers (Rudolph et al. 2023 ; Farazouli et al. 2024 ). Furthermore, essay-type works are facing special challenges (Sweeney, 2023 ). On the other hand, AI may be utilised to automate a range of educational tasks, such as test question generation, including open-ended questions, test correction, or even essay grading, feedback provision, analysing student feedback surveys, and so on (Mollick and Mollick, 2022 ; Rasul et al. 2023 ; Gimpel et al. 2023 ).
There is no convincing evidence, however, that either lecturers or dedicated tools are able to distinguish AI-written and student-written text with high enough accuracy that can be used to prove unethical behaviour in all cases (Akram, 2023 ). This led to concerns regarding the practicality and ethicality of such innovations (Yan et al. 2023 ). Indeed, the appearance of ChatGPT in higher education has reignited the (inconclusive) debate on the potential and risks associated with AI technologies (Ray, 2023 ; Rudolph et al. 2023 ).
When new technologies appear in or are considered for higher education, debates about their claimed advantages and potential drawbacks heat up as they are expected to disrupt traditional practices and require teachers to adapt to their potential benefits and drawbacks (as collected by Farrokhnia et al. 2023 ). One key area of such debates is the ethical issues raised by the growing accessibility of generative AI and discursive chatbots.
Key ethical challenges posed by AI in higher education
Yan et al. ( 2023 ), while investigating the practicality of AI in education in general, also consider ethicality in the context of educational technology and point out that related debates over the last decade (pre-ChatGPT, so to say), mostly focused on algorithmic ethics, i.e. concerns related to data mining and using AI in learning analytics. At the same time, the use of AI by teachers or, especially, by students has received less attention (or only under the scope or traditional human ethics). However, with the arrival of generative AI chatbots (such as ChatGPT), the number of publications about their use in higher education grew rapidly (Rasul et al. 2023 ; Yan et al. 2023 ).
The study by Chan ( 2023 ) offers a (general) policy framework for higher education institutions, although it focuses on one location and is based on the perceptions of students and teachers. While there are studies that collect factors to be considered for the ethical use of AI in HE, they appear to be restricted to ChatGPT (see, for example, Mhlanga ( 2023 )). Mhlanga ( 2023 ) presents six factors: respect for privacy, fairness, and non-discrimination, transparency in the use of ChatGPT, responsible use of AI (including clarifying its limitations), ChatGPT is not a substitute for human teachers, and accuracy of information. The framework by Chan ( 2023 ) is aimed at creating policies to teach students about GAI and considers three dimensions: pedagogical, governance, and operational. Within those dimensions, ten key areas identified covering ethical concerns such as academic integrity versus academic misconduct and related ethical dilemmas (e.g. cheating or plagiarism), data privacy, transparency, accountability and security, equity in access to AI technologies, critical AI literacy, over-reliance on AI technologies (not directly ethical), responsible use of AI (in general), competencies impeded by AI (such as leadership and teamwork). Baskara ( 2023b ), while also looking at ChatGPT only, considers the following likely danger areas: privacy, algorithmic bias issues, data security, and the potential negative impact of ChatGPT on learners’ autonomy and agency, The paper also questions the possible negative impact of GAI on social interaction and collaboration among learners. Although Yan et al. ( 2023 ) considers education in general (not HE in particular) during its review of 118 papers published since 2017 on the topic of AI ethics in education, its list of areas to look at is still relevant: transparency (of the models used), privacy (related to data collection and use by AI tools), equality (such as availability of AI tools in different languages), and beneficence (e.g. avoiding bias and avoiding biased and toxic knowledge from training data). While systematically reviewing recent publications about AI’s “morality footprint” in higher education, Memarian and Doleck ( 2023 ) consider the Fairness, Accountability, Transparency, and Ethics (FATE) approach as their framework of analyses. They note that “Ethics” appears to be the most used term as it serves as a general descriptor, while the other terms are typically only used in their descriptive sense, and their operationalisation is often lacking in related literature.
Regarding education-related data analytics, Khosravi et al. ( 2022 ) argue that educational technology that involves AI should consider accountability, explainability, fairness, interpretability and safety as key ethical concerns. Ferguson et al. ( 2016 ) also looked at learning analytics solutions using AI and warned of potential issues related to privacy, beneficence, and equality. M.A. Chaudhry et al. ( 2022 ) emphasise that enhancing the comprehension of stakeholders of a new educational AI system is the most important task, which requires making all information and decision processes available to those affected, therefore the key concern is related to transparency according to their arguments.
As such debates continue, it is difficult to identify an established definition of ethical AI in HE. It is clear, however, that the focus should not be on detecting academic misconduct (Rudolph et al. 2023 ). Instead, practical recommendations are required. This is especially true as even the latest studies focus mostly on issues related to assessment practices (Chan, 2023 ; Farazouli et al. 2024 ) and often limit their scope to ChatGPT (Cotton et al. 2024 ) (this specific tool still dominates discourses of LLMs despite the availability of many other solutions since its arrival). At the same time, the list of issues addressed appears to be arbitrary, and most publications do not look at actual practices on a global scale. Indeed, reviews of actual current practices of higher education institutions are rare, and this aspect is not yet the focus of recent HE AI ethics research reports.
As follows from the growing literature and the debate shaping up about the implications of using GAI tools in HE, there was a clear need for a systematic review of how first responses in actual academic policies and guidelines in practice have represented and addressed known ethical principles.
Research objective and methodology
In order to contribute to the debate on the impact of GAI on HE, this study aimed to review how leading institutions had reacted to the arrival of generative AI (such as ChatGPT) and what policies or institutional guidelines they have put in place shortly after. The research intended to understand whether key ethical principles were reflected in the first policy responses of HE institutions and, if yes, how they were handled.
As potential principles can diverge and could be numerous, as well as early guidelines may cover wide areas, the investigation is intended to be based on a few broad categories instead of trying to manage a large set of ideals and goals. To achieve this objective, the research was executed in three steps:
It was started with identifying and collecting general ethical ideals, which were then translated and structured for the context of higher education. A thorough content analysis was performed with the intention to put emphasis on positive values instead of simply focusing on issues or risks and their mitigation.
Given those positive ideals, this research collected actual examples of university policies and guidelines already available: this step was executed from May to July 2023 to find early responses addressing such norms and principles developed by leading HE institutions.
The documents identified were then analysed to understand how such norms and principles had been addressed by leading HE institutions.
As a result, this research managed to highlight and contrast differing practical views, and the findings raise awareness about the difficulties of creating relevant institutional policies. The research considered the ethics of using GAI and not expectations towards their development. The next two sections provide details of the two steps.
Establishing ethical principles for higher education
While the review of relevant ethical and HE literature (as presented above) was not fully conclusive, it highlighted the importance and need for some ideals specific to HE. Therefore, as a first step, this study sought to find highly respected sources of such ethical dimensions by executing a directed content analysis of relevant international regulatory and policy recommendations.
In order to establish what key values and ideas drive the formation of future AI regulations in general, Corrêa et al. ( 2023 ) investigated 200 publications discussing governance policies and ethical guidelines for using AI as proposed by various organisations (including national governments and institutions, civil society and academic organisations, private companies, as well as international bodies). The authors were also interested in whether there are common patterns or missing ideals and norms in this extensive set of proposals and recommendations. As the research was looking for key principles and normative attributes that could form a common ground for the comparison of HE policies, this vast set of documents was used to identify internationally recognised bodies that have potential real influence in this arena and decided to consider the guidelines and recommendations they have put forward for the ethical governance of AI. Therefore, for the purpose of this study, the following sources were selected (some organisations, such as the EU were represented by several bodies):
European Commission ( 2021 ): Regulation of the European Parliament and of the Council Laying Down Harmonised Rules on Artificial Intelligence (Artificial Intelligence Act) and Amending Certain Union Legislative Acts (2021/0106 (COD)) . Footnote 4
European Parliament Committee on Culture and Education ( 2021 ): Report on artificial intelligence in education, culture and the audiovisual sector (2020/2017(INI)) . Footnote 5
High-Level Expert Group on Artificial Intelligence (EUHLEX) ( 2019 ): Ethics Guidelines for Trustworthy AI . Footnote 6
UNESCO ( 2022 ): Recommendation on the Ethics of Artificial Intelligence (SHS/BIO/PI/2021/1) . Footnote 7
OECD ( 2019 ): Recommendation of the Council on Artificial Intelligence (OECD/LEGAL/0449) . Footnote 8
The ethical dilemmas established by these international documents (most of which is considered soft law material) were then used to inductively identify the primary aspects around which the investigation of educational AI principles may be organised.
Among the above documents, the EUHLEX material is the salient one as it contains a Glossary that defines and explains, among others, the two primary concepts that will be used in this paper: “artificial intelligence” and “ethics”. As this paper is, to a large extent, based on the deducted categorisation embedded in these international documents, it will follow suit in using the above terms as EUHLEX did, supporting it with the definitions contained in the other four referenced international documents. Consequently, artificial intelligence (AI) systems are referred to in this paper as software and hardware systems designed by humans that “act in the physical or digital dimension by perceiving their environment through data acquisition, interpreting the collected structured or unstructured data, reasoning on the knowledge, or processing the information, derived from this data and deciding the best action(s) to take to achieve the given goal” (EUHLEX, 2019 ). With regards to ethics, the EUHLEX group defines this term, in general as an academic discipline which is a subfield of philosophy, dealing with questions like “What is a good action?”, “What is the value of a human life?”, “What is justice?”, or “What is the good life?”. It also mentions that academia distinguishes four major fields: (i) Meta-ethics, (ii) normative ethics, (iii) descriptive ethics, and (iv) applied ethics ” (EUHLEX, 2019 , p. 37). Within these, AI ethics belongs to the latter group of applied ethics that focuses on the practical issues raised by the design, development, implementation, and use of AI systems. By extension, the application of AI systems in higher education also falls under the domain of applied ethics.
The selection of sample universities
The collection of cases started with the AI guidelines compiled by the authors as members of the AI Committee at their university from May to July 2023. The AI Committee consisted of 12 members and investigated over 150 cases to gauge international best practices of GAI use in higher education when formulating a policy recommendation for their own university leadership. Given the global nature of the subject matter, examples from various continents were collected. From this initial pool authors narrowed the scope to the Top 500 higher education institutions of the Shanghai Ranking list for this study, as best practices most often accrue from the highest-ranking universities. Finally, only those institutions were included which, at the time of data collection, have indeed had publicly available policy documents or guidelines with clearly identifiable ethical considerations (such as relevant internal documents, Codes of Ethics, Academic Regulations, Codes of Practice and Procedure, or Guidelines for Students and Teachers). By the end of this selection process, 30 samples proved to be substantiated enough to be included in this study (presented in Table 1 ).
All documents were contextually analysed and annotated by both authors individually looking for references or mentions of ideas, actions or recommendations related to the ethical principles identified during the first step of the research. These comments were then compared and commonalities analysed regarding the nature and goal of the ethical recommendation.
Principles and practices of responsible use of AI in higher education
Ai-related ethical codes forming the base of this investigation.
A common feature of the selected AI ethics documents issued by international organisations is that they enumerate a set of ethical principles based on fundamental human values. The referenced international documents have different geographical- and policy scopes, yet they overlap in their categorisation of the ethical dimensions relevant to this research, even though they might use discrepant language to describe the same phenomenon (a factor we took into account when establishing key categories). For example, what EUHLEX dubs as “Human agency and oversight” is addressed by UNESCO under the section called “Human oversight and determination”, yet they essentially cover the same issues and recommended requirements. Among the many principles enshrined in these documents, the research focuses on those that can be directly linked to the everyday education practices of universities in relation to AI tools, omitting those that, within this context, are less situation-dependent and should normally form the overarching basis of the functioning of universities at all times, such as: respecting human rights and fundamental freedoms, refraining from all forms of discrimination, the right to privacy and data protection, or being aware of environmental concerns and responsibilities regarding sustainable development. As pointed out by Nikolinakos ( 2023 ), such principles and values provide essential guidance not only for development but also during the deployment and use of AI systems. Synthesising the common ethical codes in these instruments has led to the following cluster of ethical principles that are directly linked to AI-related higher education practices:
Accountability and responsibility;
Human agency and oversight;
Transparency and explainability
Inclusiveness and diversity.
The following subsections will give a comprehensive definition of these ethical areas and relate them to higher education expectations. Each subsection will first explain the corresponding ethical cluster, then present the specific university examples, concluding with a summary of the identified best practice under that particular cluster.
Accountability and responsibility
Definition in ethical codes and relevance.
The most fundamental requirements, appearing in almost all relevant documents, bring forward the necessity that mechanisms should be implemented to ensure responsibility and accountability for AI systems and their outcomes. These cover expectations both before and after their deployment, including development and use. They entail the basic requirements of auditability (i.e. the enablement of the assessment of algorithms), clear roles in the management of data and design processes (as a means for contributing to the trustworthiness of AI technology), the minimalisation and reporting of negative impacts (focusing on the possibility of identifying, assessing, documenting and reporting on the potential negative impacts of AI systems), as well as the ability of redress (understood as the capability to utilise mechanisms that offer legal and practical remedy when unjust adverse impact occurs) (EUHLEX, 2019 , pp. 19–20).
Additionally, Points 35–36 of the UNESCO recommendations remind us that it is imperative to “attribute ethical and legal responsibility for any stage of the life cycle of AI systems, as well as in cases of remedy related to AI systems, to physical persons or to existing legal entities. AI system can never replace ultimate human responsibility and accountability” (UNESCO, 2022 , p. 22).
The fulfilment of this fundamental principle is also expected from academic authors, as per the announcements of some of the largest publishing houses in the world. Accordingly, AI is not an author or co-author, Footnote 9 and AI-assisted technologies should not be cited as authors either, Footnote 10 given that AI-generated content cannot be considered capable of initiating an original piece of research without direction from human authors. The ethical guidelines of Wiley ( 2023 ) stated that ”[AI tools] also cannot be accountable for a published work or for research design, which is a generally held requirement of authorship, nor do they have legal standing or the ability to hold or assign copyright.” Footnote 11 This research angle carries over to teaching as well since students are also expected to produce outputs that are the results of their own work. Furthermore, they also often do their own research (such as literature search and review) in support of their projects, homework, thesis, and other forms of performance evaluation.
Accountability and responsibility in university first responses
The rapidly changing nature of the subject matter poses a significant challenge for scholars to assess the state of play of human responsibility. This is well exemplified by the reversal of hearts by some Australian universities (see Rudolph et al. ( 2023 ) quoting newspaper articles) who first disallowed the use of AI by students while doing assignments, just to reverse that decision a few months later and replace it by a requirement of disclosing the use of AI in homeworks. Similarly, Indian governments have been oscillating between a non-regulatory approach to foster an “innovation-friendly environment” for their universities in the summer of 2023 (Liu, 2023 ), only to roll back on this pledge a few months later (Dhaor, 2023 ).
Beyond this regulatory entropy, a fundamental principle enshrined in university codes of ethics across the globe is that students need to meet existing rules of scientific referencing and authorship. Footnote 12 In other words, they should refrain from any form of plagiarism in all their written work (including essays, theses, term papers, or in-class presentations). Submitting any work and assessments created by someone or something else (including AI-generated content) as if it was their own usually amounts to either a violation of scientific referencing, plagiarism or is considered to be a form of cheating (or a combination of these), depending on the terminology used by the respective higher education institution.
As a course description of Johns Hopkins puts it, “academic honesty is required in all work you submit to be graded …., you must solve all homework and programming assignments without the help of outside sources (e.g., GAI tools)” (Johns Hopkins University, 2023 ).
The Tokyo Institute of Technology applies a more flexible approach, as they “trust the independence of the students and expect the best use” of AI systems from them based on good sense and ethical standards. They add, however, that submitting reports that rely almost entirely on the output of GenAI is “highly improper, and its continued use is equivalent to one’s enslavement to the technology” (Tokyo Institute of Technology, 2023 ).
In the case of York University, the Senate’s Academic Standards, Curriculum, and Pedagogy Committee clarified in February 2023 that students are not authorised to use “text-, image-, code-, or video-generating AI tools when completing their academic work unless explicitly permitted by a specific instructor in a particular course” (York University Senate, 2023 ).
In the same time frame (6 February 2023), the University of Oxford stated in a guidance material for staff members that “the unauthorised use of AI tools in exams and other assessed work is a serious disciplinary offence” not permitted for students (University of Oxford, 2023b ).
Main message and best practice: honesty and mutual trust
In essence, students are not allowed to present AI-generated content as their own, Footnote 13 and they should have full responsibility and accountability for their own papers. Footnote 14 This is in line with the most ubiquitous principle enshrined in almost all university guidelines, irrespective of AI, that students are expected to complete their tasks based on their own knowledge and skills obtained throughout their education.
Given that the main challenge here is unauthorised use and overreliance on GAI platforms, the best practice answer is for students to adhere to academic honesty and integrity, scientific referencing standards, existing anti-plagiarism rules, and complete university assignments without fully relying on GAI tools, using, first and foremost, their own skills. The only exception is when instructed otherwise by their professors. By extension, preventing overuse and unauthorised use of AI assists students in avoiding undermining their own academic capacity-building efforts.
Human agency and oversight
AI systems have the potential to manipulate and influence human behaviour in ways that are not easily detectable. AI systems must, therefore, follow human-centric design principles and leave meaningful opportunities for human choice and intervention. Such systems should not be able to unjustifiably subordinate, coerce, deceive, manipulate, condition or herd humans (EUHLEX, 2019 , p. 16).
Human oversight thus refers to the capability for human intervention in every decision cycle of the AI system and the ability of users to make informed, autonomous decisions regarding AI systems. This encompasses the ability to choose not to use an AI system in a particular situation or to halt AI-related operations via a “stop” button or a comparable procedure in case the user detects anomalies, dysfunctions and unexpected performance from AI tools (European Commission, 2021 , Art. 14).
The sheer capability of active oversight and intervention vis-á-vis GAI systems is strongly linked to ethical responsibility and legal accountability. As Liao puts it, “the sufficient condition for human beings being rightsholders is that they have a physical basis for moral agency.” (Liao, 2020 , pp. 496–497). Wagner complemented this with the essential point that entity status for non-human actors would help to shield other parties from liability, i.e., primarily manufacturers and users (Wagner, 2018 ). This, in turn, would result in risk externalisation, which serves to minimise or relativise a person’s moral accountability and legal liability associated with wrongful or unethical acts.
Users, in our case, are primarily students who, at times, might be tempted to make use of AI tools in an unethical way, hoping to fulfil their university tasks faster and more efficiently than they could without these.
Human agency and oversight in university first responses
The crucial aspect of this ethical issue is the presence of a “stop” button or a similar regulatory procedure to streamline the operation of GAI tools. Existing university guidelines in this question point clearly in the direction of soft sanctions, if any, given the fact that there is a lack of evidence that AI detection platforms are effective and reliable tools to tell apart human work from AI-generated ones. Additionally, these tools raise some significant implications for privacy and data security issues, which is why university guidelines are particularly cautious when referring to these. Accordingly, the National Taiwan University, the University of Toronto, the University of Waterloo, the University of Miami, the National Autonomous University of Mexico, and Yale, among others, do not recommend the use of AI detection platforms in university assessments. The University of Zürich further added the moral perspective in a guidance note from 13 July 2023, that “forbidding the use of undetectable tools on unsupervised assignments or demanding some sort of honour code likely ends up punishing the honest students” (University of Zürich, 2023 ). Apart from unreliability, the University of Cape Town also drew attention in its guide for staff that AI detection tools may “disproportionately flag text written by non-first language speakers as AI-generated” (University of Cape Town, 2023 , p. 8).
Macquarie University took a slightly more ambiguous stance when they informed their staff that, while it is not “proof” for anything, an AI writing detection feature was launched within Turnitin as of 5 April 2023 (Hillier, 2023 ), claiming that the software has a 97% detection rate with a 1% false positive rate in the tests that they had conducted (Turnitin, 2023 ). Apart from these, Boston University is among the few examples that recommend employing AI detection tools, but only in a restricted manner to ”evaluate the degree to which AI tools have likely been employed” and not as a source for any punitive measures against students (University of Boston, 2023 ). Remarkably, they complement the above with suggestions for a merit-based scoring system, whereby instructors shall treat work by students who declare no use of AI tools as the baseline for grading. A lower baseline is suggested for students who declare the use of AI tools (depending on how extensive the usage was), and for the bottom of this spectrum, the university suggests imposing a significant penalty for low-energy or unreflective reuse of material generated by AI tools and assigning zero points for merely reproducing the output from AI platforms.
A discrepant approach was adopted at the University of Toronto. Here, if an instructor indicates that the use of AI tools is not permitted on an assessment, and a student is later found to have used such a tool nevertheless, then the instructor should consider meeting with the student as the first step of a dialogue-based process under the Code of Behaviour on Academic Matters (the same Code, which categorises the use of ChatGPT and other such tools as “unauthorised aid” or as “any other form of cheating” in case, an instructor specified that no outside assistance was permitted on an assignment) (University of Toronto, 2019 ).
More specifically, Imperial College London’s Guidance on the Use of Generative AI tools envisages the possibility of inviting a random selection of students to a so-called “authenticity interview” on their submitted assignments (Imperial College London, 2023b ). This entails requiring students to attend an oral examination of their submitted work to ensure its authenticity, which includes questions about the subject or how they approached their assignment.
As a rare exception, the University of Helsinki represents one of the more rigorous examples. The “Guidelines for the Use of AI in Teaching at the University of Helsinki” does not lay down any specific procedures for AI-related ethical offences. On the contrary, as para. 7 stipulates the unauthorised use of GAI in any course examination “constitutes cheating and will be treated in the same way as other cases of cheating” (University of Helsinki, 2023 ). Footnote 15
Those teachers who are reluctant to make AI tools a big part of their courses should rather aim to develop course assessment methods that can plausibly prevent the use of AI tools instead of attempting to filter these afterwards. Footnote 16 For example, the Humboldt-Universität zu Berlin instructs that, if possible, oral or practical examinations or written examinations performed on-site are recommended as alternatives to “classical” written home assignments (Humboldt-Universität zu Berlin, 2023a ).
Monash University also mentions some examples in this regard (Monash University, 2023a ), such as: asking students to create oral presentations, videos, and multimedia resources; asking them to incorporate more personal reflections tied to the concepts studied; implementing programmatic assessment that focuses on assessing broader attributes of students, using multiple methods rather than focusing on assessing individual kinds of knowledge or skills using a single assessment method (e.g., writing an essay).
Similarly, the University of Toronto suggest instructors to: ask students to respond to a specific reading that is very new and thus has a limited online footprint; assign group work to be completed in class, with each member contributing; or ask students to create a first draft of an assignment by hand, which could be complemented by a call to explain or justify certain elements of their work (University of Toronto, 2023 ).
Main message and best practice: Avoiding overreaction
In summary, the best practice that can be identified under this ethical dilemma is to secure human oversight through a blend of preventive measures (e.g. a shift in assessment methods) and soft sanctions. Given that AI detectors are unreliable and can cause a series of data privacy issues, the sanctioning of unauthorised AI use should happen on a “soft basis”, as part of a dialogue with the student concerned. Additionally, universities need to be aware and pay due attention to potentially unwanted rebound effects of bona fide measures, such as the merit-based scoring system of the University of Boston. In that case, using different scoring baselines based on the self-declared use of AI could, in practice, generate incentives for not declaring any use of AI at all, thereby producing counter-effective results.
While explainability refers to providing intelligible insight into the functioning of AI tools with a special focus on the interplay between the user’s input and the received output, transparency alludes to the requirement of providing unambiguous communication in the framework of system use.
As the European Commission’s Regulation proposal ( 2021 ) puts it under subchapter 5.2.4., transparency obligations should apply for systems that „(i) interact with humans, (ii) are used to detect emotions or determine association with (social) categories based on biometric data, or (iii) generate or manipulate content (‘deep fakes’). When persons interact with an AI system or their emotions or characteristics are recognised through automated means, people must be informed of that circumstance. If an AI system is used to generate or manipulate image, audio or video content that appreciably resembles authentic content, there should be an obligation to disclose that the content is generated through automated means, subject to exceptions for legitimate purposes (law enforcement, freedom of expression). This allows persons to make informed choices or step back from a given situation.”
People (in our case, university students and teachers) should, therefore, be fully informed when a decision is influenced by or relies on AI algorithms. In such instances, individuals should be able to ask for further explanation from the decision-maker using AI (e.g., a university body). Furthermore, individuals should be afforded the choice to present their case to a dedicated representative of the organisation in question who should have the power to reviset the decision and make corrections if necessary (UNESCO, 2022 , p. 22). Therefore, in the context of courses and other related education events, teachers should be clear about their utilisation of AI during the preparation of the material. Furthermore, instructors must unambiguously clarify ethical AI use in the classroom. Clear communication is essential about whether students have permission to utilise AI tools during assignments and how to report actual use.
As both UN and EU sources point out, raising awareness about and promoting basic AI literacy should be fostered as a means to empower people and reduce the digital divides and digital access inequalities resulting from the broad adoption of AI systems (EUHLEX, 2019 , p. 23; UNESCO, 2022 , p. 34).
Transparency and explainability in university first responses
The implementation of this principle seems to revolve around the challenge of decentralisation of university work, including the respect for teachers’ autonomy.
Teachers’ autonomy entails that teachers can decide if and to what extent they will allow their students to use AI platforms as part of their respective courses. This, however, comes with the essential corollary, that they must clearly communicate their decision to both students and university management in the course syllabus. To support transparency in this respect, many universities decided to establish 3-level- or 4-level admissibility frameworks (and even those who did not establish such multi-level systems, e.g., the University of Toronto, urge instructors to explicitly indicate in the course syllabus the expected use of AI) (University of Toronto, 2023 ).
The University of Auckland is among the universities that apply a fully laissez passer laissez-faire approach in this respect, meaning that there is a lack of centralised guidance or recommendations on this subject. They rather confer all practical decision-making of GAI use on course directors, adding that it is ultimately the student’s responsibility to correctly acknowledge the use of Gen-AI software (University of Auckland, 2023 ). Similarly, the University of Helsinki gives as much manoeuvring space to their staff as to allow them to change the course of action during the semester. As para 1 of their earlier quoted Guidelines stipulates, teachers are responsible for deciding how GAI can be used on a given course and are free to fully prohibit their use if they think it impedes the achievement of the learning objectives.
Colorado State University, for example, provides its teachers with 3 types of syllabus statement options (Colorado State University, 2023 ): (a) the prohibitive statement: whereby any work created, or inspired by AI agents is considered plagiarism and will not be tolerated; (b) the use-with-permission statement: whereby generative AI can be used but only as an exception and in line with the teachers further instruction, and (c) the abdication statement: where the teacher acknowledges that the course grade will also be a reflection of the students ability to harness AI technologies as part of their preparation for their future in a workforce that will increasingly require AI-literacy.
Macquarie University applies a similar system and provides it’s professors with an Assessment Checklist in which AI use can be either “Not permitted” or “Some use permitted” (meaning that the scope of use is limited while the majority of the work should be written or made by the student.), or “Full use permitted (with attribution)”, alluding to the adaptive use of AI tools, where the generated content is edited, mixed, adapted and integrated into the student’s final submission – with attribution of the source (Macquarie University, 2023 ).
The same approach is used at Monash University where generative AI tools can be: (a) used for all assessments in a specific unit; (b) cannot be used for any assessments; (c) some AI tools may be used selectively (Monash University, 2023b ).
The University of Cape Town (UCT) applies a 3-tier system not just in terms of the overall approach to the use or banning of GAI, but also with regard to specific assessment approaches recommended to teachers. As far as the former is concerned, they differentiate between the strategies of: (a) Avoiding (reverting to in-person assessment, where the use of AI isn’t possible); (b) Outrunning (devising an assessment that AI cannot produce); and (c) Embracing (discussing the appropriate use of AI with students and its ethical use to create the circumstances for authentic assessment outputs). The assessment possibilities, in turn, are categorised into easy, medium, and hard levels. Easy tasks include, e.g., generic short written assignments. Medium level might include examples such as personalised or context-based assessments (e.g. asking students to write to a particular audience whose knowledge and values must be considered or asking questions that would require them to give a response that draws from concepts that were learnt in class, in a lab, field trip…etc). In contrast, hard assessments include projects involving real-world applications, synchronous oral assessments, or panel assessments (University of Cape Town, 2023 ).
4-tier-systems are analogues. The only difference is that they break down the “middle ground”. Accordingly, the Chinese University of Hong Kong clarifies that Approach 1 (by default) means the prohibition of all use of AI tools; Approach 2 entails using AI tools only with prior permission; Approach 3 means using AI tools only with explicit acknowledgement; and Approach 4 is reserved for courses in which the use of AI tools is freely permitted with no acknowledgement needed (Chinese University of Hong Kong, 2023 ).
Similarly, the University of Delaware provides course syllabus statement examples for teachers including: (1) Prohibiting all use of AI tools; (2) Allowing their use only with prior permission; (3) Allow their use only with explicit acknowledgement; (4) Freely allow their use (University of Delaware, 2023 ).
The Technical University of Berlin also proposes a 4-tier system but uses a very different logic based on the practical knowledge one can obtain by using GAI. Accordingly, they divide AI tools as used to: (a) acquire professional competence; (b) learn to write scientifically; (c) be able to assess AI tools and compare them with scientific methods; d) professional use of AI tools in scientific work. Their corresponding guideline even quotes Art. 5 of the German Constitution referencing the freedom of teaching ( Freiheit der Lehre ), entailing that teachers should have the ability to decide for themselves which teaching aids they allow or prohibit. Footnote 17
This detailed approach, however, is rather the exception. According to the compilation on 6 May 2023 by Solis ( 2023 ), among the 100 largest German universities, 2% applied a general prohibition on the use of ChatGPT, 23% granted partial permission, 12% generally permitted its use, while 63% of the universities had none or only vague guidelines in this respect.
Main message and best practice: raising awareness
Overall, the best practice answer to the dilemma of transparency is the internal decentralisation of university work and the application of a “bottom-up” approach that respects the autonomy of university professors. Notwithstanding the potential existence of regulatory frameworks that set out binding rules for all citizens of an HE institution, this means providing university instructors with proper manoeuvring space to decide on their own how they would like to make AI use permissible in their courses, insofar as they communicate their decision openly.
Inclusiveness and diversity
Para. 34 of the Report by the European Parliament Committee on Culture and Education ( 2021 ) highlights that inclusive education can only be reached with the proactive presence of teachers and stresses that “AI technologies cannot be used to the detriment or at the expense of in-person education, as teachers must not be replaced by any AI or AI-related technologies”. Additionally, para. 20 of the same document highlights the need to create diverse teams of developers and engineers to work alongside the main actors in the educational, cultural, and audiovisual sectors in order to prevent gender or social bias from being inadvertently included in AI algorithms, systems, and applications.
This approach also underlines the need to consider the variety of different theories through which AI has been developed as a precursor to ensuring the application of the principle of diversity (UNESCO, 2022 , pp. 33–35), and it also recognises that a nuanced answer to AI-related challenges is only possible if affected stakeholders have an equal say in regulatory and design processes. An idea closely linked to the principle of fairness and the pledge to leave no one behind who might be affected by the outcome of using AI systems (EUHLEX, 2019 , pp. 18–19).
Therefore, in the context of higher education, the principle of inclusiveness aims to ensure that an institution provides the same opportunities to access the benefits of AI technologies for all its students, irrespective of their background, while also considering the particular needs of various vulnerable groups potentially marginalised based on age, gender, culture, religion, language, or disabilities. Footnote 18 Inclusiveness also alludes to stakeholder participation in internal university dialogues on the use and impact of AI systems (including students, teachers, administration and leadership) as well as in the constant evaluation of how these systems evolve. On a broader scale, it implies communication with policymakers on how higher education should accommodate itself to this rapidly changing environment (EUHLEX, 2019 , p. 23; UNESCO, 2022 , p. 35).
Inclusiveness and diversity in university first responses
Universities appear to be aware of the potential disadvantages for students who are either unfamiliar with GAI or who choose not to use it or use it in an unethical manner. As a result, many universities thought that the best way to foster inclusive GAI use was to offer specific examples of how teachers could constructively incorporate these tools into their courses.
The University of Waterloo, for example, recommends various methods that instructors can apply on sight, with the same set of tools for all students during their courses, which in itself mitigates the effects of any discrepancies in varying student backgrounds (University of Waterloo, 2023 ): (a) Give students a prompt during class, and the resulting text and ask them to critique and improve it using track changes; (b) Create two distinct texts and have students explain the flaws of each or combine them in some way using track changes; (c) Test code and documentation accuracy with a peer; or (d) Use ChatGPT to provide a preliminary summary of an issue as a jumping-off point for further research and discussion.
The University of Pittsburgh ( 2023 ) and Monash added similar recommendations to their AI guidelines (Monash University, 2023c ).
The University of Cambridge mentions under its AI-deas initiative a series of projects aimed to develop new AI methods to understand and address sensory, neural or linguistic challenges such as hearing loss, brain injury or language barriers to support people who find communicating a daily challenge in order to improve equity and inclusion. As they put it, “with AI we can assess and diagnose common language and communication conditions at scale, and develop technologies such as intelligent hearing aids, real-time machine translation, or other language aids to support affected individuals at home, work or school.” (University of Cambridge, 2023 ).
The homepage of the Technical University of Berlin (Technische Universität Berlin) displays ample and diverse materials, including videos Footnote 19 and other documents, as a source of inspiration for teachers on how to provide an equitable share of AI knowledge for their students (Glathe et al. 2023 ). More progressively, the university’s Institute of Psychology offers a learning modul called “Inclusive Digitalisation”, available for students enrolled in various degree programmes to understand inclusion and exclusion mechanisms in digitalisation. This modul touches upon topics such as barrier-free software design, mechanisms and reasons for digitalised discrimination or biases in corporate practices (their homepage specifically alludes to the fact that input and output devices, such as VR glasses, have exclusively undergone testing with male test subjects and that the development of digital products and services is predominantly carried out by men. The practical ramifications of such a bias result in input and output devices that are less appropriate for women and children) (Technische Universität Berlin, 2023 ).
Columbia recommends the practice of “scaffolding”, which is the process of breaking down a larger assignment into subtasks (Columbia University, 2023 ). In their understanding, this method facilitates regular check-ins and enables students to receive timely feedback throughout the learning process. Simultaneously, the implementation of scaffolding helps instructors become more familiar with students and their work as the semester progresses, allowing them to take additional steps in the case of students who might need more attention due to their vulnerable backgrounds or disabilities to complete the same tasks.
The Humboldt-Universität zu Berlin, in its Recommendations, clearly links the permission of GAI use with the requirement of equal accessibility. They remind that if examiners require students to use AI for an examination, “students must be provided with access to these technologies free of charge and in compliance with data protection regulations” (Humboldt-Universität zu Berlin, 2023b ).
Concurringly, the University of Cape Town also links inclusivity to accessibility. As they put it, “there is a risk that those with poorer access to connectivity, devices, data and literacies will get unequal access to the opportunities being provided by AI”, leading to the conclusion that the planning of the admissible use of GAI on campus should be cognizant of access inequalities (University of Cape Town, 2023 ). They also draw their staff’s attention to a UNESCO guide material containing useful methods to incorporate ChatGPT into the course, including methods such as the “Socratic opponent” (AI acts as an opponent to develop an argument), the “study buddy” (AI helps the student reflect on learning material) or the “dynamic assessor” (AI provides educators with a profile of each student’s current knowledge based on their interactions with ChatGPT) (UNESCO International Institute for Higher Education in Latin America and the Caribbean, 2023 ).
Finally, the National Autonomous University of Mexico’s Recommendations suggest using GAI tools, among others, for the purposes of community development. They suggest that such community-building activities, whether online or in live groups, kill two birds with one stone. On the one hand, they assist individuals in keeping their knowledge up to date with a topic that is constantly evolving, while it offers people from various backgrounds the opportunity to become part of communities in the process where they can share their experiences and build new relations (National Autonomous University of Mexico, 2023 ).
Main message and best practice: Proactive central support and the pledge to leave no one behind
To conclude, AI-related inclusivity for students is best fostered if the university does not leave its professors solely to their own resources to come up with diverging initiatives. The best practice example for this dilemma thus lies in a proactive approach that results in the elaboration of concrete teaching materials (e.g., subscriptions to AI tools to ensure equal accessibility for all students, templates, video tutorials, open-access answers to FAQs…etc.), specific ideas, recommendations and to support specialised programmes and collaborations with an inclusion-generating edge. With centrally offered resources and tools institutions seem to be able to ensure accessability irrespective of students’ background and financial abilities.
Discussion of the First Responses
While artificial intelligence and even its generative form has been around for a while, the arrival of application-ready LLMs – most notably ChatGPT has changed the game when it comes to grammatically correct large-scale and content-specific text generation. This has invoked an immediate reaction from the higher education community as the question arose as to how it may affect various forms of student performance evaluation (such as essay and thesis writing) (Chaudhry et al. 2023 ; Yu, 2023 ; Farazouli et al. 2024 ).
Often the very first reaction (a few months after the announcement of the availability of ChatGPT) was a ban on these tools and a potential return to hand-written evaluation and oral exams. In the institutions investigated under this research, notable examples may be most Australian universities (such as Monash) or even Oxford. On the other hand, even leading institutions have immediately embraced this new tool as a great potential helper of lecturers – the top name here being Harvard. Very early responses thus ranged widely – and have changed fast over the first six-eight months “post-ChatGPT”.
Over time responses from the institutions investigated started to put out clear guidelines and even created dedicated policies or modified existing ones to ensure a framework of acceptable use. The inspiration leading these early regulatory efforts was influenced by the international ethics documents reviewed in this paper. Institutions were aware of and relied on those guidelines. The main goal of this research was to shed light on the questions of how much and in what ways they took them on board regarding first responses. Most first reactions were based on “traditional” AI ethics and understanding of AI before LLMs and the generative revolution. First responses by institutions were not based on scientific literature or arguments from journal publications. Instead, as our results demonstrated it was based on publicly available ethical norms and guidelines published by well-known international organizations and professional bodies.
Conclusions, limitations and future research
Ethical dilemmas discussed in this paper were based on the conceptualisation embedded in relevant documents of various international fora. Each ethical dimension, while multifaceted in itself, forms a complex set of challenges that are inextricably intertwined with one another. Browsing university materials, the overall impression is that Universities primarily aim to explore and harness the potential benefits of generative AI but not with an uncritical mindset. They are focusing on the opportunities while simultaneously trying to address the emerging challenges in the field.
Accordingly, the main ethical imperative is that students must complete university assignments based on the knowledge and skills they acquired during their university education unless their instructors determine otherwise. Moral and legal responsibility in this regard always rests with human individuals. AI agents possess neither the legal standing nor the physical basis for moral agency, which makes them incapable of assuming such responsibilities. This “top-down” requirement is most often complemented by the “bottom-up” approach of providing instructors with proper maneuvering space to decide how they would like to make AI use permissible in their courses.
Good practice in human oversight could thus be achieved through a combination of preventive measures and soft, dialogue-based procedures. This latter category includes the simple act of teachers providing clear, written communications in their syllabi and engaging in a dialogue with their students to provide unambiguous and transparent instructions on the use of generative AI tools within their courses. Additionally, to prevent the unauthorised use of AI tools, changing course assessment methods by default is more effective than engaging in post-assessment review due to the unreliability of AI detection tools.
Among the many ethical dilemmas that generative AI tools pose to social systems, this paper focused on those pertaining to the pedagogical aspects of higher education. Due to this limitation, related fields, such as university research, were excluded from the scope of the analysis. However, research-related activities are certainly ripe for scientific scrutiny along the lines indicated in this study. Furthermore, only a limited set of institutions could be investigated, those who were the ”first respondents” to the set of issues covered by this study. Hereby, this paper hopes to inspire further research on the impact of AI tools on higher education. Such research could cover more institutions, but it would also be interesting to revisit the same institutions again to see how their stance and approach might have changed over time considering how fast this technology evolves and how much we learn about its capabilities and shortcomings.
Data availability
Data sharing is not applicable to this article as no datasets were generated or analysed during the current study. All documents referenced in this study are publicly available on the corresponding websites provided in the Bibliography or in the footnotes. No code has been developed as part of this research.
For the methodology behind the Shanghai Rankings see: https://www.shanghairanking.com/methodology/arwu/2022 . Accessed: 14 November 2023.
While the original French version was published in 1954, the first English translation is dated 1964.
As the evaluation by Bang et al. ( 2023 ) found, ChatGPT is only 63.41% accurate on average in ten different reasoning categories under logical reasoning, non-textual reasoning, and common-sense reasoning, making it an unreliable reasoner.
Source: https://digital-strategy.ec.europa.eu/en/library/proposal-regulation-laying-down-harmonised-rules-artificial-intelligence . Accessed: 14 November 2023.
Source https://www.europarl.europa.eu/doceo/document/A-9-2021-0127_EN.html . Accessed: 14 November 2023.
Source: https://digital-strategy.ec.europa.eu/en/library/ethics-guidelines-trustworthy-ai . Accessed: 14 November 2023.
Source: https://unesdoc.unesco.org/ark:/48223/pf0000381137 . Accessed: 14 November 2023.
Source: https://legalinstruments.oecd.org/en/instruments/OECD-LEGAL-0449#mainText . Accessed: 14 November 2023.
The editors-in-chief of Nature and Science stated that ChatGPT does not meet the standard for authorship: „ An attribution of authorship carries with it accountability for the work, which cannot be effectively applied to LLMs…. We would not allow AI to be listed as an author on a paper we published, and use of AI-generated text without proper citation could be considered plagiarism,” (Stokel-Walker, 2023 ). See also (Nature, 2023 ).
While there was an initial mistake that credited ChatGPT as an author of an academic paper, Elsevier issued a Corrigendum on the subject in February 2023 (O’Connor, 2023 ). Elsevier then clarified in its “Use of AI and AI-assisted technologies in writing for Elsevier” announcement, issued in March 2023, that “Authors should not list AI and AI-assisted technologies as an author or co-author, nor cite AI as an author”. See https://www.elsevier.com/about/policies-and-standards/the-use-of-generative-ai-and-ai-assisted-technologies-in-writing-for-elsevier . Accessed 23 Nov 2023.
The ethical guidelines of Wiley was updated on 28 February 2023 to clarify the publishing house’s stance on AI-generated content.
See e.g.: Section 2.4 of Princeton University’s Academic Regulations (Princeton University, 2023 ); the Code of Practice and Procedure regarding Misconduct in Research of the University of Oxford (University of Oxford, 2023a ); Section 2.1.1 of the Senate Guidelines on Academic Honesty of York University, enumerating cases of cheating (York University, 2011 ); Imperial College London’s Academic Misconduct Policy and Procedures document (Imperial College London, 2023a ); the Guidelines for seminar and term papers of the University of Vienna (Universität Wien, 2016 ); Para 4. § (1) - (4) of the Anti-plagiarism Regulation of the Corvinus University of Budapest (Corvinus University of Budapest, 2018 ), to name a few.
15 Art. 2 (c)(v) of the early Terms of Use of OpenAI Products (including ChatGPT) dated 14 March 2023 clarified the restrictions of the use of their products. Accordingly, users may not represent the output from their services as human-generated when it was not ( https://openai.com/policies/mar-2023-terms/ . Accessed 14 Nov 2023). Higher education institutions tend to follow suit with this policy. For example, the List of Student Responsibilities enumerated under the “Policies and Regulations” of the Harvard Summer School from 2023 reminds students that their “academic integrity policy forbids students to represent work as their own that they did not write, code, or create” (Harvard University, 2023 ).
A similar view was communicated by Taylor & Francis in a press release issued on 17 February 2023, in which they clarified that: “Authors are accountable for the originality, validity and integrity of the content of their submissions. In choosing to use AI tools, authors are expected to do so responsibly and in accordance with our editorial policies on authorship and principles of publishing ethics” (Taylor and Francis, 2023 ).
This is one of the rare examples where the guideline was adopted by the university’s senior management, in this case, the Academic Affairs Council.
It should be noted that abundant sources recommend harnessing AI tools’ opportunities to improve education instead of attempting to ban them. Heaven, among others, advocated on the pages of the MIT Technology Review the use of advanced chatbots such as ChatGPT as these could be used as “powerful classroom aids that make lessons more interactive, teach students media literacy, generate personalised lesson plans, save teachers time on admin” (Heaven, 2023 ).
This university based its policies on the recommendations of the German Association for University Didactics (Deutsche Gesellschaft für Hochschuldidaktik). Consequently, they draw their students’ attention to the corresponding material, see: (Glathe et al. 2023 ).
For a detailed review of such groups affected by AI see the Artificial Intelligence and Democratic Values Index by the Center for AI and Digital Policy at https://www.caidp.org/reports/aidv-2023/ . Accessed 20 Nov 2023.
See for example: https://www.youtube.com/watch?v=J9W2Pd9GnpQ . Accessed: 14 November 2023.
Akram A (2023) An empirical study of AI generated text detection tools. ArXiv Prepr ArXiv231001423. https://doi.org/10.48550/arXiv.2310.01423
Alberti S (2022) Silas Alberti on X: ChatGPT is trained to not be evil. X Formerly Twitter, 1 December 2022. https://t.co/ZMFdqPs17i . Accessed 23 Nov 2023
Almaraz-López C, Almaraz-Menéndez F, López-Esteban C (2023) Comparative study of the attitudes and perceptions of university students in business administration and management and in education toward Artificial Intelligence. Educ. Sci. 13(6):609. https://doi.org/10.3390/educsci13060609
Article Google Scholar
Bang Y, Cahyawijaya S, Lee N et al. (2023) A multitask, multilingual, multimodal evaluation of ChatGPT on reasoning, hallucination, and interactivity. arXiv. https://doi.org/10.48550/arXiv.2302.04023
Baskara FXR (2023a) ChatGPT as a virtual learning environment: multidisciplinary simulations. In: Proceeding of the 3rd International Conference on Innovations in Social Sciences Education and Engineering, Paper 017. https://conference.loupiasconference.orag/index.php/icoissee3/index
Baskara FXR (2023b) The promises and pitfalls of using ChatGPT for self-determined learning in higher education: An argumentative review. Pros. Semin. Nas. Fakultas Tarb. dan. Ilmu Kegur. IAIM Sinjai 2:95–101. https://doi.org/10.47435/sentikjar.v2i0.1825
Bauer E, Greisel M, Kuznetsov I et al. (2023) Using natural language processing to support peer‐feedback in the age of artificial intelligence: A cross‐disciplinary framework and a research agenda. Br. J. Educ. Technol. 54(5):1222–1245. https://doi.org/10.1111/bjet.13336
Blalock D (2022) Here are all the ways to get around ChatGPT’s safeguards: [1/n]. X Formerly Twitter, 13 December 2022. https://twitter.com/davisblalock/status/1602600453555961856 . Accessed 23 Nov 2023
Chan CKY (2023) A comprehensive AI policy education framework for university teaching and learning. Int J. Educ. Technol. High. Educ. 20(1):1–25. https://doi.org/10.1186/s41239-023-00408-3
Chaudhry IS, Sarwary SAM, El Refae GA, Chabchoub H (2023) Time to revisit existing student’s performance evaluation approach in higher education sector in a new era of ChatGPT—A case study. Cogent Educ. 10(1):2210461. https://doi.org/10.1080/2331186x.2023.2210461
Chaudhry MA, Cukurova M, Luckin R (2022) A transparency index framework for AI in education. In: International Conference on Artificial Intelligence in Education. Springer, Cham, Switzerland, pp 195–198. https://doi.org/10.35542/osf.io/bstcf
Chinese University of Hong Kong (2023) Use of Artificial Intelligence tools in teaching, learning and assessments - A guide for students. https://www.aqs.cuhk.edu.hk/documents/A-guide-for-students_use-of-AI-tools.pdf . Accessed 23 Nov 2023
Colorado State University (2023) What should a syllabus statement on AI look like? https://tilt.colostate.edu/what-should-a-syllabus-statement-on-ai-look-like/ . Accessed 23 Nov 2023
Columbia University (2023) Considerations for AI tools in the classroom. https://ctl.columbia.edu/resources-and-technology/resources/ai-tools/ . Accessed 23 Nov 2023
Corrêa NK, Galvão C, Santos JW et al. (2023) Worldwide AI ethics: A review of 200 guidelines and recommendations for AI governance. Patterns 4(10):100857. https://doi.org/10.1016/j.patter.2023.100857
Article PubMed PubMed Central Google Scholar
Corvinus University of Budapest (2018) Anti-Plagiarism rules. https://www.uni-corvinus.hu/contents/uploads/2020/11/I.20_Plagiumszabalyzat_2018_junius_19_EN.6b1.pdf . Accessed 23 Nov 2023
Cotton DR, Cotton PA, Shipway JR (2024) Chatting and cheating: Ensuring academic integrity in the era of ChatGPT. Innov. Educ. Teach. Int 61(2):228–239. https://doi.org/10.1080/14703297.2023.2190148
Crompton H, Burke D (2023) Artificial intelligence in higher education: the state of the field. Int J. Educ. Technol. High. Educ. 20(1):1–22. https://doi.org/10.1186/s41239-023-00392-8
Dhaor A (2023) India will regulate AI, ensure data privacy, says Rajeev Chandrasekhar. Hindustan Times, 12 October 2023. https://www.hindustantimes.com/cities/noida-news/india-will-regulate-ai-ensure-data-privacy-says-rajeev-chandrasekhar-101697131022456.html . Accessed 23 Nov 2023
Ellul J (1964) The technological society. Vintage Books
EUHLEX (2019) Ethics guidelines for trustworthy AI | Shaping Europe’s digital future. https://digital-strategy.ec.europa.eu/en/library/ethics-guidelines-trustworthy-ai . Accessed 23 Nov 2023
European Commission (2021) Proposal for a Regulation laying down harmonised rules on artificial intelligence | Shaping Europe’s digital future. https://digital-strategy.ec.europa.eu/en/library/proposal-regulation-laying-down-harmonised-rules-artificial-intelligence . Accessed 23 Nov 2023
European Parliament - Committee on Culture and Education (2021) Report on artificial intelligence in education, culture and the audiovisual sector | A9-0127/2021. https://www.europarl.europa.eu/doceo/document/A-9-2021-0127_EN.html . Accessed 23 Nov 2023
Farazouli A, Cerratto-Pargman T, Bolander-Laksov K, McGrath C (2024) Hello GPT! Goodbye home examination? An exploratory study of AI chatbots impact on university teachers’ assessment practices. Assess. Eval. High. Educ. 49(3):363–375. https://doi.org/10.1080/02602938.2023.2241676
Farrokhnia M, Banihashem SK, Noroozi O, Wals A (2023) A SWOT analysis of ChatGPT: Implications for educational practice and research. Innov. Educ. Teach. Int 61(3):460–474. https://doi.org/10.1080/14703297.2023.2195846
Ferguson R, Hoel T, Scheffel M, Drachsler H (2016) Guest editorial: Ethics and privacy in learning analytics. J. Learn Anal. 3(1):5–15. https://doi.org/10.18608/jla.2016.31.2
Future of Life Institute (2023) Pause giant AI experiments: An open letter. https://futureoflife.org/open-letter/pause-giant-ai-experiments/ . Accessed 15 Nov 2023
Gamage KA, Dehideniya SC, Xu Z, Tang X (2023) ChatGPT and higher education assessments: more opportunities than concerns? J Appl Learn Teach 6(2). https://doi.org/10.37074/jalt.2023.6.2.32
Gimpel H, Hall K, Decker S, et al. (2023) Unlocking the power of generative AI models and systems such as GPT-4 and ChatGPT for higher education: A guide for students and lecturers. Hohenheim Discussion Papers in Business, Economics and Social Sciences 2023, 02:2146. http://opus.uni-hohenheim.de/frontdoor.php?source_opus=2146&la=en
Glathe A, Mörth M, Riedel A (2023) Vorschläge für Eigenständigkeitserklärungen bei möglicher Nutzung von KI-Tools. European University Viadrina. https://opus4.kobv.de/opus4-euv/files/1326/Forschendes-Lernen-mit-KI_SKILL.pdf . Accessed 23 Nov 2023
Harvard University (2023) Student Responsibilities. Harvard Summer School 2023. https://summer.harvard.edu/academic-opportunities-support/policies-and-regulations/student-responsibilities/ . Accessed 23 Nov 2023
Heaven WD (2023) ChatGPT is going to change education, not destroy it. MIT Technology Review. https://www.technologyreview.com/2023/04/06/1071059/chatgpt-change-not-destroy-education-openai/ . Accessed 14 Nov 2023
Hillier M (2023) Turnitin Artificial Intelligence writing detection. https://teche.mq.edu.au/2023/03/turnitin-artificial-intelligence-writing-detection/ . Accessed 23 Nov 2023
Howard SK, Mozejko A (2015) Considering the history of digital technologies in education. In: Henderson M, Romeo G (eds) Teaching and digital technologies: Big issues and critical questions. Cambridge University Press, Port Melbourne, Australia, pp 157–168. https://doi.org/10.1017/cbo9781316091968.017
Humboldt-Universität zu Berlin (2023a) ChatGPT & Co: Empfehlungen für das Umgehen mit Künstlicher Intelligenz in Prüfungen. https://www.hu-berlin.de/de/pr/nachrichten/september-2023/nr-2397-1 . Accessed 23 Nov 2023
Humboldt-Universität zu Berlin (2023b) Empfehlungen zur Nutzung von Künstlicher Intelligenz in Studienleistungen und Prüfungen an der Humboldt-Universität zu Berlin. https://www.hu-berlin.de/de/pr/nachrichten/september-2023/hu_empfehlungen_ki-in-pruefungen_20230905.pdf . Accessed 23 Nov 2023
Ilieva G, Yankova T, Klisarova-Belcheva S et al. (2023) Effects of generative chatbots in higher education. Information 14(9):492. https://doi.org/10.3390/info14090492
Imperial College London (2023a) Academic misconduct policy and procedure. https://www.imperial.ac.uk/media/imperial-college/administration-and-support-services/registry/academic-governance/public/academic-policy/academic-integrity/Academic-Misconduct-Policy-and-Procedure-v1.3-15.03.23.pdf . Accessed 14 Nov 2023
Imperial College London (2023b) College guidance on the use of generative AI tools. https://www.imperial.ac.uk/about/leadership-and-strategy/provost/vice-provost-education/generative-ai-tools-guidance/ . Accessed 23 Nov 2023
Ivanov S (2023) The dark side of artificial intelligence in higher education. Serv. Ind. J. 43(15–16):1055–1082. https://doi.org/10.1080/02642069.2023.2258799
Johns Hopkins University (2023) CSCI 601.771: Self-supervised Models. https://self-supervised.cs.jhu.edu/sp2023/ . Accessed 23 Nov 2023
Khosravi H, Shum SB, Chen G et al. (2022) Explainable artificial intelligence in education. Comput Educ. Artif. Intell. 3:100074. https://doi.org/10.1016/j.caeai.2022.100074
Liao SM (2020) The moral status and rights of Artificial Intelligence. In: Liao SM (ed) Ethics of Artificial Intelligence. Oxford University Press, pp 480–503. https://doi.org/10.1093/oso/9780190905033.003.0018
Lim T, Gottipati S, Cheong M (2023) Artificial Intelligence in today’s education landscape: Understanding and managing ethical issues for educational assessment. Research Square Preprint. https://doi.org/10.21203/rs.3.rs-2696273/v1
Limo FAF, Tiza DRH, Roque MM et al. (2023) Personalized tutoring: ChatGPT as a virtual tutor for personalized learning experiences. Soc. Space 23(1):293–312. https://socialspacejournal.eu/article-page/?id=176
Google Scholar
Liu S (2023) India’s AI Regulation Dilemma. The Diplomat, 27 October 2023. https://thediplomat.com/2023/10/indias-ai-regulation-dilemma/ . Accessed 23 Nov 2023
Macquarie University (2023) Academic integrity vs the other AI (Generative Artificial Intelligence). https://teche.mq.edu.au/2023/03/academic-integrity-vs-the-other-ai-generative-artificial-intelligence/ . Accessed 14 Nov 2023
Memarian B, Doleck T (2023) Fairness, Accountability, Transparency, and Ethics (FATE) in Artificial Intelligence (AI), and higher education: A systematic review. Comput Educ Artif Intell 100152. https://doi.org/10.1016/j.caeai.2023.100152
Mhlanga D (2023) Open AI in Education, the Responsible and Ethical Use of ChatGPT Towards Lifelong Learning. SSRN Electron J 4354422. https://doi.org/10.2139/ssrn.4354422
Miller GE (2023) eLearning and the Transformation of Higher Education. In: Miller GE, Ives K (eds) Leading the eLearning Transformation of Higher Education. Routledge, pp 3–23. https://doi.org/10.4324/9781003445623-3
Mollick ER, Mollick L (2022) New modes of learning enabled by AI chatbots: Three methods and assignments. SSRN Electron J 4300783. https://doi.org/10.2139/ssrn.4300783
Monash University (2023a) Generative AI and assessment: Designing assessment for achievement and demonstration of learning outcomes. https://www.monash.edu/learning-teaching/teachhq/Teaching-practices/artificial-intelligence/generative-ai-and-assessment . Accessed 23 Nov 2023
Monash University (2023b) Policy and practice guidance around acceptable and responsible use of AI technologies. https://www.monash.edu/learning-teaching/teachhq/Teaching-practices/artificial-intelligence/policy-and-practice-guidance-around-acceptable-and-responsible-use-of-ai-technologies . Accessed 23 Nov 2023
Monash University (2023c) Choosing assessment tasks. https://www.monash.edu/learning-teaching/teachhq/Assessment/choosing-assessment-tasks . Accessed 23 Nov 2023
National Autonomous University of Mexico (2023) Recomendaciones para el uso de Inteligencia Artificial Generativa en la docencia. https://cuaed.unam.mx/descargas/recomendaciones-uso-iagen-docencia-unam-2023.pdf . Accessed 14 Oct 2023
Nature (2023) Tools such as ChatGPT threaten transparent science; here are our ground rules for their use. Nature 613:612. https://doi.org/10.1038/d41586-023-00191-1 . Editorial
Article CAS Google Scholar
Nicol DJ, Macfarlane‐Dick D (2006) Formative assessment and self‐regulated learning: A model and seven principles of good feedback practice. Stud. High. Educ. 31(2):199–218. https://doi.org/10.1080/03075070600572090
Nikolinakos NT (2023) Ethical Principles for Trustworthy AI. In: Nikolinakos NT (ed) EU Policy and Legal Framework for Artificial Intelligence, Robotics and Related Technologies -The AI Act. Springer International Publishing, Cham, Switzerland, pp 101–166. https://doi.org/10.1007/978-3-031-27953-9
O’Connor S (2023) Corrigendum to “Open artificial intelligence platforms in nursing education: Tools for academic progress or abuse?” [Nurse Educ. Pract. 66 (2023) 103537]. Nurse Educ. Pr. 67:103572. https://doi.org/10.1016/j.nepr.2023.103572
OECD (2019) Recommendation of the Council on Artificial Intelligence. https://legalinstruments.oecd.org/en/instruments/OECD-LEGAL-0449#mainText . Accessed 23 Nov 2023
OpenAI (2022) Introducing ChatGPT. https://openai.com/blog/chatgpt . Accessed 14 Nov 2022
Paek S, Kim N (2021) Analysis of worldwide research trends on the impact of artificial intelligence in education. Sustainability 13(14):7941. https://doi.org/10.3390/su13147941
Perkins M (2023) Academic Integrity considerations of AI Large Language Models in the post-pandemic era: ChatGPT and beyond. J. Univ. Teach. Learn Pr. 20(2):07. https://doi.org/10.53761/1.20.02.07
Princeton University (2023) Academic Regulations: Rights, rules, responsibilities. https://rrr.princeton.edu/2023/students-and-university/24-academic-regulations . Accessed 23 Nov 2023
Qadir J (2023) Engineering education in the era of ChatGPT: Promise and pitfalls of generative AI for education. In: 2023 IEEE Global Engineering Education Conference (EDUCON). IEEE, pp 1–9. https://doi.org/10.1109/educon54358.2023.10125121
Rasul T, Nair S, Kalendra D et al. (2023) The role of ChatGPT in higher education: Benefits, challenges, and future research directions. J. Appl Learn Teach. 6(1):41–56. https://doi.org/10.37074/jalt.2023.6.1.29
Ray PP (2023) ChatGPT: A comprehensive review on background, applications, key challenges, bias, ethics, limitations and future scope. Internet Things Cyber-Phys. Syst. 3:121–154. https://doi.org/10.1016/j.iotcps.2023.04.003
Reiser RA (2001) A history of instructional design and technology: Part I: A history of instructional media. Educ. Technol. Res Dev. 49(1):53–64. https://doi.org/10.1007/BF02504506
Roose K (2023) GPT-4 is exciting and scary. New York Times, 15 March 2023. https://www.nytimes.com/2023/03/15/technology/gpt-4-artificial-intelligence-openai.html . Accessed 23 Nov 2023
Rudolph J, Tan S, Tan S (2023) War of the chatbots: Bard, Bing Chat, ChatGPT, Ernie and beyond. The new AI gold rush and its impact on higher education. J. Appl Learn Teach. 6(1):364–389. https://doi.org/10.37074/jalt.2023.6.1.23
Solis T (2023) Die ChatGPT-Richtlinien der 100 größten deutschen Universitäten. Scribbr, 6 May 2023. https://www.scribbr.de/ki-tools-nutzen/chatgpt-universitaere-richtlinien/ . Accessed 23 Nov 2023
Stokel-Walker C (2023) ChatGPT listed as author on research papers: Many scientists disapprove. Nature 613:620–621. https://doi.org/10.1038/d41586-023-00107-z
Article ADS CAS PubMed Google Scholar
Sweeney S (2023) Who wrote this? Essay mills and assessment – Considerations regarding contract cheating and AI in higher education. Int J. Manag Educ. 21(2):100818. https://doi.org/10.1016/j.ijme.2023.100818
Taylor and Francis (2023) Taylor & Francis clarifies the responsible use of AI tools in academic content creation. Taylor Francis Newsroom, 17 February 2023. https://newsroom.taylorandfrancisgroup.com/taylor-francis-clarifies-the-responsible-use-of-ai-tools-in-academic-content-creation/ . Accessed 23 Nov 2023
Technische Universität Berlin (2023) Inklusive Digitalisierung Modul. https://moseskonto.tu-berlin.de/moses/modultransfersystem/bolognamodule/beschreibung/anzeigen.html?nummer=51021&version=2&sprache=1 . Accessed 05 Aug 2024
Tokyo Institute of Technology (2023) Policy on Use of Generative Artificial Intelligence in Learning. https://www.titech.ac.jp/english/student/students/news/2023/066592.html . Accessed 23 Nov 2023
Turnitin (2023) Turnitin announces AI writing detector and AI writing resource center for educators. https://www.turnitin.com/press/turnitin-announces-ai-writing-detector-and-ai-writing-resource-center-for-educators . Accessed 14 Nov 2023
Uchiyama S, Umemura K, Morita Y (2023) Large Language Model-based system to provide immediate feedback to students in flipped classroom preparation learning. ArXiv Prepr ArXiv230711388. https://doi.org/10.48550/arXiv.2307.11388
UNESCO (2022) Recommendation on the ethics of Artificial Intelligence. https://unesdoc.unesco.org/ark:/48223/pf0000381137 . Accessed 23 Nov 2023
UNESCO International Institute for Higher Education in Latin America and the Caribbean (2023) ChatGPT and Artificial Intelligence in higher education. https://www.iesalc.unesco.org/wp-content/uploads/2023/04/ChatGPT-and-Artificial-Intelligence-in-higher-education-Quick-Start-guide_EN_FINAL.pdf . Accessed 14 Nov 2023
Universität Wien (2016) Guidelines for seminar and term papers. https://bda.univie.ac.at/fileadmin/user_upload/p_bda/Teaching/PaperGuidlines.pdf . Accessed 23 Nov 2023
University of Auckland (2023) Advice for students on using Generative Artificial Intelligence in coursework. https://www.auckland.ac.nz/en/students/forms-policies-and-guidelines/student-policies-and-guidelines/academic-integrity-copyright/advice-for-student-on-using-generative-ai.html . Accessed 24 Nov 2023
University of Boston (2023) Using Generative AI in coursework. https://www.bu.edu/cds-faculty/culture-community/gaia-policy/ . Accessed 23 Nov 2023
University of Cambridge (2023) Artificial Intelligence and teaching, learning and assessment. https://www.cambridgeinternational.org/support-and-training-for-schools/artificial-intelligence/ . Accessed 23 Nov 2023
University of Cape Town (2023) Staff Guide - Assessment and academic integrity in the age of AI. https://docs.google.com/document/u/0/d/1o5ZIOBjPsP6Nh2VIlM56_kcuqB-Y7xTf/edit?pli=1&usp=embed_facebook . Accessed 14 Nov 2023
University of Delaware (2023) Considerations for using and addressing advanced automated tools in coursework and assignments. https://ctal.udel.edu/advanced-automated-tools/ . Accessed 14 Nov 2023
University of Helsinki (2023) Using AI to support learning | Instructions for students. https://studies.helsinki.fi/instructions/article/using-ai-support-learning . Accessed 24 Nov 2023
University of Oxford (2023a) Code of practice and procedure on academic integrity in research. https://hr.admin.ox.ac.uk/academic-integrity-in-research . Accessed 23 Nov 2023
University of Oxford (2023b) Unauthorised use of AI in exams and assessment. https://academic.admin.ox.ac.uk/article/unauthorised-use-of-ai-in-exams-and-assessment . Accessed 23 Nov 2023
University of Pittsburgh (2023) Generative AI Resources for Faculty. https://teaching.pitt.edu/generative-ai-resources-for-faculty/ . Accessed 23 Nov 2023
University of Toronto (2019) Code of behaviour on academic matters. https://governingcouncil.utoronto.ca/secretariat/policies/code-behaviour-academic-matters-july-1-2019 . Accessed 23 Nov 2023
University of Toronto (2023) ChatGPT and Generative AI in the classroom. https://www.viceprovostundergrad.utoronto.ca/strategic-priorities/digital-learning/special-initiative-artificial-intelligence/ . Accessed 20 Nov 2023
University of Waterloo (2023) Artificial Intelligence at UW. https://uwaterloo.ca/associate-vice-president-academic/artificial-intelligence-uw . Accessed 23 Nov 2023
University of Zürich (2023) ChatGPT. https://ethz.ch/en/the-eth-zurich/education/educational-development/ai-in-education/chatgpt.html . Accessed 23 Nov 2023
Wach K, Duong CD, Ejdys J et al. (2023) The dark side of generative artificial intelligence: A critical analysis of controversies and risks of ChatGPT. Entrep. Bus. Econ. Rev. 11(2):7–24. https://doi.org/10.15678/eber.2023.110201
Wagner G (2018) Robot liability. SSRN Electron J 3198764. https://doi.org/10.2139/ssrn.3198764
Wiley (2023) Best practice guidelines on research integrity and publishing ethics. https://authorservices.wiley.com/ethics-guidelines/index.html . Accessed 20 Nov 2023
Yan L, Sha L, Zhao L et al. (2023) Practical and ethical challenges of large language models in education: A systematic scoping review. Br. J. Educ. Technol. 55(1):90–112. https://doi.org/10.1111/bjet.13370
York University (2011) Senate Policy on Academic Honesty. https://www.yorku.ca/secretariat/policies/policies/academic-honesty-senate-policy-on/ . Accessed 23 Nov 2023
York University Senate (2023) Academic Integrity and Generative Artificial Intelligence Technology. https://www.yorku.ca/unit/vpacad/academic-integrity/wp-content/uploads/sites/576/2023/03/Senate-ASCStatement_Academic-Integrity-and-AI-Technology.pdf . Accessed 23 Nov 2023
Yu H (2023) Reflection on whether Chat GPT should be banned by academia from the perspective of education and teaching. Front Psychol. 14:1181712. https://doi.org/10.3389/fpsyg.2023.1181712
Download references
The authors have received no funding, grants, or other support for the research reported here. Open access funding provided by Corvinus University of Budapest.
Author information
Authors and affiliations.
Covinus University of Budapest, Budapest, Hungary
Attila Dabis & Csaba Csáki
You can also search for this author in PubMed Google Scholar
Contributions
AD had established the initial idea and contributed to the collection of ethical standards as well as to the collection of university policy documents. Also contributed to writing the initial draft and the final version. CsCs had reviewed and clarified the initial concept and then developed the first structure including methodological considerations. Also contributed to the collection of university policy documents as well as to writing the second draft and the final version.
Corresponding author
Correspondence to Attila Dabis .
Ethics declarations
Competing interests.
The authors declare no competing interests.
Ethical approval
This research did not involve any human participants or animals and required no ethical approval.
Informed consent
This article does not contain any studies with human participants performed by any of the authors. No consent was required as no private data was collected or utilized.
Additional information
Publisher’s note Springer Nature remains neutral with regard to jurisdictional claims in published maps and institutional affiliations.
Rights and permissions
Open Access This article is licensed under a Creative Commons Attribution 4.0 International License, which permits use, sharing, adaptation, distribution and reproduction in any medium or format, as long as you give appropriate credit to the original author(s) and the source, provide a link to the Creative Commons licence, and indicate if changes were made. The images or other third party material in this article are included in the article’s Creative Commons licence, unless indicated otherwise in a credit line to the material. If material is not included in the article’s Creative Commons licence and your intended use is not permitted by statutory regulation or exceeds the permitted use, you will need to obtain permission directly from the copyright holder. To view a copy of this licence, visit http://creativecommons.org/licenses/by/4.0/ .
Reprints and permissions
About this article
Cite this article.
Dabis, A., Csáki, C. AI and ethics: Investigating the first policy responses of higher education institutions to the challenge of generative AI. Humanit Soc Sci Commun 11 , 1006 (2024). https://doi.org/10.1057/s41599-024-03526-z
Download citation
Received : 21 February 2024
Accepted : 29 July 2024
Published : 06 August 2024
DOI : https://doi.org/10.1057/s41599-024-03526-z
Share this article
Anyone you share the following link with will be able to read this content:
Sorry, a shareable link is not currently available for this article.
Provided by the Springer Nature SharedIt content-sharing initiative
Quick links
- Explore articles by subject
- Guide to authors
- Editorial policies

- All Opportunities
- Staff Opportunities
- Faculty Opportunities
- Librarian Opportunities
- CUPE 3902 (Unit 3) Opportunities
- CUPE 3902 (Unit 3) Emergency Posting Opportunities
- UTemp (Short-Term)
- Affiliated Hospital Opportunities
- Student Opportunities
- Federated Colleges Opportunities
- Frequently Asked Questions
- Working at U of T
- HR & Equity
- Workplace Inclusion
- Join Our Talent Network
- USW Staff Appointed Opportunities
People Strategy, Equity & Culture
Communications Officer, Long COVID Web
Date Posted: 08/07/2024 Req ID: 36631 Faculty/Division: Dalla Lana School of Public Health Department: Inst of Health Policy, Mgmt & Evaluation Campus: St. George (Downtown Toronto) Position Number: 00056278
Description:
About us: The Dalla Lana School of Public Health is a Faculty of the University of Toronto that originated as one of the Schools of Hygiene begun by the Rockefeller Foundation in 1927. The School, which plays a criticalrole in the COVID-19 pandemic response, went through a dramatic renaissance after the 2003 SARS crisis and it is now the largest public health school in Canada, with more than 850 faculty, 1,000 students, and research and training partnerships with institutions throughout Toronto and the world. With $76 million in research funding per year, including more than $31.5 million held at DLSPH, the School contributes to improving population health and health policy and health systems through discoveries and innovation in data science and AI, maternal, child and reproductive health, climate change [response], implementation and improvement sciences, preventable disease through vaccines and prevention through [and] wellness such as with diabetes, comparativehealth policy, sustainable and equitable health systems, global and Indigenous health, among many other areas. Your opportunity: The Institute of Health Policy, Management and Evaluation (IHPME), a graduate unit within DLSPH, has the largest and most productive group of scholars working in health policy, health services, health informatics, clinical epidemiology and health care research in Canada today. Our students represent all sectors of the health care system; we have consistently been able to attract exceptional applicants from diverse backgrounds with a wealth of experiences. Our alumni have moved into leadership positions throughout the health care system and remain actively involved in ensuring we achieveour goals. And, finally, our donors and partners have been very generous in terms of developing and supporting new initiatives and addressing the financial needs of our students. IHPME hosts the knowledge mobilization team for the Canadian Institutes of Health Research (CIHR)–funded national research network on Long COVID involving scientists, patients, clinicians and decision makers – Long COVID Web (LCW). As the Communications Officer for LCW, you will lead in the planning, development, and execution of Long-COVID web’s key marketing, event & social media strategies. Working collaboratively with the knowledge mobilization team, the operations team based at University Health Network and members of the network across Canada, you’ll create high quality and high impact communication solutions that promote Long COVID web and engage with stakeholders. Your exceptional digital media skills, proven track record of success and passion for tactful and strategic marketing will bekey to advancing our institutes overall goals and objectives. Your responsibilities will include:
- Developing and implementing communications and marketing plans that support strategic objectives
- Developing and implementing community engagement strategies and plans including the production of promotional and outreach materials
- Promoting programs and service offerings to internal and/or external contacts
- Analyzing data to determine trends and recommend target markets
- Conceptualizing, organizing, and executing event activities
- Writing story pitches and other media correspondence
- Creating and maintaining presence on social media and tracking social media analytics
- Building and strengthening relationships with stakeholders and partners of strategic importance
Essential Qualifications:
- Bachelor's Degree in Communications, public relations, marketing, journalism or related discipline or acceptable combination of equivalent experience
- Minimum four (4) years experience working in marketing, communications and/or public relations.
- Experience developing and implementing a combination of communications, marketing, and community engagement strategies.
- Experience working with social media platforms (Facebook, Twitter, Instagram)
- Demonstrated experience with social media and digital communications in a professional setting, including tracking and reporting on meaningful metrics
- Experience with planning and coordinating large scale events and conferences
- Experience maintaining good working relationships with communication leads and generating positive media coverage
- Working knowledge of electronic, print media and marketing techniques
- Proficiency with Microsoft Office Suite Applications (Word, Excel, PowerPoint), Web content management and design software including Adobe Creative Suite and/or Canva
- Excellent attention to detail and solid copyediting and proofreading skills
- Understanding the needs and sensitivities of different audiences and adapting appropriate writing style and content
- High standard of professionalism with superior communication skills (Oral and written)
- Strong interpersonal and relationship building skills
- Excellent organizational and time management skills
- Ability to deliver in a fast-paced, deadline driven environment
To be successful in this role you will be:
- Communicator
- Possess a positive attitude
- Resourceful
This is a term position of 3 years.
Closing Date: 08/23/2024, 11:59PM ET Employee Group: USW Appointment Type : Grant - Term Schedule: Full-Time Pay Scale Group & Hiring Zone: USW Pay Band 11 -- $75,223. with an annual step progression to a maximum of $96,196. Pay scale and job class assignment is subject to determination pursuant to the Job Evaluation/Pay Equity Maintenance Protocol. Job Category: Communication/Media/Public Relations Recruiter: Andrea Varicak
Lived Experience Statement Candidates who are members of Indigenous, Black, racialized and 2SLGBTQ+ communities, persons with disabilities, and other equity deserving groups are encouraged to apply, and their lived experience shall be taken into consideration as applicable to the posted position.
All qualified candidates are encouraged to apply; however, Canadians and permanent residents will be given priority.
Diversity Statement
The University of Toronto embraces Diversity and is building a culture of belonging that increases our capacity to effectively address and serve the interests of our global community. We strongly encourage applications from Indigenous Peoples, Black and racialized persons, women, persons with disabilities, and people of diverse sexual and gender identities. We value applicants who have demonstrated a commitment to equity, diversity and inclusion and recognize that diverse perspectives, experiences, and expertise are essential to strengthening our academic mission. As part of your application, you will be asked to complete a brief Diversity Survey. This survey is voluntary. Any information directly related to you is confidential and cannot be accessed by search committees or human resources staff. Results will be aggregated for institutional planning purposes. For more information, please see http://uoft.me/UP .
Accessibility Statement
The University strives to be an equitable and inclusive community, and proactively seeks to increase diversity among its community members. Our values regarding equity and diversity are linked with our unwavering commitment to excellence in the pursuit of our academic mission. The University is committed to the principles of the Accessibility for Ontarians with Disabilities Act (AODA). As such, we strive to make our recruitment, assessment and selection processes as accessible as possible and provide accommodations as required for applicants with disabilities. If you require any accommodations at any point during the application and hiring process, please contact [email protected] .
Job Segment: Marketing Communications, Event Marketing, PR, Digital Media, Communications, Marketing, Publishing
- Accessibility
- Land Acknowledgment
- utoronto.ca
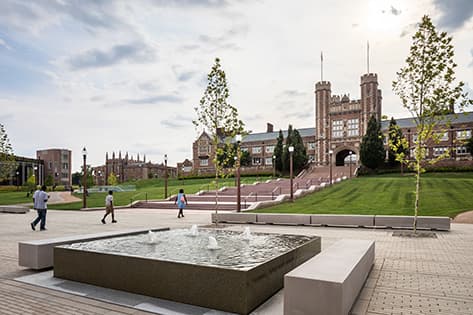
It's easy to start your application.
Trending Searches
- graduate admissions
- academic programs
- financial aid
- academic calendar
- maps & directions
- summer school
Humans change their own behavior when training AI
McKelvey’s Chien-Ju Ho working with Art & Sciences’ Wouter Kool, DCDS PhD student Lauren Treiman to understand how human behavior changes in training AI
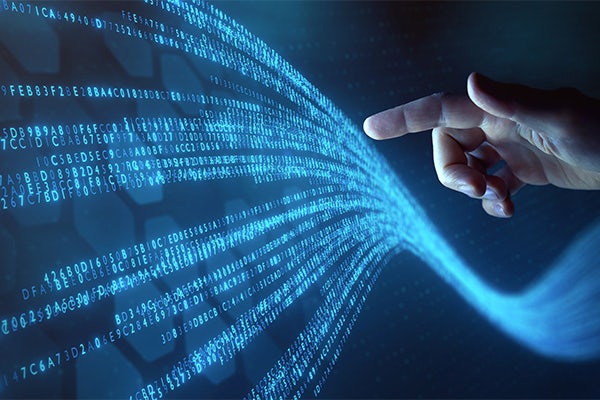
A new cross-disciplinary study by WashU researchers has uncovered an unexpected psychological phenomenon at the intersection of human behavior and artificial intelligence: When told they were training AI to play a bargaining game, participants actively adjusted their own behavior to appear more fair and just, an impulse with potentially important implications for real-world AI developers.
“The participants seemed to have a motivation to train AI for fairness, which is encouraging, but other people might have different agendas,” said Lauren Treiman, a PhD student in the Division of Computational & Data Sciences (DCDS) and lead author of the study. “Developers should know that people will intentionally change their behavior when they know it will be used to train AI.”
The study, published in PNAS, was supported by a seed grant from the Transdisciplinary Institute in Applied Data Sciences (TRIADS) , a signature initiative of the Arts & Sciences Strategic Plan. The co-authors are Wouter Kool , assistant professor of psychological and brain sciences in Arts & Sciences, and Chien-Ju Ho , assistant professor of computer science and engineering in the McKelvey School of Engineering. Kool and Ho are Treiman’s graduate advisors.
The study included five experiments, each with roughly 200-300 participants. Subjects were asked to play the “Ultimatum Game,” a challenge that requires them to negotiate small cash payouts (just $1 to $6) with other human players or a computer. In some cases, they were told their decisions would be used to teach an AI bot how to play the game.
The players who thought they were training AI were consistently more likely to seek a fair share of the payout, even if such fairness cost them a few bucks. Interestingly, that behavior change persisted even after they were told their decisions were no longer being used to train AI, suggesting the experience of shaping technology had a lasting impact on decision-making. “As cognitive scientists, we’re interested in habit formation,” Kool said. “This is a cool example because the behavior continued even when it was not called for anymore.”
Still, the impulse behind the behavior isn’t entirely clear. Researchers didn’t ask about specific motivations and strategies, and Kool explained that participants may not have felt a strong obligation to make AI more ethical. It’s possible, he said, that the experiment simply brought out their natural tendencies to reject offers that seemed unfair. “They may not really be thinking about the future consequences,” he said. “They could just be taking the easy way out.”
“The study underscores the important human element in the training of AI,” said Ho, a computer scientist who studies the relationships between human behaviors and machine learning algorithms. “A lot of AI training is based on human decisions,” he said. “If human biases during AI training aren’t taken into account, the resulting AI will also be biased. In the last few years, we’ve seen a lot of issues arising from this sort of mismatch between AI training and deployment.”
Some facial recognition software, for example, is less accurate at identifying people of color, Ho said. “That’s partly because the data used to train AI is biased and unrepresentative,” he said.
Treiman is now conducting follow-up experiments to get a better sense of the motivations and strategies of people training AI. “It’s very important to consider the psychological aspects of computer science,” she said.
Treiman LS, Ho CJ, Kool W. The consequences of AI training on human decision-making. Proceedings of the National Academy of Sciences (PNAS) Aug. 6, 2024.DOI: https://doi.org/10.1073/pnas.2408731121
Click on the topics below for more stories in those areas
- Graduate Students
- Computer Science & Engineering
Faculty in this story
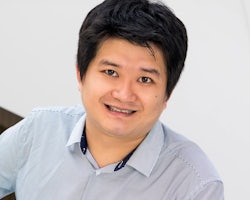
Chien-Ju Ho
Assistant Professor
You may also be interested in:
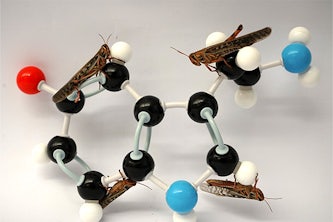
Studying how serotonin alters locust’s sense of smell
Raman lab studies how sensory neural networks process olfactory cues to support different behaviors.
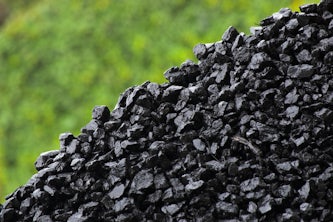
Sustainable technology to extract critical materials from coal-based resources
Young-Shin Jun to develop novel technology to extract, recover and enrich rare earth elements from coal-based resources.
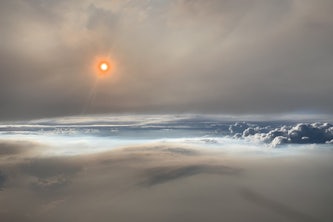
WashU researchers quantify solar absorption by black carbon in fire clouds
New findings from Chakrabarty lab will help make climate models more accurate as massive wildfires become more common.
Follow U of T News
What makes a chess move brilliant? Researchers use AI to find out
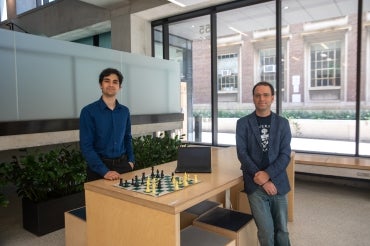
U of T Engineering researchers Kamron Zaidi, left, and Michael Guerzhoy, right, use game trees and deep neural networks to enable chess engines to recognize brilliant moves (photo by Safa Jinje)
Published: August 7, 2024
By Safa Jinje
Researchers at the University of Toronto have designed a new AI model that understands how humans perceive creativity in chess.
In a recent paper presented at an international conference, researchers in U of T’s Faculty of Applied Science & Engineering describe how they used techniques such as game trees and deep neural networks to enable chess engines to recognize brilliant moves.
The development could lead to chess engines that can find the most creative and clever path to victory in game, rather than just making moves to maximize win rates. That, in turn, could have implications for other AI systems tasked with creative endeavours.
“A chess move can be perceived as brilliant, or creative, when the strategic payoff isn’t clear at first, but in retrospect the player had to follow a precise path in gaming out all the possibilities to see so far into the future,” says paper co-author Michael Guerzhoy , an assistant professor, teaching stream, of mechanical and industrial engineering and engineering science who wrote about the research on his Substack .
“We wanted our system to understand human perception of what constitutes brilliance in chess and distinguish that from just winning.”
Most of the current research into chess AI is focused on enabling moves that create a higher chance of winning. But this doesn’t always make for an exciting game.
Skilled human chess players, on the other hand, can play in a more dramatic or imaginative way by making moves that may break traditional rules –for example, sacrificing a piece in a way that may initially look like a mistake, but ultimately, paves the way to a win.
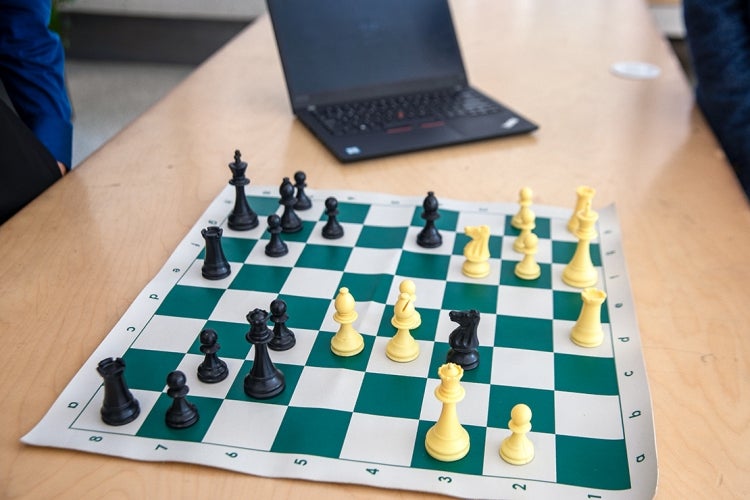
The team worked with Leela Chess Zero , a top chess engine that learns through self-play and has played over 1.6 billion games against itself. They also employed Maia , a human-like neural network chess engine developed by U of T computer science researchers.
“We used the two neural network chess engines to create our game trees at different levels of depth in a game,” says paper co-author Kamron Zaidi , a recent U of T Engineering graduate.
“Using these game trees, we extracted many different features from it. We then fed the features into a neural network that we trained on the Lichess database of online chess games, which are labelled by human users of the database.”
A game tree in chess represents the current state of a chess board along with all the possible moves and counter moves that can occur. Each board position is represented as a node and the game tree can be expanded on until the game is either won, drawn or lost.
The researchers began with small game trees then slowly increased the size, adding more nodes to the tree. They found that when the neural network looks at all the game tree features and makes a prediction as to whether the move is brilliant or not, it reached an accuracy rate of 79 per cent using the test data set.
The research – based on Zaidi’s undergraduate engineering science thesis, which was supervised by Guerzhoy – was presented at the International Conference on Computational Creativity in Jönköping, Sweden .
“There were people from all over the world presenting research on more traditional aspects of creativity, but we were all focused on the same thing, which is, ‘How can we use AI to enhance our interactions and understandings of creativity?’” says Zaidi.
The work has also received media coverage in outlets, including New Scientist , where English chess grandmaster Matthew Sadler says that a model that can understand brilliance could be used as a training tool for professionals and potentially lead to a more entertaining engine opponent for amateur players.
The team sees their system as having broad applicability when it comes to perception of creativity and brilliance.
“One of the biggest areas that is of interest to me is characterizing what we perceive as creativity,” says Guerzhoy.
“Not just in board games but in other creative endeavours, including music and art, where there is a formal framework and rules that need to be followed. Highly creative work involves planning in advance and gaming out the possibilities.
“But everyone I’ve talked to since the paper came out wants to know when they can play against our brilliant chess engine. So, I think making that possible is the obvious next step for us.”
Share this page
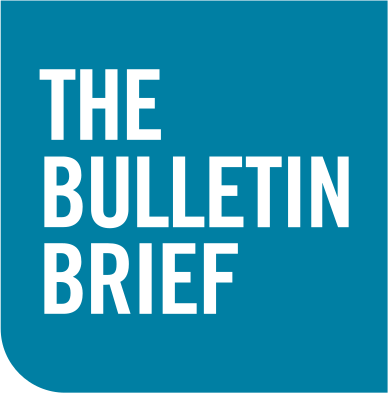
Subscribe to The Bulletin Brief
More u of t news.
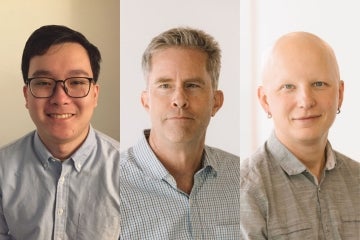
- Center for Teaching Excellence
- Location Location
- Contact Contact
- Offices and Divisions
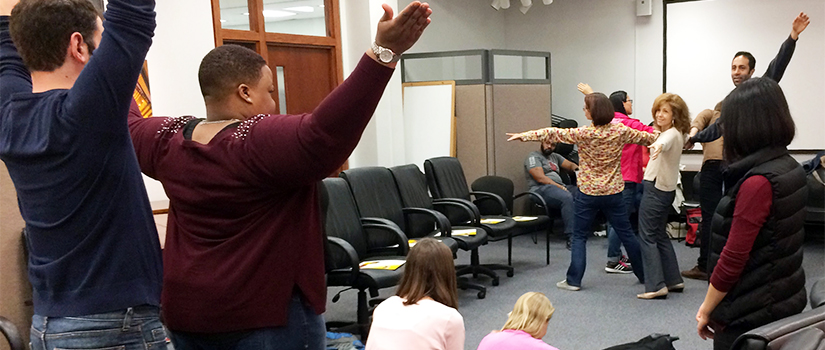
Our programs enhance teaching effectiveness through certificates of completion, grants, communities of practice, and other teaching development initiatives.
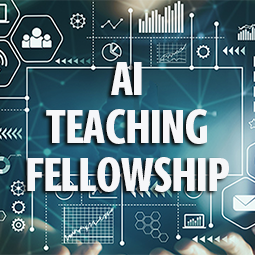
AI Teaching Fellowship
This intensive 12-month fellowship equips full-time faculty members with the expertise to leverage generative artificial intelligence (GenAI) for transforming teaching and learning.
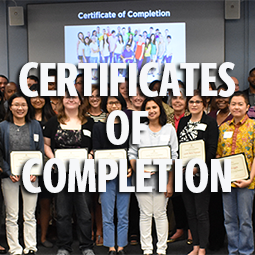
Certificates of Completion
Our certificates of completion, offered in partnership with other university offices, provide educators with a deeper understanding of a variety of professional development topics.
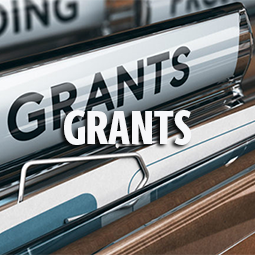
Our grants provide resources and support for educators to explore and implement innovative teaching approaches that will enhance student learning.
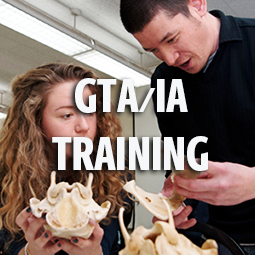
Graduate Teaching/Instructional Assistant (GTTA/IA) Training
All newly appointed GTA/IAs must participate in a two-part training program that includes GTA/IA Orientation and GRAD 701.
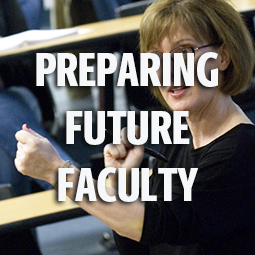
Preparing Future Faculty
Preparing Future Faculty (PFF) is a national professional development program that aids graduate students and postdocs in becoming college faculty.
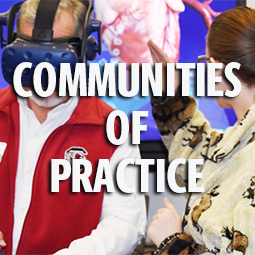
Communities of Practice
Communities of Practice allow for informal conversation and idea sharing among colleagues involved in a particular area of teaching.
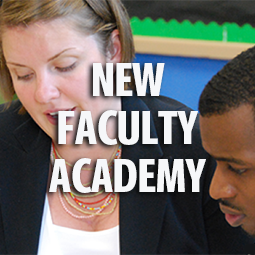
New Faculty Academy
New Faculty Academy is a series of professional development, networking, and mentoring sessions that launch new faculty into highly-productive careers.
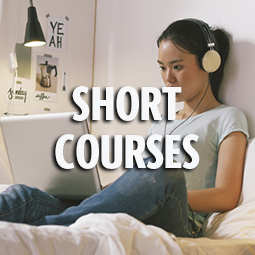
Short Courses
Short Courses provide opportunities for sustained and deeper engagement with teaching related topics.
Challenge the conventional. Create the exceptional. No Limits.

- Admissions & Aid
- Life at the iSchool
- Current Students
- Faculty and Staff
- Alumni and Friends
M.S. in Applied Human Centered AI Application Checklist
Here you’ll find everything you need to apply for our applied human centered ai program. and if you have questions, let us know. we’re here to help you..
The following application checklist is for those applying to the on-campus M.S. in Applied Human Centered AI .
If you have any additional questions that are not addressed in this checklist, please reach out to us at [email protected] and a member of our Enrollment Management team will respond.
Graduate Program Application
You can apply to the on-campus program using the Graduate Program Application .
You will receive an email from Syracuse University when your application has been received and processed.
$75 Non-refundable Application Fee
The non-refundable application fee is $75.
Please include a check or money order payable to Syracuse University. Do not send cash.
If you wish to apply to more than one Syracuse University program, you must file a separate application and application fee for each program.
Post-9/11 veterans of the armed forces may have their admissions fee waived upon verification by Veterans Resource Center staff. Identify yourself as a Post-9/11 Veteran while completing the online admissions application and contact the Veterans Resource Center ( [email protected] ) for further instructions.
Academic Credentials
One (1) copy of records of all previous postsecondary education.
Contact the Registrar’s Office of each higher educational institution that you attended and have one copy of your transcript(s) sent to the Syracuse University Enrollment Management Processing Center. Address and submission instructions are below.
You do not need to send transcripts from secondary schools as part of this application.
We can consider your application with unofficial transcripts, but we recommend that you send official transcripts. If you choose to submit an unofficial transcript, all offers of admission from Syracuse University are conditional pending receipt of official academic credentials showing that a U.S. bachelor’s degree (or equivalent) has been conferred upon you.
Failure to submit official degree-bearing credentials may result in revocation of an offer of admission, or dismissal from the graduate program if admission has already been granted.
Personal Statement
The personal statement is a statement of your general academic plans, personally written by you, the applicant.
Submit as an uploaded word-processing document through the online application.
In approximately 500 words, describe:
- Your main academic and personal interests
- Experiences in school or work that have helped to prepare you for this course of study
- Why you wish to study for the degree you’ve chosen
- Why you wish to study at Syracuse University
- Your plans for the future after you receive your degree
Two (2) Letters of Recommendation
The School of Information Studies requires two letters of recommendation for applicants to the master’s programs. Our requirement of two letters of recommendation is different from the general Syracuse University Graduate School requirement of three letters of recommendation.
You can request letters of recommendation, and your recommenders can submit their recommendations through the OnBase online application system. You will receive an email once your recommender submits their recommendation through OnBase.
If you are unable to request letters electronically through OnBase, you must mail letters to the Graduate Enrollment Management Center (address and mailing instructions below) in sealed envelopes, on which the recommender has signed across the seal.
Exam Scores
GRE General Exam score
- Due to the current issues related to the global pandemic, the submission of GRE Exam scores is currently optional for all applicants. If you take the exam, then you are encouraged to submit your scores.
- We welcome the submission of GMAT or LSAT scores in lieu of GRE scores.
Official TOEFL or IELTS score (international applicants only)
- All international applications are required to submit either a TOEFL, IELTS or Duolingo score. You may submit one score or the other; you do not need to submit all of your English proficiency examinations.
- Use the institution code 2823 when requesting ETS (Educational Testing Services) to send your scores electronically to Syracuse University. It is not necessary to request that scores be sent to more than one department.
- If you have completed a four-year degree (or higher) from a US based institution of higher education, or from select countries that are exempt from providing English language exam scores, it is possible to waive the TOEFL/IELTS score requirement. Email [email protected] to confirm your eligibility.
- International applicants whose native language is English, or, who are citizens of English-speaking countries are not required to submit TOEFL or IELTS scores.
- For more information on TOEFL scores, whether you need to submit your scores, and other common questions for international applicants to Syracuse University, please visit the International Student FAQ .
Resume or Curriculum Vitae
A copy of your up-to-date resume or curriculum vitae (CV) is required.
International Student Financial Documents
Funding Requirements for Financial Documentation
Financial Documents are not required for the review of your application for admission. Students may wait and submit financial documents after they receive admission.
The United States government requires international students to demonstrate sufficient funding for at least the first year of graduate study. Once you have done this, Syracuse University can issue the visa eligibility document (also known as an I-20) that you will need to have your student visa authorized.
- If you are a privately sponsored applicant , you will need to demonstrate acceptable evidence of your funding. This consists of a certified current bank statement on official bank letterhead, signed by an authorized bank official or an official letter stating an approved or sanctioned loan that indicates sufficient funds exist to meet at least first-year expenses in U.S. dollars as per Syracuse University’s current estimate.
- If you are a government-sponsored applicant , you will need to submit an original award letter (or a certified copy of an award letter). The letter must state the annual amount of the award in U.S. dollars. All financial documents must be written in English and valid within one year of the start of the semester. You may email the document per the instructions below.
Please contact Syracuse University Graduate Admissions at [email protected] for any questions regarding funding requirements.
Your financial documents have no bearing on your consideration for scholarships or awards. Program application reviewers do not have access to view your financial documents.
Applicants who choose to submit financial statements after an offer of admission is made may experience processing and delivery delays that can impact the receipt of the I-20 and the schedule of your visa appointment.
How to submit financial documentation
- By email: You can scan your financial documents and email them to Syracuse University Enrollment Management at [email protected] .
- By fax: You can fax your funding documentation to 001-315-443-3423
On all documents you send via fax, you must include: Your full name Date of birth Name of the program you are applying to Your SU I.D. number (this is issued to you after you submit your application)
Please visit the website of the Center for International Services for additional information.
Additional application submission instructions
Submitting your application electronically using the graduate program application.
We strongly encourage that all application materials and credentials be submitted electronically using the Graduate Program Application.
You will receive an email confirmation from Syracuse University when all your application materials have been received.
Submitting your application by mail
Any materials that cannot be submitted electronically can be mailed.
Any letters of recommendation that you are unable to submit electronically must be submitted in sealed envelopes, on which the recommender has signed across the seal.
For all other materials, you must include your first name, last name and SU I.D., and mail the materials in a sealed envelope to:
Enrollment Management Processing Center Syracuse University Graduate Admissions Processing P.O. Box 35060 Syracuse New York 13235-5060
If you are sending materials using a package delivery company (i.e. FedEx, UPS, DHL), use this address:
Enrollment Management Processing Center Syracuse University Graduate Admissions Processing 400 Ostrom Avenue Syracuse, New York 13244
To verify the status of your application, please contact Bridget Crary at [email protected] .
iSchool Success and Employability Policy for International Students
We are dedicated to supporting our international students’ success and employability. For this reason, we require that students with TOEFL scores below 100 or IELTS scores below 7.0 to take IST 678 – Communication for Information Professionals.
If you are a student who falls into this category, you will take an English assessment exam when you arrive on campus. If your exam score is high and indicates that this course would not be beneficial to you, then you will be given the option to not take IST 678 – Communication for Information Professionals.
IST 678 – Communication for Information Professionals is a three credit hour course that will not apply to the required credits for your academic program, but will apply to your grade point average (GPA). The iSchool believes that this course is very important to your academic and employment success, and you will not be charged tuition for taking this course.
- News Releases >
- New AI + Education Learning Community Series Launched by UB's Graduate School of Education
News Release
Published January 4, 2024
New AI + Education Learning Community Series Launched by UB's Graduate School of Education
BUFFALO, N.Y. – The Graduate School of Education at the University at Buffalo (UB) is launching the AI + Education Learning Community Series. In collaboration with the Institute for Artificial Intelligence and Data Science and the Center for Information Integrity at UB as well as the National Science Foundation/Institute of Education Sciences-funded National AI Institute for Exceptional Education , this series aims to create a collaborative platform for professionals in K-12 and higher education to better understand AI in education. Together, educational researchers, learning scientists, AI experts, K-12 educators and leaders and practitioners in related fields will explore the vast potential AI holds for personalizing learning and optimizing educational outcomes while addressing potential ethical and equity concerns.
Scheduled to occur every fourth Tuesday of the month via Zoom from 4:00 until 5:00 p.m., the series will kick off with the inaugural session "Introduction to AI and Its Use in Educational Settings." Over the subsequent months, a diverse array of topics will be covered, including leveraging machine learning for personalized education, ethical considerations of AI in education, data privacy and security, mental health and well-being innovations, learner engagement and much more.
"We are thrilled to provide a platform where education professionals can enhance their understanding of AI's implications in educational settings," said Suzanne Rosenblith , UB’s Graduate School of Education dean and professor. "This series will serve as a sandbox for practitioners and researchers enabling them to collaborate to better understand the promises and affordances of AI in educational settings.”
The AI + Education Learning Community Series is structured to cater to the multifaceted needs of educators, researchers and practitioners. It seeks to not only deepen the understanding of AI's role in education but also foster connections with potential collaborators and community partners.
"We envision this series as a catalyst for innovation and collaboration," added X. Christine Wang , professor and associate dean for research at UB’s Graduate School of Education. "By addressing critical topics such as educational equity, adaptive learning experiences, and immersive technologies, we aim to empower educators and students with the knowledge and tools necessary for the evolving landscape of education." The AI + Education Learning Community Series is open to all interested professionals in the education sector and related fields. Registration details and the full schedule of sessions can be found on the UB Graduate School of Education's website .
Series Schedule Overview:
- January (1/23): Introduction to AI and Its Use in Educational Settings
- February (2/27): Leveraging Machine Learning for Personalized Education/Special Needs
- March (3/26): Navigating Ethical Implications of AI in Education
- April (4/23): Ensuring Data Privacy and Security
- May (5/28): Enhancing Mental Health and Wellbeing through AI Innovations
- June (6/25): Improving Learner Engagement: AI and Humanization
- July (7/23): Synergizing AI with Teaching Strategies for Classroom Excellence
- August (8/27): AI in Assessment: Crafting Adaptive Learning Experiences
- September (9/24): Immersive Learning with VR and AR Technologies
- October (10/22): Bridging Formal and Informal Learning via AI
- November (11/19): Promoting Educational Equity and Accessibility via AI
- December (12/17): AI Literacies for K-12 Students and Teachers
Photo Assets for Publication
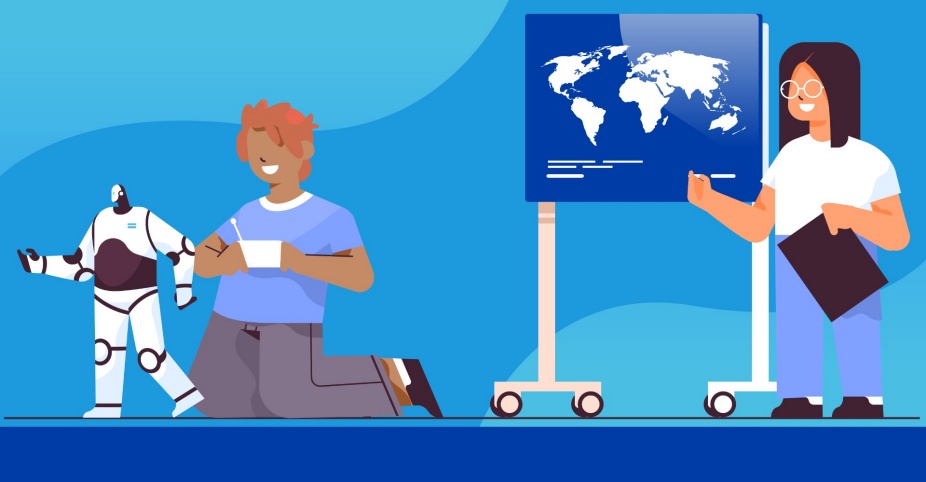
Media Contact

Amber M. Winters
Assistant Dean for Marketing and Director of Communications and Events
University at Buffalo Graduate School of Education
Phone: 716-645-4590
Email: [email protected]

COMMENTS
A doctoral dissertation that demonstrates original and advanced research in computer science. Program Length: 4 years for PhD after a recognized Master's degree. 5 years for Direct Entry PhD after a Bachelor's degree. Guaranteed Funding Period: 43 months if master's degree was completed in this department.
Students must successfully complete six graduate level courses (totalling 3.0 Full Course Equivalents (FCEs))* as follows: Three courses (1.5 FCEs) in the area of Artificial Intelligence. Two courses (1.0 FCEs) must be selected from the core list of AI courses (see list below). One course (0.5 FCE) must be chosen from additional AI courses ...
The University of Toronto is a world-leader in Artificial Intelligence (AI) research, including Machine and Deep Learning, Natural Language Processing, and computer vision, as well as the applications of cutting-edge technologies in areas such as human health, sustainability, humanities, engineering, finance, and e-commerce.
Our Artificial Intelligence faculty work in six sub-areas: Cognitive Robotics. Computational Imaging. Computational Linguistics and Natural Language Processing. Knowledge Representation. Machine Learning. Robotics. Acorn. U of T Main.
MSc and PhD Research Interests. Below is a listing of research areas represented in the Department of Computer Science. For some areas, their parent branch of Computer Science (such as Scientific Computing) is indicated in parentheses. Artificial Intelligence (AI) natural language processing (NLP), speech processing, information retrieval ...
e-mail: urtasun (at) cs (dot) toronto (dot) edu. tel: +1 (416) 946-8482. Raquel Urtasun is the Founder and CEO of Waabi . She is also a Full Professor in the Department of Computer Science at the University of Toronto and a co-founder of the Vector Institute for AI. From 2017 to 2021 she was the Chief Scientist and Head of R&D at Uber ATG.
Overview. In response to the rapidly evolving landscape of generative artificial intelligence (AI) use in academic and educational settings, this preliminary guidance has been produced to address frequently asked questions (FAQ) in the context of graduate thesis work at the University of Toronto.More detailed guidance on this topic, as well as new or updated policies may be issued in future ...
Ilya Sutskever Co-founder and Chief Scientist of OpenAI. I spent three wonderful years as a Research Scientist at the Google Brain Team. Before that, I was a co-founder of DNNresearch. And before that, I was a postdoc in Stanford with Andrew Ng's group. And in the beginning, I was a student in the Machine Learning group of Toronto, working with Geoffrey Hinton.
The Department of Computer Science at the University of Toronto has several faculty members working in the area of machine learning, neural networks, statistical pattern recognition, probabilistic planning, and adaptive systems. In addition, many faculty members inside and outside the department whose primary research interests are in other ...
The AI group. One of the eleven research groups in the Department of Computer Science, the AI group is the University of Toronto's main outlet for research in Artificial Intelligence. The group is further divided into 5 groups: ML, KR, CL, Vision, Bio .
From machine learning to computational medicine and biology, Artificial Intelligence (AI) is the future of healthcare. By establishing the Temerty Centre for Artificial Intelligence Research and Education in Medicine (T-CAIREM), we are integrating AI, such as machine learning, and analytics into our research and education.. Far from replacing humans at the forefront of healthcare and science ...
The Temerty Centre for Artificial Intelligence Research and Education in Medicine (T-CAIREM) launched this week at U of T, solidifying Toronto's place at the nexus of AI, data science and the health sciences. "Toronto is uniquely positioned to lead globally in artificial intelligence in healthcare," says Professor Muhammad Mamdani , who ...
Armed with vast data sets scientists in the field of Deep Learning and Artificial Intelligence in healthcare leverage techniques rooted in mathematics, statistics, computer science, and machine learning to develop novel algorithms and models that positively impact healthcare. This data encompasses a wide spectrum including medical imaging ...
Published: December 9, 2019. By Geoffrey Vendeville. Eight University of Toronto artificial intelligence researchers - four of whom are women - have been named CIFAR AI Chairs, a recognition of pioneering work in areas that could have global societal impact. One of the new chairs is Anna Goldenberg, an associate professor of computer ...
Apply for your Certificate. Upon completing your certificate requirements, you must request your certificate by submitting a Certificate Request Form. Are you ready to advance your career in IT, engineering, data management or technology? Then this leading-edge certificate program in Artificial Intelligence (AI) is for you. Through three ...
Since 2017, the Ethics of AI Lab at the University of Toronto's Centre for Ethics has fostered academic and public dialogue about Ethics of AI in Context —the normative dimensions of artificial intelligence and related phenomena in all aspects of private, public, and political life. It is interdisciplinary from the ground up, by pursuing ...
An interdisciplinary research group at the intersection of AI, data, and society. Part of Computer Science at the University of Toronto. About Research People Blog Datasets. ... We are recruiting graduate students to start Fall 2024. Apply to join us! Nov 26, 2021
An interdisciplinary research group at the intersection of AI, data, and society. Part of Computer Science at the University of Toronto. ... 3rd-year PhD. [email protected]; Jessica (Yi Fei) Bo Human-centered design of intelligent systems. 1st-year PhD ... University of Toronto 40 St. George Street, Room 4283 ...
Artificial Intelligence. June 21, 2024 With U of T innovators front and centre, Collision conference wraps up five-year Toronto run. June 19, 2024 Waabi, founded by U of T's Raquel Urtasun, raises US$200 million to launch self-driving trucks. June 14, 2024 AI safety, cybersecurity experts take on key roles at Schwartz Reisman Institute for ...
The proposed AI and Healthcare concentration aims to provide a training background for students who desire to enter the field as either medical experts or computer scientists/engineers. There is currently no program in Canada that is truly joint between the Departments of Computer Science and Medicine that would achieve the rigour required for ...
At the University of Toronto, we remain committed to providing students with transformative learning experiences and to supporting instructors as they adapt their pedagogy in response to this emerging technology. Many generative AI systems have become available, including Microsoft Copilot, ChatGPT, Gemini, and others. These AI tools use ...
I am a Senior Research Scientist at NVIDIA Research in the Toronto AI Lab.I earned my PhD in Machine Learning and Computer Vision from the University of Toronto under the supervision of Prof. Sanja Fidler.During this time, I was also affiliated with the Vector Institute for AI.In 2018, I completed my Master's Degree in Applied Computing at the same institution.
Entry into PhD program after completion of a bachelor's degree (i.e., direct entry): A four-year bachelor's degree in engineering, medicine, dentistry, physical sciences, or biological sciences, or its equivalent, with an average of at least 3.7 on a 4.0 grade point average scale (i.e., A minus) in the final two years of study from a recognized university; or
Similarly, the University of Toronto suggest instructors to: ask students to respond to a specific reading that is very new and thus has a limited online footprint; assign group work to be ...
Date Posted: 08/07/2024 Req ID: 36631 Faculty/Division: Dalla Lana School of Public Health Department: Inst of Health Policy, Mgmt & Evaluation Campus: St. George (Downtown Toronto) Position Number: 00056278 Description: About us: The Dalla Lana School of Public Health is a Faculty of the University of Toronto that originated as one of the Schools of Hygiene begun by the Rockefeller Foundation ...
A new cross-disciplinary study by WashU researchers has uncovered an unexpected psychological phenomenon at the intersection of human behavior and artificial intelligence: When told they were training AI to play a bargaining game, participants actively adjusted their own behavior to appear more fair and just, an impulse with potentially important implications for real-world AI developers.
Researchers at the University of Toronto have designed a new AI model that understands how humans perceive creativity in chess. In a recent paper presented at an international conference, researchers in U of T's Faculty of Applied Science & Engineering describe how they used techniques such as game trees and deep neural networks to enable chess engines to recognize brilliant moves.
AI Teaching Fellowship. ... Our certificates of completion, offered in partnership with other university offices, provide educators with a deeper understanding of a variety of professional development topics. ... (PFF) is a national professional development program that aids graduate students and postdocs in becoming college faculty. ...
Syracuse University Graduate Admissions Processing P.O. Box 35060 Syracuse New York 13235-5060. If you are sending materials using a package delivery company (i.e. FedEx, UPS, DHL), use this address: Enrollment Management Processing Center Syracuse University Graduate Admissions Processing 400 Ostrom Avenue Syracuse, New York 13244
The Graduate School of Education at the University at Buffalo (UB) is launching the AI + Education Learning Community Series. This series aims to create a collaborative platform for professionals in K-12 and higher education to better understand AI in education. Together, educational researchers, learning scientists, AI experts, K-12 educators and leaders and practitioners in related fields ...